Abstract
This study explores the use of non-stationary models, which can be considered characteristic and representative of specific acceleration time series. The ARMA tool is used to generate the three acceleration time series under study, the variances of which have been pre-stabilized. During the experiment, descriptive parameters are estimated for each series to characterize the series stabilized and the modulating recording function. Among others, the paper calculates maximum displacement plasticity demand and hysteretic energy values for systems whose rigidity is regarded as bilinear. The paper also estimates the spectral characteristics of demand as a function of time series model parameters.
Summary
General
To many engineering professionals, acceleration data in the form of time series are of high interest and value. These series are highly irregular in time-varying amplitude and frequency characteristics. All acceleration data are simply time series with nonstationary characters. Each acceleration time series is one realization of a nonstationary stochastic process. Two approaches are used to model the time series: a frequency domain approach and a time-domain approach. Most analysis has been based on the stationarity and nonstationary of the amplitude and frequency content of the acceleration time series. The early simulated acceleration time series were stationary stochastic processes where a time-invariant spectral density function was assumed to reflect the frequency content of the most intense part of the acceleration time series. Models with nonstationary amplitude were introduced later, and more recently, the time-varying nature of the frequency content has been considered with an evolutionary density function. Such representation is instrumental in describing the frequency content.
It is well known that the use of ARMA models to solve time-domain problems has been a trend in recent decades. The relationship between theory and practice has led to a practical technic to model acceleration time series using ARMA models, which are sufficiently flexible to describe a real acceleration time series. Linear and non-linear response spectra analysis indicate that the ARMA (2,2) process using ten simulated acceleration time series samples provides a reliable description of the information contained within the acceleration time-series records. The dependence patterns for displacement plasticity and hysteretic energy were also described.
Objectives and Approach
This study investigates the application of simple nonstationary ARMA models, which can be characteristics of acceleration time series. The primary objectives of this research are:
- Establish minimal stochastic acceleration models which effectively summarize the damage potential in the acceleration events.
- Examine system response parameters to ARMA model parameters.
- Propose a relationship between model parameters and response parameters.
In this study, measured records of acceleration time series are considered, namely Ain Defla (duration 25 seconds), Afroun (duration 80 seconds), and Dar Beida (duration (28 seconds). These acceleration time series are assumed to be a single realization from a nonstationary stochastic process that characterizes the acceleration involved. The paper uses a mathematical modeling approach in which ten simulated acceleration time series were created for each of the three directly measured acceleration time series. The parameter for the transfer between the actual model and the simulation model was the ARMA process’s characteristics.
Linear and nonlinear response spectra are used to validate the simulated acceleration time series obtained from the ARMA model used. In summary, three key conclusions were drawn from the time-series estimation of the acceleration of the initial models:
- Through simulated ARMA process systems in a time-based approach, good results can be achieved with a limited number of parameters.
- The damage potential and average response spectra can be sufficiently estimated and described through the use of an acceleration time series model. As has been shown, this not only corresponds to the event but also descriptively characterizes the realization of a sample of a whole population of such series.
- Finally, acceleration time series can be correctly described using ARMA simulations (2,2).
Concepts and Previous Studies
Basic Concepts and Review of Previous Studies
The existing modeling of acceleration time series as a stochastic process has been based on two approaches: a frequency domain approach and a time-domain approach. Most analysis has been based on the stationarity and nonstationary of the amplitude and frequency content of the acceleration time series.
Stochastic Models in the Frequency Domain
In acceleration time series engineering, much statistical modeling is concerned with acceleration time series models in which the observed data is analyzed using frequency-domain techniques. This approach involves conventional spectral analysis, or in general, simply using frequency domain expressions to calculate what is needed and related to the identification procedure (Jenkins and Watts [12]).
Most acceleration time-series records consist of a starting buildup phase, a nearly “constant intensity” phase during the strong oscillation, and a gradually decaying phase. Early studies considered modeling the nearly constant phase with a white noise process. The white noise process is usually obtained by generating a sequence of Gaussian independent samples of random numbers spaced at equal intervals and assuming a linear variation of ordinates in each interval (Clough and Penzien [12]). Other stochastic models for acceleration time-series records are based on filtered white noise or filtered shot noise, where the white noise is generated first and multiplied by a deterministic envelope function and then filtered. The time-multiplier envelope function was introduced to account for non-stationarity in amplitude and many suggested shapes were used (Shinozuka [11]).
Most filters used are simple 1-degree of freedom oscillators. The filtering process is obtained by solving the differential equation of the oscillator characterized by a natural frequency ω and a damping constant ε. These parameters are obtained from predominant spectral characteristics of accurate acceleration time-series records. A superposition of a large number of sinusoidal functions at different intervals with equal frequency difference ∆ω, in which the phase angles having a uniform probability density function was random, is weighted in combination using the power of filtered stationary white noise expressed through the spectral density. This procedure allows a successful simulation of time series acceleration. Alternatively, one can generate a white noise sequence with an average spectral amplitude over frequency equal to one and multiply the spectrum of the white noise with the specified spectrum of the acceleration time series and transform it back to the time domain.
The modeling of acceleration time series has been suggested by academics. In the frequency domain approach, three methods of simulation have been used based upon assumptions of (1) stationarity in the amplitude and frequency content, (2) non-stationarity in the amplitude only, and (3) non-stationarity in both amplitude and frequency content.
Simulation in the Time Domain
The time-domain approach concerned with using ARMA models to describe acceleration time series data is relatively innovative. For example, Kozin’s article [6] is an excellent methodological aid for evaluating the possibility of applying the ARMA approach to acceleration time series data. Acceleration time-series records are digitized uniformly at equidistant time intervals. This set of observations forms a discrete-time series. A model which describes the probability structure of a sequence of observations is called a stochastic process. Key classes of stochastic processes include autoregressive (AR), moving average (MA), and their combination (ARMA). The autoregressive model denoted by AR(p) is generally written as:
where () are constant coefficients, (
) is a sequence of equally random distributed independent Gaussian quantities, and (
) indicates the sequence of data investigated. This model is of order (p).
Another general linear model of time series analysis is the autoregressive moving average (ARMA) model. This model is obtained by adding a moving average (MA) component to the autoregressive (AR) component. It is defined by:
where () and (
) are constant coefficients, and (p, q) is the order of the model.
The model contains p+q+1 unknown parameters ,
,
,
which are usually estimated from data based on maximum likelihood and the order is based on the partial AR functions.
Previous Studies
The first work in modeling acceleration time series data as time series using linear models was completed by Robinson [14], Liu [45], and Kozin [7]. Robinson used a moving average process to generate artificial acceleration time-series records for experimental purposes. Liu studied and compared several models. ARMA models were specified as a potential approach to characterize acceleration time-series records. However, the concern of that paper was a general study and comparison of stochastic models. Kozin [38] proposed a continuous: nonstationary time model:
where a(t) and b(t) are polynomials,
∅(t) is a function obtained by a cubic spline fit to the envelope of the actual record, and w(t) is Gaussian white noise. The parameters are to be estimated from data using non-linear filtering techniques. Such techniques are extensions of the methods of recursive estimation of Kalman filtering. It was found that if the initial parameters were not close to the actual values, the recursive computation would be unstable. Since there were no convergence theorems about the non-linear method applied to estimate the desired parameters, Kozin used maximum likelihood estimators instead of ARMA models. Two theoretical papers by Nakajima and Kozin [10] and Kozin and Nakajima [5] discussed the general problem of convergence, and a fundamental theorem that guarantees convergence of parameters was obtained. The Akaike Information Criteria was extended to include nonstationary models such as the model proposed by Kozin [3] and [4] on the modeling of nonstationary time series, which has the following form:
where y(k) is the acceleration time series data, u(k) is white noise, and a(k), g(k) are time-varying functions estimated from the data. The coefficients a(k) were parameterized as a linear combination of discrete orthogonal functions.
Acceleration Time Series Process Models
Acceleration Time Series Process Models (ARMA)
The acceleration time-series event is a time-dependent phenomenon initiated by regular slippage along faults for which it is not possible to write a deterministic model that allows exact calculation. Thus, an acceleration time-series event is considered as a sample of the whole set of time series that could be generated by the stochastic process. Since acceleration time-series records show a highly irregular motion and have finite durations, they are modeled as a non-stationary stochastic process. As a general concept in this analysis, the damage potential is characterized by the properties of the population generated by the stochastic process model. Thus, ARMA (p, q) process model could be represented as follows:
where () and (
) are constant coefficients, and (p, q) is the order of the model. The model contains p+q+1 unknown parameters
,
,
,
which are usually estimated from data based on maximum likelihood and the model order is based on the Akaike information criteria (AIC) [Ref].
The Autocorrelation Function
To provide information about the choice of the order (p, q) of the ARMA process, which may be used to represent an acceleration time series record, it is essential to refer to the behavior of the theoretical autocorrelation function R(k). The theoretical autocovariance function, C(k), may be derived by multiplying Eq. 2.1 by and taking expectations.
where
.
]. The autocorrelation function R(k) is obtained by dividing Eq. 2.2 by C (0) and a similar form is obtained:
The fact that the values of are correlated only to white noise values up to time t-k implies that:
for k > 0
There are some differences in the nature of the function depending on the instrument chosen. Thus, for processes using AR, the function will take the form of an exponentially decaying decay or series of waves as lag K increases. Alternatively, for MA, when lag K reaches a specific order g, the function becomes equal to zero. Finally, when the ARMA process is used, when the first g-p lag is overcome, the function takes the form of a combination of exponentially decaying waves.
The Function of Partial Autocorrelation
Partial autocorrelation function should be understood as such a way of describing the time dependence of the series, when combined with the use of AR, it becomes possible to accurately identify the order and type of the model being estimated. For stationary autoregressive (AR) processes, the autocorrelation function is infinite in extent. Therefore, it is very convenient to describe the autoregressive process by the number of non-zero functions of the autocorrelations. The theoretical partial autocorrelation function P(k) could be found by the Yule-Walker equations (Box and Jenkins, [2]).
For the ARMA process, which can be characterized as a stationary and invertible one, the partial autocorrelation function P(k) s is dominated by a damped exponential and sine waves depending on the moving average parameters after the first p-q lags.
AIC Riteria
One of the essential difficulties encountered during the run was the qualitative and quantitative estimation of the parameters. Specifically, it was necessary to determine the number of parameters needed as well as their numerical values in order to reduce the gap between the simulation model and the real-time series. The problem is that the order of the model was previously studied (Akaike Information Criteria, Akaike [15]), while the estimation of its parameters is based on the least-squares method of a nonlinear function. Thus, Akaike has approached this problem using the maximum information criteria. If one considers independent observations entropy measure and Kullback-Liebler information criteria. Based on the AIC criteria for a stationary time series, the model to be chosen is the one that minimizes:
where N is the sample size and is the maximum likelihood estimate of the residual variance.
Modulating Function
Another difficulty in performing the simulation was estimating the variance or, in other words, the envelope function. The main problem was to quantify this function since, for given conditions, its use is critical. Thus, the variance controls the non-stationarity of the processes and also takes into account some statistical parameters, among which is the response of the structure or extreme values of acceleration. The variance of ( ), the acceleration time series data, considered as random variables, is given as follows:
The assumption that
has been commonly used in acceleration time series simulations. Ellis [16] used equally weighted two-second time windows with time intervals of 0.02 sec and estimated the variance as follows:
f(t) provides an approximate estimate of the modulating function. However, this approach does not have a criterion to distinguish between stationarity and non-stationarity data. For practical purposes, it is essential to characterize the variance function with a minimum number of parameters. In this study, a moving window of time interval equal to 0.5 seconds is utilized to determine the acceleration time series variance under question. This method to determine the variance is used in all three acceleration time series used. MATLAB is used to do all necessary calculations.
Parametric Envelope Function
For practical purposes, it is essential to characterize the variance function with a minimum number of parameters. One approach used by Kozin [4] to estimate the envelope function is to use a cubic spline interpolation that follows the irregularities in the acceleration time series. The spline is applied by fitting functions of the following form to a number of segments of the acceleration time-series records.
Continuity at the intersections of these functions is assured by imposing a condition of an equal slope. The need to account for a large number of parameters limits the use of the cubic spline, even though it can be an excellent tool for fitting a record. In general, a simple function with a limited number of parameters may be a satisfactory answer for the current problem, to use a model approximation. A smoothed function is used in this study of the form:
where , are constants found by fitting the function to the estimated variance using the least squares method. This function is effective in fitting modulating functions with narrow peaks.
Modeling Procedure
Determining the subset of models and the corresponding orders was realized through the necessary to compare the estimated AR and partial AR functions with the behavior of the corresponding theoretical AR and partial AR functions, respectively. Thus, a satisfactory estimate of the AR function R(k) for a time series with zero mean at lag k is given as follows:
where
It is essential to compute the variance of the estimated autocorrelation coefficients as a criterion to decide that the autocorrelation function is zero after certain lag K. Standard errors of the autocorrelation estimates is given by Bartlett [1]
The partial autocorrelation function may be estimated either by the Yule-Walker equations or by successively fitting autoregressive (AR) processes of order 1, 2,…,k. The Yule-Walker equations are obtained by substituting estimated autocorrelation coefficients R(k) obtained using Eq. 2.11 and solving for successive values of k = 1,2,…, K. Using the Yule-Walker equations may lead to problems when the parameters are close to the boundary values of the non-stationarity condition. Therefore, the use of fitting autoregressive processes of k orders is adopted to estimate the partial autocorrelation function. Again, the standard errors of partial autocorrelation estimates are needed to decide if specific values may be considered to be zero beyond some lag K. Quenouille [17] has shown that for an AR process of order P, the estimates of the partial autocorrelation function of order p+1 or higher are nearly independent. The variance is given by:
Based on the estimated and theoretical autocorrelation and partial autocorrelation functions, subclasses from the general ARMA (p, q) process could be selected for further investigation.
The selected subclasses of ARMA models are used to model the stationary time series obtained. The idea of maximum likelihood is usually used for the estimation of the parameters in the stochastic model. The maximum likelihood function is defined as the function associated with fixed observations Z, the variable set of parameters. In this study, the set of parameters refers to the p+q+1 parameters 〖() of the ARMA model. If the original observation time series (
), which is in our case the stabilized acceleration record being modeled by the ARMA (p, q) model. For a stationary invertible ARMA (p, q) model, it could be written the following:
with the assumption that , for
, and
, for
For any set of parameters , the values
could be calculated successively.
The a’s are assumed to be independent normally distributed.
For any given parameters , the probability distribution
is associated with a given data
. In this study, the likelihood function is Eq. 2.48. It is convenient to work with log-likelihood function
.
Application of ARMA Models
Models Adopted
In this study of acceleration time series, the choice of a model depends on the nature of the intended application. For design purposes, it is essential to employ the smallest possible number of acceleration time-series parameters in the analysis. The model adopted is the autoregressive moving average ARMA model used in conjunction with a parametric envelope function. From previous sections, it is understood that the acceleration time-series event is a non-stationary time series. As the first step in modeling procedures, the event is divided by the modulating function so as to obtain the stationary value of the series. The modulating function obtained is used to fit a smoothed parametric envelope function. A simple form for an event with a single peak is given by:
where , are constants found by fitting the function to the estimated variance using the least squares method. This function is effective in fitting modulating functions with narrow peaks. Subsequently, the stationary series is used to perform an estimation analysis of the model parameters.
Acceleration Time Series Modeled
It is worth noting that data from three acceleration time series were consistently used for this study. These included Afroun with 16000 data points (0.005-second digitization increment), Ain Defla with 5000 data points (0.005-second increment), and Dar Beida with 5528 data points (0.005-second increment). Thus, the critical difference between the series was the number of points to estimate. Shown in Fig. 3.1, Fig. 3.2, and Fig. 3.3 are the measured acceleration time series plots.
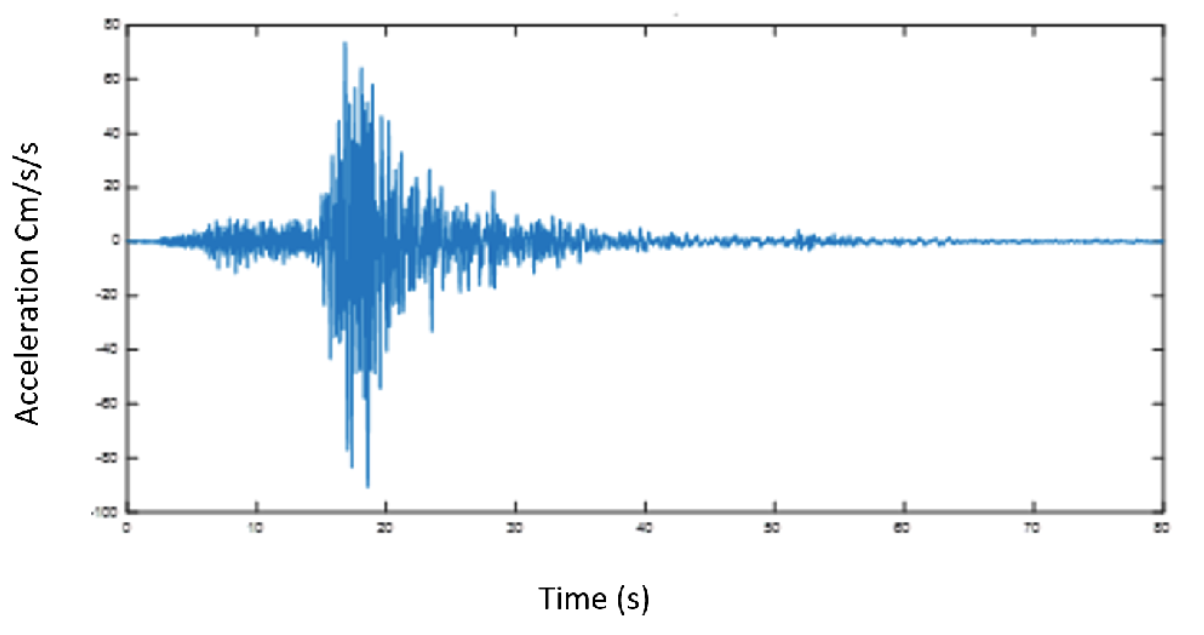
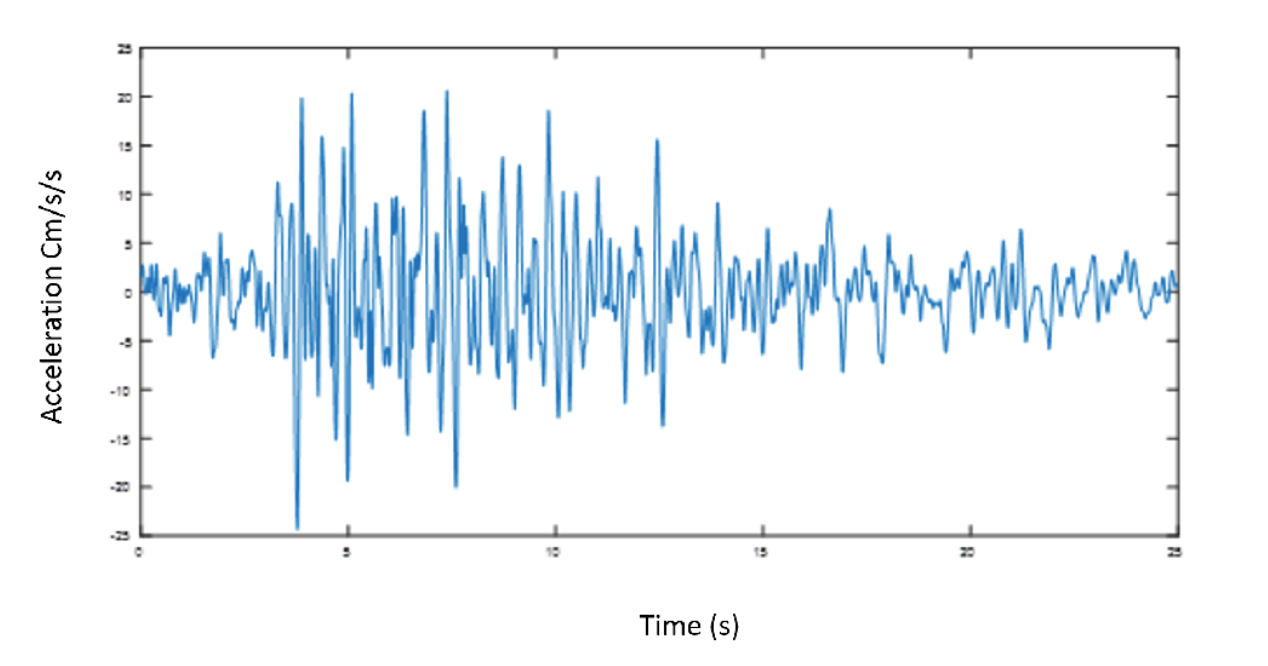
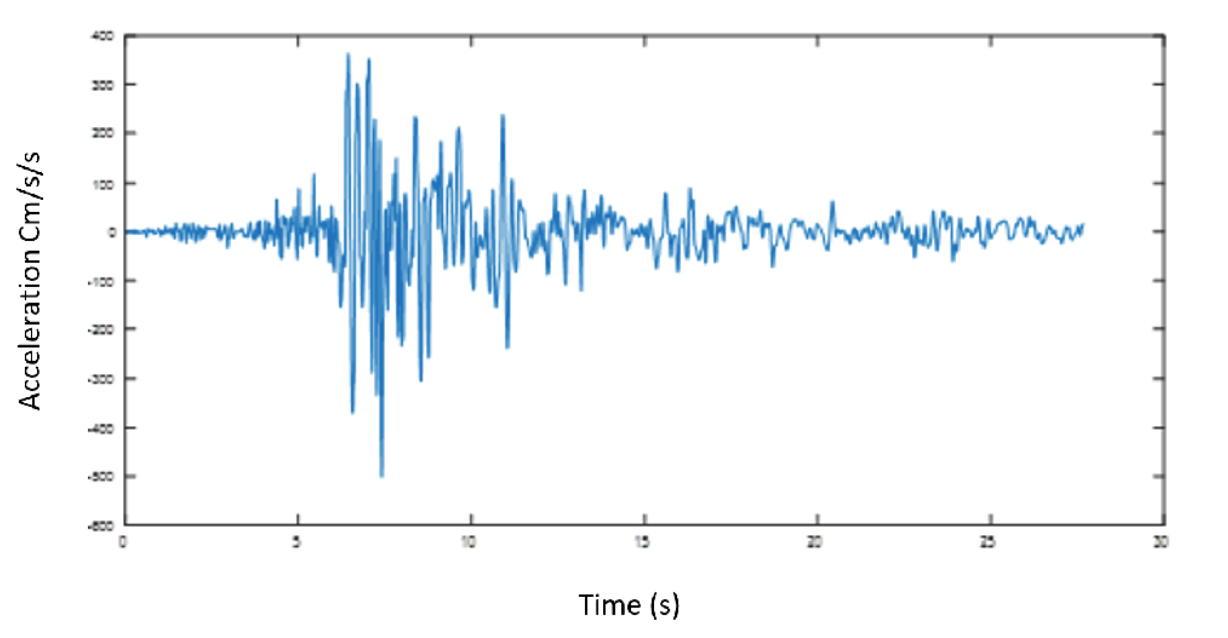
As the first step in model identification, the modulating function f(t) was computed for each measured acceleration record using Eq. 2.7 The results are shown in Figs. 3.4, 3.5, and 3.6. It could be concluded that the non-stationarity is significant in each event.
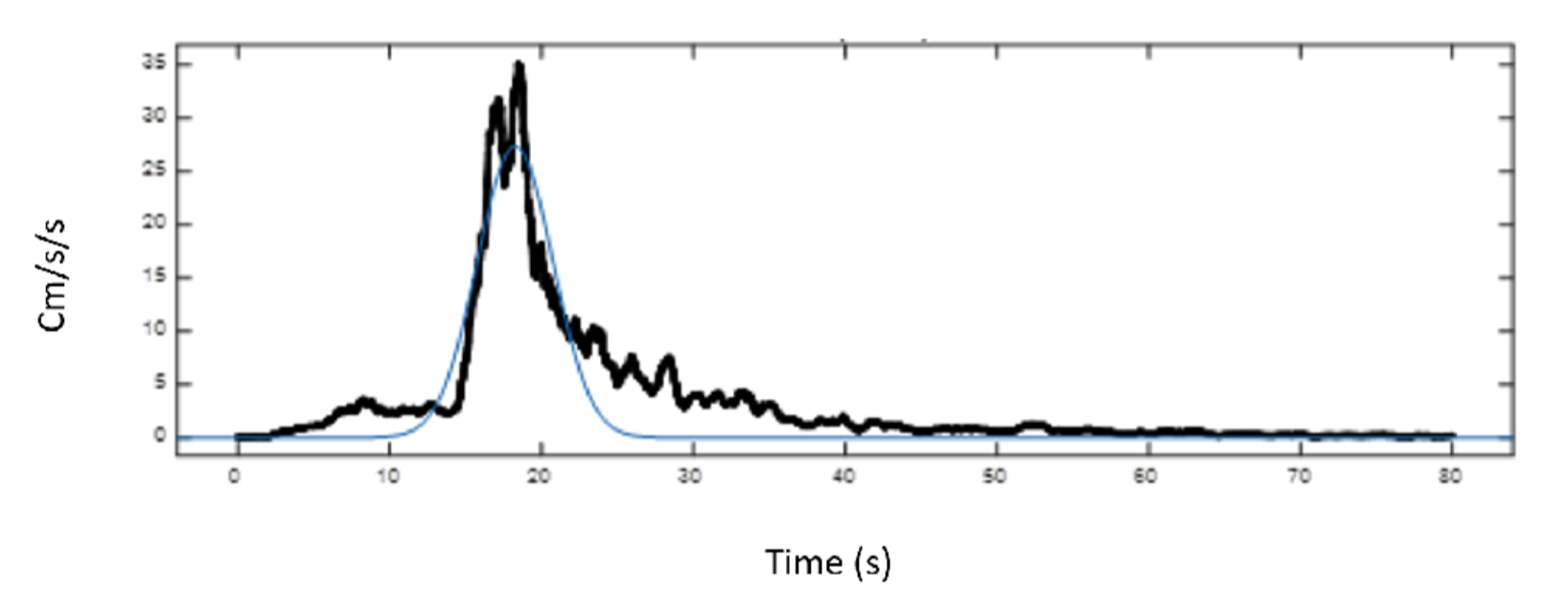
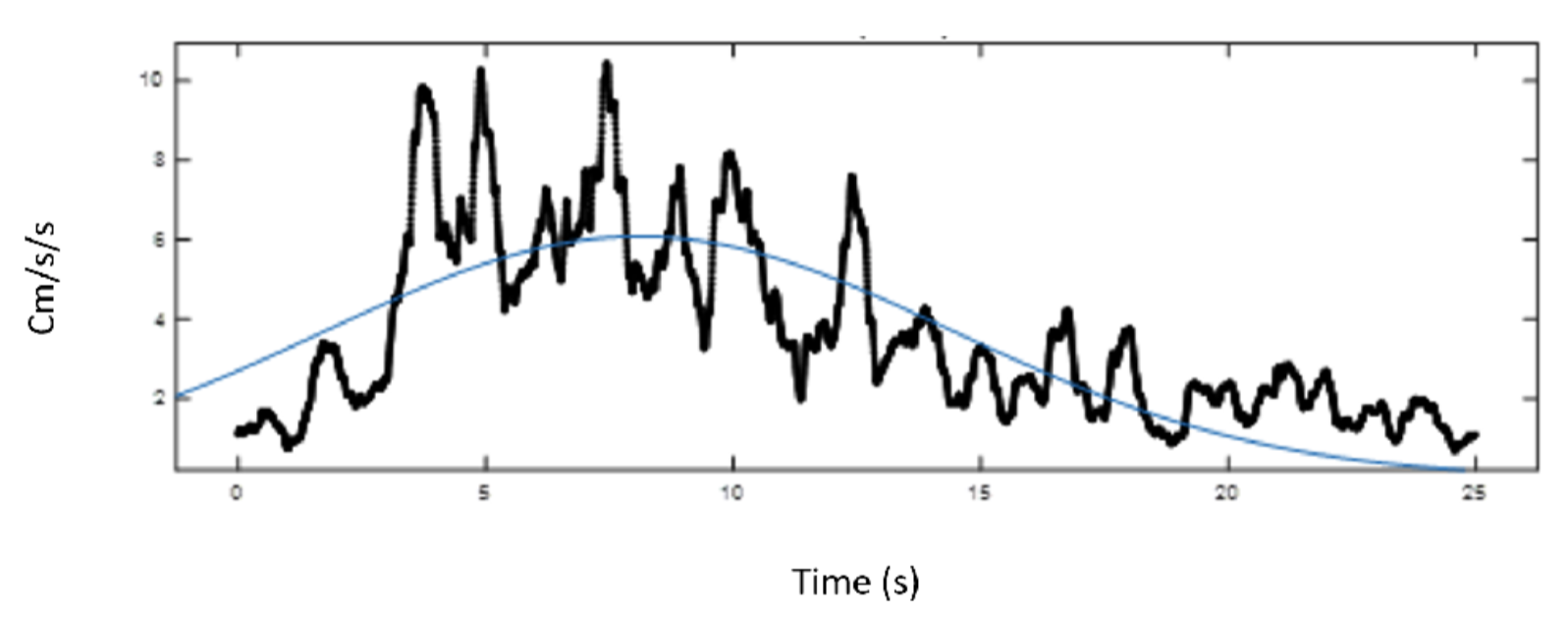
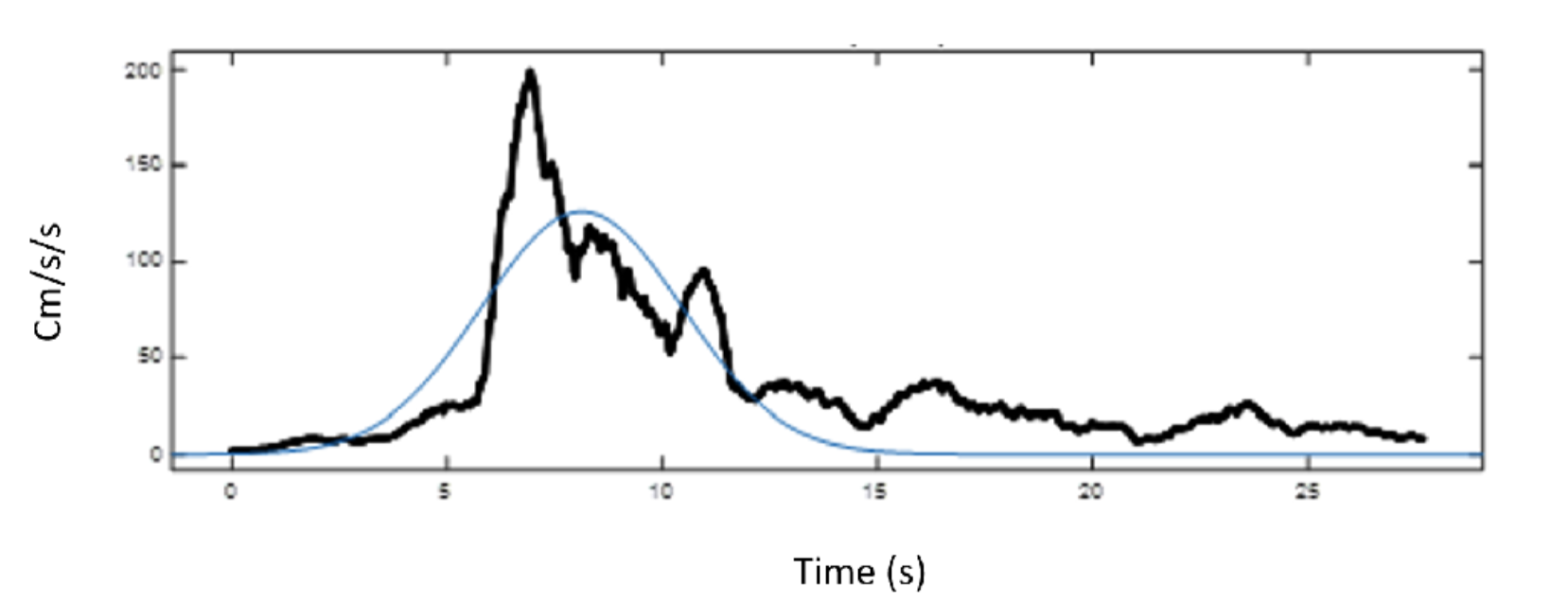
The one peak envelope function proposed in Eq. 3.1 is fitted to each of the modulating functions of the measured records using the least square method. Measured and fitted functions are shown for the four acceleration time series in Figs. 3.4, 3.5, and 3.6. The original acceleration record and the modulating function obtained are then utilized to estimate the stabilized (stationary in the broad sense) acceleration time series. The stabilized time series obtained for the three events are shown in Figs. 3.7, 3.8, and 3.9 The variance of the series is approximately one with zero mean value. The frequency content of each of the time series will be included in the ARMA model parameters.
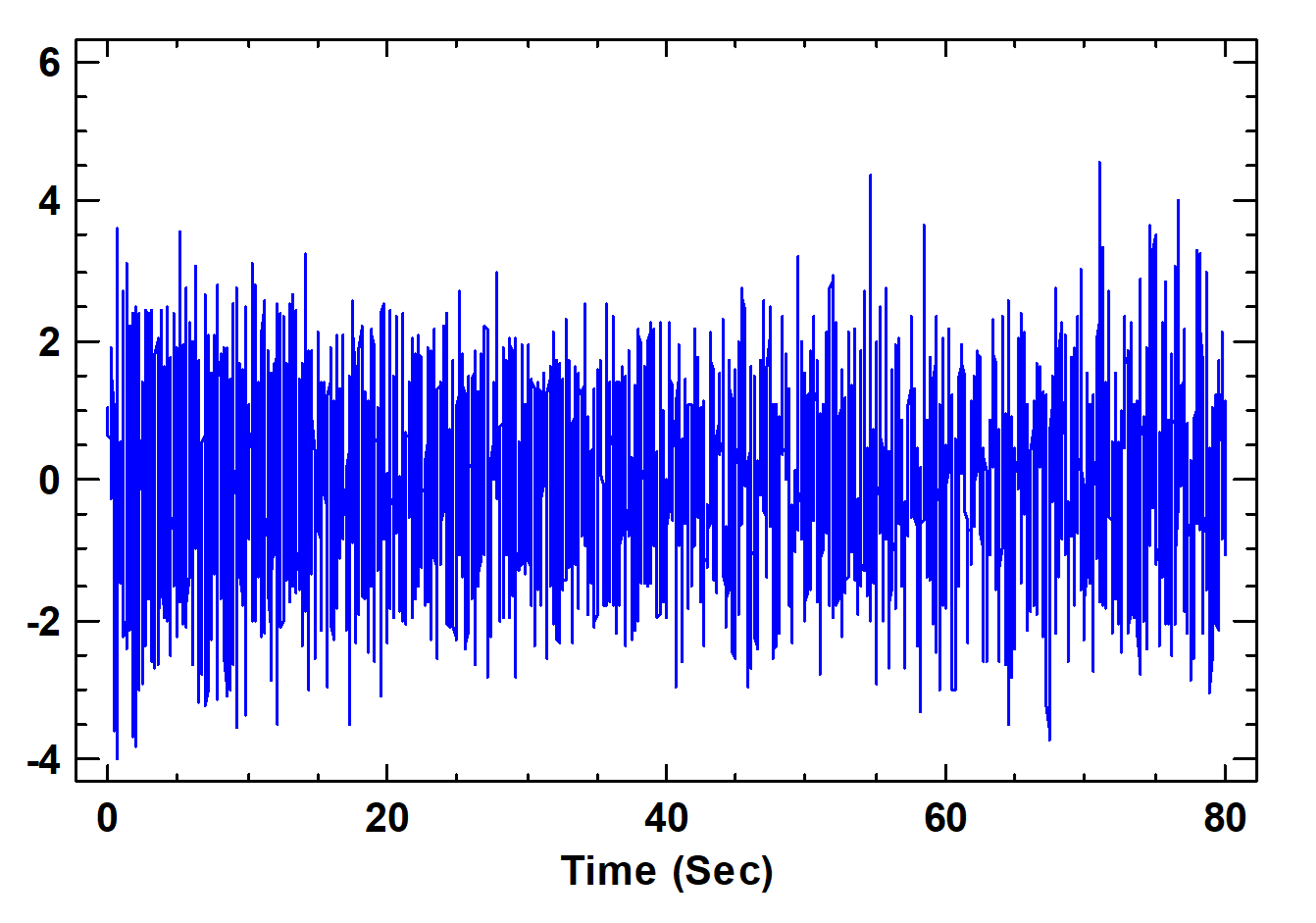
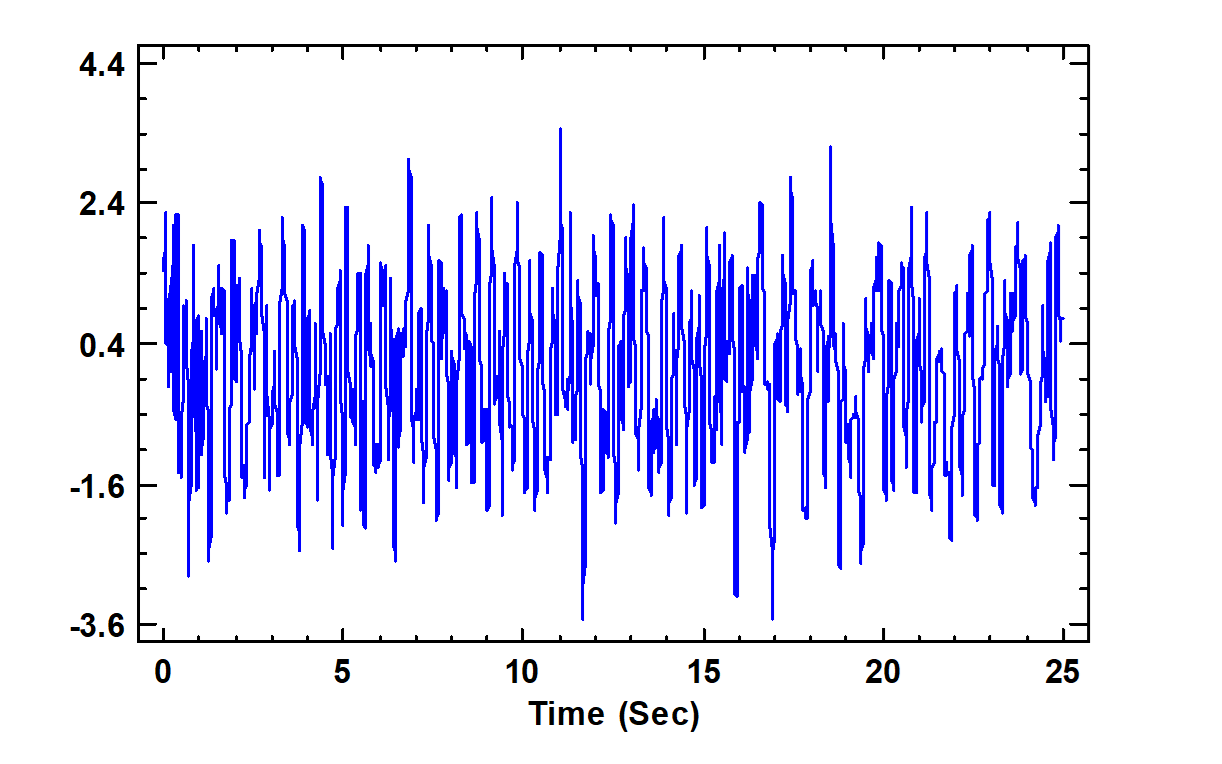
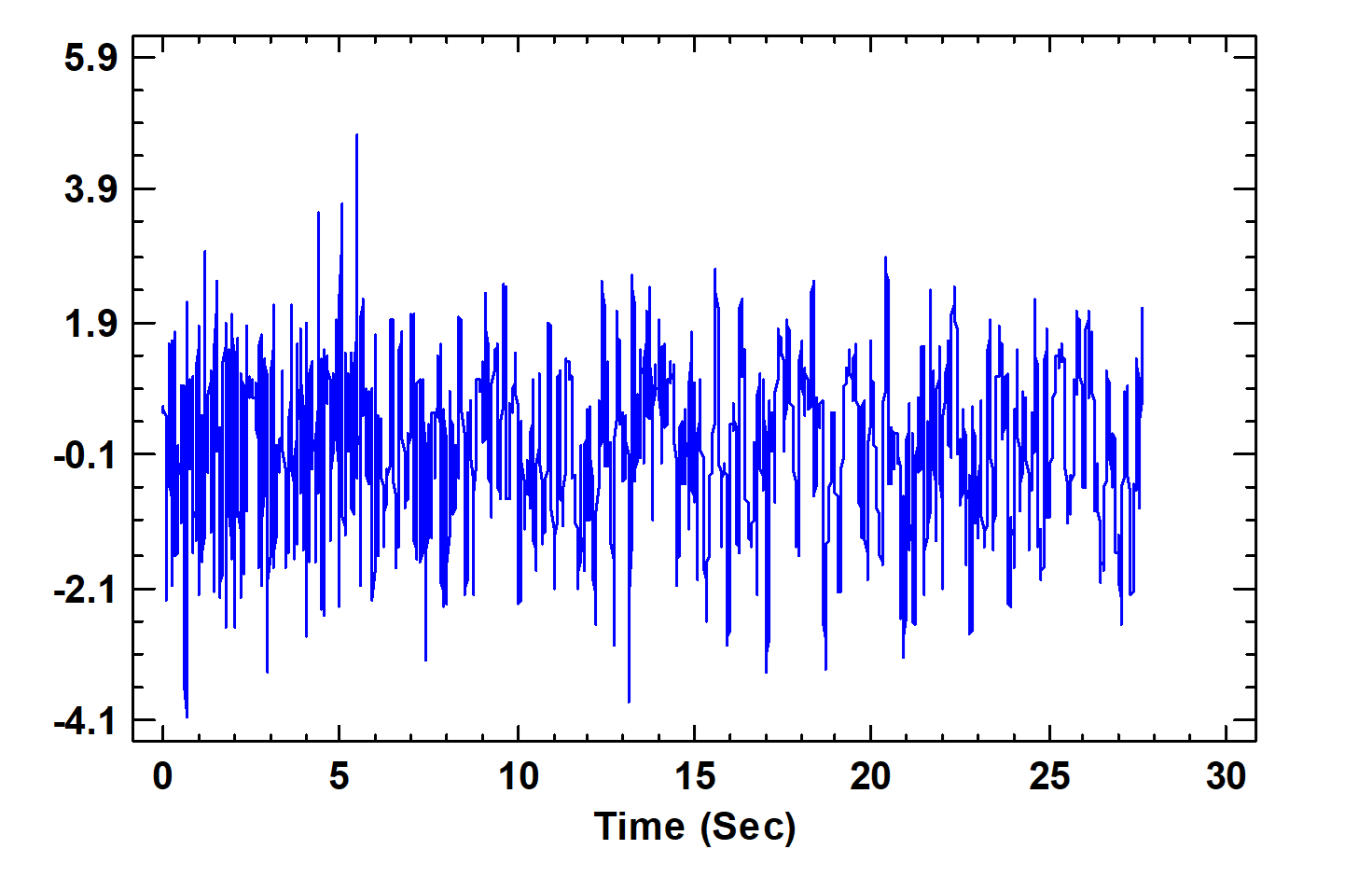
Results
The estimated autocorrelations for the three stabilized time series of the measured records were computed using Eq. 2.10. For illustration, the autocorrelation functions obtained are plotted in Figs. 3.10, 3.11, and 3.12. The tendency of the autocorrelation functions to die out rapidly indicates that none of the roots of the characteristic equations is close to the boundary of the unit circle. This ensures the time series stationarity obtained.
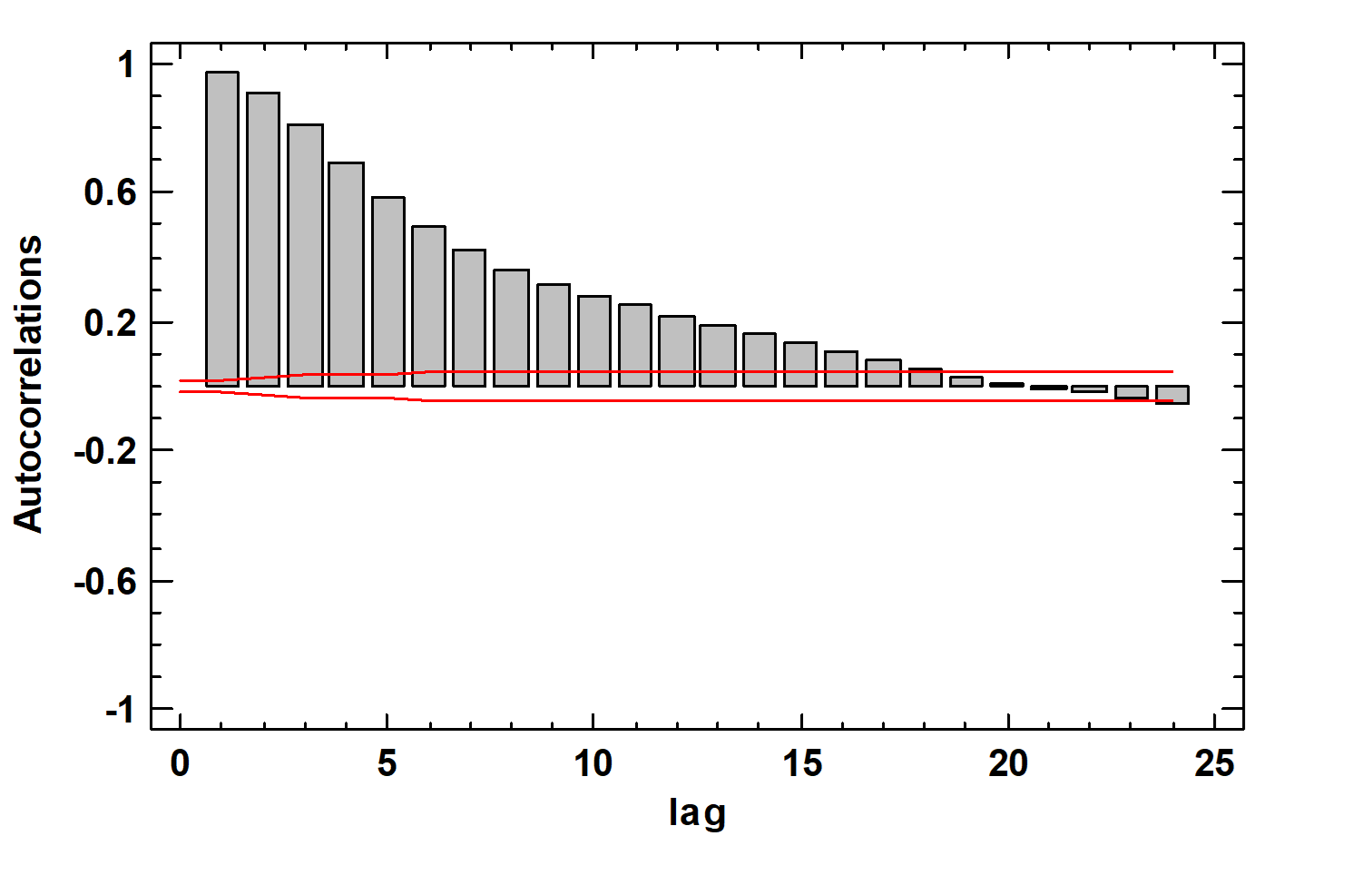
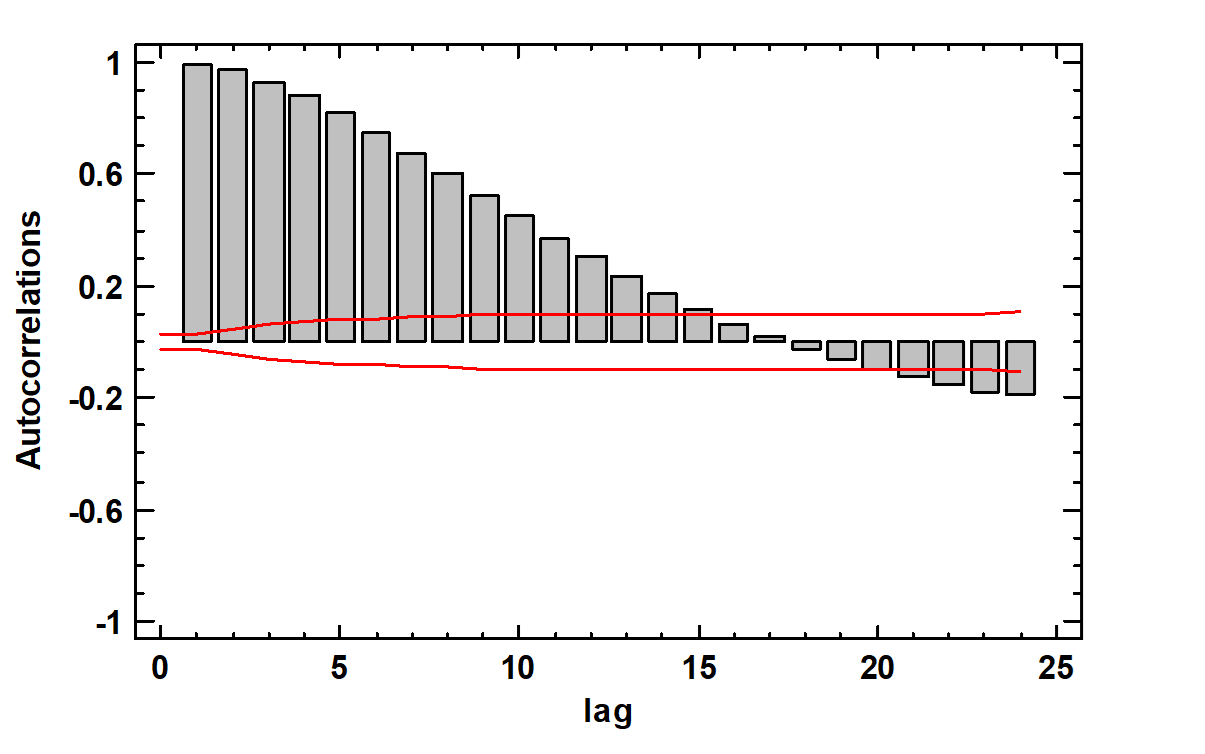
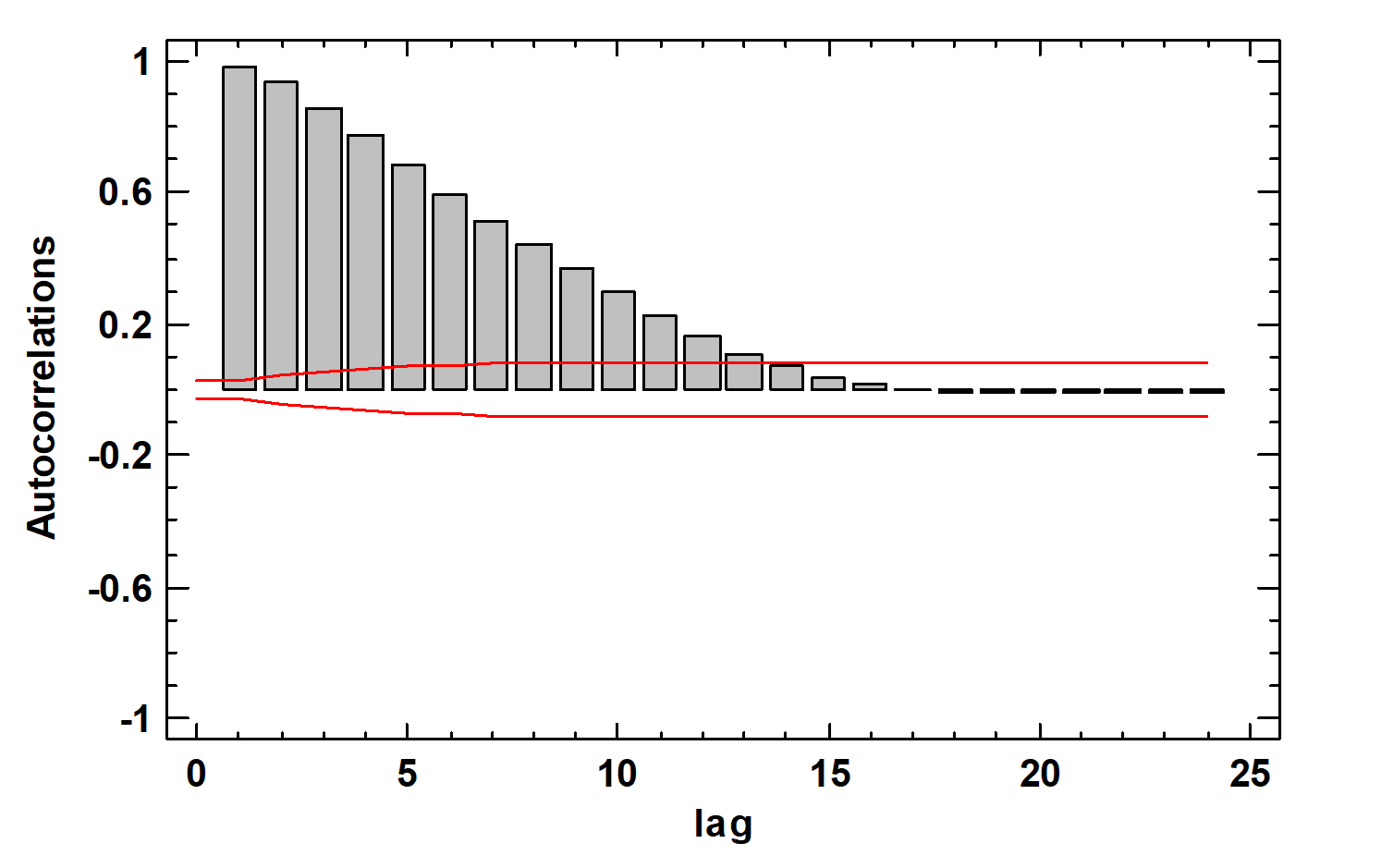
As explained previously, the partial autocorrelation functions for a record are estimated by fitting successive autoregressive processes of k orders using MATLAB. The results are plotted in Figs. 3.13, 3.14, and 3.15. From the partial autocorrelation function of Afroun, Ain Defla, and Dar El Beida time series, it is seen that after lag k = 2, or k = 3, the correlations decrease. This suggests the use of an ARMA model of order (p, q) such that p-q = 2 or 3. A model ARMA (p, q) of p-q around p-q=2 or 3 should be tried. The use of AE and partial AR functions suggested the process models which might be used. To obtain efficient estimates of the parameters, all the models suggested by the AR and partial AR functions were applied to the three events under investigation. A variety of ARMA models were fitted to the experimental records for the three acceleration time series using the maximum likelihood estimates, which could be approximated by the least-squares method. The comparison needed to select the order of the model could be done through the use of the AIC criteria. Subsequently, several ARMA simulations that had one or two MAs were found during the computation phase. In other words, the AIC (p, q) comparison clearly characterizes the simulation model with the minimum AIC value as the final one. Table 3.2 displays AIC values for ARMA (p, q) models for each event. The results indicate that Afroun, Ain Defla, and Dar El Beida acceleration time series are best characterized by ARMA (2, 2) processes. Shown in Table 3.1 are the autoregressive parameters the moving average
and the envelope function parameters α,β,γ, corresponding to maximum likelihood estimates for each event.
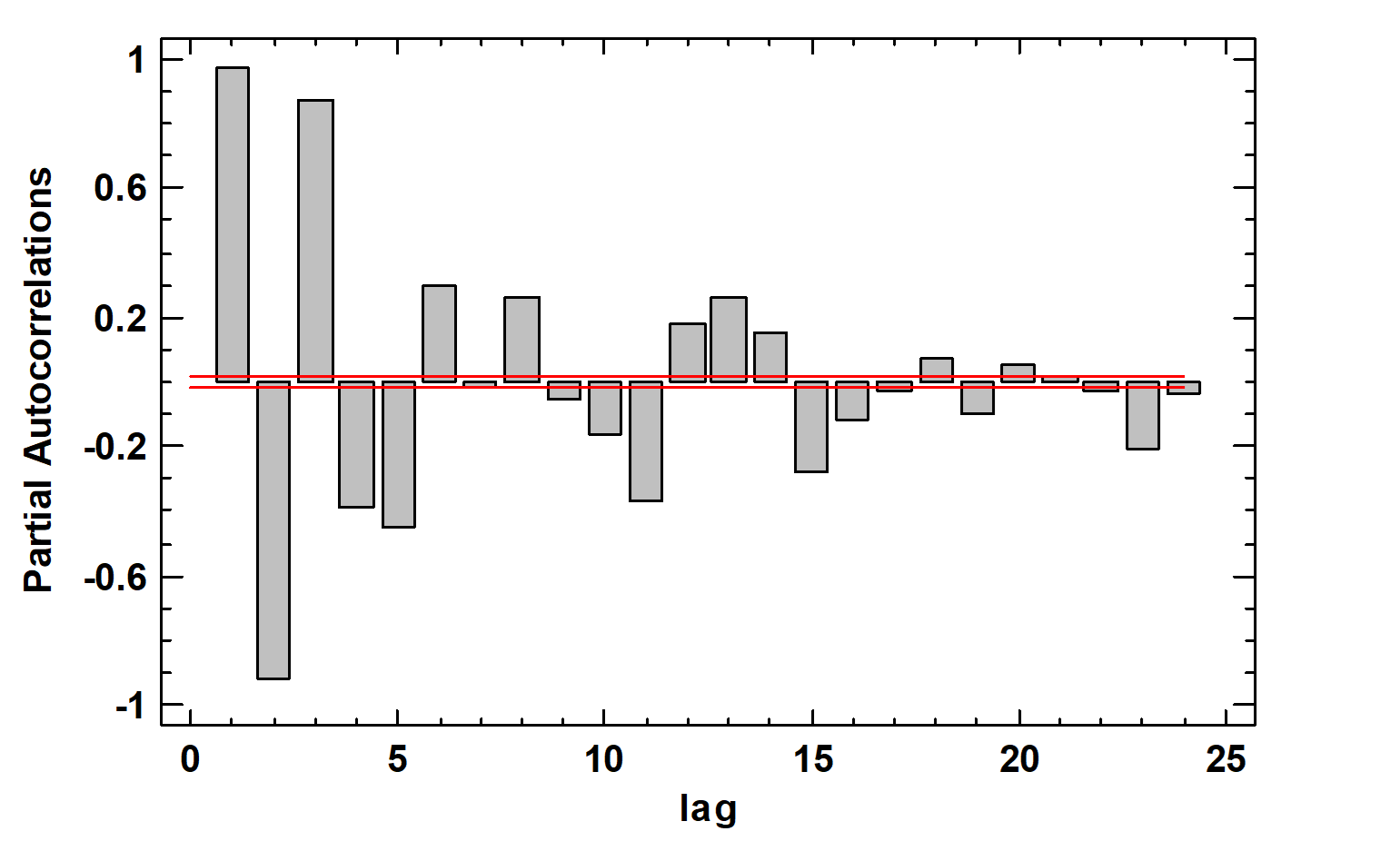
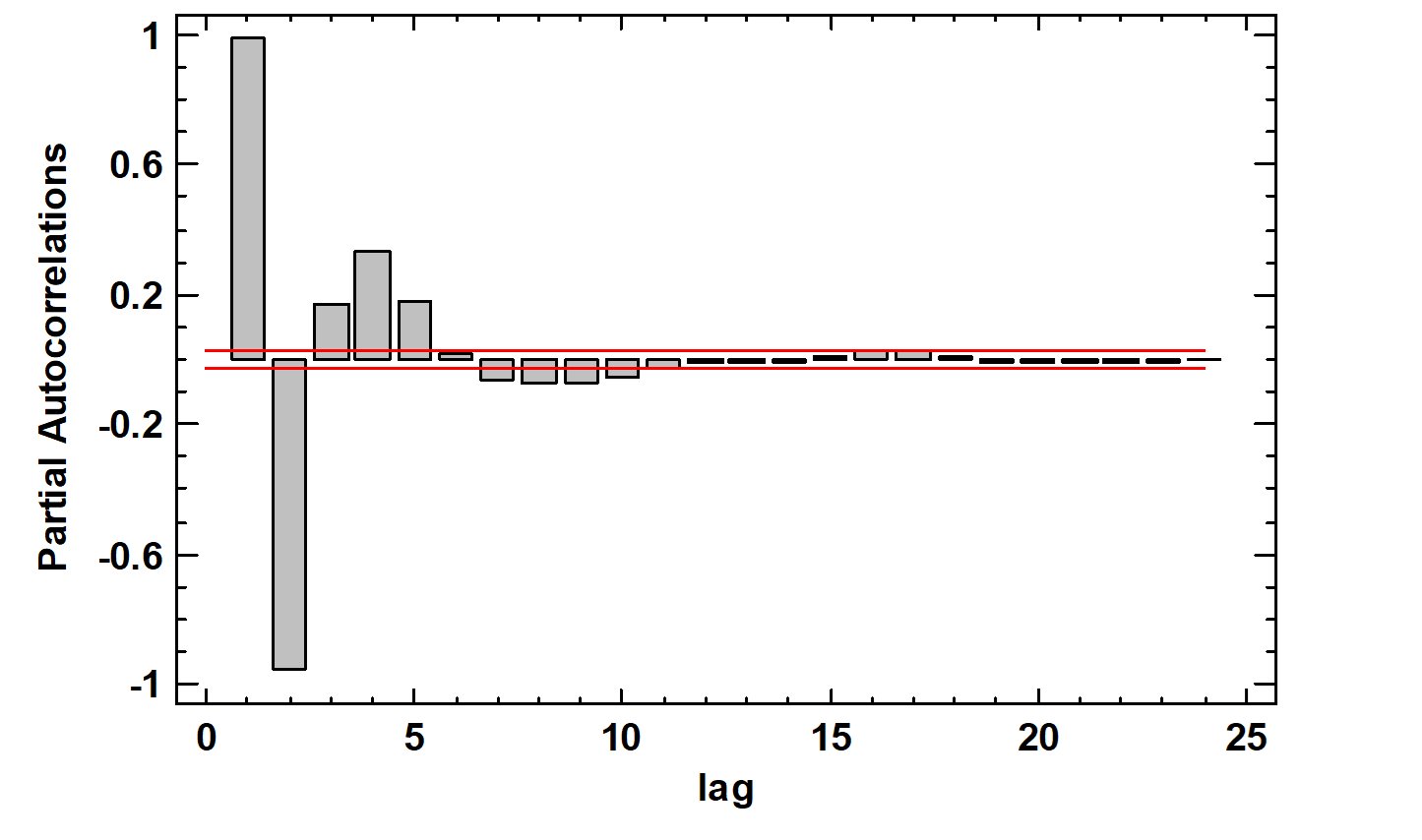
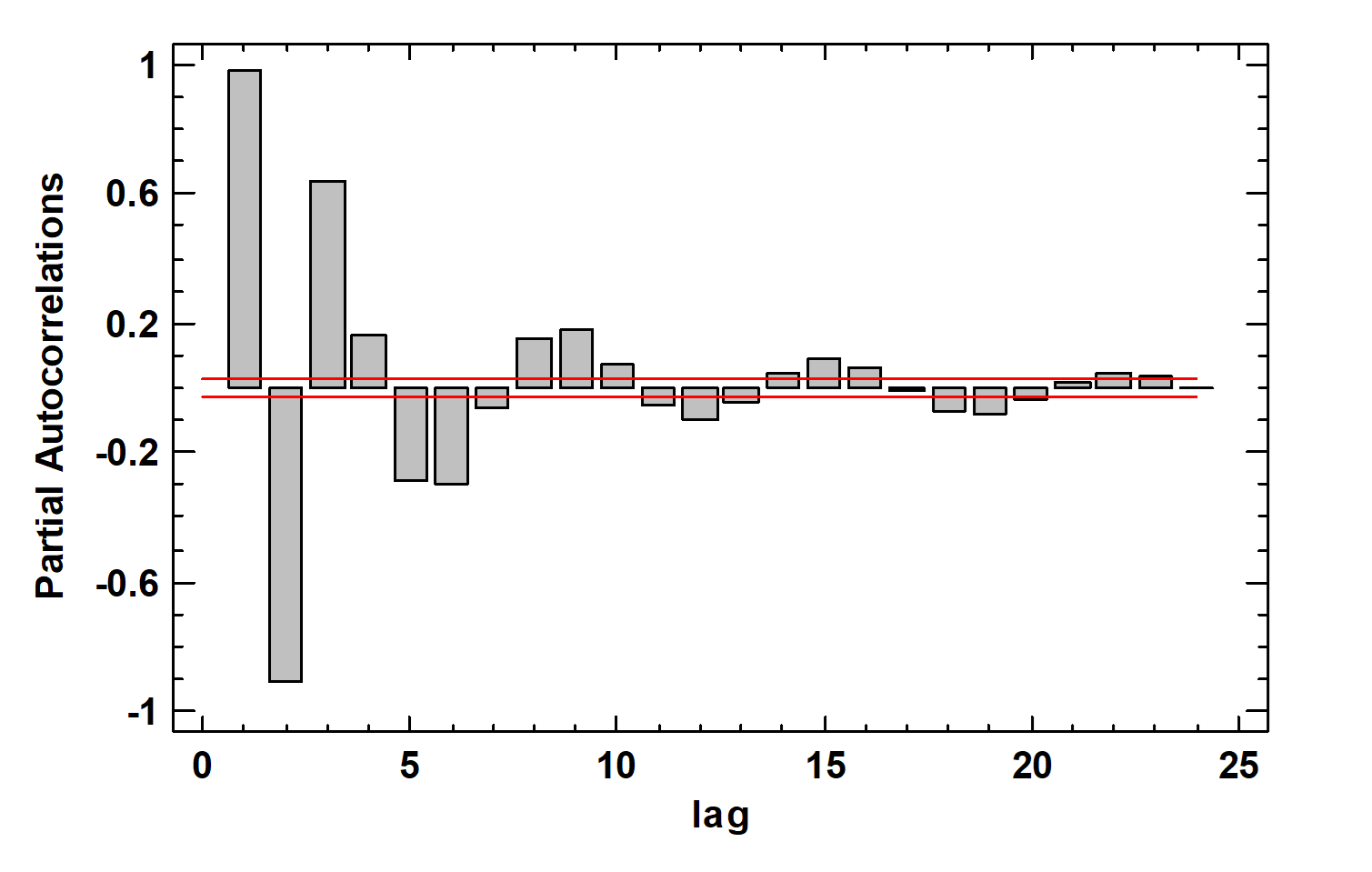
Acceleration Time Series Simulation
As previously stated, through the use of simulated ARMA process systems in a time-based approach, good results can be achieved with a limited number of parameters. To produce the population that describes the observed acceleration and be used for response spectra and damage, acceleration time series simulation is needed. The experimental procedure used was as follows: first of all, the station time series were generated using the ARMA model. Once this was done, the stationary series was multiplied by the function s(t), an envelope form. In addition, the assumption that ARMA is treated as a linear combination of Gaussian random variables () and already existing values (
) was used: this means that the generated time series can be simulated recursively. Hence, shown in Figs. 3.16, 3.17, and 3.18 are three simulations of the acceleration time series.
Table 3.1: ARMA and Envelope Function Parameters
Table 3.2: AIC Values for ARMA (p, q) Models
*Optimal Set by AIC Criterion
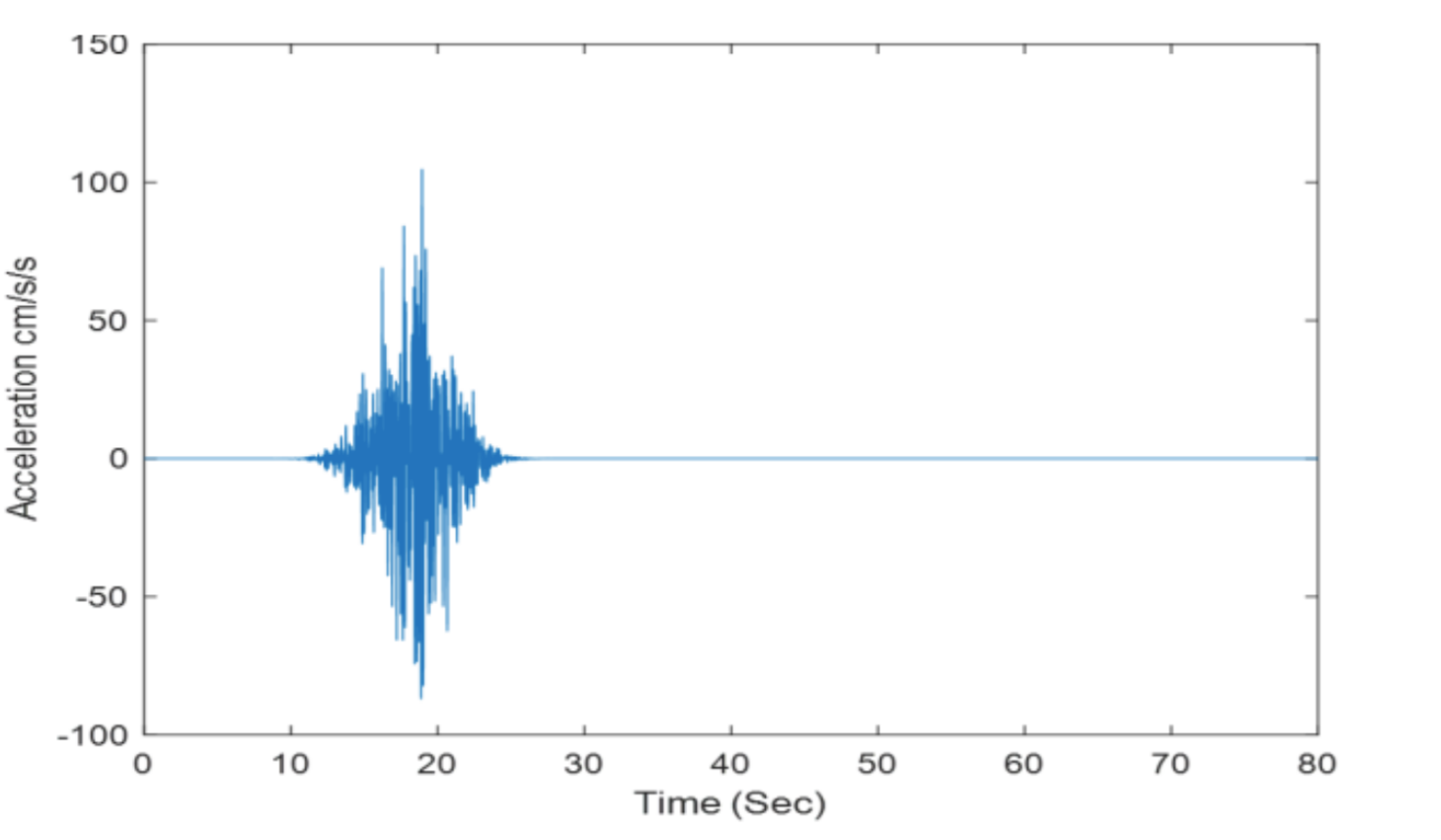
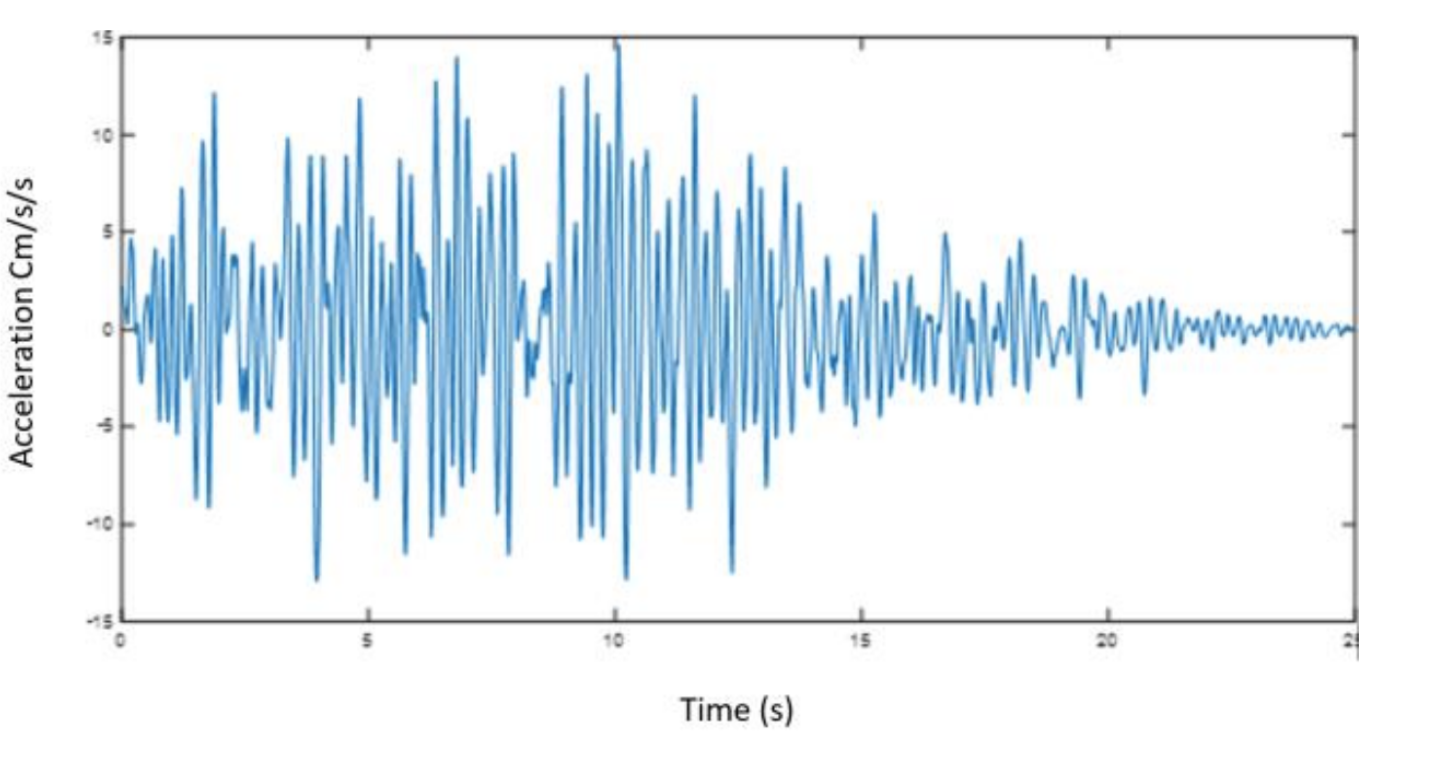
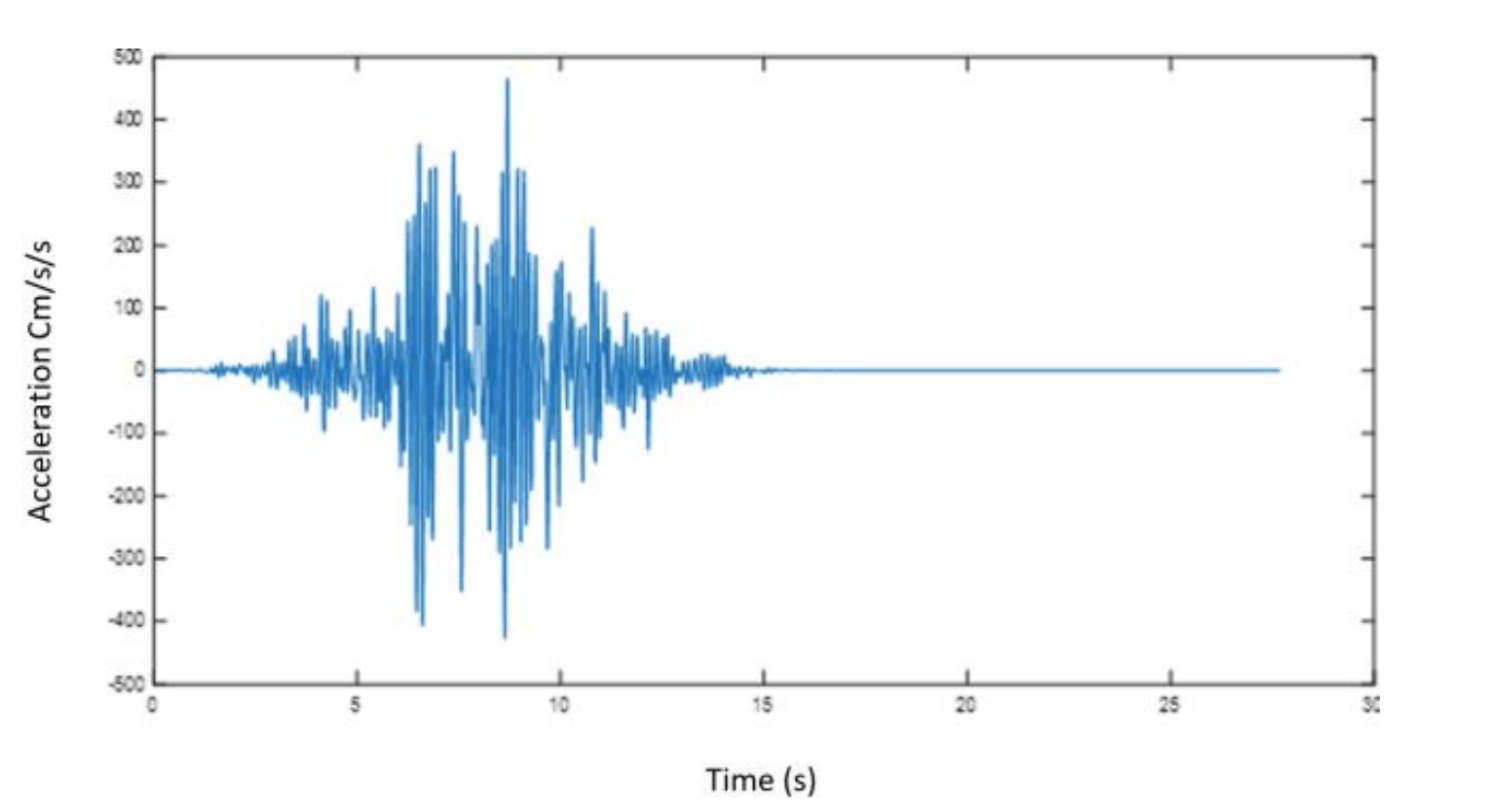
Response Spectra
The need for high accuracy characterizes structural analysis, so sensitive predictive models find great importance in this issue. More specifically, ARMA models are characterized as an effective analytical tool to describe qualitatively and quantitatively the non-stationarity of the input data for analysis. Thus, each acceleration time series used is treated as an output event of a series of multiple stochastic processes. At the same time, the description of the ground acceleration time series can be accomplished using structural response spectra. In turn, to obtain them, systems with one degree of freedom and viscous damping were used. It should be taken into account that the system can have either bilinear or degenerate stiffness. This means that for successful modeling, it is necessary to use an integration method in which linear acceleration is taken into account at each step. [18]. Thus it becomes possible to obtain the spectral response for the series. The equation is:
where is the mass,
is the relative mass displacement, c is the damping coefficient and
is the acceleration of M, and
is the restoring force.
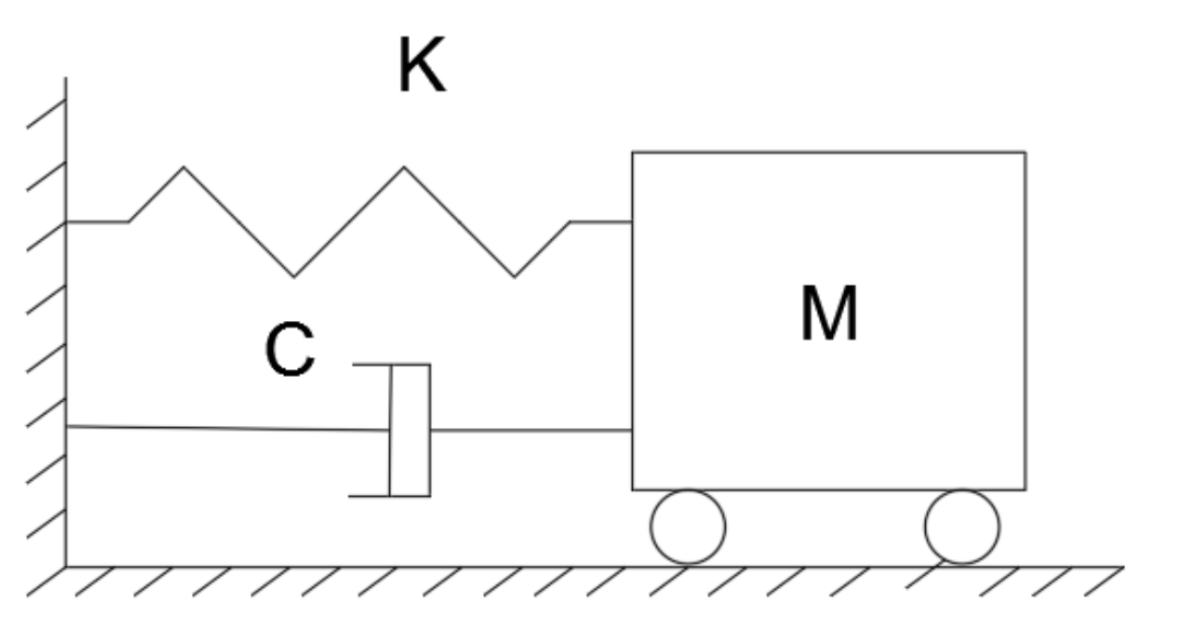
Maximum Displacement Ductility
It is usually sufficient to have the maximum response value in mind for system design purposes:
Thus, this function allows us to relate the maximum value to the period of natural oscillations of the system: this makes it possible to create a spectrum for systems in which the damping values and the periodic range are the same.
Normalized Hysteretic Energy
Thus, hysteretic energy can be obtained by dividing the dissipation energy by twice the absorbed energy. In such a case, the energy dissipated in a structure with a hysteretic load-strain relation is given as follows:
where is the elastic strain energy:
is the restoring force
Then, the normalized hysteretic energy:
Where is the yield force.
Application and Results
In the course of this work, several of the proposed ARMA models showed excellent ability to be used for structural analysis of three-time series that differ in the number of data points. The results of the predicted ARMA simulation characteristics, as well as information about the properties of their envelope functions, are available in Table 3.1. The actual simulation procedure was performed through a series of sequential steps. First, three real-time series were used to generate a sample of their ten simulation series. The second step was the response analysis, for which damping coefficients ε = 0.05 were used with yield coefficients of 0.05, 0.1, 0.15, 0.2, 0.3. It is fair to note that the calculation of hysteretic energy was done through alternative output coefficients. Third, the mean displacement ductility was utilized to determine the hysteretic energy demand spectra. Fourth, standard deviations were used to estimate the confidence interval: the results are shown in Figures 4.2-4.13. It was observed that the ordinates of the spectra decreased comparably with the increasing period, whereas the mean response spectra described a smooth curve with changing frequency. Finally, it was found that the spectral ordinates were broadly similar for reduced stiffness and bilinear stiffness systems.
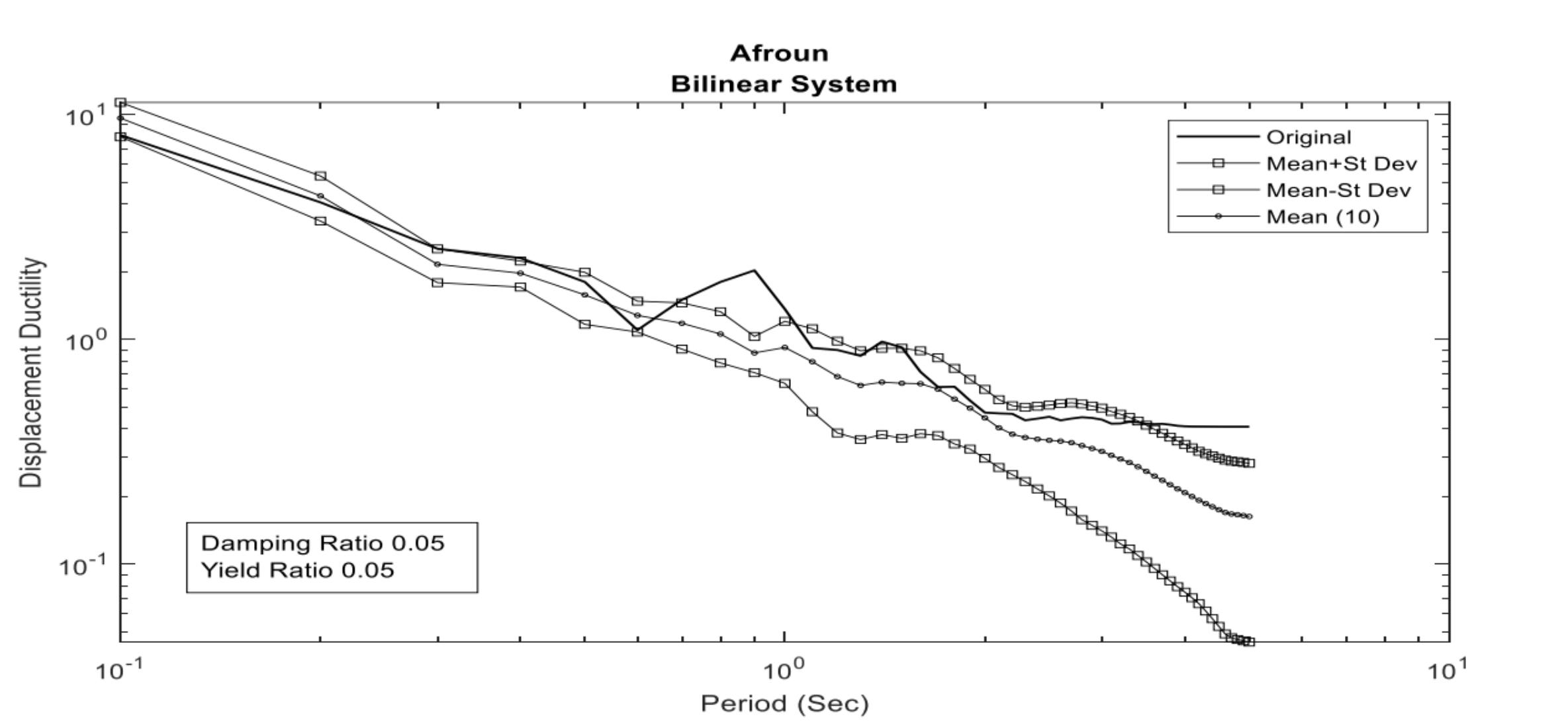
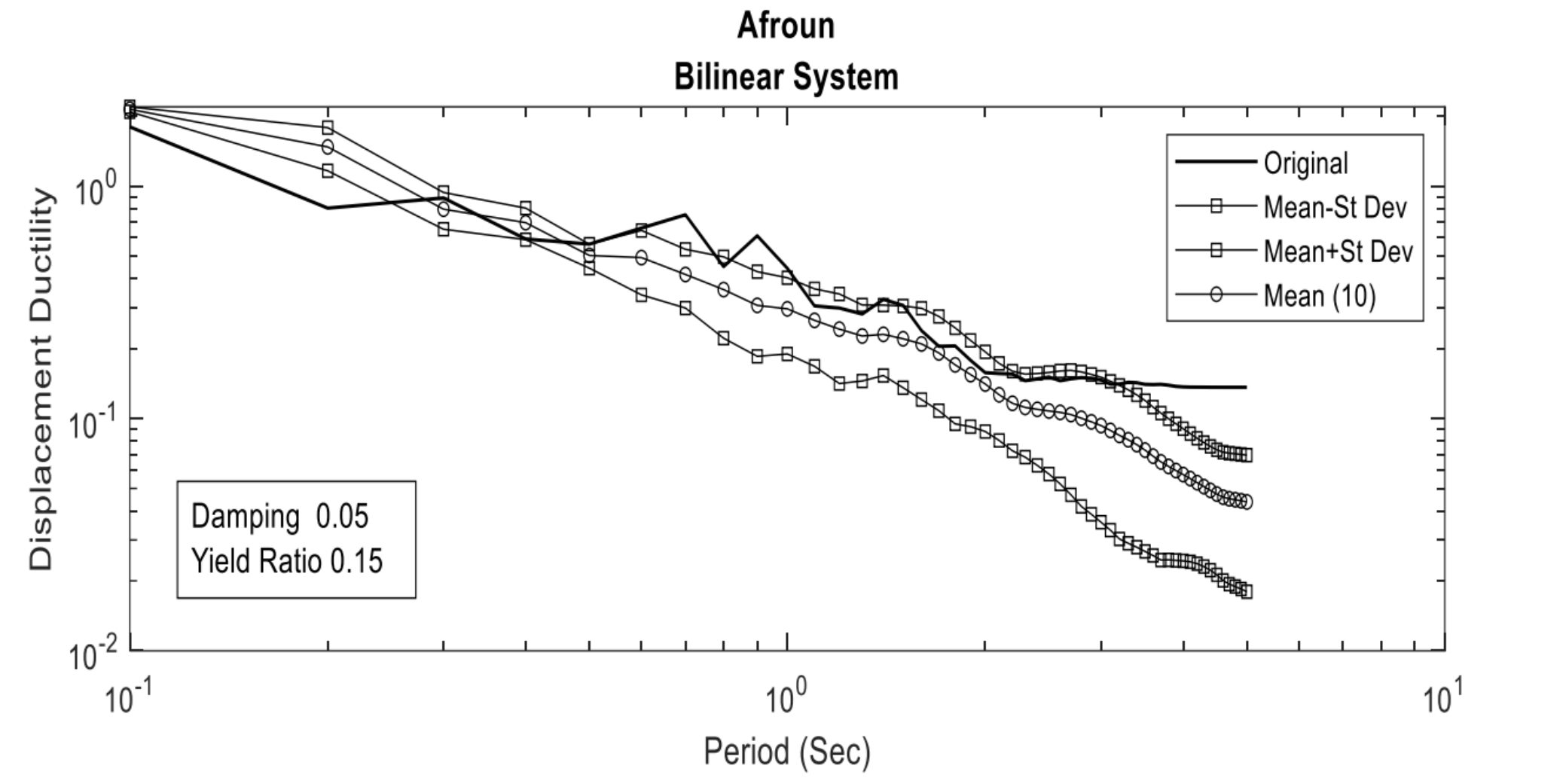
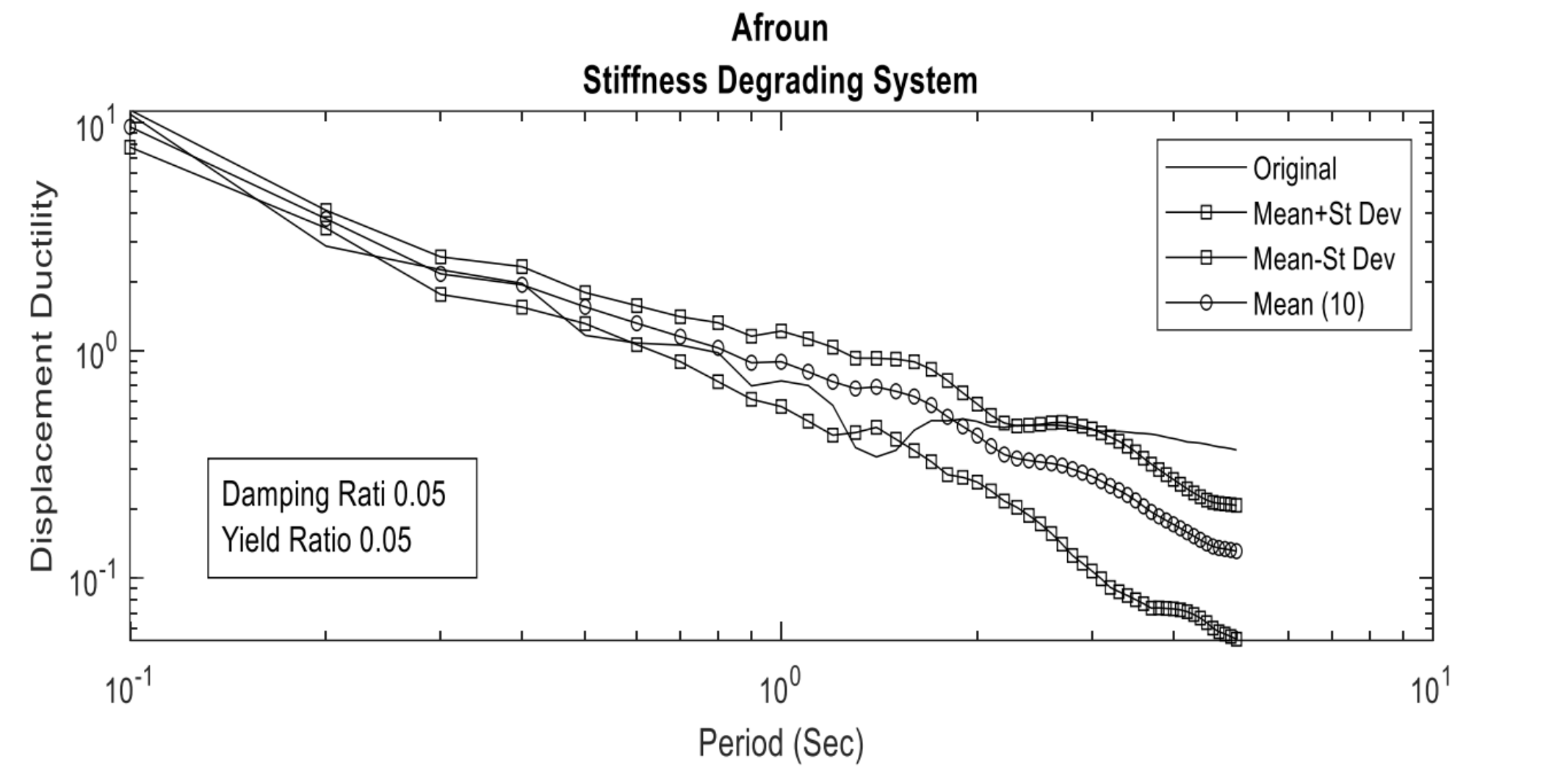
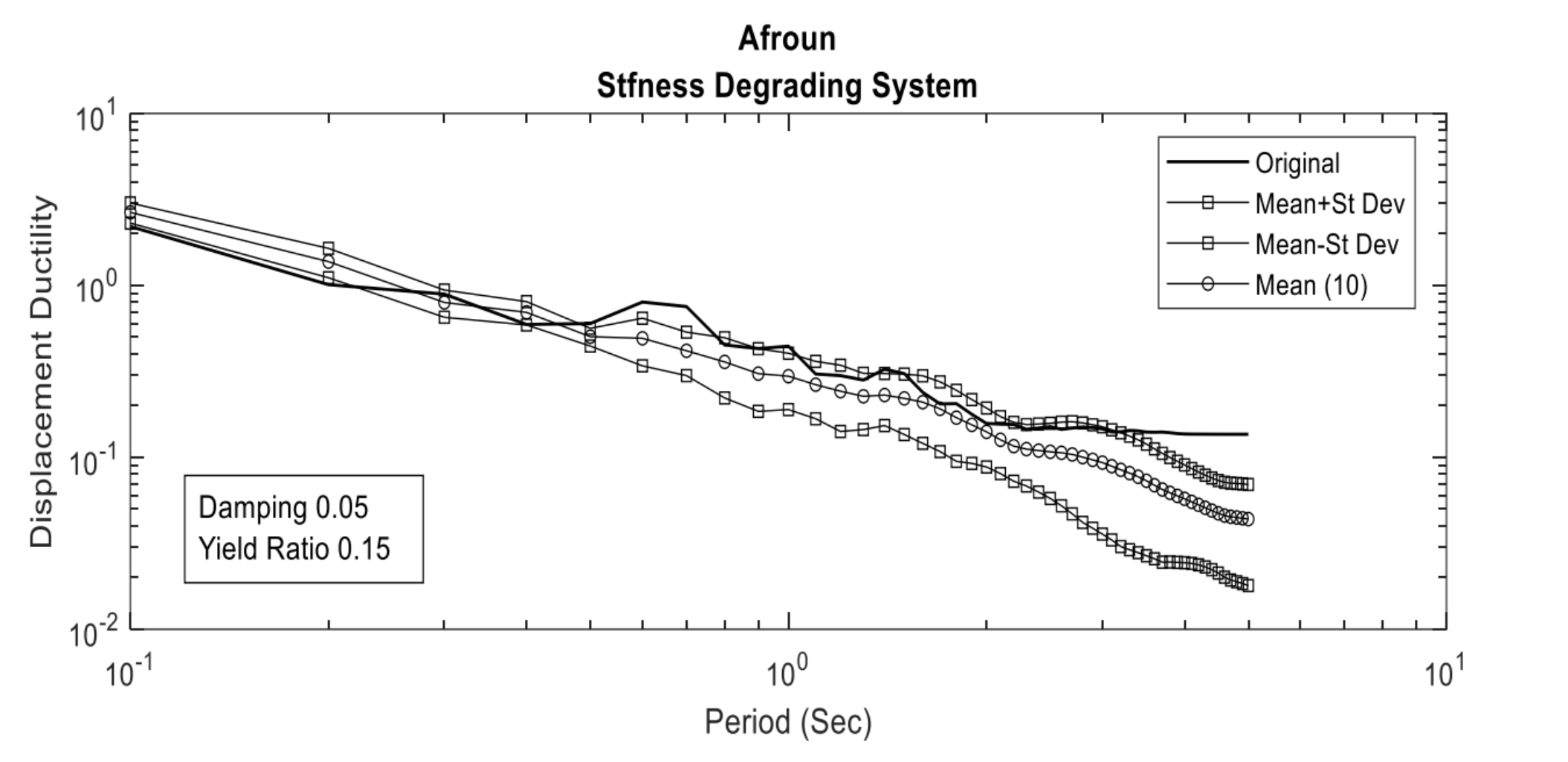
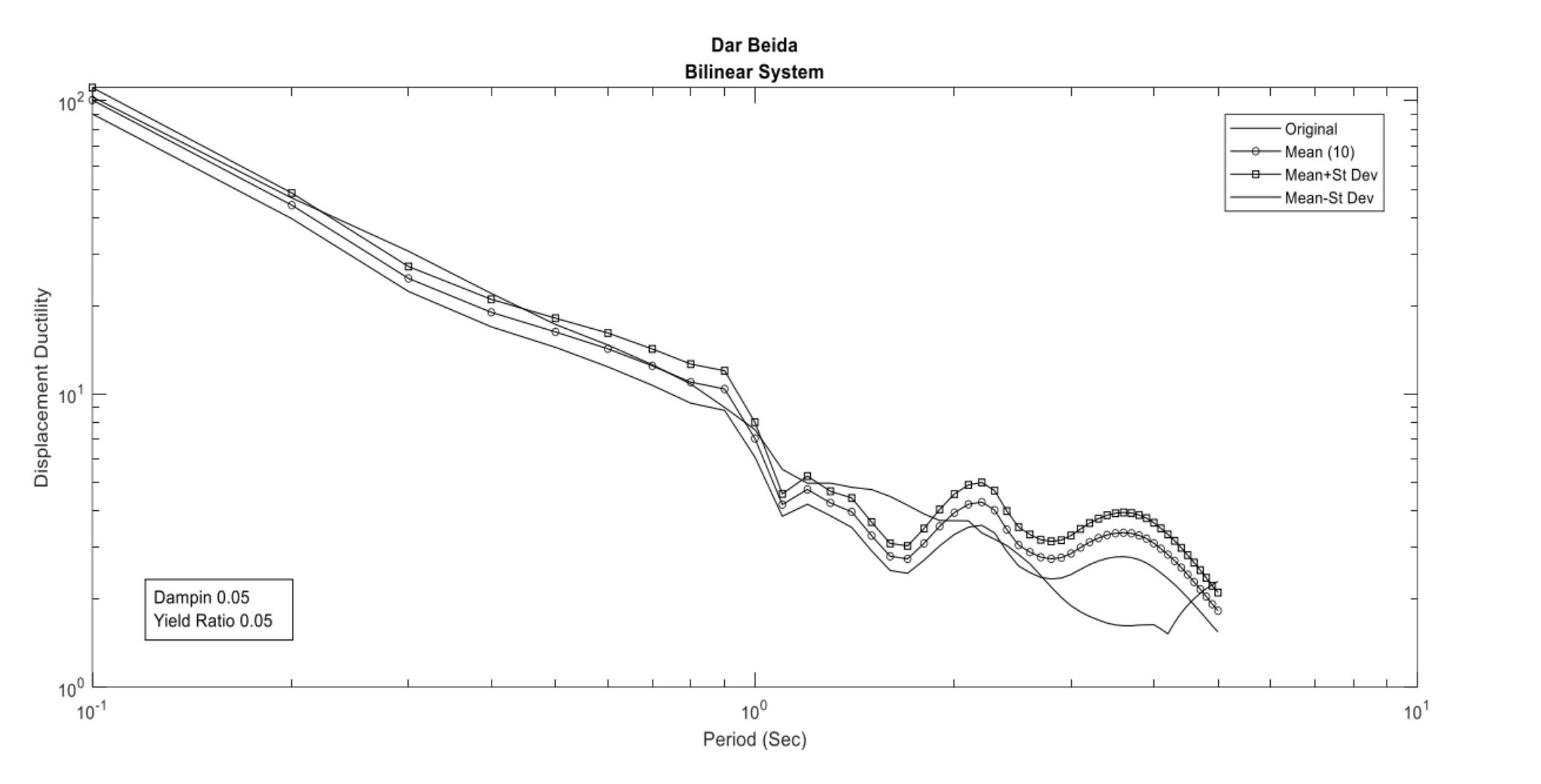
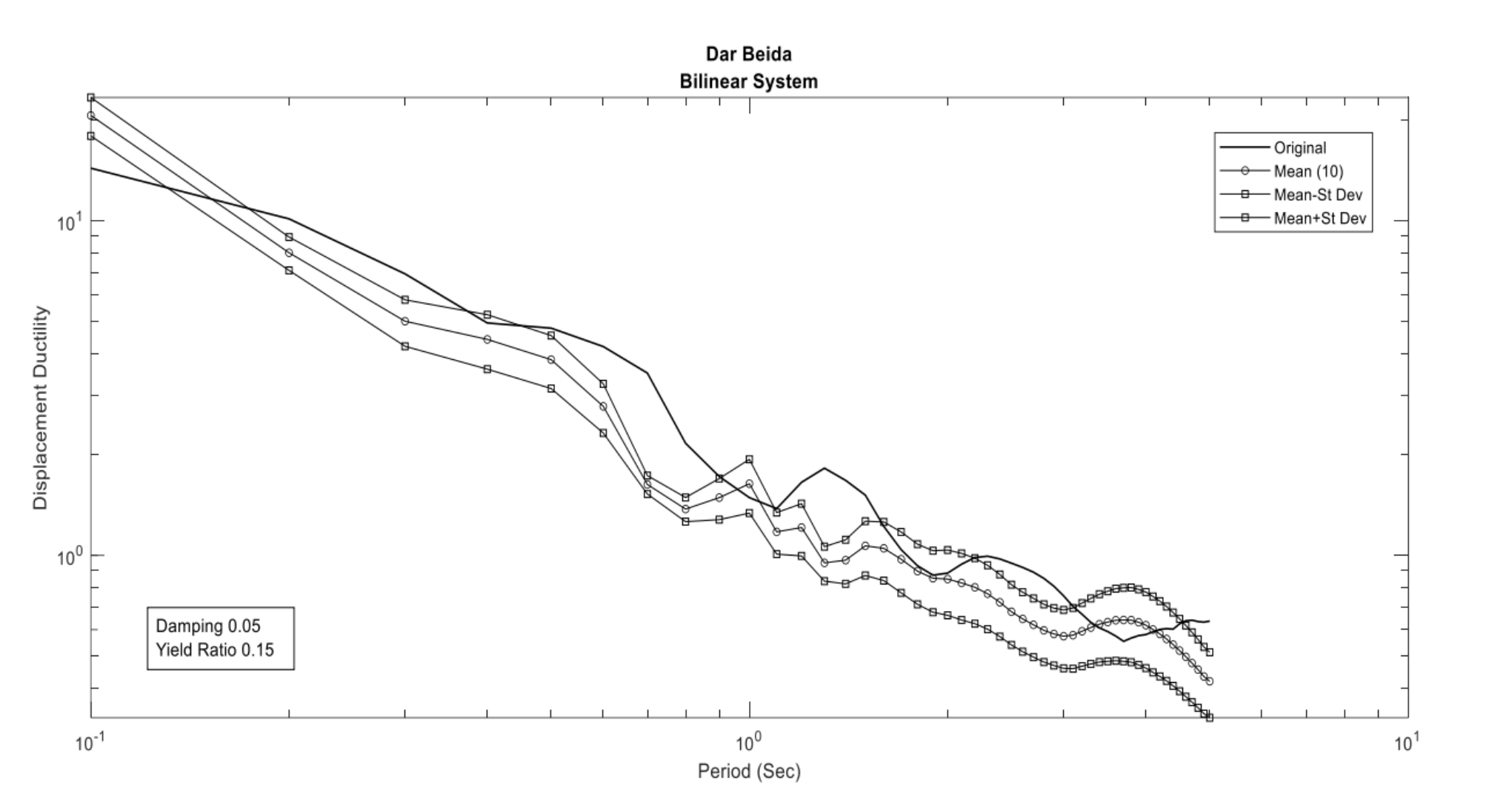
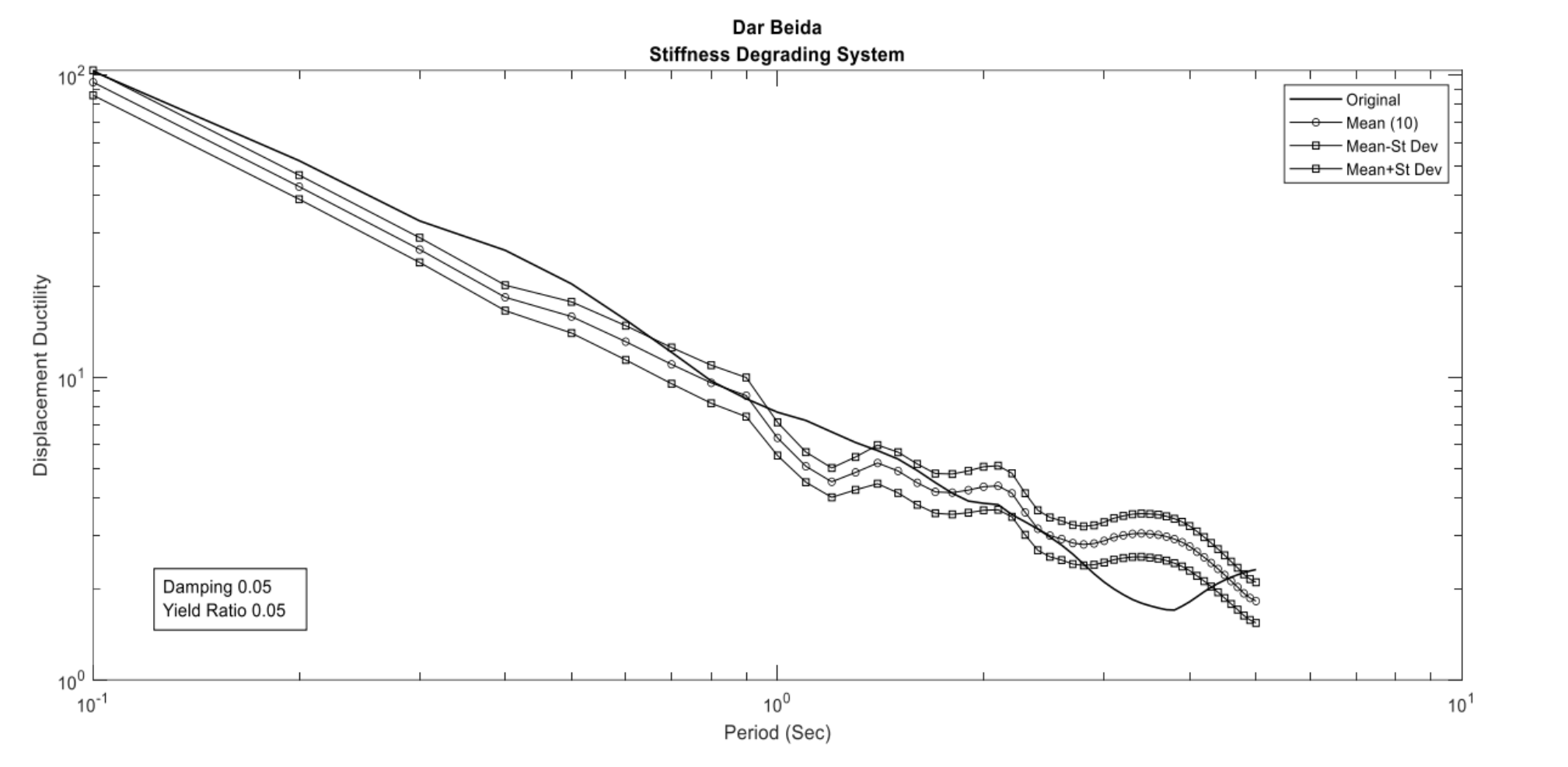
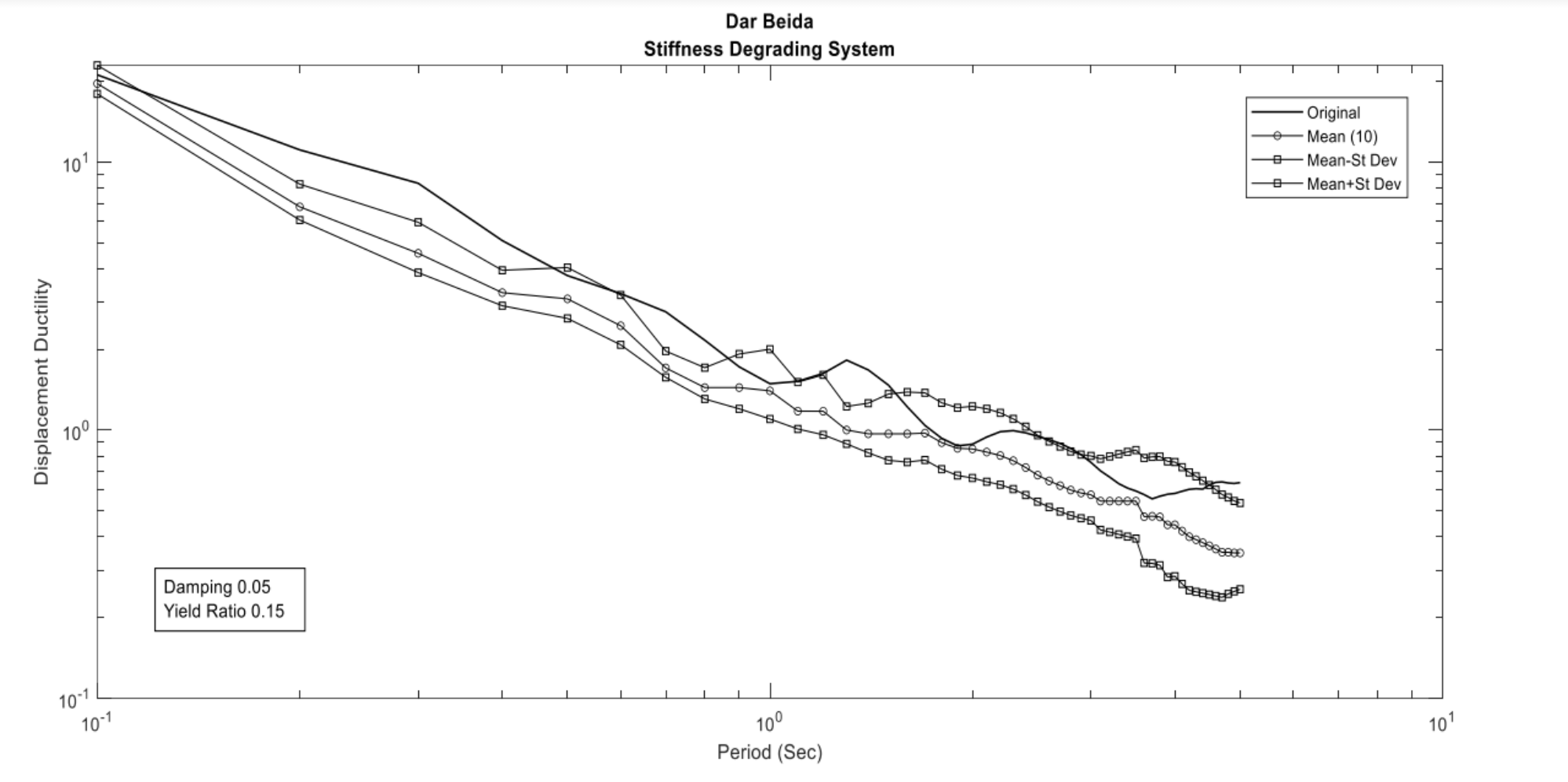
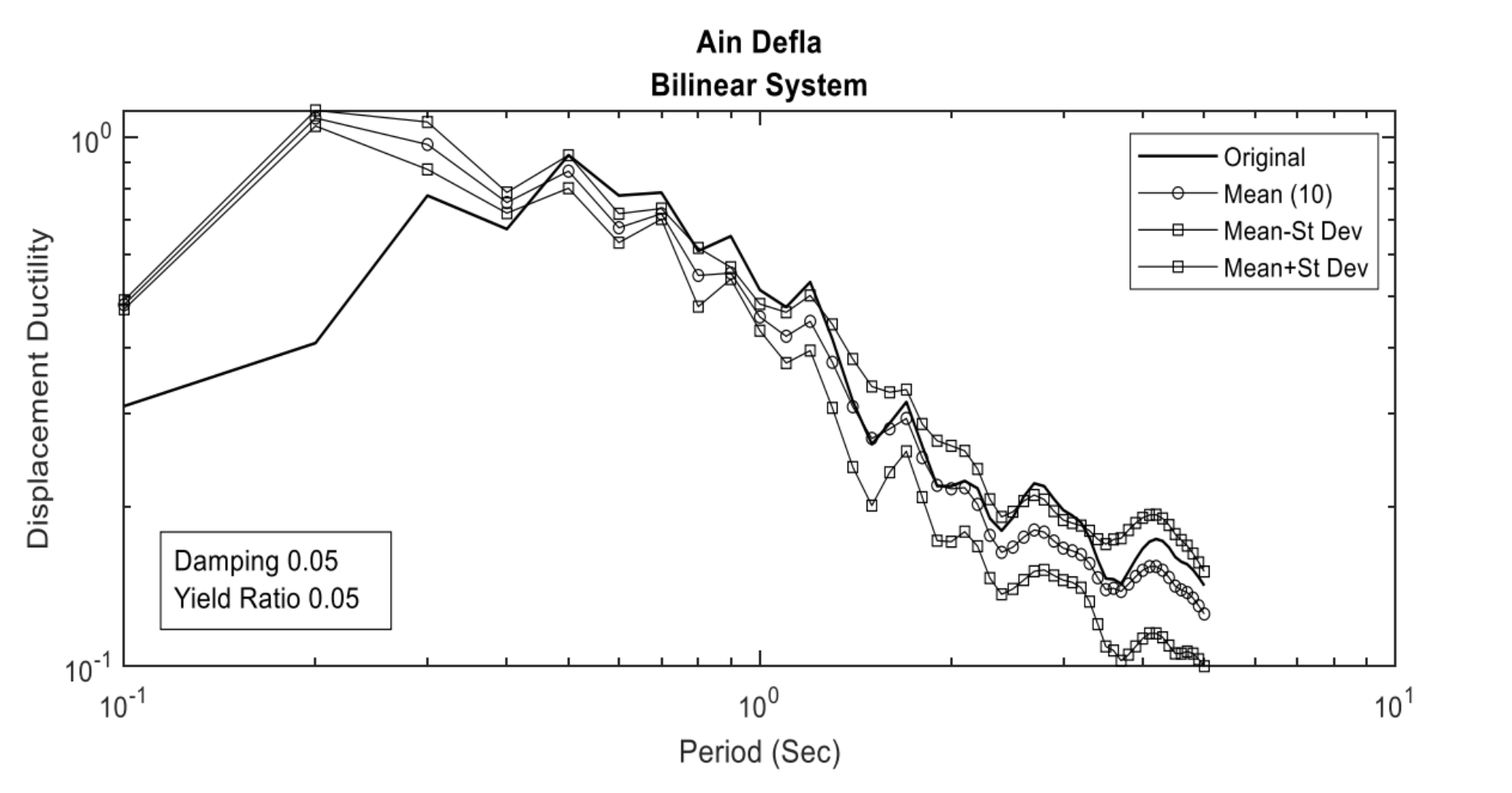
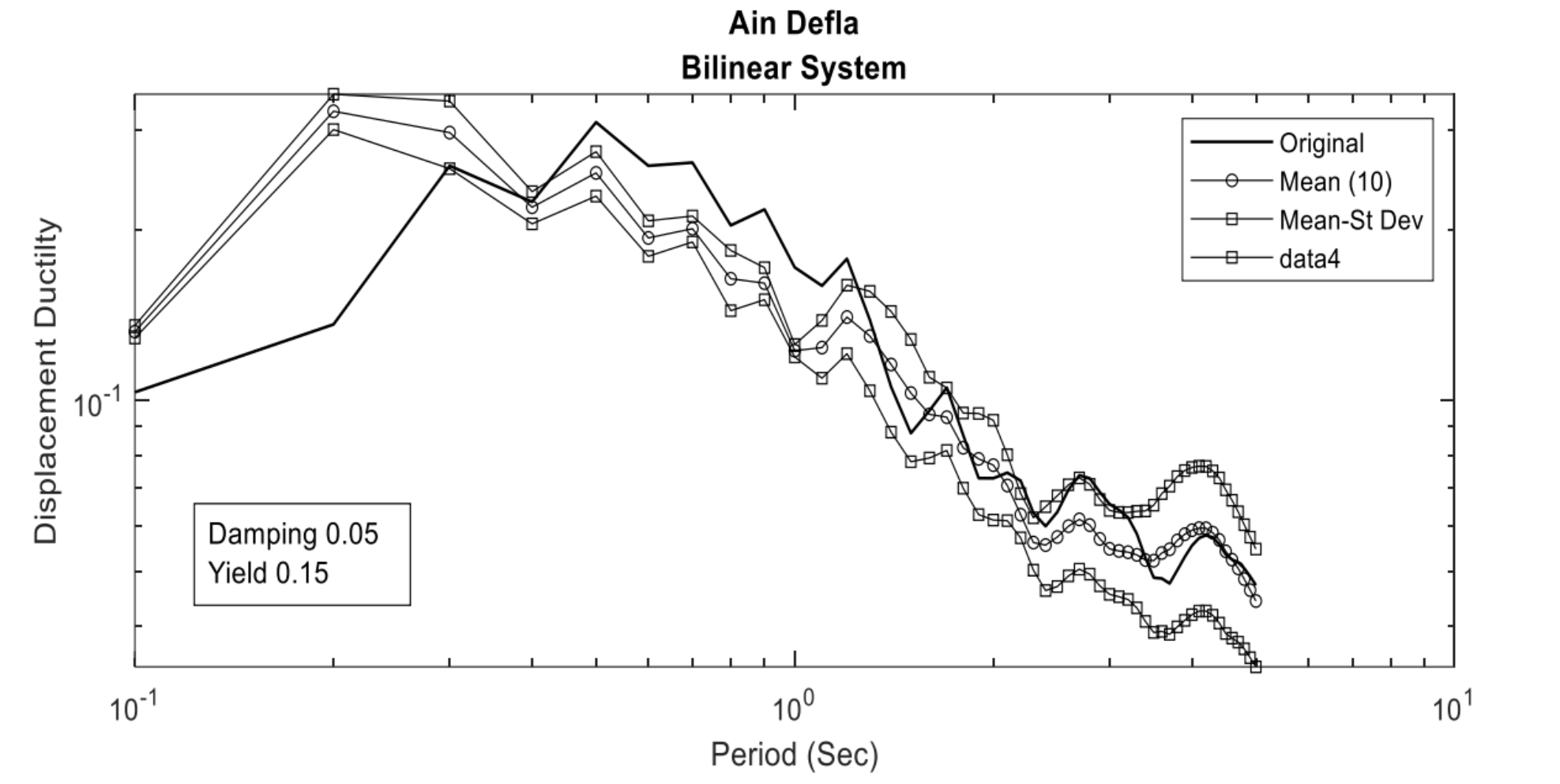
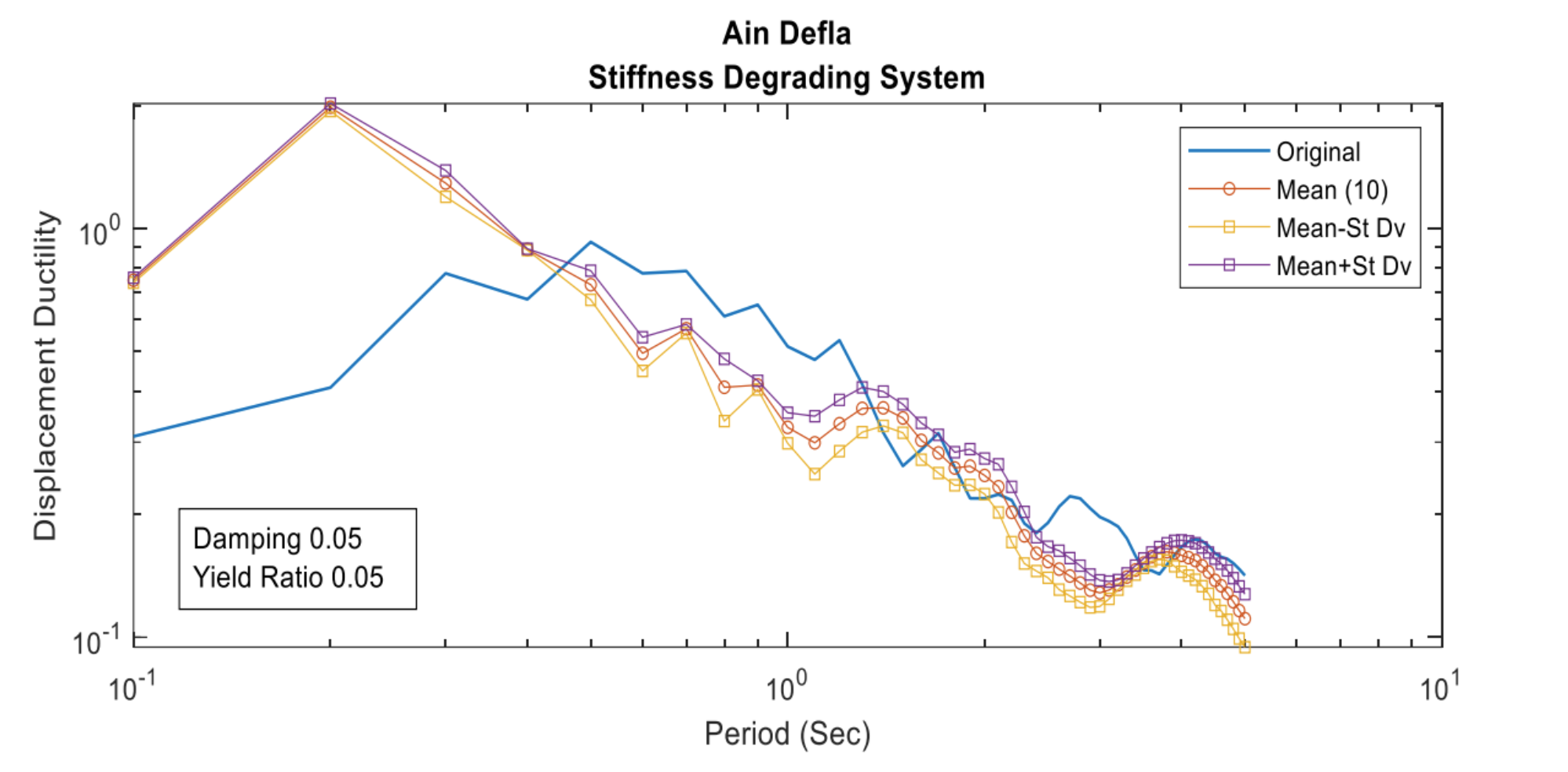
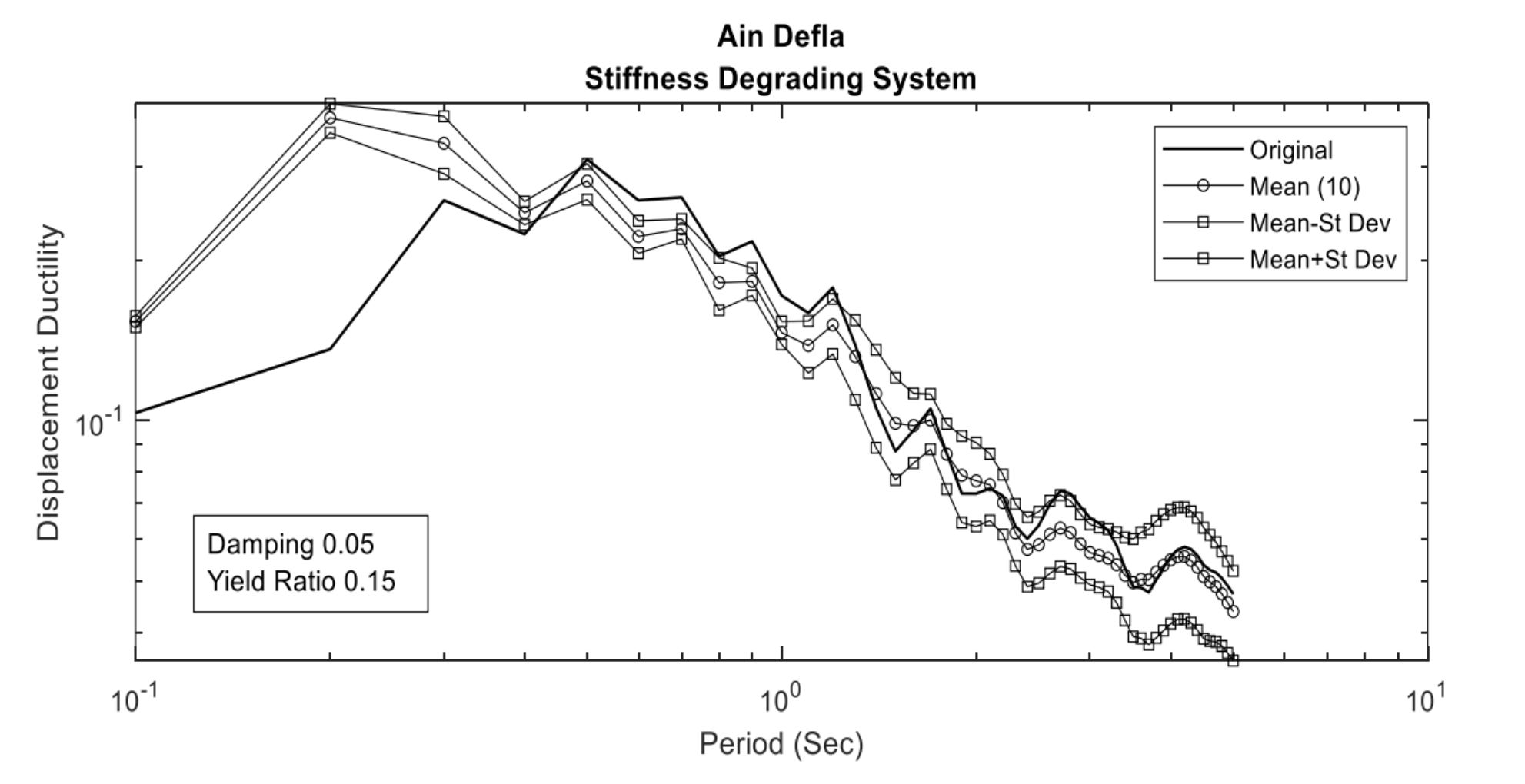
One of the most critical factors in the structural analysis of nonlinear systems has been identified as the yield coefficient since the inelastic response depends on the initial yield displacement. Thus, Figures 4.14, 4.15, and 4.16 illustrate classical descriptions of mean displacement ductility at increasing yield coefficients, provided that 5% damping is performed for a system with bilinear stiffness.
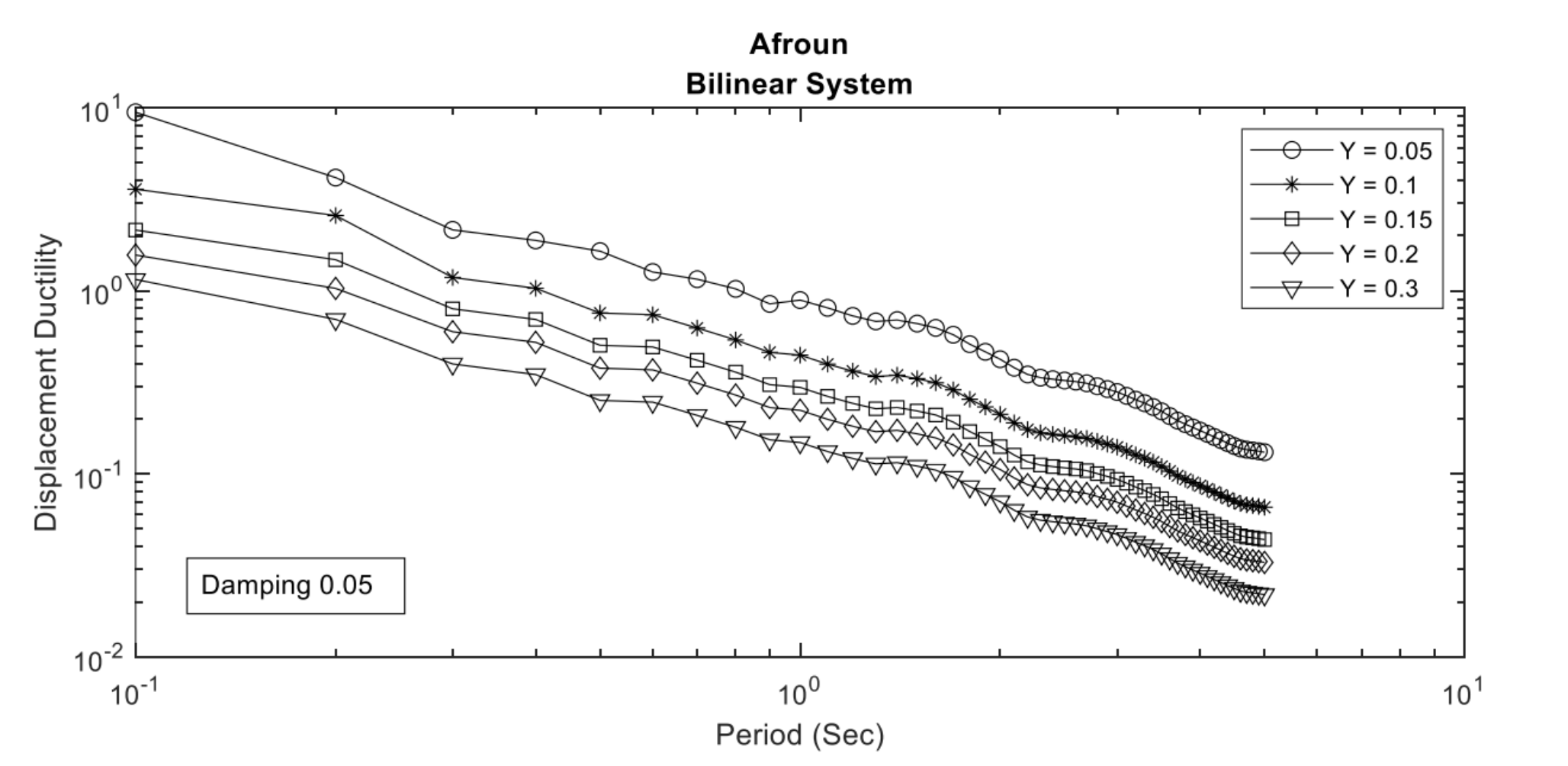
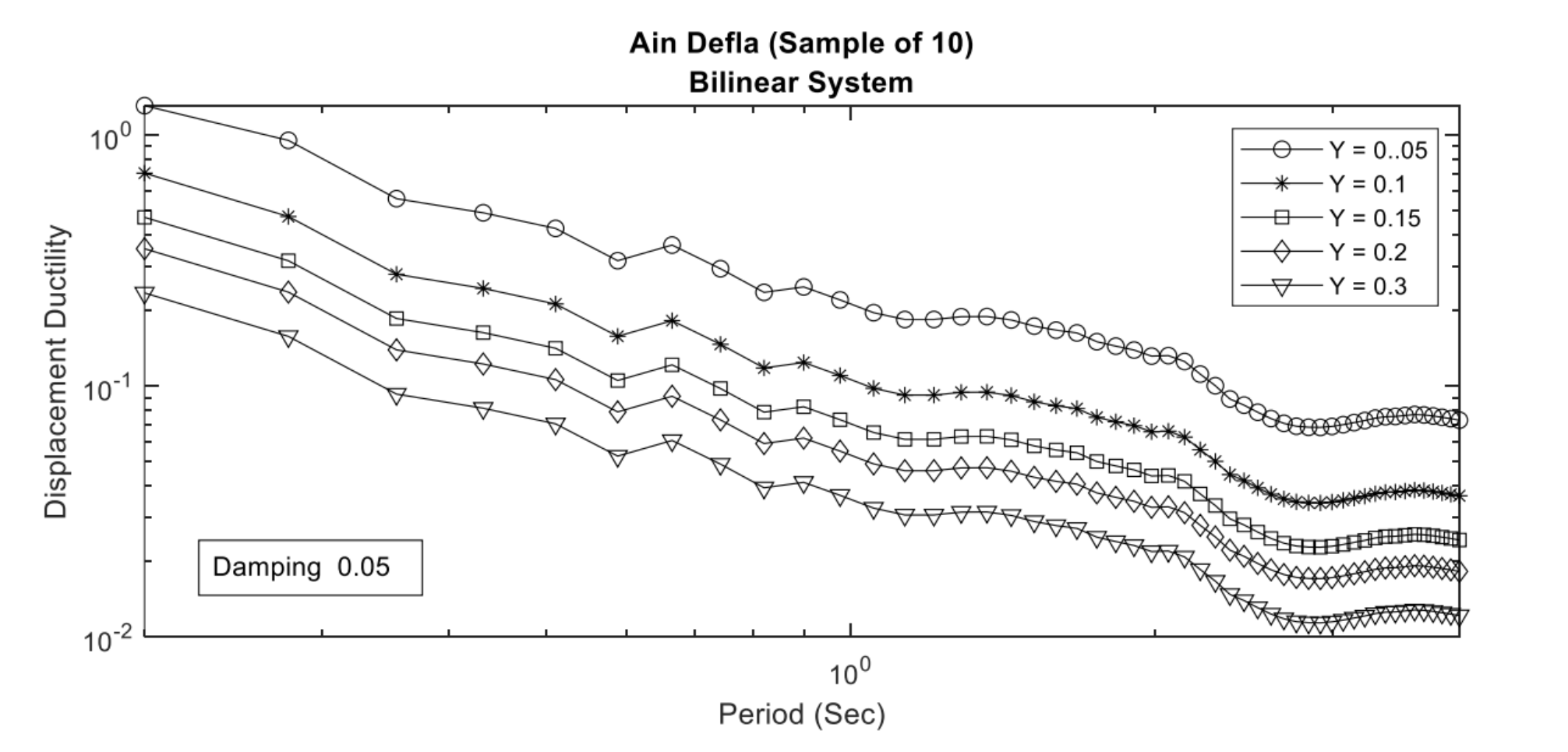
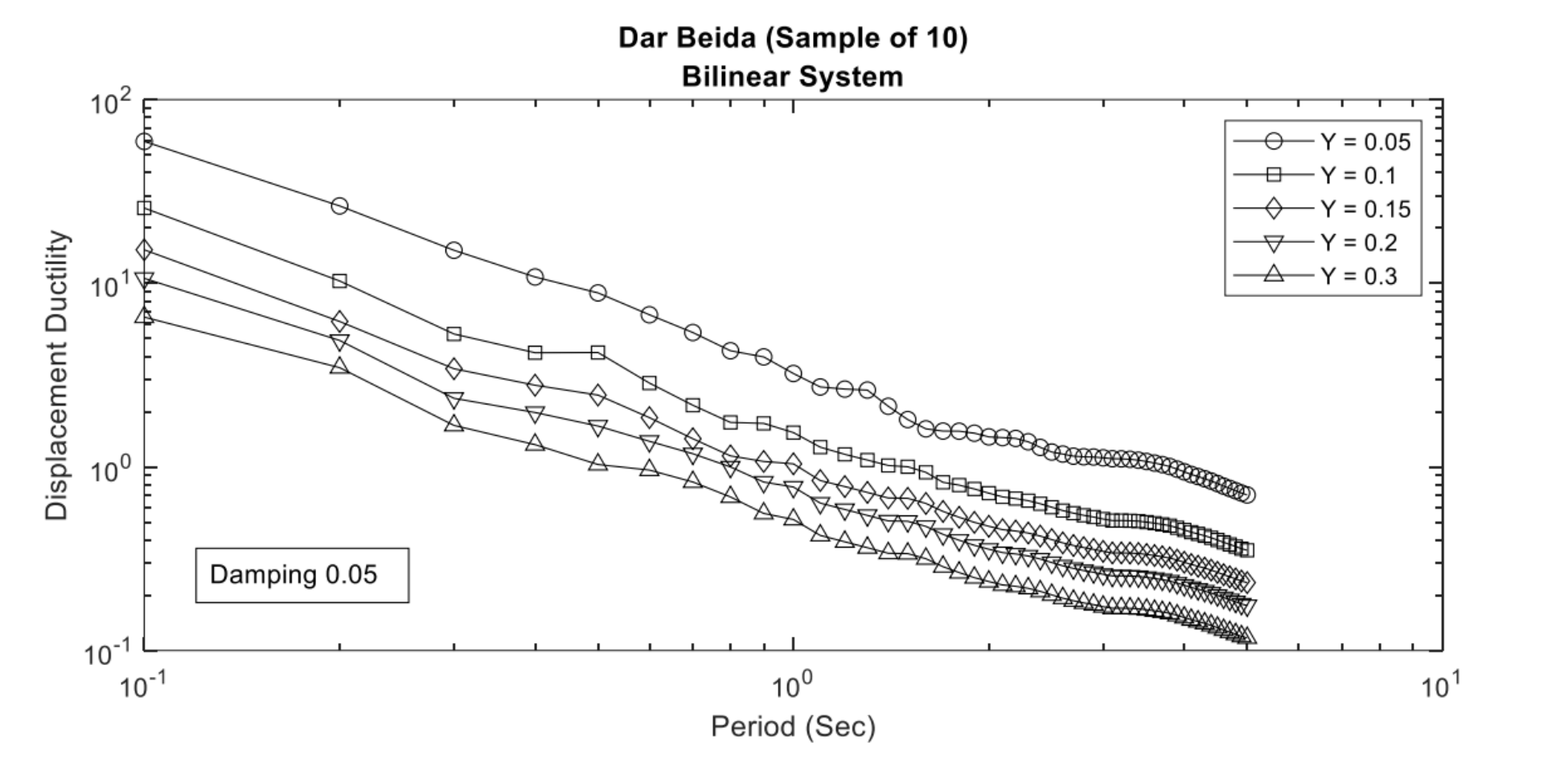
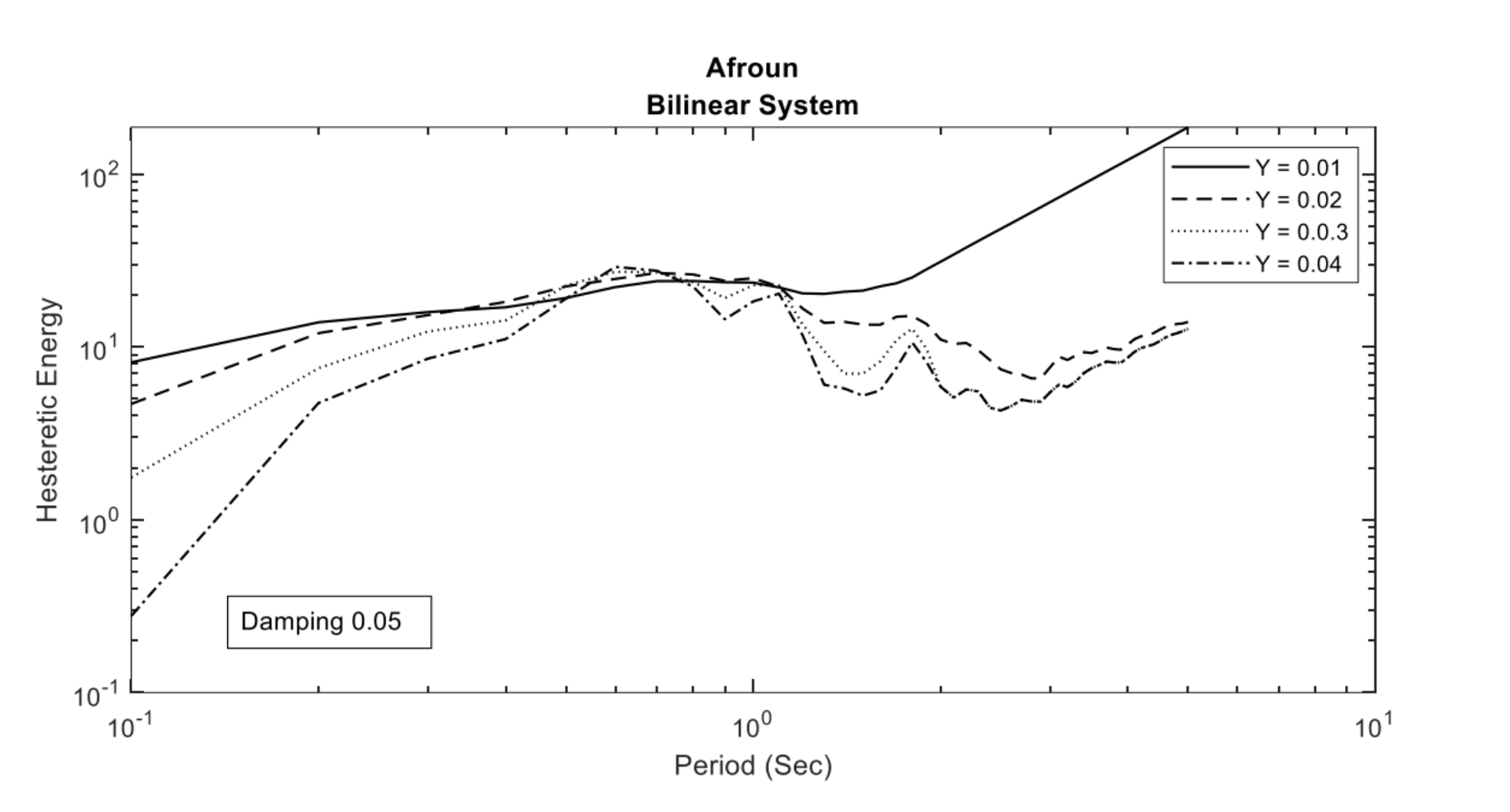
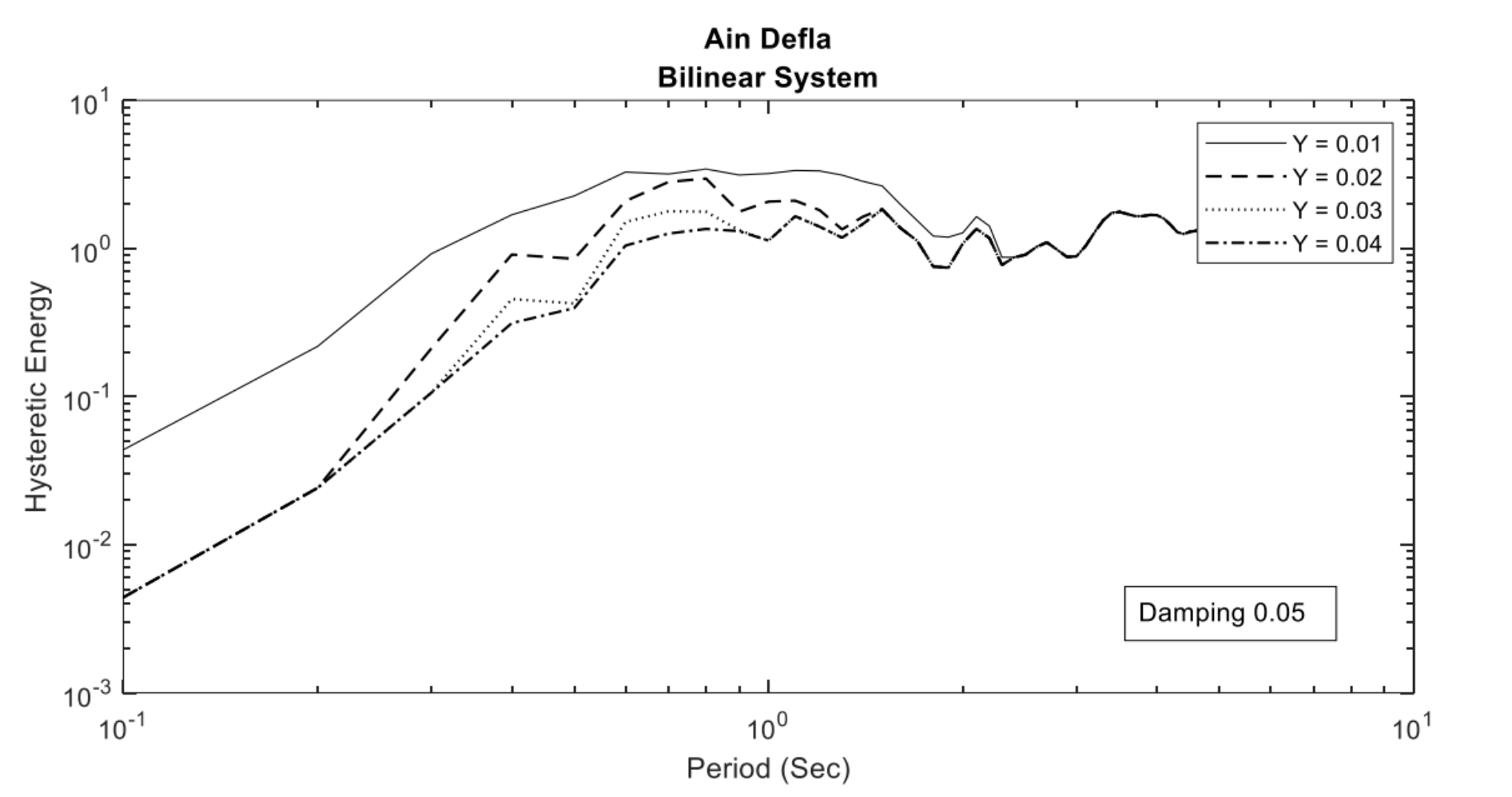
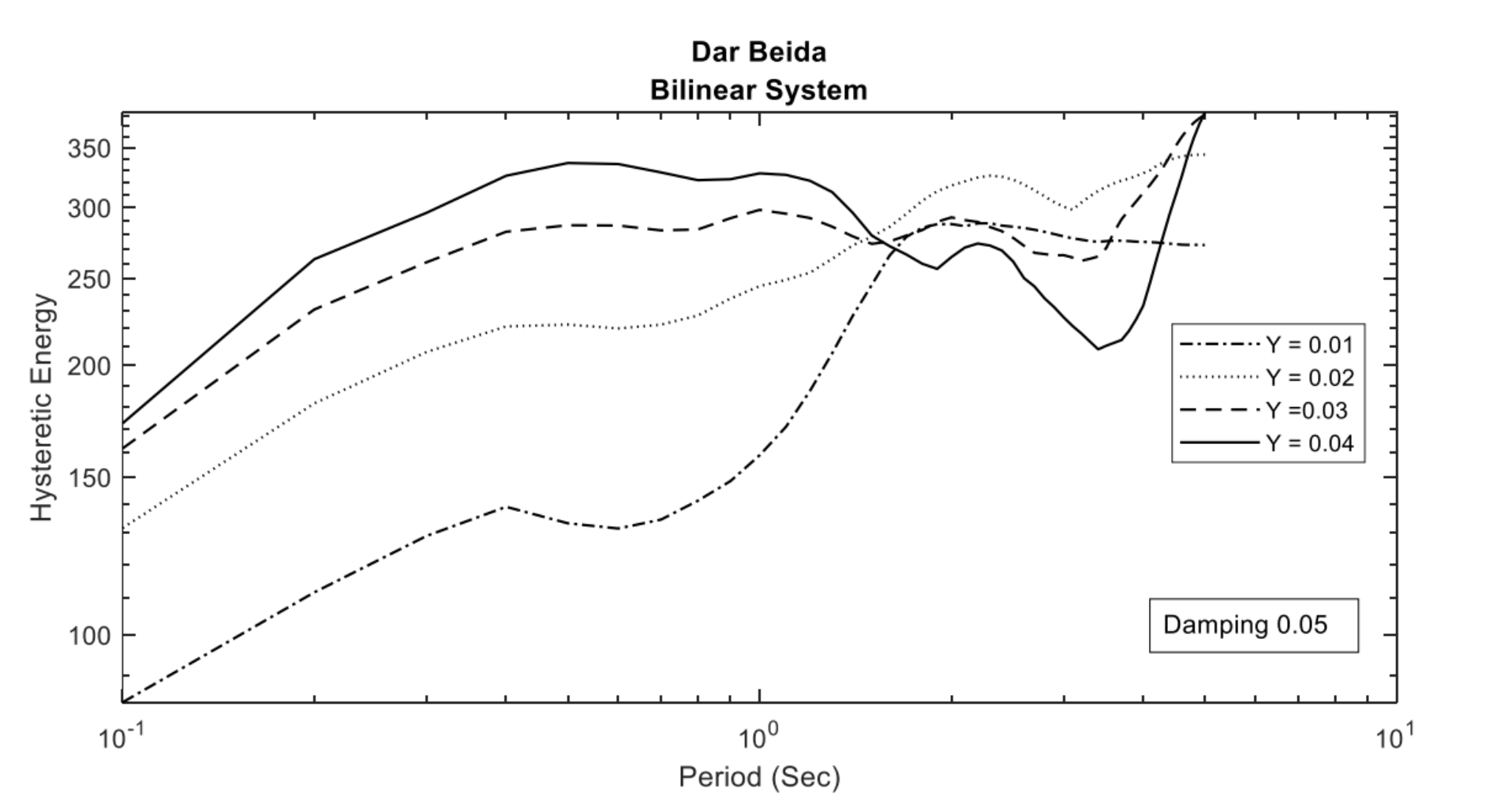
Therefore, in summary, two intriguing results were found for this structural analysis. First, the logarithms of the average nonlinear demand spectrum and of the period were linearly related. Second, through the use of normalized hysteretic demand spectra and mean displacement ductility, the following statements were obtained:
where ,
,
,
are constants, and T is period.
Conclusions
With a limited number of methods, the use of ARMA simulation gives generally reliable results, but it requires a large number of assumptions and restrictions on the number of parameters. In addition, it has been used that through the use of simulated ARMA process systems in a time-based approach, good results can be achieved with a limited number of parameters. It has also been studied that the logarithms of the average nonlinear response spectrum and the natural period of the system are linearly related. Finally, as the period value of the system increases, a decrease in its mean displacement ductility and hysteretic energy is observed.
References
Barlett, M.S., 1964, “on the theoretical Specification of sampling properties of autocorrelated time series,” Jour. Royal Stat. Soc. B8, 27.
Box G.E.P., and Jenkins, G.M., 1976, “Time Series Analysis, “ Holden-Day, Oakland, California, pps Conf. on System Science, University of Hawaii, pp. 187-189
Kozin, F., Lee, T.S., 1976, “Consistency of Maximum Likelihood Estimators for a Class of Nonstationary Models,” 9th Hawaii Conf. on System Science, Univ. of Hawaii, pp. 187-189.
Kozin, F., 1977, “Estimation and Modelling of nonstationary Time Series,” Proc. Symposium on Applied Computational Methods in Engineering, Univ. Southern California, Los Angeles.
Kozin, F., and Nakajimi, F., 1980, “The Order Determination Problem for Linear Time-varying AR models,” Transactions on Auto. Control, IEEE, Vol. AC-25, No. 2.
Kozin ,F., 1988, “Autoregressive Moving Average Models of Earthquake Records,” Probabilistic Engineering Mechanics,(to be published).
Kozin, F., Gran, R., 1973, “Analysis and Modeling of Earthquake Data,” Paper No. 364, Proc. 5th World Congress, Earthquake Eng., Rome.
Lawrence M.,1986, “A Random Variable Approach to Stochastic Structural Analysis, “Doctoral dissertation, University of Illinois, Urbana.
Liu, S.C., 1970, “Synthesis of Stochastic representation of Ground Motions,” Bell System Technical Journal, Vol.49, pp521-541.
Nakajima, F., Kozin, F., 1979, “A Characterization of consistent Estimators, ” IEEE Trans. Auto. Control. Vol. 24, pp. 755–765.
Shinozuka, M.,1973, ” Digital Simulation of Ground Accelerations Proc. 5th WCEE, Rome
.Jenkins, G.M. and Watts, D.G., 1968, “Spectral Analysis and its Application,” Holden-Day
Clough, R.W., and Penzien, J.,1975, “Dynamics of Structures, “McGraw Hill, New York.
Robinson, E.A. 1957, Predictive Decomposition of Seismic Traces,” Geophysics, Vol. 22, pp. 767-778
Akaike, H., 1974, “ A New Look at Statistical Model Identification, “IEEE Trans. Auto Contr. Vol. 19, pp. 716-723
Ellis, G.W., and Cakmak, A.S.,1987, “Modelling Earthquake Ground 7 Motions in Seismically Active Regions Using Parametric Time Series Methods, “National Center for Earthquake Engineering Research report No NCEER-87-0014.
Quenouille, M.H., 1957, “ Analysis of Multiple Time Series, “Hafner, New York
Boore, D.M., and Atkinson, 1987, “Stochastic Prediction of Ground Motion and Spectral Response Parameters at hard-Rock Sites in Eastern North America,” Bull. Seismo. Soc. Am., pp. 440-465