The Technology
Data Mining Technology (DTM) is a well-developed technology that assists organizations to make the best informed decisions rather than assumptions and guesswork by mining or extracting useful information from large volumes of data set collected in the past (Wook, Yusof, & Nazri, 2014). The DMT consists of different approaches and techniques that have been thoroughly deployed in business and industry processes. It has been noted that data mining techniques applicable in these organizations can also be applied in the education sector, specifically higher education. The paper proposes the implementation and use of data mining in the Canadian University Dubai.
Data mining refers to “the extraction of hidden predictive information from large databases, and it is a powerful new technology with great potential to help companies focus on the most important information in their data warehouses” (Thearling, 2012, p. 1). Given the robust nature of data mining technology, it can be applied in understanding unique elements of data stored in educational databases (Luan, 2004). The aim of mining data in the education environment is to enhance the quality of education for the mass through proactive and knowledge-based decision-making approaches. By assessing such benefits, Institutions of Higher Learning should focus on Data Mining Technology approaches as new strategies for comprehending and improving processes of learning and teaching. In fact, higher education requires technology to enhance competition and improve educational outcomes.
While some institutions of higher learning have made significant investments in Data Mining Technology, they have paid much attention either on the invention of complex algorithms or technical elements (Luan, 2004). However, these institutions have not indulged much about user perception of data mining technologies. In addition, not many studies have focused on understanding utilization of data mining technology that could inhibit adoption and appreciation (Jan & Contreras, 2011). Just like other technologies applicable in different environments, user support is extremely critical for such technologies to succeed; otherwise, DMT may experience low rates of adoption and acceptance irrespective of notable outcomes and benefits (Jan & Contreras, 2011). It is therefore imperative to observe user behaviors before proposing and implementing any technologies. This approach would reduce low usage rates or utter abandonment.
Technology Acceptance Model (TAM) and various models have been developed to understand specific user behavior toward technology adoption. Hence, it is advisable for institutions of higher learning to integrate such tools in their strategies before adopting DMT.
Who use it?
As previously noted, several organizations, businesses and industries use data mining technology to enhance decision-making. That is, data mining technology cuts across all sectors and organization, including small and mid-sized organizations. For instance, one pharmaceutical firm has been analyzing the past sales activities to enhance customer targeting and identify the most robust marketing strategies. Financial institutions have jumped into data mining to leverage abundant data from customer transactions for credit scoring and identify customers who are most likely to apply for new credit facilities. Customer experiences and needs can be used for market segmentation. Finally, retailers may mine data from loyalty cards to understand buying patterns, improve sales processes and customer experience. Generally, any organization can apply Data Mining Technology, including universities.
How it works?
Data Mining Technology in education is possible. Educational Data Mining (EDM) is “the area of scientific inquiry centered on the development of methods for making discoveries within the unique kinds of data that come from educational settings” (de Baker, 2010, p. 2). It also reflects the use of data mining techniques to a given set of data in an educational setting.
Data analysts or scientists have used different techniques and a wide range of methods, such as clustering, prediction and relationship mining, decision tree, k-means, Bayesian networks and neural network among others (Erdoğan & Timor, 2005). These techniques and methods are applicable in educational setting and data (Romero & Ventura, 2010). Specifically, neural networks, Bayesian networks and decision trees have been noted as more appropriate for the education sector (Romero & Ventura, 2010).
In education, data mining focuses on analysis of large volumes of data from various sources for improved comprehension of learning, processes and outcomes. According to figure 1, the process of Educational Data Mining involves transformation of large volumes of data into valuable knowledge for users.
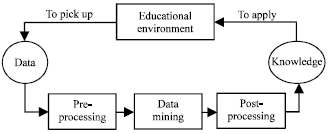
Figure 2 shows the “phases, and the iterative nature of a data mining project” (Oracle, 2015, p. 1). From this process, one can observe that the flow of the process never stops even if a specific solution is found and implemented. Instead, new questions emerge, which can be subsequently used to create models that are more robust.
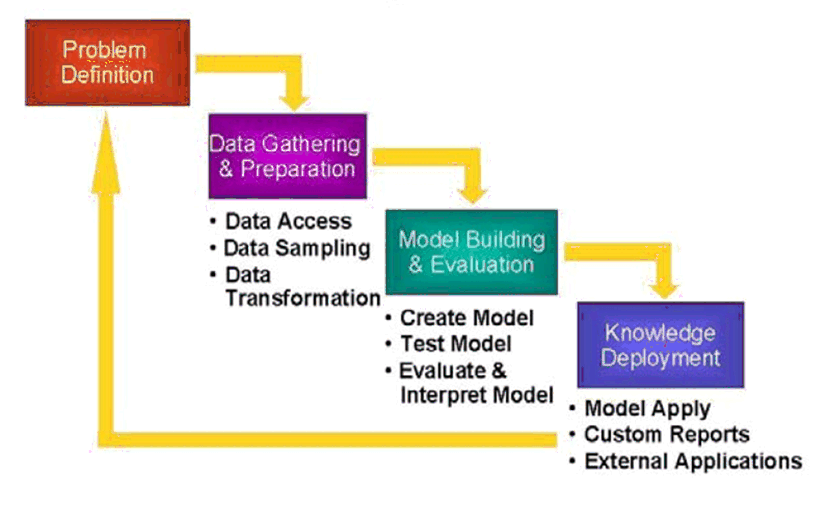
Data Mining Technology is useful for gaining access to data from both structured and unstructured sources – from the Web and traditional data storage tools.
Data can be mined to determine or predict possible behaviors and trends of learners in course session. These behaviors and trends may include performance and curriculum. For instance, effective deployment of Data Mining Technology can predict students’ failure or success in a given course. Classifiers or variables are used to determine relationships between variables in predicting outcomes. At the same time, the university can also determine dropout rates and develop appropriate intervention systems to control the number of learners inclined to dropout from their courses.
At the same time, the recent use of Data Mining Technology has increased within the educational Web-based setting. For instance, data obtained from e-learning, learning management applications, tutoring systems and adaptive systems have been crucial in helping educators to better comprehend learners’ online learning behaviors and trends.
The predictive approaches rely on students’ activities and other related pieces of information, including time spent online, assignment submission, content studied and study results. In this case, educators can use results from predictive analytics to identify at-risk students. Consequently, they can design the most effective intervention methods to enhance outcomes for such students. In this regard, educators and institutions can rely on Data Mining Technology to enhance learning environments for learners and improve their operational activities.
TCO of such technology
Many Data Mining technology tools are available in the market today. Data mining tools such as WEKA by the University of Waikato, New Zealand are absolutely free. On the other hand, some vendors, such as Oracle, SAP, IBM SPSS and SAS offer these data mining tools at varied costs, which could be significant. Hence, it is imperative to understand whether the CUD can deploy open source tool or use most touted expensive data mining software from specific vendors.
Costs associated with hiring or training data scientists for the University could be enormous. It is imperative to note that there are currently no enough data scientists to meet the rising demands.
Literature Review about Selected Technology
Many scholars have recognized myriads of challenges facing higher learning institutions (Delavari, Phon-Amnuaisuk, & Beikzadeh, 2008). Specifically, decision-making processes have become more difficult because of many interrelated factors within the education system. In this regard, data mining by using more efficient technology and expertise has been identified as a technique that can assist universities in decision-making processes. Information needed to facilitate decision-making lies in databases possessed by universities. Only tools and techniques of are required to gain new knowledge from data (Thearling, 2012).
Researchers continue to develop new data mining models for use in higher education institutions (Al-Twijri & Noaman, 2015). These models are designed to facilitate decision-making processes and control elements of student admission and graduation. In this regard, major characteristics of learners that could lead to higher retention and graduation can be mined from available data and predicted earlier enough within the first semester (Raju & Schumacker, 2015).
For a long time, studies have continuously demonstrated that learners are unique, have diverse knowledge levels, learn at different paces, face different socioeconomic challenges and topic familiarity differs. On this note, an intelligent curriculum should account for unique needs of every learner rather than using a standard curriculum for all. Learning contents should be flexible, adaptive and regularly reviewed. This calls for analytics so that significant insights can be discovered and applied to shape the content for different learners and improve learning experiences (Wagner & Ice, 2012).
At the lower levels, higher education should use data mining for student acquisitions; course selection; improving performance; student work groups; retention; teacher effectiveness; and attrition (Schmarzo, 2014). These diverse applications show that higher education outcomes can be enhanced through Data Mining Technology. Data Mining can act as the basis for making informed decisions, changing curriculum design and delivery, learning content evaluation, student learning activities, resource allocations and outcome monitoring among others.
Universities need to go deeper with analytics beyond student acquisition and attrition rates. While it is a good starting point, deeper insights can be obtained by monitoring, for instance, students at greater risk of dropping a course or out of college. By identifying these issues earlier enough, higher education can develop programs to reduce dropout rates effectively.
Rapid expansion of universities, online education and technologies are putting much pressure on these institutions to increase performance and graduation rates. Fortunately, universities can benefit from data analytics to enhance education outcomes. Universities can realize and exploit the identified opportunities from studies and adopt data mining to demonstrate how they can solve higher education multiple challenges. The process requires collaboration with technology vendors and data experts to show how these new technologies can support learning in universities. Universities should adopt best practices and models for data mining to provide them with opportunities to transform learning experiences and outcomes for students, teachers and other stakeholders.
Applying the Technology in the Canadian University Dubai
The Data Mining Technology is proposed for Canadian University Dubai (CUD). As globalization increases, global universities have focused on expanding their reach. The University was established in 2006 in Dubai. It provides students with “Canadian education but with the respect of the culture and values of the United Arab Emirates” (Canadian University Dubai, 2015, p. 1).
The goal of the University is help every student to “move forward and ensure well-rounded lifelong learner” (Canadian University Dubai, 2015, p. 1). Consequently, it has focused on academic achievement and extracurricular engagement. While all these goals sound good, the University’s goals are most likely to take longer than necessary because it lacks robust decision support systems. The University has not adopted Data Mining Technology to gain useful insights from its existing database.
To demonstrate how the University can benefit from Data Mining Technology, various case studies will be used to show how it can overcome specific challenges, as well as benefits of the outcomes. For instance, the University can use Data Mining to understand students that take most credit hours, classes that are most likely to be popular, students that are most likely to come for additional classes, or even predict pledges that alumni will make.
A certain institution of higher learning wanted to create consequential learning outcome typologies, but it faced the challenge of limited understanding of student. To overcome this issue, unsupervised data mining was applied (Luan, 2004). A typical university would have an enrolment of about “15,000 with students grouped as ‘transfer based’, vocational or basic skill upgraders” (Luan, 2004, p. 4). These means of student identification contain basic information of what learners declared during enrolment. In addition, they do not reflect specific differences noted between each learner type. In this case, the university used data mining techniques to develop the exact typologies for its 15,000 students. The researchers applied two techniques of “clustering algorithms, TwoStep and K-means and used the algorithms on the three general classifications noted above and obtained mixed results” (Luan, 2004, p. 4). There were no distinctions between boundaries of clusters even after data cleaning and repeated measures. No significant improvements were observed in the result. Thus, the researchers concluded that the initial declaration at enrolment did not reflect actual behaviors of learners. They then applied a replacement technique by concentrating on “educational outcomes alongside lengths of study” (Luan, 2004, p. 4).
The educational outcomes were difficult to define. Specific learning period was required to ensure that a learner has attained a given milestone. Dropout was also assessed as an “outcome of learning while researchers had to deal with ‘stopouts’ – learners who dropped out but later returned to continue with studies” (Luan, 2004, p. 5). Hence, the data scientist must be able to account for all these diverse variables in order to answer specific typology question and research objective. After taking care of the outliers by eliminating or including them in other cluster, TwoStep algorithm generated the following clusters: “Transfers,” “vocational students,” “basic skills students,” “students with mixed outcomes,” and “dropouts” (Luan, 2004, p. 5). The researchers then used k-means to validate the generated clusters. The length-of-study was also factored into the variables for every cluster, and new perspectives emerged. “Some transfer cluster learners completed quickly; some vocational learners took longer; and other students appeared to simply take one or two courses at a time” (Luan, 2004, p. 5).
The results were informative for the university. From data mining, the university was able to understand demographic and other related information about student typologies. It was also established that “some older students took more time to complete their studies while younger ones with more socioeconomic advantages settled for high credit courses and completed studies faster” (Luan, 2004, p. 5). The university was able to group students as ‘transfer speeders, college historians, fence sitters and skill upgraders among others. The typologies ensured that the university could understand students beyond the normal homogenous grouping. The data mining project discovered hidden vital information that the university could use to meet diverse needs of learners.
Another demonstration to show how the University can apply Data Mining Technology is in the area of academic planning and interventions (Luan, 2004). In this case study, the college faced the most difficult challenge of accurately predicting academic outcomes in order to develop appropriate interventions for learners. Colleges apply data mining techniques to determine learners that are at risk of low performance. Consequently, they can develop appropriate interventions to prevent failure even before learners realize the risks. Transferring through the four-year of academic at the university is the main objective of many students. However, academic challenges lead to extended periods of transferring while other students completely fail to transfer. Traditionally, it has been difficult to understand these issues and transferring among students. However, data miners can mine and match data available from various sources to understand behaviors and characteristics of students that transfer or fail to transfer. Thus, data scientists and decision-makers can relate these data and academic behaviors and outcomes of students to determine transfer outcomes.
The solution to the transfer issue was found after application of data mining techniques. Various typologies and domain knowledge were used to develop appropriate data mining model (Luan, 2004). In this case, it was determined that transfer education domain knowledge the most reliable means of handling transfer was to identify transfer-oriented students within the earliest opportunities. Training students who are potential candidate to transfer is more relevant than concentrating on students who have gathered adequate points to transfer. By relying on transfer outcome data, data miners developed “a dataset with different students’ variables under the major transfer clusters of laggards and speeders” (Luan, 2004, p. 5).
The researchers then created a test dataset and validation dataset from the original dataset through proprietary randomization technique (Luan, 2004). Transfer was regarded as outcome variable while other variables included “units earned, courses taken, demographics, and financial assistance were classified as predictors” (Luan, 2004, p. 5). Thus, they were analyzed without a focus on stepwise testing for significance (Luan, 2004). The data mining process “tolerated interactions between variables and non-linear relations” (Luan, 2004, p. 5). The researchers used supervised data mining for the study. Thus, neural network and rule induction algorithms were “performed at the same time for ease of comparing and contrasting the accuracy of the prediction” (Luan, 2004, p. 5).
The result allowed the college to identify students with better transfer opportunities. The extensive machine learning through “neural network algorithm increased the accuracy of the prediction” (Luan, 2004, p. 5). Thus, the researchers could easily identify patterns found in the data.
These are just few cases, which illustrate how the Canadian University Dubai can apply Data Mining Technology to improve learning and teaching outcomes. Robust algorithms and tools can be applied with highly qualified data scientists to help the University to attain its goals.
Discussion and Analysis
One major issue that institutions of higher learning encounter now is predicting possible outcomes of their students and alumni. It is necessary for universities to determine enrolment and students that would require help to transfer. Other issues associated with traditional management of learning continue to motivate universities to seek for better alternatives. Consequently, some universities have noted the potential of applying Data Mining Technology to overcome such challenges. Data mining gives different organizations abilities to exploit their current data resources and data mining tools, techniques and expertise to uncover and comprehend hidden patterns in large databases. The identified patterns are developed into data mining models to provide useful information that universities can use to predict student behaviors. From the insight obtained, universities can allocate teaching and learning resources more accurately and effectively.
Data Mining Technology would, for instance, provide an opportunity and information for the university to predict drop out and develop appropriate interventions to avert drop out or possible provide more resources for a given course based on the prediction.
Past studies in the subject of data mining and its application in higher learning reveal a powerful tool that can transform the education sector. At-risk students can be identified and get the necessary support they require, for example. In addition, there are specific functional areas in higher education that analytics and prediction can be applied to support outcomes. Analytics, for instance, can be applied in critical areas such as finance and budgeting, enrollment, instructional and student progress management among others (Mattingly, Rice, & Berge, 2012).
Although few institutions of higher learning have embraced Data Mining Technology, they can leverage student performance, usage, behaviors, faculty performance and social insights such as tendencies, propensities and trends to maximize learner engagement processes, reduce rates of attrition, enhance lifetime value and promote learning advocacy (Schmarzo, 2014).
Summary
The paper proposes the implementation and use of data mining in the Canadian University Dubai. Data Mining refers to the “extraction of unknown predictive information from large databases” (Thearling, 2012, p. 1). The technology is considered powerful with robust predictive analytics that can assist organizations to concentrate on valuable data stored in their databases. Data Mining Technology helps organizations to predict possible future trends. From the results, organizations can then act proactively based on available knowledge and, therefore, decisions can be more reliable. It is imperative to recognize that data mining is robust and goes beyond data analysis. It includes extraction of large volumes of data from various sources, which are analyzed to support decisions. Many data mining tools, including open, freely available ones can tackle some challenging issues that were once considered complex or tedious. These tools can scour multiple databases for any information that is predictive and can provide potential future behaviors.
Results from organizations, including few institutions of higher learning that have embraced data mining show that data mining is a robust analytical tool that can assist organizations to overcome some challenges. For instance, institutions of higher learning can use data mining techniques to understand student behaviors, improve resource and staff allocation and enhance relationships with alumni. The hidden patterns can provide critical information based on predictive models to manage issues of enrolment, dropouts, graduation and even alumni.
Different organizations have deployed data mining tools and techniques to analyze large volumes of data and get insights that can aid decision-making. Canadian University Dubai can also adopt Data Mining Technology for analytical purposes. It however requires expertise in data science and effective machine learning tools that can handle large volumes of structured and unstructured data from different sources.
References
Al-Twijri, M. I., & Noaman, A. Y. (2015). A New Data Mining Model Adopted for Higher Institutions. Procedia Computer Science, 65, 836–844. Web.
Canadian University Dubai. (2015). About the Canadian University Dubai. Web.
de Baker, R. J. (2010). Data Mining for Education. Oxford, UK: Elsevier.
Delavari, N., Phon-Amnuaisuk, S., & Beikzadeh, M. R. (2008). Data Mining Application in Higher Learning Institutions. Informatics in Education, 7(1), 31–54.
Erdoğan, Ş. Z., & Timor, M. (2005). A Data Mining Application in a Student Database. Journal of Aeronautics and Space Technologies, 2(2), 53-57.
Jan, A. U., & Contreras, V. (2011). Technology acceptance model for the use of information technology in universities. Computers in Human Behavior, 27(2), 845–851. Web.
Luan, J. (2004). Data Mining Applications in Higher Education. Chicago: SPSS Inc.
Mattingly, K. D., Rice, M. C., & Berge, Z. L. (2012). Learning analytics as a tool for closing the assessment loop in higher education. Knowledge Management & E- Learning: An International Journal, 4(3), 236-247.
Oracle. (2015). Data Mining Concepts. Web.
Raju, D., & Schumacker, R. (2015). Exploring Student Characteristics of Retention that Lead to Graduation in Higher Education Using Data Mining Models. Journal of College Student Retention: Research, Theory & Practice, 16(4), 563-591. Web.
Romero, C., & Ventura, S. (2010). Educational Data Mining: A Review of the State of the Art. IEEE Transactions on Systems, Man, and Cybernetics, Part C: Applications and Reviews, 40(6), 601 – 618. Web.
Schmarzo, B. (2014). What Universities Can Learn from Big Data – Higher Education Analytics. Web.
Thearling, K. (2012). An Introduction to Data Mining. Web.
Wagner, E., & Ice, P. (2012). Data Changes Everything: Delivering on the Promise of Learning Analytics in Higher Education. EDUCAUSE Review, 47(4).
Wook, M., Yusof, Z. M., & Nazri, M. Z. (2014). Data Mining Technology Adoption in Institutions of Higher Learning: A Conceptual Framework Incorporating Technology Readiness Index Model and Technology Acceptance Model 3. Journal of Applied Sciences, 14, 2129-2138. Web.