IMBA Statistics
IMBA professional plus makes use of two major statistics to establish an association between envisaged and calculated data. These two statistics are the chi-square statistics, which is denoted by χ2 and the autocorrelation coefficient that is represented by the symbol ρ.
Chi-Square Statistics
The chi-square statistic test illustrates the extent of the association between the measured values and the envisaged values (Puncher 2007). The incorporation of the chi-square test into the IMBA software enables it to approximate the bioassay intake function. The chi-square scheme entails a cluster of distributions influenced by degrees of freedom. The degree of freedom is arrived at by subtracting one from the total number of the measured data points. Consequently, the chi-square probability (which is also referred to as the P value) can be established from the data using the degrees of freedom of the measured values. One of the most significant uses of the chi-square test is hypothesis testing. The following equation illustrates how the chi parameter is computed.
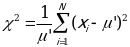
In the above equation, μ’ signifies the average of the experimental data while xi denotes the actual value in the group of data (Knoll 2000).
Small values of chi-square are obtained when P is greater than 0.95. Such small values of P show that there is a good relationship between the calculated and predicted variations in the data. In contrast, high chi-square values are obtained when P is smaller than 0.05. Such high values are signs of inadequate fits between the measured and expected variations in the data.
Autocorrelation Coefficient
The autocorrelation coefficient in a chain of events presents an indication of the lack of arbitrariness. For this reason, the autocorrelation coefficient is useful in uncovering inappropriate connections in the data. For a string of residuals, the autocorrelation coefficient is computed using the equation R=…………………. In this equation, Ri denotes the residual in the ith position in a succession of N residuals. The value of N must always be greater than 4 (Puncher 2007).
Provided that the tallying process presumes the evaluated qualms are disseminated in a log-normal fashion with an equal geometric standard deviation presupposed for each quantity, then each residual is computed as
Ri =…………… (Puncher 2007).
As a result, all values of ρ that are close to 1 are signs of inadequate fitting of models. The autocorrelation coefficient (ρ) is a statistical measure, which implies that the independent value of ρ does not supply adequate information on the subject of ‘goodness of fit.’ It is imperative for the statistic to be slotted into a test that determines the goodness of fit for it to yield meaningful information.
Several studies have looked into the ρ statistic as a valuable instrument for evaluating the goodness of fit following the tallying of biokinetic models with information on bioassays. These studies have brought to light the fact that the ρ test enables the unprejudiced detection of partiality in the succession of residuals following the fitting of a poor archetype to a series of data.
The autocorrelation coefficient test does not take into consideration the degree of miscalculations that are made by the evaluator. The chi-square (χ2) test, on the other hand, is susceptible to the slightest inaccuracies made by the evaluator. Another point worth noting is that it is impossible to employ the ρ test in instances where a given data array contains less than four values (Puncher 2007).