Introduction
This case study involves a chartered organization by the US government called the Paralyzed Veterans of America (PVA). According to the case study, PVA is a philanthropic organization that focuses on serving the needs of American veterans who have suffered from spinal cord diseases and injuries (Stephenson & Taylor, 2021). Established in 1946, PVA raises money to support the Veteran’s healthcare advocacy, insurance cover, education, and research involving spinal injuries and diseases (Stephenson & Taylor, 2021). The organization raises money by sending mails, postages, and requests for donations through gifts.
However, most of their donation comes from direct mailings, where the organization sends greeting cards or free address labels to potential donors and requests for donations once the gifts are received (Hanks, Wright, & McKinnon, 2021). In 2016, the organization received more than $100 million from its donors. Out of this amount, $40 million was spent by the organization on postages (DeVeaux, Sharpe, & Velleman, n.d.). However, due to the solicitation of mail by the donors, the organization established that only a few donors responded.
Analysis Plan
Quantifiable Factors
As the name suggests, quantifiable factors are mainly used for financial analysis and help the company to make financial decisions. Consequently, PVA will use these factors to quantify the category of donors that is most cost-effective to the firm. In this case, the decision by PVA to use quantitative factors is due to the large amount of funding involved and the large amount of those funds used in sending the mail. Thus, the company wants to quantify the donor group that will have optimal donations with an almost negligible cost. On the contrary, quantifiable factors are less critical when less money is impacted by the decision made.
Problem Statement
Paralyzed Veterans of America, as a chartered organization, is a non-profit making organization. The organization obtains funds from well-wishers to address health needs for armed forces veterans who are having issues with spinal injuries or dysfunction. The organization reaches donors by sending postage or mailings. However, the cost of sending these emails is ineffective as some of the recipients do not respond. For instance, out of $100 million of donations, the company uses an estimated $40 million on mail and presents- 40% of the donations. Therefore, the company has noted that its operations are not effective and sustainable.
To be more efficient, they would like to know how past giving and demographic variables of their donors affect future donations or whether any correlation exists between past giving and demographic variables with future contributions. Using this information, the organization will identify the specific class of donors that the company will focus on. For instance, a segment whose past giving is unsatisfactory should not be prioritized when sending mails and postages.
Strategy
An appropriate strategy that PVA can use is to create scatterplots that will be used to measure the GIFAMNT against the predictor variables. The assignment is founded on the assumption that through scatterplots and regression analysis, PVA can help solve the problem of how to reduce the cost of mailings by determining the most cost-effective donor group (Shao et al., 2017). Below are screenshots of the six significant variables.
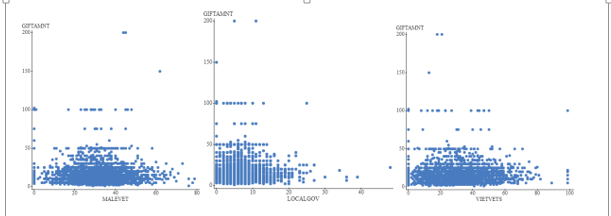
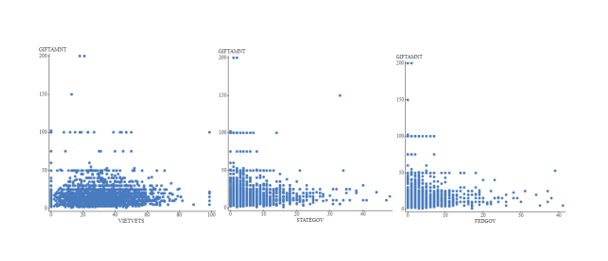
In creating the above scatterplots, each variable is first placed in a Statcrunch. The independent variable was placed on the X-axis since it was not affected by manipulation. In contrast, the dependent variable was placed on the Y-axis since it was affected by manipulating the X-axis (Pokhariyal, 2019). Scatter plots for all the future predictor variables were created, as shown above. As evidenced above, the first scatterplot shows the relationship between GIFTAMNT and MaleVets, which shows the average amount of gifts received from male veterans is slightly below $50.00.
The other five scatterplots can be analyzed in the same manner. From the scatterplots, the organization can determine which donors they will send mailings and postage to based on future donations and previous campaigns. Therefore, using Statcrunch is an effective way for PVA can perform regression analysis and predict future donation behaviors for different donor groups. Thus, the choice of scatterplots is based on the fact that the decision-maker can use the plots to determine the variables that have a significant positive impact on the topic of interest- such variables should be incorporated in future donations. At the same time, scatter diagrams will identify variables with a negative effect on the topic of interest and should thus be ignored. Consequently, scatterplots allow PVA to confidently determine factors that matter most (significant donors) and variables that should be ignored (less significant donors).
Statistical Methods
Statistical Methods
As noted in the earlier section, the importance of this investigation is to predict future donations by exploring the demographic and past contributions of various donors. Consequently, investigators can use different statistical tools for this prediction. The four statistical tools employed in this investigation are: StatCrunch, scatter diagrams, regression & multiple regression, and Excel Spreadsheet analysis. Scatterplots will be obtained through Statcrunch and help the decision maker determine the general direction of the trend between GIFTAMNT against the demographic factor under consideration.
The second statistical method is regression analysis which uses the general trend of the slope to come up with a regression equation. If the gradient of the equation is positive, then the variable is significant. However, the variable should be ignored if the gradient is negative. Consequently, by running a regression analysis, the PVA will likely predict the next group of donors and thus send mailings and postages without incurring costs (Foley, 2018). The first step in regression analysis is to run a correlation matrix test to see which of the 26 factors positively correlate to the GIFTAMNT and to what extent.
Furthermore, a multiple regression analysis is done using the p-values of each variable to determine if the factor will be included in the final regression equation (Foley, 2018). StatCrunch and Microsoft Excel are appropriate tools for this analysis since they comprise a collection of statistical and mathematical formulas that allow PVA to analyze large volumes of data. The Excel Data Analysis tool is a convenient tool to use in this analysis. The choice of Excel data analysis is that it can be used to filter or sort data and arrange data in ascending or descending order. Moreover, scatter plots and regression analysis can also be performed using Excel data analysis.
Justification of the Methods Used
StatCrunch
StatCrunch is Pearson Education’s web-based statistical software application for analyzing raw data. The software was created to be used in colleges offering statistics courses. However, with machine learning and extensive data analysis, StatCrunch has grown to a full-featured statistics package and is now used in financial analysis and other statistical analysis purposes. The reason for using StatCrunch is that it simplifies all the aspects of data analysis from presentation interpretation and also provides calculations based on the confidence level. The final outcomes are presented in a simplified table.
Consequently, the choice for the StatCrunch is due to the multiple variables that require stepwise regression when calculating the multiple regression. Excel does not provide this special feature. Furthermore, StatCrunch enables the decision-maker to describe data with its correlation. As such, PVA can use StatCrunch to determine the correlation between the demographic variables and past donations with the future amount. In this way, PVA can better forecast future gifts so that they can easily target the responsive segments. This way, mailing, and postage will be sent to individuals who will respond; thus, the campaign will be efficient, and the organization’s activities will become sustainable.
Scatterplots
Another statistical tool used in Milestone 1 is scatterplots. A scatterplot helps determine the relationship between two variables. This relationship can be between two variables, which can be positively, negatively, or not correlated (Mishra et al., 2019). The first variable is independent, and the second depends on the first. To analyze the relationship pattern, change the independent variable and observe the change in the dependent variable. A scatterplot can have two independent variables used to measure their relationship.
Therefore, the reasons for using the scatterplot are: First, the scatterplot helps determine how closely two variables are related and predicts the behavior of the dependent variable as a measure of the independent variable (Rentala, 2019). A reasonably good correlation between the two variables can be expected if the dots are very dense, such as very close. On the other hand, if the points are widely distributed, a poor correlation between them can be expected.
Secondly, scatter plots are easy to understand and interpret. If an uptrend goes up from the lower-left corner towards the top-right corner, then the relationship is a positive correlation or otherwise negative (Mishra et al., 2019). For instance, the correlation appears to be positively collated in the scatter diagram of GIFT AMNT against the MaleVets. In contrast, in the scatter diagram GIFT AMNT against the StateGov, the trend seems to move from the top left corner to the bottom right corner, thus showing a negative correlation.
Regression and Multiple Regression
It is important to note that scatter diagrams go in hand with the regression equation, where a positive gradient shows a positive correlation. In contrast, a negative slope shows a negative correlation. A zero gradient (a straight line) shows the absence of correlation (Plonsky & Oswald, 2017). For instance, in the scatter diagram of GIFT AMNT against FedGov, the projected regression equation has a zero gradient which means that the two variables are not correlated. Using the scatter diagram will help PVA to select a population with a positive correlation. For instance, in decision-making, PVA could prioritize MaleVets in postages and mailing as opposed to StateGov since a positive correlation maximizes returns.
Regression analysis is a quantitative tool for sorting variables that can have an impact. While using regression analysis, businesses can determine the factors or variables that are most important and ignore those deemed unimportant (De Menezes et al., 2021). For instance, PVA has 26 variables, some of which are important while others can be ignored. Using regression analysis and scatter diagrams, PVA will identify factors that will increase revenue collection and cut costs on expenditure. Regression analysis is a powerful tool that allows decision-makers to explore the relationship between two or more variables of interest. It is important to note that the importance of this assignment was to predict future behaviors based on the current demographic factors of the donor. Consequently, a key advantage of regression analysis is the ability to predict or forecast.
Regression involves predicting future opportunities and risks and is the most widespread application of regression analysis in economics. For example, Morrissey and Ruxton (2018) argued that predictive analytics could include needs analysis that tries to predict the number of goods a consumer will buy in the future. Using predictive analytics, PVA can analyze the future donation behavior of the targeted population using the derived regression equation (Sarstedt et al., 2021). For instance, given that the GIFTAMNT is the Y-variable and the MaleVets is the X-variable, it is possible to calculate the predicted GIFTAMNT by substituting the value of X in the regression equation obtained.
Microsoft Excel’s Data Analysis Tool
A fourth statistical approach that was applied in this assignment is the use of Excel data analysis through the application of spreadsheets. Spreadsheets are still relevant and are an excellent tool for studying data. In using Excel in this assignment, it is possible to organize data, evaluate, and calculate some essential elements such as p-value, mean, percentages, and plot graphs (Incerti et al., 2019). In addition, Blayney and Sun (2019) opined that Excel helped users create equations that allowed them to provide more data about essential business functions such as workflow, project performance, financial forecasts and budgets, and even inventory and utilization levels. Consequently, the ability to organize large amounts of data using Excel into logical and orderly spreadsheets and charts is also demonstrated in this task. Organized data is much easier to analyze and digest, primarily when used to create charts and other visual representations of data.
Using descriptive statistics from Microsoft Excel’s data analysis tool, data can be analyzed to determine where the wasted postal money is most likely coming from. It is possible to arrange the data based on alphabetical order using Excel sheet analysis or regression (Mishra et al., 2019). For instance, from the 26 groups that letters were sent to, it is possible to use an Excel sheet to find the average, median, and range of contribution, thus predicting the group that is most effective.
Data-Driven Decisions
Process
This investigation aimed to use statistical analysis to help PVA make decisions that will help the company’s operations to be sustainable and effective. In conducting this statistical analysis, a four-step process was used by the investigator as summarized below.
Step 1: Plan
Every process must start with a plan. Consequently, in conducting this statistical analysis, it is paramount that the investigator plans for various aspects. For instance, formulation of statistical questions or formulating hypotheses. For example, how can PVA predict future donations based on the trends of past GIFTAMNT received?
Step 2: Data Collection
The second step is to collect data. In this case, data will be collected based on how PVA receives GIFTAMNT from different donors. The data will be gathered based on male vets, Fedgov, age, etc. For this investigation, data will be downloaded from PVA’s website- a secondary data collection method.
Step 3: Data Analysis
This step organizes and summarizes the collected data using numerical or graphical methods. As noted in the above section, PVA could use four data analysis tools: StatCrunch, regression, scatterplots, and excel spreadsheet. For StatCrunch, PVA could select option ‘regression’ and then identify the variables, in this case, “Gift amount” and “Lastgift. After the data has been put, the summary of the output will be shown together with calculated ANOVA (analysis of variance) and Regression Analysis. The output will also show whether the data is significant or insignificant with respect to the selected variables. From our analysis, the value of R is 0.7 while p<0.05 shows a significant relationship between “Gift amount” and “Lastgift.
Step 4: Interpret the Result of the Analysis
PVA should use step 3 to interpret the findings and answer the research question. In this case, the results show a significant positive correlation between GIFTAMNT and past donations. Consequently, PVA can argue that one-time donations can be used to predict future gift amounts.
Step 5: Application of the Findings
It should be noted that the importance of this investigation was to help the organization make its operations efficient. Consequently, a statistical analysis of the company’s demographic variables based on past donations has been done, and interpretation made. A correlation between past and future contributions has been established in this case. PVA should use the findings to make its campaign efficient in receiving the results. For instance, the organization should focus on the segment with significant contributions and ignore the element with a meager and inconsistent contribution. In this way, PVA will make use of this report.
Data-Driven Decisions
Data Mining
Data mining is an essential step in research. It is the process through which hidden data is looked at, valid and valuable patterns of a dataset identified, and other helpful information also gathered. Data mining is mainly done during the literature review. Consequently, data mining is used by the investigator to discover unsuspected or unknown relationships among the data. PVA’s dataset is an example of metadata used in this case scenario.
Moreover, a regression analysis tool was used in this case study to uncover relationships and patterns between data. In particular, the regression analysis was used to determine the likelihood of a specific variable impacting future donations. The variables are veteran status, gender, age, homeownership, or gift amount. Data was also mined on the importance of statistical analysis in making financial-based decisions.
Structured vs. Unstructured
Structured data is defined as information that can be presented in the form of rows and columns where every column is a variable and observations are represented in rows. On the contrary, unstructured data is represented in various ways, including graphical representation, images, screenshots, or data images. In this application, screenshots will represent unstructured data, while charts will be used for structured data. Therefore, the mined data need to be converted to a format that readers can easily consume or in a manner that can easily be compared. Scatter diagrams will be used in this scenario to compare independent and dependent variables. The investigator will use regression analysis for structured data since it is straightforward in obtaining the response in the GIFTAMNT outcome. Consequently, PVA obtains a relationship between independent and dependent variables through structured and unstructured datasets, which helps the organization make informed decisions.
Variables
Variables play a significant role in data analysis. In this case, the focus was mainly on the relationship between GIFT AMOUNT and other variables, while the organization’s goal was to establish whether there existed a correlation between demographics and past giving behavior with future giving. Consequently, each of the six scatterplots presents a unique understanding of how each variable could help address the research question. On the scatterplots, each independent variable did not affect the X-axis and the dependent variable on the Y-axis. This indicates that the average amount of gifts a PVA can receive from male veterans is under $50.00. This scatterplot must be able to assist PVA in its determination (see MALE VETMALE VET variable). This indicates that the average prize amount a PVA can receive from a male veteran is under $50.00.
However, it should be noted that the independent variables in this investigation were 26 with 3635 observations in each variable. This a “big data” which could represent challenges in computation and comparison. For effective comparison, the investigator only focused on six independent variables drawn from the initial 26 variables.
Recommend Operational Improvements
Data-Driven Calculations
Through regression analysis, one shall attempt to show the relationship (whether dependent or not dependent) between the predictor and response variables. First, the analysis will run an independence test between the variables. Second, a regression test will be conducted to determine the dependent variable’s causal effect (Zhao & Hastie, 2021). The p-values are set to equal to <0.0001.
Since the used p-value is <0.05, one can conclude that donor characteristics and GIFTAMNT are not independent. Further, since the two are not independent, one can conduct a regression analysis between the two variables to predict future trends (Gunst & Mason, 2018). On the other hand, the calculated R square is 54.60%, meaning that more than half of the variation in donor characteristics is related to gift amounts (Karch, 2020). This means that PVA can predict the causal relationship between donors and their characteristics to predict future donation behaviors.
The table below represents ANOVA for the multiple regression model:
Table showing Analysis of variance table for the multiple regression model
Parameter Estimates
A table showing parameters of estimates
Summary of the Line of Best Fit
- MSE root = 8.14
- R-squared = 0.5460
- R-squared when adjusted = 0.5430
The calculation above means that R-squared is equal to 0.5460 or 54.6%. The value of R-squared is only greater than 50%, which shows a weak positive correlation between the variables. Despite the weak positive correlation, it means that 54.6% of the donors’ characteristics variation correlates with the GIFTAMNT. Thus PVA can use the stated variables to forecast future donations of the selected demographic variables.
Summary of Results
Since the organization wants to improve its operations by looking for efficiency in determining the correlation between past donations and demographic factors and their impact on future contributions, the analysis has shown a positive correlation. Consequently, the organization should prioritize the demographic variables that have shown consistency in higher contributions and give special attention to this variable by sending mailing cards and greetings. Indeed, the regression analysis has shown a relationship between donors and how much GIFTAMNT the organization receives from past donations. By analyzing this information, the organization can improve its efficiency in the donation and save some costs, such as mailing and buying greeting cards to insignificant donor segments. Instead, the organization would use this cost to focus on the new donor segment. Therefore, the organization can forecast its future donations in an efficient program through statistical analysis.
Solution
The regression model showed that 54.34% of the variance in donor characteristics was associated with the number of gifts. Therefore, it can be argued that PVA can track donors’ behavior through this regression analysis, thereby creating an efficient way of targeting these donors. The organization will prioritize the donors and determine who will receive personalized attention in its annual campaigns. Besides, the analysis suggests that by reducing the number of mail and postages, the organization will improve its fundraising performance by saving the amount spent on administration, buying gifts, and mailings. Indeed, if PVA can save on some of these costs, it will reallocate the funds to programs that directly benefit disabled veterans.
Conclusion
As noted in Milestone 1, PVA raises money in various ways, fundraising through direct mailings and postage campaigns. However, the challenge with this campaign is the high cost of shipping and posting the gift cards since PVA uses over $40 million to collect a donation of about $100 million. In assignment one, statistical methods were used to help the company make decisions on future contributions by using current information on donors’ behavior to predict future gift amounts.
In conclusion, this paper has demonstrated that the descriptive quantitative analysis tools used in this assignment are easy to understand, cheap, and provide accurate data. For instance, Excel spreadsheets are found in the Microsoft packages, while regression analysis is located in the Data Analysis Toolkit of an Excel spreadsheet. The availability and ease of application compelled me to use these tools over ANOVA and SPSS software, which is challenging to compute. Most importantly, the selected tools have allowed me to sort the data into different categories.
Looking at the data, a group stands out unfavorably for the mailers provided by PVA. Consequently, based on the three statistical tools, this paper demonstrates that PVA should prioritize MaleVets, LocalGov, and VietVets as these groups are predicted to have higher future returns based on scatter diagrams and regression equations. Furthermore, using the multiple regression analysis, the paper demonstrates that PVA can compare the returns from different donors and decide on the most cost-effective donor.
References
Blayney, P. J., & Sun, Z. (2019). Using Excel and Excel VBA for preliminary analysis in big data research.Advances in Data Mining and Database Management, 110-136. Web.
De Menezes, D., Prata, D., Secchi, A., & Pinto, J. (2021). A review of robust M-estimators for regression analysis.Computers & Chemical Engineering, 147, 107254. Web.
DeVeaux, D., Sharpe, N., & Velleman, P., (n.d.). Pearson MyStatLab. Pearson Publishers.
Foley, B. (2018). What is regression analysis and why should I use it? Web.
Hanks, M., Wright, A. E., & McKinnon, J. (2021). Wounded veterans in parks and the joint recovery team: American veterans in underwater archaeology.Journal of Veterans Studies, 7(1). Web.
Karch, J. (2020). Improving on adjusted R-squared.Collabra: Psychology, 6(1). Web.
Plonsky, L., & Oswald, F. L. (2017). Multiple regression as a flexible alternative to ANOVA in L2 research. Studies in Second Language Acquisition, 39(3), 579–592. Web.
Pokhariyal, G. P. (2019). Importance of moderating and intervening variables on the relationship between independent and dependent variables.International Journal of Statistics and Applied Mathematics, 4(5), 1-4. Web.
Rentala, S. (2019. Basics in nursing research and biostatistics. Jaypee Brothers Medical Publishers.
Shao, L., Mahajan, A., Schreck, T., & Lehmann, D. J. (2017, June). Interactive regression lens for exploring scatter plots. John Wiley & Sons.
Stephenson, P. L., & Taylor, M. V. (2021). Consumer health information for spinal cord injury and paralysis.Journal of Consumer Health on the Internet, 25(1), 102-111. Web.
Zhao, Q., & Hastie, T. (2021). Causal interpretations of black-box models.Journal of Business & Economic Statistics, 39(1), 272-281. Web.