Introduction
The migration from the analog to the digital world was viewed as a major milestone in the management of data. However, cyber insecurity has emerged as the greatest threat to this evolution. Cybercriminals acting individually, as a group, or in state-sponsored syndicate agencies are keen on mining data from government agencies and large commercial corporations. The goal of such cyber-attacks varies depending on various factors. Advanced persistent threat (APT) is an example of a cyber-attack that involves a state-sponsored group that gains unauthorized access to the computer networks of a targeted entity and remains undetected for a long period (Stojanović & Bostjancic, 2020). Machine learning can help an organization to fight such cyber threats. In this paper, the researcher seeks to discuss threats posed by ATP30 to East Asian states and corporations, and how machine learning can help neutralize the threat.
Concepts of Machine Learning and Data Analytics and Applying Them in Cyber Security
It is necessary to start by defining the concept of machine learning and data analytics to help understand how the tool can help enhance cyber security within an organization. According to IBM Cloud Education (2020, para. 5), machine learning “is a branch of artificial intelligence (AI) and computer science which focuses on the use of data and algorithms to imitate the way that humans learn, gradually improving its accuracy.” The goal is to make the machine capable of making decisions, purely based on data, as a human would be expected to do in an organizational setting. On the other hand, data analytics refers to the process of collecting, processing, transforming, and organizing data to help make predictions, draw conclusions, and make informed decisions. They involve the use of data analytics to help make decisions and solve challenges that an organization faces.
The APT is one of the challenges that this tool can be used to address. Figure 1 below defines the APT threat lifecycle and why it is sometimes successful. The process started with defining the target and then finding an organizing accomplice who would facilitate the attack. The next step involves acquiring or building the relevant tools, which is followed by researching the infrastructure of the target, The team will then conduct a test to ensure that they are not detected before deploying the malware. The initial intrusion comes next before initiating an outbound connection (Choo & Dehghantanha, 2021). The team will then focus on expanding their access and obtaining credentials as they strengthen their foothold. The final stage is the exfiltration of data and covering of tracks to remain undetected but active for as long as possible.
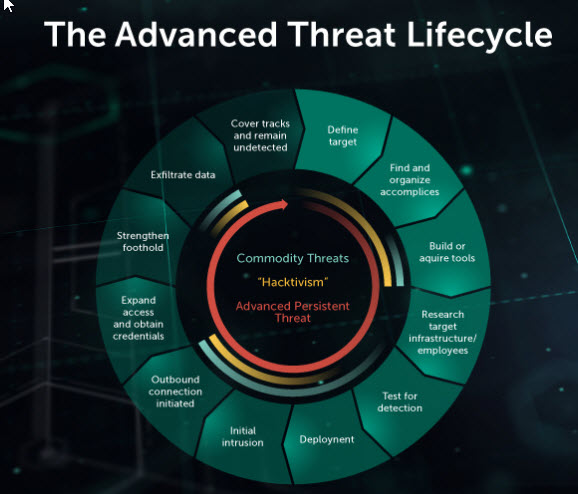
The use of machine learning and data analytics can be applied to cyber security. It will help evolve this field as artificial intelligence (AI) becomes a critical tool in fighting cyber-attacks. Arai (2022) explains that through machine learning, computers can be trained to detect some of the remotest and well-hidden malware. As computers become better through rigorous learning, they improve their skills of detecting and neutralizing attacks and intrusions.
How Machine Learning and Data Analytics Could Have Detected and Prevented the APT
APT30 has emerged as a sustained cyber-attack that majorly targets government entities and commercial institutions in Southeast Asia. Some of the countries that have confirmed these attacks include India, South Korea, Malaysia, Vietnam, Saudi Arabia, Thailand, and the United States, as shown in Figure 2 below. Countries that have likely targets of APT30 include Singapore, Japan, Cambodia, Philippines, Nepal, Bhutan, Brunei, Indonesia, Myanmar, and Laos.
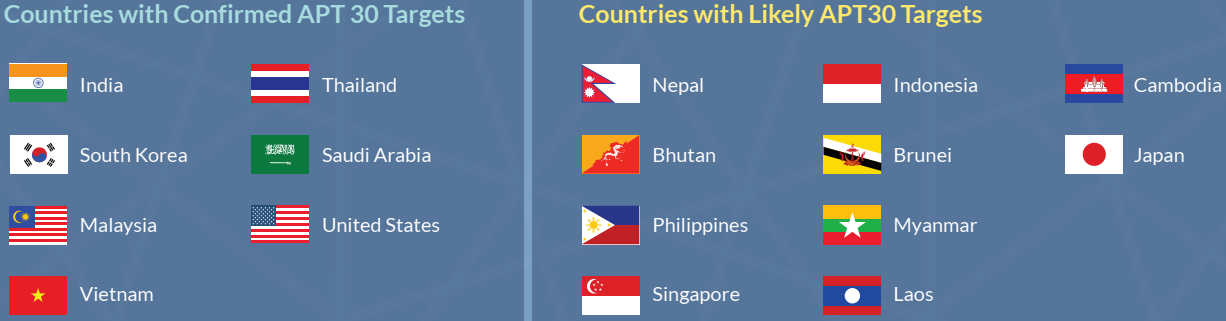
Figure 3 below shows some of the malware families that APT30 has actively used for its attacks for over 10 years. State agencies and corporate entities have suffered serious data breaches because of these attacks. Machine learning and data analytics could have helped in detecting and preventing these attacks had the victims deployed the technology at the right time.
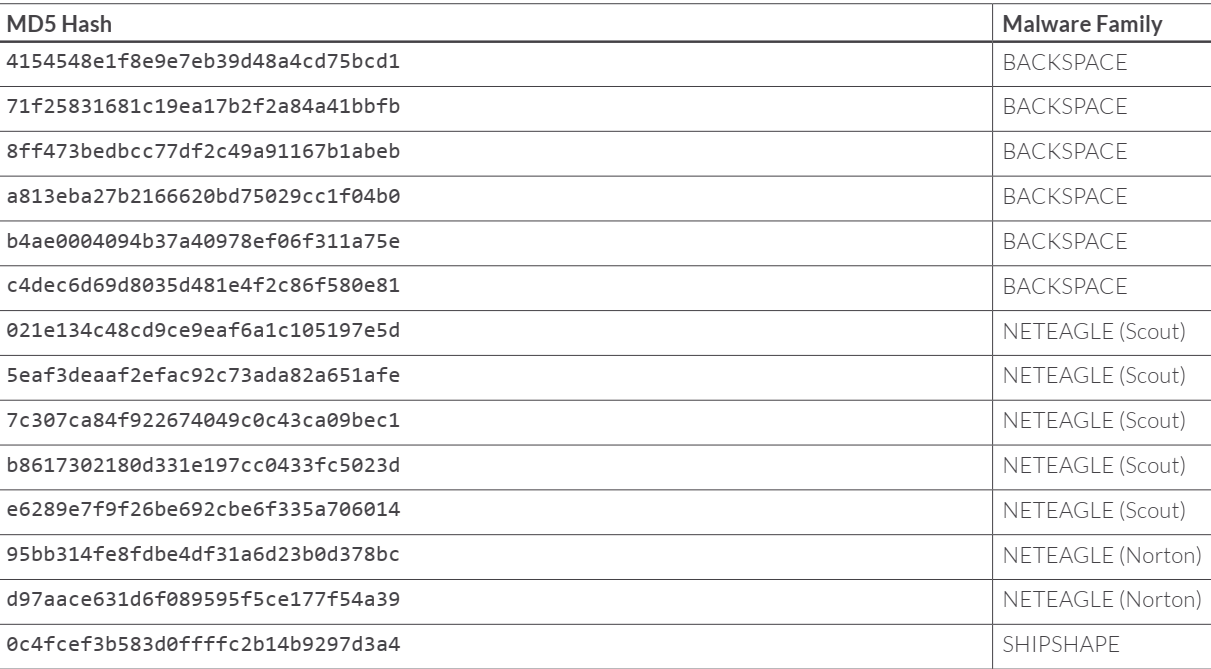
One of the most notable APT attacks was the breach of data at Community Health Systems Inc. Patil and Vohra (2021) explain that the corporation has 206 hospitals in 29 states and a database of tens of millions of patients. The breach enabled the Chinese attack to access critical information about patients. Machine learning and data analytics would have enabled this institution to detect and prevent this crime. If computer systems are well-trained, they can easily detect unauthorized access. They can also monitor how unauthorized access is gained and the activities of the alien. It can then decide to mislead the attacker, by directing it to decoy data or stop the hack. If this victim had embraced that technology, it would have prevented the attack.
Determining if the Vulnerabilities Exploited by the APT Constitute an Ethical Failure
The vulnerabilities exploited by the ATP at Community Health Systems Inc. constitute an ethical failure by the defender. This institution has a database that stores health records of millions of patients across 29 states. These patients trust this organization to safely store this information and only release it when it becomes necessary and to a specific authorized individual who will act in the interest of the patient. Cybersecurity is one of the biggest concerns for those who store digital data (Halder & Ozdemir, 2018). This company is expected to be aware of the potential threats that such sensitive data faces, especially in the hands of hostile foreign powers such as China. It was ethically necessary for this institution to put in place every measure possible to protect its database. It should have deployed machine learning and data analytic technologies to enable it to detect and neutralize any form of threat to the database.
Identifiable Harms to Privacy or Property during the Community Health System’s Attack
The APT attack on the Community Health System Inc. had a major harm on the privacy of data of patients. In its report, the company revealed that the attack involved the theft of social security numbers among other sensitive personal data that belongs to 4.5 million patients (Humer, 2014). The information breached the confidentiality, integrity, and availability (CIA) of the patients’ data. The information became accessible to unauthorized persons who were not acting in the interest of patients, which meant that the confidentiality aspect was breached. It meant that these criminals could use the information to steal from the clients of this firm or impersonate them in other criminal activities. The integrity of the company to protect the interest of its patients was put into question by this massive breach. The fact that the intruders could manipulate the data meant that accessibility was also compromised because the cybercriminals could delete patients’ data.
Transparency of Community Health System about the APT Attack
One of the most challenging yet ethical actions that a firm should take when it faces a cyber-attack such as what the Community Health System faced is to be transparent about it. Dehghantanha, Conti, and Dargahi (2018) argue that the firm has the responsibility to inform its clients of the theft of sensitive data so that they can act accordingly. In this scenario, the management of the Community Health System informed the public about the attack. They explicitly explained the type of data that was targeted. Transparency was essential because the clients realized the need to change some of their particulars to protect their finances and their identity. It was an ethical decision that demonstrated the willingness of the company to address the problem. Managing any problem within a firm starts with acknowledging the existence of the problem. It helps to inspire confidence among clients even in the face of such a major challenge.
Conclusion
Investigations have revealed that APT30 is most likely sponsored by the Chinese government, which explains why it has remained resilient and consistent in its attacks since it started its activities in 2005. The study shows that the malware poses serious threats to the political stability and economic progress of the targeted nations. Governments in these regions and individual institutions have to take deliberate measures to counter such attacks. Machine learning offers an effective solution to fighting such attacks and ensuring the confidentiality, integrity, and availability of data in these institutions.
References
Arai, K. (2022). Intelligent systems and applications: Proceedings of the 2022 intelligent systems conference. Springer.
Choo, R., & Dehghantanha, A. (2021). Handbook of big data analytics and forensics. Springer.
Dehghantanha, A., Conti, M., & Dargahi, T. (2018). Cyber threat intelligence. Springer.
Fire-Eye, Inc. (2015). APT30 and the mechanics of a long-running cyber espionage operation: Special report. Fire-Eye, Inc.
Halder, S., & Ozdemir, S. (2018). Hands-on machine learning for cyber security: Safeguard your system by making your machines intelligent using the Python ecosystem. Packt Publishing.
Humer, F. (2014). Community Health says data stolen in cyber-attack from China. Reuters. Web.
IBM Cloud Education. (2020). Machine learning. IBM Cloud Learn Hub. Web.
Kaspersky Lab. (2022). 5 Warning signs of advanced persistent threat and how to prevent advanced persistent threats. Web.
Patil, B., & Vohra, M. (Eds.). (2021). Handbook of research on engineering business and healthcare applications of data science and analytics. Engineering Science Reference.
Stojanović, D., & Bostjancic, S. (2020). Cyber security of industrial control systems in the future Internet environment. Information Science Reference.