Introduction
Banks and other financial institutions have an elaborate criteria used to approve or reject loans. The principle approval method is based on the ability of the loan applicant to repay the loan. To give these loans, banks rely on personal information which they synthesize in order to approve or reject the loan. The main factors affecting these loan approvals can be deduced by analyzing financial loan records. In this project, data provided by the lending club was analyzed to determine the main reasons for rejecting a loan application.
Statement of the problem
Most businessmen and individuals rely on loans granted by financial institutions to develop their business, homes and other ventures. In some cases a loan application may be rejected based on a person’s data and other information. Such rejections affect the applicant the applicant financially. Examining the main factors and issues that result to rejection of the loan is important as it will guide businessmen and individuals during the loan application process.
Research questions
In this project, the following questions were answered:
- Does an applicant resident state affect the loan rejection rate?
- Does an individual’s credit score (FICO score) affect the loan rejection rate?
- Does the employment length affect the loan rejection rate?
- Does the reason for applying loan affect the rejection rate?
Project objectives
The main objective of this project was to determine the factors that affect loan rejection rate. The specific objectives are
- To determine if an applicant’s residence state affect his loan approval
- To determine if the employment status affect the loan approval process
- To determine if income affect the loan approval process
- To determine if the loan title or reasons for applying the loan affect the rejection rate.
Hypothesis
The null hypothesis statement is: The state, income, loan title and employment details influence the rejection rate of a loan application.
Data collection methods
The data used in this analysis was collected from lending club website (Lending Club 1). Lending club brings together investors and borrowers by providing financial information to them. It assist borrower identify pertinent requirements that they must fulfill for a successful loan approval. The website provides information about particulars of the loan applicant and includes details on whether the loan was accepted or not. The data provided in this site is divided into approved loans and rejected loans. For this project, the researcher relied on rejected loans data in order to establish if there are patterns in the loan’s rejection rate.
Sampling method
Sampling can be regarded as a method of selecting some elements from the entire population. The selected sample must be a representative of the population. It is important that an appropriate sampling method is used to ensure that bias and other systematic errors are reduced. In this project, simple random sampling was used to select the sample. Simple random sampling is a probabilistic method of sampling where sample elements are picked at random from the population (Levy and Lemeshow 48). To carry out simple random sampling, the following procedure was used:
- Determination of the sampling frame: this is the entire population from which the sample was selected. In this case, the sampling frame includes all the rejected loans for the year 2013. In this sampling frame, there were 566665 individuals whose loans were rejected. The particulars of these individuals are also provided.
- Determination of Sample size: The sample size was computed from the sampling frame. It is the number of samples that need to be collected from the whole data. for large populations, the sample size is calculated using the formula n=Z²-pq / e², where n is the sample size, Z is determined from the normal table graphs by selecting a confidence level of 95% or 99%, p is the estimated proportion of the attribute to be measured, q=(1-p) and e is the precision level. For very large populations, the sample size is normally equal to 1100 (Israel 5).
- Random sampling method: though there are many techniques for random sampling, the 1100 elements were randomly picked from the population. To avoid repetition during the sampling, the already picked elements were shaded.
Data analysis
After collecting the data, data analysis was carried out. The steps followed during the data analysis process are outlined below (Creswell 19).
Data grouping and classification
The data collected from the sampling frame was mixed up. The first step as to group this data so that summary statistics. In this process, the data was arranged in the following groups
- The loan score rate
- The years an applicant has been employed
- The applicant state
- Loan title
Summary statistics
After grouping the data, summary statistics were drawn from the sample. For example, the applicants from each state were grouped together so as to try and identify a trend. This was done for all the groups.
Graphs
Bar charts and pie charts were used to represent information in the different groups. From these graphs, the researcher was able to deduce important patterns and trends in the loan rejection rates.
Computations of measures of central tendency
The mean and mode were computed in some cases to assist the researcher determine trends in the loan rejection rate.
Results
Effects of the borrower credit rating on rejection rate
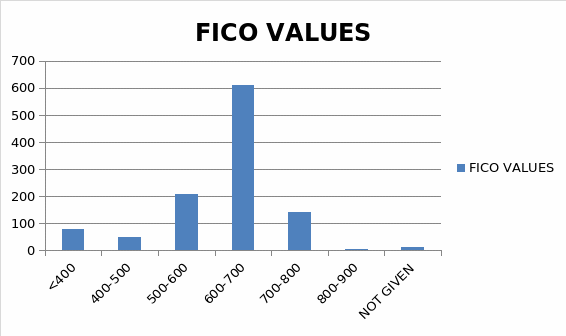
From the graph, it can be seen that applicants who had a FICO (borrower power) range of 600-700 received the highest rejection rates for their application. Checking from the past records, it can be seen that most applicants have an average FICO of 500 to 700. The computation of the FICO average is shown in table 1 below
Mean μ =fx/f = 656700/1088=603.5
Number of years in employment
The number of years that one has been employed is likely to affect the loan rejection rate. To determine this, the sample data was grouped based on the number of years an applicant has been employed. The pie chart in figure 2 shows these statistics
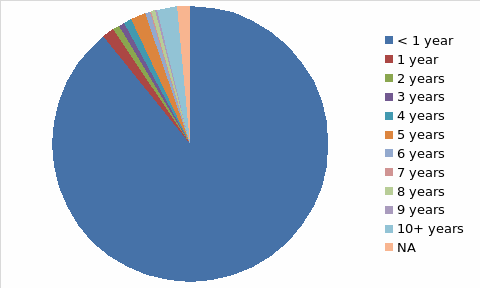
From figure 2, it can be seen that most loan rejection cases are those of employees with less than one year in employment. Out of a sample of 1100 rejected applicants, 981 had less than 1 year experience. This translates to 89% of the total sample elements. Most of these employees may still be in their probation stage and are not yet stable. Therefore, the lending institution rejected most of their applications.
Rejection rate based on the loan title/ purpose of the loan
The next objective was to determine if the loan application title or the purpose of the loan had an impact on the rejection rate. To do this, the reasons for applying the loan were grouped and summary statistics deduced. The pie chart in figure 3 below shows this information.
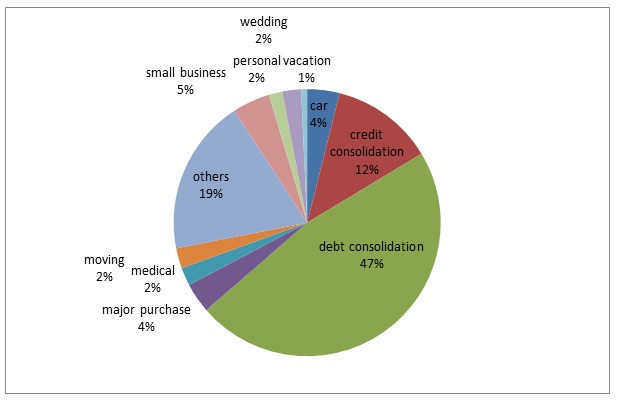
From figure 3, it was noted that 47% of all the rejected loan application were based on debt consolidation, 19% were based on the other reasons while 12% were based on credit consolidation. From figure 3, applicants giving credit and debt consolidation as the reason for applying the loan may not receive the loan as the rejection rate is very high.
Rejection rate among the different state
The next objective was to determine was whether the state of the applicant affected the rejection rate. The data in table 3 shows this information.
From table 3, it can be seen most application from California, Texas, New York and Florida were rejected. However, other states had less than 30 rejected applications. The analysis reveals that the applicant’s residence has an impact on the loan rejection rate. The difference in the number of rejected applications among different states could also be caused by the number of applications. For example, states with high population may have a high number of approvals as well as rejection rates while state with lower population may have a proportionately low approval and rejection rate.
Discussions
From the results obtained, it can be seen that the rejection rate for loans followed a particular pattern and was influenced by a number of factors. One of the major factors affecting the loan approval and rejection is the number of years that the applicant has been working. The data reveals most of the rejected loans are for applicants who have worked less than one year. This means that the employment length is a critical factor during the loan approval process.
Secondly, the state that an individual comes from affects the rejection rate. Most applications from California, Texas and Florida were rejected as compared to other states in the US. It can therefore be deduced that the applicant resident state affects the rejection rate. Other factors affecting the rejection rate were the level of income and purpose of the loan.
The major source of error in this project was during sampling. Random sampling used to select the sample from the population introduced sampling errors. The data source also came from one source and there was no means of validating this information. Future research should focus on comparing loan approvals with the rejected loans.
Conclusions
In conclusion, the data from lending club was analyzed to determine if there exist trends in the loan rejection rates (Lending club 1). Random sampling was used to select the sample from the large dataset. Data analysis of this dataset revealed that the loan rejecting rate is affected by the applicant employment period, state of residence, reasons for seeking the loan and income levels.
Works cited
Creswell, John. Research Design – Qualitative, Quantitative, and Mixed Methods Approaches. Thousand Oaks, California: Sage Publications, 2003. Print.
Israel, Glenn 2009. Determining Sample Size. PDF file. 2013. Web.
Lending club. 2013. Leading club statistics: loan data. 2013. Web.
Levy, Paul, and & S. Lemeshow. Sampling of populations: Methods and applications, New York: Wiley & Sons, 2008. Print.