Abstract
Due to the increasing complexity of making optimal business decisions, organizations require tools to understand current processes and act preemptively. This work articulates tools used in this process: analysis techniques applied to innovative solutions, cause-and-effect approach to risk evaluation, and strategies of investment decision analysis. The United Arab Emirates has been effective and successful in business decision analysis. This paper discusses instances in which well-executed decisions have highly improved organizational operations. The study will largely uncover crucial aspects and decision techniques while critically examining each of them to ascertain the workability of the techniques, especially within the UAE context.
Descriptive, Predictive, and Prescriptive Analytics Techniques
Descriptive Analytics
Descriptive analytics entails the technique focused on analyzing past data, providing insights that can assist organizations in making decisions. The analysis of previously obtained data can be applied to determine what has happened, which can then determine the likelihood of future events (Fahl, 2017). Descriptive analytics can help with various population management tasks such as health data analytics (El Morr & Ali Hassan, 2019). However, the use spans beyond the healthcare realm; most of the statistics used in daily business operations fall into the descriptive analysis category (Fahl, 2017). This analysis has been largely adopted and utilized in many businesses around the United Arab Emirates to solve managerial issues.
Innovative solutions often come as an organizational response to novel challenges. In social sciences, descriptive analytics is used to analyze contemporary tasks when boundaries between the actual phenomenon and real life are not evident (Mahmood et al., 2019). This approach is used in UAE increasingly: for instance, UAE has experienced a rapid rise in technological diversity in the XXI century. Organizations face the inevitable complexity in distinguishing the present and anticipated changes, requiring a scientific approach to problem-solving. Within the framework of decision science, a descriptive insight allowed to attain the prospective solution.
The transition in technology has resulted in managerial problems within UAE. Therefore, practical decision-making was required to solve the underlying problem. Specifically, organizations had to produce methods of utilizing the changing technology to institute performance among employees. The use of descriptive analysis allowed UAE businesses to observe trends such as, for instance, average salaries in given years or individuals’ spending power (Fahl, 2017). Analyzing such data allowed to form a comprehensive evaluation and to meet the increasing performance demands of the organization.
Predictive Analytics
Predictive analytics is the summative assessment technique that mainly relies on predictive modeling. It is a method of obtaining information from already collected data and predicting behavioral patterns and trends (Fahl, 2017). Predictive modeling helps to minimize uncertainty not just in the future but also in the present and past (Fahl, 2017). Data-driven predictive analytics models detect patterns or relationships in historical data and then project the discovered relationships into the future (Greasley, 2019). Such an approach is convenient since it predicts an outcome that can enhance organizational ability to adjust their decision accordingly.
Predictive analytics in the business world mainly deals with experimental queries: for example, predicting an organization’s annual revenue or a product demand at a future time. This approach can be used for problems that involve decision science, for instance, in online marketing. Recently, UAE was faced with several marketing challenges, which called for a change in its strategy (Alomar et al., 2020). Dubai, which has been experiencing an increase in tourism, struggled to showcase its products globally (Alomar et al., 2020). The predictive analysis technique has a profound impact on the ways organizations market their products to reach global coverage.
Predictive analysis, however, is capable of solving much more complex challenges than single-sentence queries. Decision-makers can effectively apply predictive analytics by performing the process of data mining and hypothesis testing (Dinesh Kumar, 2017). With the help of relevant variables, a statistical model can then be developed. Such models have been adopted to solve performance-related challenges (Sun et al., 2021). Predictive modeling plays a major role in performance evaluation, allowing for efficient metrics consideration and enhancing the accuracy of outcomes.
Prescriptive Analytics
Prescriptive analytics is the frontier of business analytics, which identifies the optimal solution for a problem or generally the best course of action. It mainly relies on artificial intelligence to assist the decision-maker with identifying the optimal solution, given the problem context (Dinesh Kumar, 2017). This framework can produce several optimized alternatives depending on the problem complexity and within a reasonable period (Mahammed et al., 2018). Often, computer-generated solutions are highly efficient for solving complex organizational challenges.
Organizations use prescriptive analytics to solve complex optimization problems of the modern era. A recent analysis by Mahmood et al. (2019) revealed that the UAE adopted this technique to analyze the rational use of medicine and prescription drugs within several local hospitals. For instance, the study adopted a multicenter prospective cross-sectional comparative design to analyze four critical locations (Mahmood et al., 2019). The prescriptive analysis highly influenced the decision and affirmed the importance of such strategies in minimizing the consequences of poly-pharmacy.
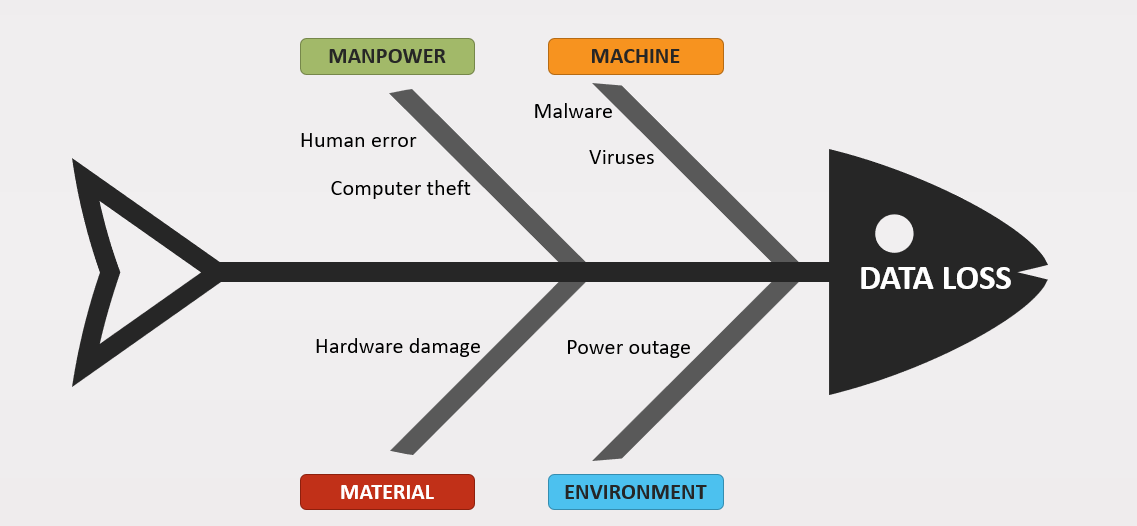
Data Management Risks’ Evaluation
A well-regulated and secure data management system is crucial for successfully maintaining a business. Breach of security is commonly attributed to human or machine aspects, and organizational flaws. Misplacement of data negatively impacts organizations’ operations since time and resources are diverted towards retrieving the lost information. Irreparable file loss often damages the operations and thus employees’ performance. Data loss occurs in several forms: it can happen through electronic erasure or physical damage to data storage. Whichever instance it is, all of them may render data unusable for the intended purposes.
While, in some cases, data loss is recoverable, there is no guarantee for recovering the entirety of data every time. Accidental file deletion has been a major data threat to some aspects of operations, especially when backing up files is not commonly practiced (Hubbard, 2020). Recoverable deletion mainly relies on artificial intelligence and backup sources; it also relies on costly and skills of the IT department experts. Both suffering the data loss and recovering the data may be substantially expensive.
The occurrence of data loss has resulted in catastrophic impacts, most notable including disruption of the organizational activities in terms of day-to-day operations. Several risks have been associated with data loss, which has proved costly for the organization to manage. For instance, there may be a 5% annual chance of a major data breach. If the breach occurs, there is a 90% chance the financial impact will be between $1 million and $20 million (Hubbard, 2020). Besides the cost factor, organization productivity suffers in data loss, particularly on the loss of production-related data.
Causes
Manpower Causes: Human Errors
Human resources cause is directly associated with people’s actions, whether it be employees, higher management, or any other human beings. Human errors are classified under two categories: intentional and unintentional errors. Intentional errors are purported actions: purposeful deletion of data from a computer system or leaking vital information to outsiders. Unintentional include accidental deletion of data or accidental sharing of files. Arguably, human error is managed by administering proper training to enhance human skills (Hubbard, 2020). Additionally, human access to machines can be controlled to manage intentional actions of individuals on data loss.
Manpower Causes: Computer Theft
Computer theft is the second human-related action that results in data loss. It entails an actual loss of machines containing crucial data of the organization. Recently, analysis indicated the transition from immobile computers to personal laptops; the resulting increase in portability raises the instances of theft (Paluzzi, 2019). Often, an employee’s loss of their laptop results in the subsequent loss of data or breach in security (Ponemon Institute LLC, 2018). Overall, loss of property such as personal computers or hard drives frequently results in the subsequent breach of data safety.
Machine Causes: Malware and Viruses
Viruses are unintended computer programs that alter the functionality of computer applications. The occurrence of viruses commonly results in increased loss of data due to the destruction of the operating system. Viruses enter systems through connections such as file downloads and email links. Such links contain malware that alters the operation and damages computer software. Backing up data while keeping installed antivirus will prevent data loss within the organization (Hubbard, 2020). However, the optimal and most applied solution for the problem is installing antivirus software that then acts as a guard against malware.
Material and Hardware Damage
Hardware is a vital component of the computer; it stores a voluminous amount of data that is crucial for its operation. Damage to hardware may result in a total loss of data. For instance, hard drives often occur as secondary storage; their fragile construction renders them susceptible to damage. 140,000 drives crash daily, resulting in a total loss of data stored on them, which indicates the vulnerability of this method (Mohammed, Ambak, Mosa, & Syamsunur, 2019). To prevent data loss that results from hard drive damage, additional secondary storage should be attached to the system while keeping backup constantly and periodically. Companies may administer personnel training to minimize damage related to handling.
Environmental Causes: Power Outage
Power is critical in every organization; an outage resulting in a sudden shutdown of servers and personal computers could result in total data loss. Businesses may install backup generators in workplaces to quickly mitigate the impact of power outages. Natural disasters may also have a sudden and drastic effect on data security through physical impact. For instance, floods, earthquakes, and tsunamis may directly result in the machine destruction and total loss of data. Businesses may create a solution to this through modern technologies such as cloud backups.
Investment Analysis
Maximax Optimistic
Under the maximax approach, the decision-maker chooses the action to result in the best payoff in the market. Following the Maximax criterion, the manager in this situation would aim for the decision alternative with the highest potential profit. In the best-case scenario, commodity investment may provide a payoff of $32,760. Based on the optimistic approach, the most desirable decision is to invest in commodities. This decision may provide the decision-maker with comparatively higher rates than either mutual funds or cryptocurrency.
Minimax Regret
The minimax regret approach is adapted to institute an investment decision with the least regrets or, in other words, with losses minimized. Based on the analysis, mutual funds have the minimax regret with the value of $6,110. Based on the minimax regret approach, the most desirable decision is to invest in mutual funds. This decision may secure the decision-maker with comparatively lesser losses than commodities or cryptocurrency.
Decision Tree
Based on the decision tree strategy, investment in commodities is considered the best alternative in the investment portfolio. Based on the decision tree approach, the most desirable decision is to invest in commodities. Subsequently, a comparative analysis of the remaining alternatives results in a preference for cryptocurrency over mutual funds. Thus, the order of preference for the decision-maker would be commodities, followed by cryptocurrency, and finally by mutual funds. Overall, all three strategies would produce different outcomes, and it would be up to the decision-maker to see which one they seem most fit.
Conclusion
In brief, the study focused on analyzing vital decision-making techniques. It did conclusive coverage on descriptive, predictive, and prescriptive analytics. The paper reiterated the importance of each analytical approach and ascertained its efficacy. An oversight into the UAE operations validated the importance of all aspects discussed. The fishbone diagram pointed out cause-effect analysis of risks associated with incomplete data, data security, and ethics. Furthermore, it identified data loss as the only problem and elucidated several causes. Finally, an analysis of the best investment was conducted, in which a different platform was attained depending on the strategy used.
References
Alomar, M., Tawfiq, A. M., Hassan, N., & Palaian, S. (2020). Post marketing surveillance of suspected adverse drug reactions through spontaneous reporting: Current status, challenges, and the future.Therapeutic Advances in Drug Safety, 11, 2042098620938595. Web.
Dinesh Kumar, U. (2017). Business analytics: The science of data-driven decision making. Wiley India.
El Morr, C., & Ali-Hassan, H. (2019). Descriptive, predictive, and prescriptive Analytics. In C. El Morr & H. Ali-Hassan (Eds.), Analytics in Healthcare: A Practical Introduction (pp. 31–55). Springer International Publishing. Web.
Fahl, J. (2017). Data analytics: A practical guide to data analytics for business, beginner to expert. CREATESPACE.
Greasley, A. (2019). Simulating business processes for descriptive, predictive, and prescriptive analytics. De G Press.
Hubbard, D. W. (2020). The failure of risk management: Why it’s broken and how to fix it (2nd ed.). Wiley.
Mahammed, N., Benslimane, S. M., Ouldkradda, A., & Fahsi, M. (2018). Evolutionary business process optimization using a multiple-criteria decision analysis method.2018 International Conference on Computer, Information and Telecommunication Systems (CITS), 1–5. Web.
Mahmood, A., Elnour, A. A., Ali, A. A. A., Hassan, N. A. G. M., Shehab, A., & Bhagavathula, A. S. (2019). Evaluation of rational use of medicines (RUM) in four government hospitals in UAE. Saudi Pharmaceutical Journal, 24(2), 189–196. Web.
Mohammed, A. A., Ambak, K., Mosa, A. M., & Syamsunur, D. (2019). A Review of the traffic accidents and related practices worldwide.The Open Transportation Journal, 13(1), 65–83. Web.
Paluzzi, M. (2019). Paying Prices for Swiped Devices: Addressing the issue of medical identity theft from unencrypted stolen laptops. University of Illinois Law Review, 2019, 1415.
Ponemon Institute LLC. (2018). Cost of data breach study: Impact of business continuity management (p. 33) [Benchmark research]. Ponemon Institute LLC.
Sun, H., Burton, H. V., & Huang, H. (2021). Machine learning applications for building structural design and performance assessment: State-of-the-art review. Journal of Building Engineering, 33, 101816. Web.