Introduction
In today’s modern world, computers play an important part in human lives. Developers from all over the world have invented different applications, which help users to perform tasks with ease. The applications range from those in the social field to those designed for educational purposes. Clark, Fox, and Lappin (2010, p. 65) observe that learning software has a number of functionalities. The uses include diagnosing and handling of errors, lesson tutoring, archival of documents archival, and language test.
One of the software developed to help students learn is Intelligent Language Tutoring Systems (ILTSs). According to Kumar (2011, p. 2), ILTSs are computer systems designed to provide learners with feedback and instructions in the absence of a teacher. According to Durlach and Lesgold (2012, p. 55), there are numerous types of ILTSs. Each of them has its pros and cons. The applications are used in different settings, such as in professional and formal education. In spite of the fact that there are a number of ILTSs, computer systems are designed to meet a common goal among learners. The primary purpose of the software is to reduce scholars’ over-dependency on tutors. Graf (2012, p. 78) observes that the applications deliver erudition materials to every student through the cyberspace channel.
To enable free input of sentences and phrases, Intelligent Language Tutoring Systems apply Natural Language Processing (NLP) practices. NLP is a component of artificial intelligence, computer science, and computational linguistics (Kumar 2011). It focuses on the relations between human and computer languages. Consequently, NLP is defined as the ability of computer applications to comprehend spoken speech in natural languages, such as English, and not in artificial forms, such as C++.
In this paper, the author will discuss in detail the relevant aspects of Intelligent Language Tutoring Systems and NLP. The writer will shed more light on different kinds of ILTSs, such as web-based German Tutor, auto-tutor, and CIRCSIM-Tutor. In addition, the author will discuss the aspects and characteristics of the Arabic Language in relation to NLP.
The rest of this paper is organized as follows: section two provides background on Natural Language Processing, Intelligent Language Tutoring Systems, and characteristics and aspects of Arabic Lingo. Section three of the paper will discuss the development and progression of Arab ILTS in comparison to other languages. Section four will focus on the future prospects of the study. Finally, section five will concentrate on the discussion and conclusion of the literature.
Background
Intelligent Tutoring Systems
The possibility of intelligence contraptions has been a subject of discussion for centuries. The notion of using intelligent machines for instructional application is traced back to 1924 (Fulcher & Jain 2008). It is the year when Sidney Pressey from Ohio State University developed a contrivance to instruct scholars in the absence of a tutor. The device, which resembled a typewriter, was fitted with a number of keys and a window for displaying questions. Nkambou, Bourdeau, and Mizoguchi (2010, p. 98) hold that the learning machine offered a response to students by recording their score on a counter. In spite of its major advancements and capabilities, Pressey’s device was not termed as intelligent. The reason was that the contraption was operated mechanically. In addition, the machine could only handle one question at a time. However, the device opened pathways for future projects.
Over the past decades, there have been major developments and improvements in Intelligent Tutoring Systems. According to Madureira, Reis, and Marques (2013, p. 102), highly advanced ITSs provide students with knowledge in a more interactive manner compared to the traditional method of learning. To improve the results, the systems incorporate a number of techniques. Such techniques entail consistent explanation, error detection, and correction. In addition, the software is designed to use different languages. Such languages include Arabic, German, and English. Lytras and Naeve (2006, p. 85) note that ILTS teaches natural lingo to first or second language learners.
Natural Language Processing
Natural Language Processing is a field of research that concentrates on the manner in which computers interpret and process natural human language. The history of NLP dates back to the 1950s. However, the most successful systems were created in the 1960s. According to Indurkhya and Damerau (2010, p. 112), the NLPs that were widely used at the time were SHRDLU and ELIZA. The former operated on limited blocks and worlds. On its part, the latter provided a more human-like interaction with learners. In addition, the system provided a standard response. For example, it could respond to “My stomach is aching” with “Why do you say your stomach is aching?”.
Major developments in NLPs were made in the late 1980s. The period saw the foreword of contraption learning algorithms for language processing. Reports from research studies reveal that NLP incorporates Natural Language Understanding (NLU) and Natural Language Generation (NLG). Researchers have explored a number of NLP functions over the years. The tasks range from stand-alone to large or development environment utilities (Rutkowski 2008). NLP has various limitations. However, the tasks are not restricted to tokenization, parsing, part of speech tagger, and segmentation.
There are different NLP applications that serve diverse purposes. The functions include speech recognition, tutoring, artificial intelligence, and machine translation. Stankov, Glavinic, and Rosic (2011, p. 105) hold that users require expertise in NLP to process and analyze huge amounts of text. In addition, there are two primary NLP techniques. They include Latent Semantic Analysis and Entailment.
Latent Semantic Analysis
Latent Semantic Analysis (LSA) is an NLP technique used to gauge the correspondence between two segments of a text. It is also applied to derive correlations between expected contextual uses of words in discourse. Over the years, LSA has been employed in a number of applications. Such applications include Auto-tutor. An Auto-tutor is used to appraise students’ responses during conversations (Evens & Michael 2006). One of the major advantages of LSA is its capability to categorize learners’ levels, such as good, vague, and erroneous.
Studies in LSA report that Latent is used to describe what has been written. On its part, Semantic refers to what was intended. For example, some phrases have more than one meaning in multiple contexts. On the other hand, there are instances where several words share a similar denotation. As an NLP technique, LSA is used to deal with such cases. However, the system has various restraints. For example, it lacks the ability to encode word order and detect negation (Woolf 2009).
Entailment
It refers to the task of determining whether or not two words in a sentence are interlinked. For example, it is used to detect if one phrase refers to the other. It is used in various applications, such as machine translation, information retrieval, and language translation (Champaign 2012). However, the technique has a number of constraints. One of the major limitations is the appraising of students’ responses in Intelligent Language Tutoring Systems. The restraint arises in cases where there are fixed answers. In such instances, Entailment has to figure out if the learner’s response matches the correct retorts. For example, learners may provide such answers as (a): Mary has a passion for poetry and music, and (b): Mary loves both domains. When this happens, Entailment has to decide whether or not (a) is similar to (b). To solve such challenges, various techniques have developed over time.
The table below depicts some of the means designed to tackle entailment problems:
Table 1: Addressing entailment problems.
Aspects of Arabic Language
Arabic is both challenging and important. According to Versteegh (2009, p. 106), the language is viewed as exigent due to its syntactic and morphological intricacy. It is also regarded as essential due to its rich history and heritage. The language is Semitic in nature. It has similarities to Hebrew, Phoenician, Aramaic, and Ugaritic languages. Current studies reveal that Arabic is spoken by over 330 million people from all over the world (Thurlow & Mroczek 2011). Given that it is a complex language, discretization plays an important part in ensuring proper disambiguation and pronunciation of words. As such, small diacritical marks are often used to embody three short vowels (a, i, u). On their part, letters (ݴ.ؼ.ى ) are used to represent vocal length. The diacritical symbols are positioned either above or below the letters. The reason for this is to point out the phonetic information linked with each letter and clarify the sense and meaning of the word. Obaidi (2007, p. 96) notes that there are noticeable discrepancies between spoken and written forms of Arabic words. In some cases, multiple words may have a similar orthography. For example, ابن may mean صبي
Arabic is an old language that predates Islam (Versteegh 2009). The fact is supported by the discovery of pre-Islamic Arabic writings dating back to the second and fourth centuries. In spite of the emergence of other languages, Arabic has managed to retain its originality for the last fifteen centuries. Some of the words that have remained unchanged include أمير amīr, الطوبة al-tūba, and قند qand + قندي qandī. As a result, persons with minimal knowledge of Arabic can read 1500 years old writings with ease. Over time, researchers have managed to classify the Arabic language into three types. The first is Classical or Quranic. The second is Colloquial. Finally, there is Modern Standard Arabic. According to Kumar (2011), Modern Standard Arabic “اللغة العربية المعيارية الحديثة al-lughah al-ʻArabīyah al-miʻyārīyah al-ḥadīthah” (p. 115) is the progressing variety. In addition, it borrows a lot from other languages. The reason is to cope with modern challenges. Classical “اللغة العربية التراثية al-lughah al-ʻArabīyah al-turāthīyah” is the formal version of the lingo. On its part, colloquial is the informal version spoken on a daily basis.
Over the decades, Arabic NLP has experienced major progression. The growth is confirmed by the creation and introduction of numerous applications in different domains, such as Machine Translation, Tutoring Systems, Information Retrieval and Extraction, and Text to Speech. To promote extensive development in Arabic NLP systems, researchers should focus more on characteristics and challenges affecting the lingo. One of the major issues for learners working with Arabic NLP is ambiguity. The uncertainty is experienced at different levels. A study by Landauer (2011) reveals that there is an average of 2.3 ambiguities for each symbol in most languages. When dealing with Intelligent Language Tutoring Systems, it is important to choose the correct evaluation of the student’s input. The reason for this is because picking a wrong analysis leads to flawed detection of mistakes. In addition, erroneous scrutiny may provide learners with the wrong feedback.
Efforts to Develop ILTS
Intelligent Language Tutoring Systems are applications that concentrate on the natural language of students. Zemp (2010, p. 103) notes that ILTSs have the ability to enhance efficiency. The systems achieve the goal by automating erudition processes. Applying NLP techniques into Intelligent Language Tutoring Systems has numerous advantages. One of the benefits associated with incorporating NLP into ILTS is the development of the right channels to analyze learners’ responses. The correct evaluations are made possible by the ILTS ability to detect text-input errors and offer instant feedback.
The development of Intelligent Language Tutoring Systems is similar to that of the instructional design process. The advancement is categorized into four primary stages. The phases are needs assessment, cognitive task evaluation, initial tutor execution, and evaluation.
A needs assessment is the initial phase of ILTS development. The stage is often the first in most instructional design processes (Xhafa 2010). The period is the first part of the advancement of the expert and learner domain. The primary goal of this stage is to provide a curriculum structure. The plan is developed by defining the tasks to be undertaken and the understanding students’ behaviors linked to the duties. To ensure the best curriculum is designed, three important aspects need to be looked at. The factors include the prospects of a learner to be able to tackle questions, duration taken to attain the highest performing level, and possibility that the student will use the acquired knowledge in the future.
The second advancement stage of ILTS development which is cognitive task analysis focuses on creating a valid computational model of the obligatory problem tackling comprehension. The primary methods of instituting a domain model are interviewing experts, carrying out protocol and think loud studies, and examining of tutors and learners behavior. The observation of instructors and students helps create a dialogue feature in intelligent tutoring systems (Woolf 2009).
The third stage of ILTS development referred to as initial tutor implementation entails creating a problem tackling setting. Such an environment facilitates an authentic learning process.
The evaluation which is the final stage of the ITLS development process is characterized by the pilot and parametric studies, formative analysis, and summative evaluations.
Development of Intelligent Language Tutoring Systems
To provide an in-depth analysis, this section will discuss a number of ILTSs that have been created over time for different lingos.
Auto-Tutor
An Auto-Tutor is a computer instructor that imitates the course patterns and educational approaches of a real human teacher (Stankov, Glavinic & Rosic 2011). The system works by initiating a discourse with the student using natural language. Auto-tutors have been implemented in different institutions across the continent to help in students learning. One of the notable institutes is the University of Memphis. The tutor was used as a Tutoring Research group to aid scholars in learning a computer literacy course. Champaign (2012, p. 104) notes that the device performs the duties of a human instructor by trying to understand inputs typed by students. Once an input has been made, the Auto-Tutor creates a dialog and provides corrections, feedback, and hints. Since the introduction of the first Auto-Tutor, there have been major developments in overtime. The modern machine is fitted with a 3D interactive interface.
To ensure effective learning, an Auto-Tutor asks a student a number of complex questions that require long texts of correct responses. The computer tutor is designed in a manner that each query is linked with a specific set of right and wrong answers (Durlach & Lesgold 2012). In order to be more useful to learners’, the contraption is built with the capability of correcting wrong responses and providing the right answers to students. The ability to differentiate expectations and misconceptions is made possible by the use of the LSA pattern matching technique. However, numerous experiments reveal, LSA is affected by a number of limitations. To counter the constraints, experts encourage the use of the Lexico-Syntactic approach. The technique works by concentrating on syntactic information while analyzing students’ responses.
CIRCSIM-Tutor
CIRCSM-Tutor is an Intelligent Language Tutoring System used in the medical field. The system uses natural language discourse with students’ through employing a number of tutoring plans (Evens & Michael 2006). The training tactics are meant to imitate human-to-human lessons. One of the places where CIRCSIM-tutor is extensively used is at Illinois Institute of Technology. The system is utilized to train the first year medical CIRCSIM uses natural dialogue for both output and input. The primary rationale for developing the system was to formulate pedagogically and linguistically sound wordings. CIRCISM as an ILTS is made of various components. The modules include a student model, input recognizer, problem solver, and screen manager. Other constituents are the knowledge base and text generator. In addition, CIRCSIM-tutors operate on four primary approaches (Clark, Fox & Lappin 2010).
The table below illustrates the four primary CIRCSIM-tutor tactics
Survey reports reveal CIRCSIM-Tutor functionalities are not affected by hints of flaws or constraints.
Web-based Intelligent Language Tutoring Systems
One of the Web-based Intelligent Language Tutoring Systems designed to help learners is the German tutor. The application is designed to form a syntax practice for offering a course in German through a web-based environment. According to Fulcher and Jain (2008, p. 82), the system is programmed to use German grammar and a parser that evaluates students’ input. To ensure effectiveness, the web-based ILTS maintains all students’ profiles and provides correct answers and feedback. In addition, the system has the ability to assign exercises. Graf (2102, p. 97) notes that one of the features behind Web-based ILTS success is its robust design. The web-server interrelates with learners via Java and CGI scripts. In addition, the system has a different server with a primary function of processing responses.
The figure below is an illustration of a German Tutor Architecture.
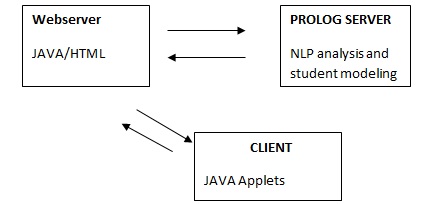
To confirm its effectiveness in helping students, research was conducted using 25 students over a one-hour class session. The findings revealed that 86% of the learners’ claimed the Web-based system was robust (Zemp, 2010). The reason for this is because it provided them with instant feedback enhancing their syntax practice.
Authoring Systems
Authoring Systems are ILTSs that allow actual tutors to present their knowledge in natural language. Instructors can add information, spot, and correct errors without automation in attaining the task. To ensure the maximum effectiveness of Authoring Systems, researchers have developed and proposed different techniques.
The table below shows some of the techniques, constraints, and approaches created to ensure the proper functioning of Authoring Systems.
Automated Language Tutoring Framework
The Automated Language Tutoring Framework operates on a string search algorithm designed to extract word patterns from question lists. The tutoring system helps non-native speakers of the set lingo to get enough language efficiency with ease (Graf 2012). One of the primary reasons for developing the Automated Language Tutoring Framework was to tackle the constraints affecting earlier systems such as REAP. Survey reports reveal REAP did not facilitate appropriate interaction between the system and learner. To counter such problems, the Tutoring Framework has integrated features such as instant and coherent response. The facets are incorporated with the multimedia aptitude to allow interactive voice feedback. In addition, the feature enables the evaluation of text input from the student. The functionality of the system is made possible by the utilization of auto-generated letterings developed by the use of authoring tools.
According to Landauer (2011, p. 113) Automated Language Tutoring Framework is incorporated with an ANTLR parser. ANTRL is used to formulate parse trees in accordance with the grammatical organization of students’ answers. The technique enables detection analysis of syntactic, contextual, and semantic inaccuracies in responses. Automated Language Tutoring Framework has an average rating of 94% based on its capabilities (Madureira, Reis & Marques 2013).
Extracting Learning Concepts from Educational text in an ITS
The Intelligence Learning System was designed to differentiate between mathematic contents and concepts to be learned by students. The ITLS uses statistical language techniques and theoretical map modeling to formulate a catalog of concepts to be taught. The primary purpose of the statistical language model is to derive the linguistics attributes of a natural lingo (Champaign 2012).
To ensure effectiveness, the Extracting Learning Concept is made of ten corpora with two distinct mathematics subjects. The arithmetical language model aims to estimate the distribution of words in a text. The reason for this is to determine how regular a phrase appears in a sentence correctly.
Beetle II System
Beetle II is a tutorial discourse system designed to recognize and understand students’ inputs without many constraints. In addition, the system is programmed to provide learners with multiple tutorial models and discourse plans. Research studies reveal students engage with Beetle II via an interactive chat platform using a keyboard. To identify words with similar meanings, the system is fitted with a natural language dialogue parser.
According to Zemp (2010, p. 94), Beetle II was developed to determine if computers equipped with NLP techniques could support self-explanation. In addition, the system was designed to ask students to exemplify their answers in order to receive detailed responses. The capabilities ensure that learners get correct feedback without to their instructors.
Development of Arabic-based Intelligent Language Tutoring Systems
Over the past decades, numerous researches have been conducted focusing on the development of Arabic Language in Natural Language Processing (NLP). The results and findings of the studies have led to the progression and implementation of Arabic Intelligence Language Tutoring Systems. Initially, there were two proposed forms of Arabic ILTSs architecture. The two were prototype and expert systems based. Prototypes Arabic ILTSs are applications designed using a technology that is able to read Arabic syntax (Woolf 2009). Due to this, the systems have the capability of detecting syntactic Arabic errors. Expert systems based are created and used to formulate the rules of Arabic grammar. Through this, the expert systems help in the development of Arabic NLP applications.
The first Arabic Grammar Checker developed was by Shaalan. The application was named Arab GramCheck. Upon its creation and testing, the system was said to be more efficient compared to the Arab Syntax Checker entrenched with Microsoft word version. One of the primary advantages of the system is its ability to detect and solve complex grammatical errors. In addition, the application provides users with immediate feedback (Versteegh 2009). However, the responses are meant for standard Arab word processor users.
The next phase in the development of Arabic ILTS saw the creation of Arabic Intelligent Computer-assisted Language Learning (Arabic ICALL). The system was designed to teach primary school learners Arabic by utilizing complex NLP techniques such as syntax and morphological evaluators. Clark, Fox, and Lappin (2010, p. 110) observe that ICALLs provided students with feedback by matching their answers with retorts fed to the system. The technique of ICALLs entails helping learners to spot their errors and correct them with ease. In spite of their benefits, a number of ICALLs had various constraints. Some of the limitations associated with the systems include the inability to make deep error analysis and complex inaccuracies correction, and offering slow feedback. The found challenges led enhanced experts’ desire to develop more sophisticated Arabic grammar systems. The new Arabic NLP tools were fitted with more advanced error, morphological, and syntax analyzers (Graf 2012). Compared to the initial applications, the latest ICALLs allow online students to spot and rectify their errors without human tutor’s help.
The advancement of Arabic ILTS has to lead to the development of systems that have the capability to analyze responses of both well and ill learned students. The applications achieve the goal by evaluating each input from learners and providing a set of possible correct analysis (Kumar 2011). All the generated evaluations are sent to the disambiguation interface to determine the correct scrutiny in accordance with the set morphological attributes. The right analysis is used to determine possible students’ errors and provide immediate feedback.
Through extensive research over time, experts introduced a new Arabic ILTS referred to as Analysis and Feedback of Erroneous Arabic Verbs System. The new application works by generalizing findings made by the ICALL system. The extensive improvements of the software reveal there has substantial progress in Arabic NLP. According to Zemp (2010, p. 83), growth has been influenced by the efforts of Arabic speaking scholars. In spite of the considerable progress, there are various concerns that are not yet entirely resolved. One of the primary factors affecting Arabic NLP progress in terms of thorough error diagnosis is the lack of linguistic tools and resources.
Comparison between Arab, German, and English ILTSs
Over the past decades, there have been major developments in Intelligence Language Tutoring Systems. However, most of the systems developers have focused less on the Arabic ILTS. Studies reveal that there has been major progress in English and German ILTS compared to Arabic. Nkambou, Bourdeau, and Mizoguchi (2010, p. 102) are of the view that there are numerous applications designed to tutor students in German and English language. However, there are very few Arabic ITLSs. In addition, the Arabic systems suffer from numerous limitations such as failure to detect complex Arabic grammar errors.
In spite of the big development gap between Arab, German, and English ILTSs, all the systems designed to serve the same purpose. The primary goal of the applications is to enhance students’ learning without the help of a human instructor. Some of the applications designed to help students in the three languages include Arab Tutor, German Tutor, and Web PVT.
Arab Tutor
Arab Tutor is a Web-based ILTS used for teaching subjects in Arabic Language (Obaidi, 2007). The system merges the flexibility and aptitude of ITSs with the accessibility of World Wide Web applications.
German Tutor
German Tutor is an ILTS designed for teaching the German Language. The system utilizes the Wizard Pattern to help students move through tasks. “Weiter” in the application refers to the next.
Web PVT
Web PVT is an Intelligence Language Tutoring System designed to teach English passive voice. The system records concepts grasped by the student and the level of mastering (Madureira, Reis & Marques 2013). In addition, the application records errors in past interactions and provides the best solution for each inaccuracy.
Structure of Intelligence Language Tutoring Systems
Intelligent Language Tutoring Systems comprises of four primary components. The constituents are domain, tutor, student, and user interface model.
The domain model is also referred to as the cognitive or expert knowledge mold. The ILTS component is developed on ACT-R theory which tries to follow all the procedures necessary to tackle a problem. The model comprises of rules, notions, and solution-finding strategies of the domain to be learned (Stankov, Glavinic & Rosic 2011). The primary functions of the constituent are analyzing learners’ performance and acting as a source of professional knowledge. In addition, the component is used for detecting syntax errors.
The student model is considered to be an overlay on the domain model. Due to this, it is termed as the main constituent of the Intelligent Language Tutoring Systems. The reason behind this is because it focuses on learners’ affective and cognitive aspects. In addition, the model concentrates on the students’ development as the learning process progresses. Champaign (2012, p. 116) claim ILTS employs a process referred to as model tracing as students tackle their problems. In cases where the Student Model stray from the domain mold, the system detects and signals the occurrence of an error.
The tutor model receives information from both the domain and student models. The constituent then makes decisions about coaching strategies and actions. Anytime when using the model, a student can ask for guidance on what to tackle next. Fulcher and Jain (2008, p. 118) claim the model has the capability of detecting instances where a learner diverges from the set production guidelines. Once the system has recognized the deviation, it alerts the student on an instant to follow the right rules. Another feature of the tutor model is the ability to categorize learners’ progress in two ways. Each time a scholar uses a rule to tackle a problem, the system evaluates whether the student has learned the procedures or not. The tutor model guides students until their learned level reaches an average of 95%. According to Nkambou, Bourdeau, and Mizoguchi (2010, p. 78), the tutor model via knowledge tracing follows up learners’ progress from one problem to the next. At each step, the system creates a record of strengths and weaknesses in accordance to the production rules.
The User Interface model incorporates three forms of information vital in creating discourse. The three types are communication intent, dialogue content, and patterns of interpretation and action knowledge.
Principles of Intelligent Language Tutoring Systems design and development
ITLS has undergone major advancements over time. The progress and success of the systems have been facilitated by eight key principles that dictate their purpose (Xhafa 2010). The guidelines include the ability to communicate the goal structure vital in problem-solving, lessen memory working load, embody student proficiency as a production set, and provide instant feedback on errors. Other principles are the ability to change the grain dimension of instruction with learning, provide students with instructions in problem-solving contexts, and make successive estimations to the target skill.
Limitations of Intelligent Learning Tutoring Systems
Intelligent tutoring systems have proved to be effective tools for enhancing students’ learning. However, numerous studies reveal the systems are affected by a number of limitations. The drawbacks affecting ILTSs include
Intelligent Learning Tutoring Systems are expensive to create and put into operation (Woolf 2009). Numerous studies are made to ensure developers build affordable intelligent applications. However, the research period is considered to be the most expensive. The reason for this is because the phase necessitates cooperation and support of people across different fields such as computer science, interface design, artificial intelligence, and cognitive psychology. In addition, the stage requires the contribution of experts from different disciplines.
Practical issues in terms of authorities and sponsors’ proclivity are a major limitation of ILTS. Before a system is developed, there should be parties willing to implement it (Kumar 2011). In addition, the authority or financiers must offer the total support required to ensure the application passes through all the development stages without setbacks.
Most Intelligent Learning Tutor Systems use more finances and time to build than expected. Programming an hour of online instruction can take up to 300hours of development time for touring content (Zemp 2010). The creation of a Cognitive Tutor, for example, took development to an instruction time ratio of 200:1 hours. The time factor limits the number of ILTS that can be developed within various domains.
Over time, the pedagogy of instant response and hints series has been criticized by most experts. Pedagogy is the feature that enables the application to be intelligent (Rutkwoski 2008). However, human instructors cite that component does not facilitate in-depth learning in students. Numerous observations reveal some learners turn to hints before attempting to solve the problems on their own. In such cases, students are able to complete the task fast but do not learn anything.
Current and Future prospects
Numerous research findings reveal some aspects that can be performed by human tutors cannot be carried out by Intelligent Learning Tutoring Systems. Some of the areas which suffer from constant criticism and need improvement are dialogue and feedback. ILTSs do not possess the ability to interpret affective states of learners as compared to human instructors. Future research should focus on how to enhance such capabilities and make the systems more effective.
Human tutors have the ability to understand and differentiate students’ tonal variations during a dialogue (Thurlow & Mroczek 2011). Most of the intelligent systems do not have this capability. However, developers are striving to ensure they create applications that can simulate natural discourses and maintain continuous dialogue. Instant feedback and communication can facilitate the asking of specific questions and guide students through their tasks in a better way.
At the current time, experts are focusing on developing ILTSs which can understand and interpret different emotional states. Affective processes play an essential part in learning (Durlach & Lesgold 2012). Due to this, future ILTSs can be developed with the ability to read and students’ different signs of affect. The feature will create room for more effective learning. However, such a move can suffer from various setbacks. One of the primary challenges is that effects are expressed in different ways. Due to this, it will difficult for the systems to understand all expressions. In addition, ILTS developers can create more interactive systems with the ability to detect distraction and boredom. To help students counter monotony, the applications can be equipped with a re-engagement or fun feature.
Discussion and Conclusion
Intelligent Learning Tutoring Systems have and continue to play an important part in replicating a one-on-one teaching process with the learners. Natural Language Processing is a field that focuses on the interaction between human languages and computers (Clark, Fox & Lappin 2010). By integrating NLP techniques, ILTSs acquire the ability to analyze, understand, and process students’ input text. In addition, the systems are able to provide immediate and correct feedback.
In this survey, it has been discovered NLP has two primary techniques which are Latent Semantic and Entailment. On its part, Arabic Intelligent Language Tutoring Systems are still in the initial advancement stages compared to English and Germany ILTSs. However, great effort is being made to ensure progression and filling of the gap. Using Intelligent systems in educational settings helps students’ to gain a better learning experience. The erudition process is enhanced by ILTSs capabilities to detect input errors and provide instantaneous feedback (Grafs 2012).
References
Champaign, J 2012, Peer-based intelligent tutoring systems: a corpus-oriented approach, University of Waterloo, Waterloo, Ontario.
Clark, A, Fox, C & Lappin, S 2010, The handbook of computational linguistics and natural language processing, Wiley-Blackwell, Chichester, West Sussex.
Durlach, P & Lesgold, A 2012, Adaptive technologies for training and education, Cambridge University Press, Cambridge.
Evens, M & Michael, J 2006, One-on-one tutoring by humans and computers, Lawrence Erlbaum, Mahwah, New Jersey.
Fulcher, J & Jain, L 2008, Computational intelligence: a compendium, Springer, Berlin.
Graf, S 2012, Intelligent and adaptive learning systems: technology enhanced support for learners and teachers, Information Science Reference, Hershey, PA.
Indurkhya, N & Damerau, F 2010, Handbook of natural language processing, Chapman & Hall/CRC, Boca Raton, Florida.
Kumar, E 2011, Natural language processing, I.K. International Publishing House, New Delhi.
Landauer, T 2011, Handbook of latent semantic analysis, Routledge, New York.
Lytras, M & Naeve, A 2006, Intelligent learning infrastructure for knowledge intensive organisations: a semantic web perspective, Information Science Publishers, Hershey, PA.
Madureira, A, Reis, C & Marques, V 2013, Computational intelligence and decision making: trends and applications, Springer, Dordrecht.
Nkambou, R, Bourdeau, J & Mizoguchi, R 2010, Advances in intelligent tutoring systems, Springer, Berlin.
Obaidi, O 2007, Grammar of Arabic language, Shubhi Publications, Gurgaon, India.
Rutkowski, L 2008, Computational intelligence: methods and techniques, Springer, Berlin.
Stankov, S, Glavinic, V & Rosic, M 2011, Intelligent tutoring systems in e-learning environments: design, implementation, and evaluation, Information Science Reference, Hershey, PA.
Thurlow, C & Mroczek, K 2011, Digital discourse: language in the new media, Oxford University Press, Oxford.
Versteegh, C 2009, The Arabic language, Edinburgh University Press, Edinburgh.
Woolf, B 2009, Building intelligent interactive tutors: student-centred strategies for revolutionising e-learning, Morgan Kaufmann/Elsevier, Amsterdam.
Xhafa, F 2010, Computational intelligence for technology enhanced learning, Springer, Berlin.
Zemp, B 2010, Intelligent learning, Spiraldynamik Akad, Zurich.