Introduction
Operation of organisations requires the possession of an immense wealth of information, which makes the application of data warehouses and data mining valuable in modern business operation environments. Fundamentally, enterprise data warehouse, alternatively termed as a data warehouse, refers to databases that are deployed in data analysis coupled with reporting (Inmon 5).
Data warehouses are meant to provide a storeroom for historical and recent data that is deployed for the making and dispensation of information that are utilised when preparing superior management plans such as the assemblage of periodical and yearly reports for comparison purposes.
Data contained in the warehouses is normally uploaded to various operational systems of an organisation including sales and marketing, among others. Opposed to data warehouse, data mining refers to “the computational process of discovering patterns in large data sets involving the methods of intersection of artificial intelligence, machine learning, statistics, and data systems” (Haughton et al. 290).
The main aim of putting in place a system for data mining in an organisation is to provide a means of retrieving reports from data stores with the overall objective of converting the retrieved reports into forms, which can be altered into an appropriate arrangement.
Considering that both data warehouse and data mining may avail an incredible data to an organisation, which can be deployed in making vital decision and or aid in taking corrective measures upon its analysis, it sounds imperative for all nations to have organisations placing central emphasis on data warehouse and data mining.
This paper aims at conducting a comparative analysis for the extent to which data warehouse and data mining have been deployed in the UAE and Europe. Before this section, a brief history of data mining and data warehouse is considered.
Research Project Questions
Data warehouse and data mining are incredible in providing business support solutions. From this assertion, the two important research questions related to this research include:
- In which industry are data warehousing and data mining most beneficial? As revealed later, data mining and data warehousing have been applied in science and engineering, surveillance, medical fields, and in the business field in both the UAE and the international arena. However, it is most beneficial in the business field.
- Is the application of data mining and data warehousing a reserve of some organisations, and to what extent are they utilised within the UAE and other parts of the world? In response, data mining and data warehousing are in all organisations, with the UAE organisations using it the most in all its organisations.
History of data warehouse and data mining
Data warehouse
The concept of data warehousing was first introduced at a practical level in the 1980s. In this time, an IBM researcher Barry Delvin and his colleague Paul Murphy developed the concept with the intention of providing a model for enhancing the flow of various forms of organisational data from an operation system into an environmental decisions support system.
With regard to Inmon, “the concept attempted to address the various problems associated with this flow, mainly the high cost associated with it” (13).
The circumstances leading to the establishment and development of the concept of data warehousing was attributed to the fact that failure to have a data warehouse led to the need of putting in place large amounts of redundancy to help in supporting a myriad of decision support environments.
As information systems within large organisation become complex coupled with disintegration of a single organisation into several parts to enhance service delivery and specialisations, it becomes crucial for each department within an organisation to have its information database and buffer systems.
Consequently, “…in large corporations, it was typical for multiple decision support environments to operate independently” (Zhu and Davidson 67). For instance, in a business set up, an organisation can implement a data warehouse plan that monitors clients’ purchases, as shown below.
Source: (Browning and Mundy)
However, even though different departments held different information, each department’s operations depended on the information held by other departments. Data stored by various departments within an organisation is also similar in one way or another.
For this reason, Barry Delvin and his colleague Paul Murphy found it necessary to develop a “process of gathering, cleaning and integrating data from various sources, usually from long-term existing operational systems, which could be replicated for each environment” (Zhu and Davidson 71).
Although the 1980s marked the period in which the concept of data warehousing was developed into a form that could be utilised within an organisation, the initial formulations of the concept can be traced as early as1960s. The pyramid below shows the beneficiaries of data warehousing and the extent to which they use the technology.
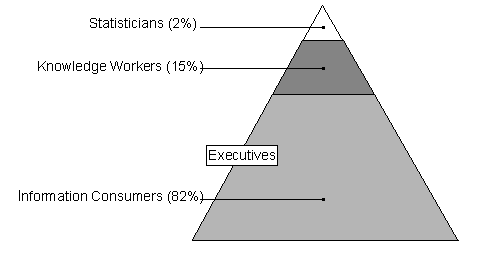
In 1960, General Mills came up with the terms facts and dimensions as they are applied in data warehousing. A decade later, IRI together with ACNielsen speared headed the invention of data marts utilised in retail sales.
In the same decade, Bill Inmon attempted to define the term data house. In 1975, Sperry Univac introduced the concept of MAPPER. It refers to “data management and reporting systems that include the world’s first 4GL, which formed the first platform specifically designed for building information centres (a forerunner of contemporary enterprise data warehousing platform)” (O’Brien and Marakas 93).
The work acted as an insight into the work of Taradata in 1983 who designed and practically tested a database management system meant to enhance decision support. Development of data warehouse in the application of decision support systems acquired principal focus in the 1990s when Raph Kimball introduced the red brick warehouse.
This data management system was particularly designed for data warehousing. Bill Inmon developed further this work when he designed software for data warehouse development in 1991. Later, in the year 2000, Daniel Linstedt released Data Vault.
Data Mining
Data mining has established central roles in knowledge engineering and artificial intelligence discourses. It refers to “the process of discovering meaningful correlations, patterns, and trends by sifting through large amounts of data stored in databases” (Haughton et al. 290) following the procedure below.
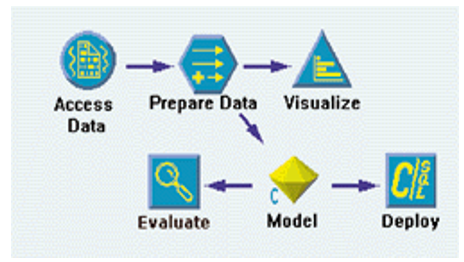
Its roots are ingrained in statistics and machine learning though applied mainly in computer science. The subject of data mining has attracted an immense interest over the last two decade, although its study started four decades ago. Data mining started as a statistical analysis tool advocated for by two main companies: SPSS and SAS (O’Brien and Marakas 31).
Nevertheless, some of the applications of data mining in the past four decades remain relevant today. They include regression analysis and cluster analysis, among others. The modern statistics routines used in data mining have incredibly improved on the past application of data mining.
New approaches to data mining like heuristics, fuzzy logic, and neural networks began to gain substantive scholarly attention in the 1980s. With improved computational power of computers, more extensive analysis and analyses that are more powerful could be done through interactive classification.
Workshops on data mining, alternatively called knowledge discovery in databases, were first held in the 1990s. However, such conferences have been incredibly on the hike over the last decade. Precisely, data mining can be traced from three primary sources. These are statistics, artificial intelligence, and machine learning. Data mining can be accomplished through simple strategies such as graphing coupled with charting.
A good example of enhancing data mining through charting is the case of scatter graphs. In an organisation, data mining has broad applications including identification of customer patterns, finding various associations existing between various demographic traits of clients, and identification of the most royal customers, among other tasks. Arguably, multiple departments within an organisation need such information.
Comparison and evaluation of data warehousing and data mining in the UAE vs. International level
Different nations across the globe, including the UAE, have received and applied data warehousing and data mining in a variety of fields.
Data mining has been applied in science and engineering, surveillance, medical fields, and in the business field in both the UAE and the international arena. However, this section discusses the application of data mining and data warehousing in business only since other application are beyond the scope of the paper.
In commercial applications, data warehouse and data mining are incredibly helpful in analysing various chronological performances of a company often available in the company’s records in the form of inactive data. The purpose of the analysis of data is principally to unveil trends and patterns of hidden business performance.
This task is accomplished in data mining through the deployment of data mining software, which makes use of “advanced pattern recognition algorithms to sift through large amounts of data to assist in discovering previously unknown strategic business information” (O’Brien and Marakas 107). Data mining has been used throughout the world, including in the UAE to perform several business-related tasks.
The tasks include market analysis in the effort to identify potential new products, determine the causes of manufacturing problems, prevent withdrawal of some customers from consuming an organisation’s products, acquire new customers, generate customer profiles for purposes of evaluation, and determine the appropriate marketing strategies to sell across both existing and potential customers.
Valid examples of global organisation have invested heavily on data warehousing and data mining to enhance their performances in the dynamic business environment. For instance, “Wal-Mart processes over 20 million point-of-sale transactions every day” (O’Brien and Marakas 141) with the transactions’ information being contained in a centralised database.
Every department within Wal-Mart has to extract information that is necessary to perform its operations from the database. Although information extracted this way is crucial for the making of decisions that would help to better the performance of every department within the company, such raw data is not of any use without having some sort of software to analyse the data.
The need to analyse such data underlines the significance of data mining software within any company having a large pool of customers and with global operations.
For the case of Wal-Mart, the use of data mining techniques to conduct an analysis of the information contained in the centralised database enables the company to develop necessary campaigns for marketing and even predict loyalties developed by customers in relation to a myriad of brands offered by the company with better precision.
Data mining techniques are also helpful in aiding to build and manage customer relationships across all organisations in the world, including in the UAE. From the analysis of data contained in a data warehouse of an organisation using data mining techniques, the UAE organisations have ceased from maintaining a centralised call centre for contacting potential customers.
Rather, the focus shifts to targeting customers who have the highest probability for responding to a given offer from the analysis of their purchasing patterns.
Additionally, with regard to Zhu and Davidson, from the analysis of the data contained in a data warehouse using data mining statistical and other tools, “more sophisticated methods may be used to optimise resources across campaigns so that one may predict to which channel and to which offer an individual is most likely to respond (across all potential offers)” (88).
Once analysis is done to determine response patterns for potential clients for an organisation’s products, automation of mails can be done in the effort to solicit the potential customers to respond. This practice has been well developed and used not only in the UAE but also in the global arena.
Real world examples for the application of data warehousing and data mining used by the UAE organisations
Data warehouses and data mining used by the UAE are available in a variety of places across the globe. For instance, they are used in India in health care settings. One of such practical application is the use of data mining to aid in decision making as tools for evaluation of treatment choice for various ailments such as fibroids (Campbell 201).
In the installation process of such software, the main challenging question that appeared was whether data mining could indeed be applicable in facilitation of the treatment process for fibroids to provide means of predicating possible treatment choices.
If the answers were yes, the hospital technologists within India questioned on the most appropriate data mining techniques that would help to predict the outcomes of various treatment options. In the effort to address these queries, generation of an archive of data relating to different available treatment options and the responses of patients upon undergoing the procedure were maintained.
Based on the characteristics of the patients, analysis of the data warehouse helps to predict the most viable treatment option that is consistent with every patient’s fibroid treatments needs in India.
Extension of this decision through data mining and database warehouse in India to the diagnosis and treatment of other complex ailments has seen the nation rise up as one of the nations in the world housing the most successful treatment facilities with many cases of ailments requiring advanced treatment being referred to India.
Oracle software: how it supports the business
The Oracle Company makes and sells various software applications for business support across the globe. The oracle software carries a myriad of functional modules deploying RDBMS, which functions as back-end.
The various functional modules ingrained within business support software produced by oracle include Oracle HRMS (human resource management systems), oracle CRM (customer relations management), Oracle financials, oracle projects, and oracle procurement among others.
The first business support software to be released by oracle was oracle financials in the 1980s. Later, the company released a myriad of business support software, including the database warehouse management systems and data mining software.
The oracle data-mining module (ODM) is essentially an optional relational data management system (RDBMS). It constitutes data analysis and data management algorithms designed to support business through easing tasks such as feature selection, classification of data, regression analysis, detection of various anomalies, making predictions, and other specialised analytics necessary when making organisational decisions.
With regard to Davenport, oracle data mining software is pivotal in “providing a means of creation, management, and operational deployment of data mining models inside the database” (214). Making of decisions within an organisation requires immense scrutiny and analysis of quantitative data that helps to explain trends in business operations and in general the industry trends.
Purposing to help an organisation to realise these functions, regression analysis-support and modules are incorporated into the ODM. The implementation of various business functional-support modules are done such that “the implementations are integrated right into the oracle database kernel operating natively on stored data in the relational database tables” (Davenport 215).
From these databases, different departments of an organisation can tap the necessary data, which upon its analysis helps in making vital decisions.
Use of Carrefour Technology in Europe and in the UAE
Carrefour is one of the leading global distributors of various commodities. The company has various classes of distribution networks such as supermarkets and hypermarkets coupled with convenience and hard discount stores in America, Europe, the UAE, and other regions in the world. The organisation has been endeavouring to maintain its leadership within a competitive grocery industry.
The company planned to achieve this goal through “gaining control over its marketing process and more effectiveness in leveraging its business intelligence- with the ultimate aim of strengthening customer loyalty” (IBM Para 4).
The solution to resolve challenges facing Carrefour was arrived at by strategically deciding to partner with IBM coupled with other parents to design and implement a system for in-house promotion throughout the supermarkets and hypermarkets owned by the organisation.
This strategy was found to be incredible in aiding to plan and execute more focused marketing plans, which would ensure rapid assessment of the campaign feedbacks. It was essential in the quick evaluation of marketing efforts of the company. Getting information on how customers respond to a given promotional campaign may be measured from various dimensions.
The most significant way of measuring is assessing changes in sale levels of an organisation. Given that Carrefour has many retail stores across the globe, garnering all such information requires the creation of computer-enabled interfaces.
Faced with the need of rapid analysis of sales data, Carrefour had limited options rather than deploying data mining and data warehousing technology, which consisted of various solution components, which were an IBM Supermarket Application coupled with IBM DB2 from the paradigm of software.
Following the success of its technology in helping to establish better relationships with customers, suppliers, and in helping to plan for short but effective marketing campaigns, the Carrefour technology has been used in many European and the UAE organisations to the extent of being deployed in the retail industry and national organisations.
Pros, Cons, Strengths, and Weaknesses with respect to data warehousing and data mining systems
The goal of using data management systems is to facilitate decision-making processes in a more rapid way. However, irrespective of the extent to which systems may be helpful to achieve this noble purpose, no systems lack pros and cons, and strengths and weakness. In case of data warehouse, copies of information garnered form transactions within organisations are maintained.
This case provides an essential opportunity for an organisation to “congregate data from multiple sources into a single database so that a single query engine can be used to present data” (Inmon 52).
Data warehouse also makes it possible to keep historical records of transactions even though systems for the transaction may fail to do so. This data is utilised in making future forecasts, which help to inform critical activities of a business, including the sale and forecasting of production patterns in the future.
Through a data warehouse, it also becomes possible for an organisation to come up with a means of integration of data from a number of sources in the effort to create an overall means for decision making in an entire enterprise.
It also enables an organisation to provide data and information that is consistent since the results of the analysis are representative of both current and historical trends from the analysis of data using data mining tools.
Furthermore, data warehousing is essential in “restructuring data so that it makes sense to the business users, restructuring the data so that it delivers an excellent query performance, even for complex analytic queries, without impacting the operational systems, and adding value to operational business applications, notably customer relationship (CRM) systems” (Inmon 55).
Consequently, through data warehousing, it is possible to provide data models for all data of interest irrespective of the sources of the data within an organisation. Although data warehousing has all these merits, it has central weaknesses (cons) in that data is usually stored in a ‘soft’ form.
The link between data warehousing and data mining is that it is easier to mine data, which is properly housed meaning that the effectiveness of data mining is dependent on data housing. Consequently, data mining has the demerit that it cannot be effective without the existence of an integrated organisational information database.
In case databases are corrupted, or information contained in them is lost through malicious acts of some employees of an organisation or unauthenticated access to an organisation’s database systems, it implies that data mining software loaded in an organisation’s systems would have no data source from which to carry out analysis.
In addition, as Haughton et al. reckon, “if a data mining query has to run through terabytes of data spread across multiple databases, which sit on different physical networks, the query is not efficient and that getting results will take a long time” (301). This demerit puts a pre-condition to data systems developers since data warehouse systems must be developed such that they are able to interconnect with other databases.
Market analysis for data warehousing and data mining systems
The market for data warehouses and data mining systems is immense in the UAE and in the international fronts across many industries. For instance, in the discipline of human resource, data mining can be utilised in the identification of employees who are most productive to an organisation based on their historic efficiency and outputs recorded and maintained in an organisation’s integrated database (data warehouse).
This argument means that the market is substantive for the systems in organisations, which want to have an overall means for evaluation and interrelating data for different organisations’ departments for integration followed by the analysis of the integrated data in the effort to draw more decisions that are conclusive.
The market potential for data warehouse and data mining software has grown in multiple figures following the high demand for decision support systems in the field of engineering and general science. Such areas include education, genetics, bioinformatics, and mechanical and electrical engineering without negating the discipline of medicine.
For instance, with regard to Zhu and Davidson, “in human genetics, sequence mining addresses the important goal of understanding the mapping relationship between the inter-individual variations in human DNA sequence and the variability in disease susceptibility” (109).
The main aim for using data mining in the human genetics is to help in the determination of how alterations of gene structure influence the danger for contracting ailments such as tumour. This argument is significant in the attempt to design and implement strategies for ailment prevention, diagnosis, and treatments. Arguably, the subject of immunology is beyond regional physical boundaries between nations.
Consequently, data mining software such as the software for the multifactor dimensionality reduction would gain positive market reception in the UAE and internationally, including Europe. In fact, European nations have been committing hefty amounts of money to investments in the new immunology and diseases diagnosis technologies in the past three decades.
From a business dimension, the UAE has been noted as being a significant business hub within Middle East. Organisations operating in the region largely depend on information garnering, integration, and processing to make decisions for vibrant growth.
These organisations are able to withstand global market dynamics so that minimal impacts of global financial crunch are experienced in the UAE organisations in comparison to organisations operating in Europe. Growth and the ability to develop resilience to business dynamic depend on how much an organisation is risk-aware.
Analysis of the degree of susceptibility of an organisation to risks is dependent on cute establishment of the trends of business performance based on the historical data. Indeed, this goal cannot be realised without analysis tools such as data mining techniques.
As argued before, these tools and techniques cannot operate free of data. Since the goal of any organisation is to ensure its long-term operation in the effort to return value to its owners, data warehousing and data mining possess an incredibly high potential not only in the UAE but also globally.
Main suppliers’ systems
Data warehousing and data mining systems can be designed in-house within an organisation. However, such an attempt would attract hefty costs. The alternative is hiring the service of an external supplier of the systems. Such suppliers include IBM and Oracle Foundation, among others.
It is important to note that, with globalisation and diversification of markets, it is no longer necessary to consider sources of suppliers for information systems of an organisation from just only potential supplier operating with a particular trade region or a country.
Rather, consideration of global dimensions is vital while evaluating the best information systems for procurement. Important aspects for considering when seeking the suppliers to procure data warehousing and data mining systems include the system availability, quality, reliability, and system security, among others.
Trends, perspectives, recommendations, and conclusion
Many industries in all business fields operating in a competitive business world recognise the relevance of developing information-based organisations to help in decision supports.
The trend in the corporate world has been to seek mechanisms of ensuring that decisions affecting the business of an organisation are based on data that is accumulated by an organisation for a span of time in the effort to make the future forecast-based for instance on data on consumption patterns of certain customers in particular markets.
In the attempt to remain competitive through the establishment of specific market campaigns that would see potential competitors pose lesser threats of new entrants, organisations must integrate all data handled by different organisation’s departments into a single source from which all departments can tap.
The paper discussed this matter as one of noble factors that favour positive reception of data warehousing technology both in the UAE and in the international arena. The trend of data mining has been the deployment of three leading data mining techniques.
These are clustering, neural networks, and association rule and decision trees. They have been vital in alleviating the challenge of an organisation having too much data accompanied with little information.
Although an organisation can hold a tremendous amount of data in its warehouse that documents every transaction, such data is worthless if a means of its analysis is not available so that appropriate decisions based on the statistical data inferences can be made. In this perspective, the paper argued that data mining is the aspect that enables an organisation to conduct an analysis of data to reveal business trends.
Works Cited
Browning, Dave, and Joy Mundy. Data Warehouse Design Considerations, 2000. Web.
Campbell, Kevin. Exploration of Classification Techniques as a Treatment Decision Support Tool for Patients with Uterine Fibroids: Proceedings of International Workshop on Data Mining for HealthCare Management. New York: PAKDD, 2010. Print.
Davenport, Henry. “Competing on analytics.” Harvard Business Review 3.1 (2006): 213-217. Print.
Haughton, Dominique et al. “A review of software packages for data mining.” The American Statistician 57. 4(2003): 290–309. Print.
IBM. Carrefour strengthens customer loyalty and its brand with a new promotions strategy, 2013. Web.
Inmon, William. Building the Data Warehouse. New York, NY: John Wiley and Sons, 2005. Print.
O’Brien, Jonhston, and George Marakas. Management Information Systems. New York, NY: McGraw-Hill/Irwin, 2011. Print.
Zhu, Xingquan, and Ian Davidson. Knowledge Discovery and Data Mining: Challenges and Realities. New York, NY: Hershey, 2007. Print.