Pearson’s Correlation Coefficient
The researcher is interested in describing a pattern of mortality from coronary heart disease (CHD) during a particular year. To perform the analysis, it was decided to use hypothetical death rates from a sample of ten states and correlate those to per capita cigarette sales in dollar amounts per month. Based on the initial observations, the highest mortality from CHD was observed in states with the most cigarettes sold, while the lowest was recorded for the least sales. Hence, the hypothesis is that smoking cigarettes contributes to fatal cases of CHD.
A Pearson’s correlation test was run to evaluate the relationship between cigarette sales and death rates based on the evidence from 10 states. There was a strong positive correlation between cigarette sales and death rates, r(8) =.826, p <.003. Further, the Ryan-Joiner (similar to Shapiro-Wilk) test was further performed to check both variables for normality. As shown in Figures 1 and 2, the correlation coefficients for both death rates and cigarette sales are very close to 1, which means that the population is very likely to be normal (Rosner, 2016). Figure 3 also shows the two-way scatterplot of the relationship between the variables that confirms previous findings because of the upward data trend. Hence, we can conclude that elevated smoking could be considered as a major reason for deaths from CHD.
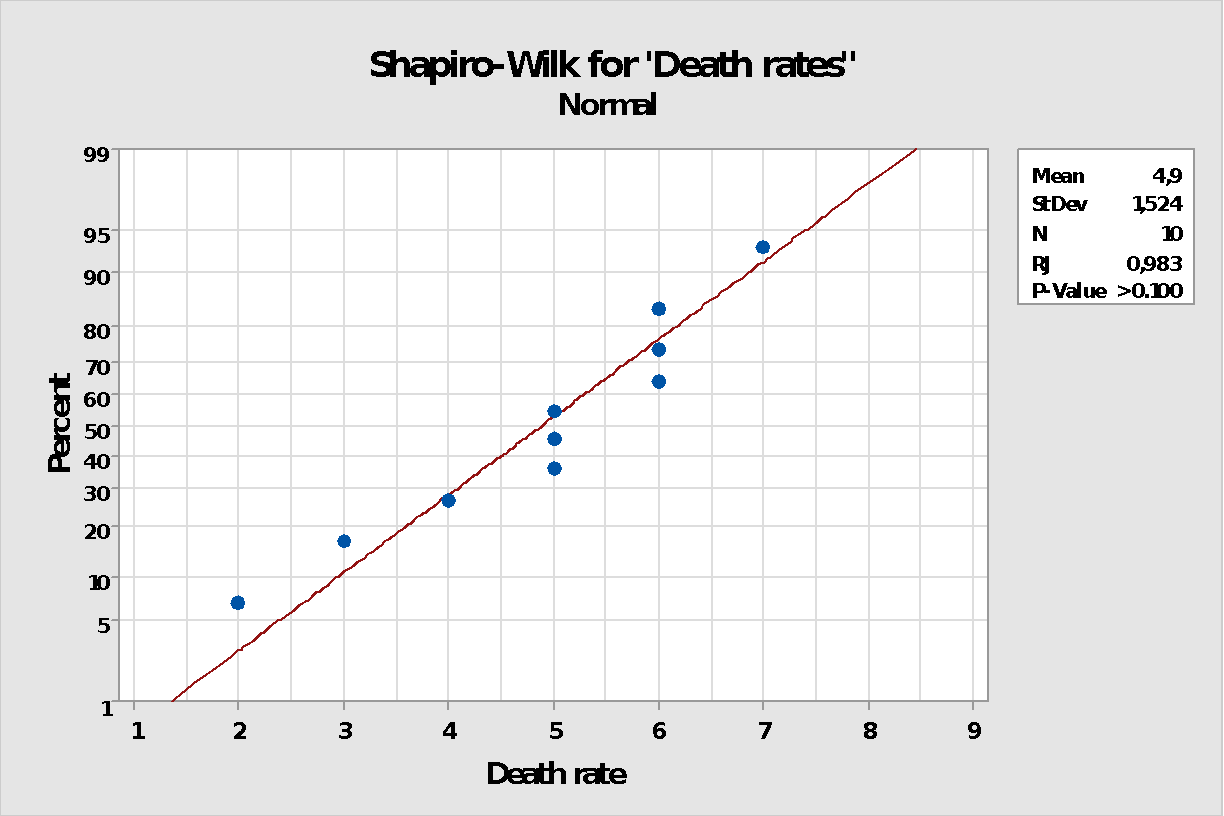
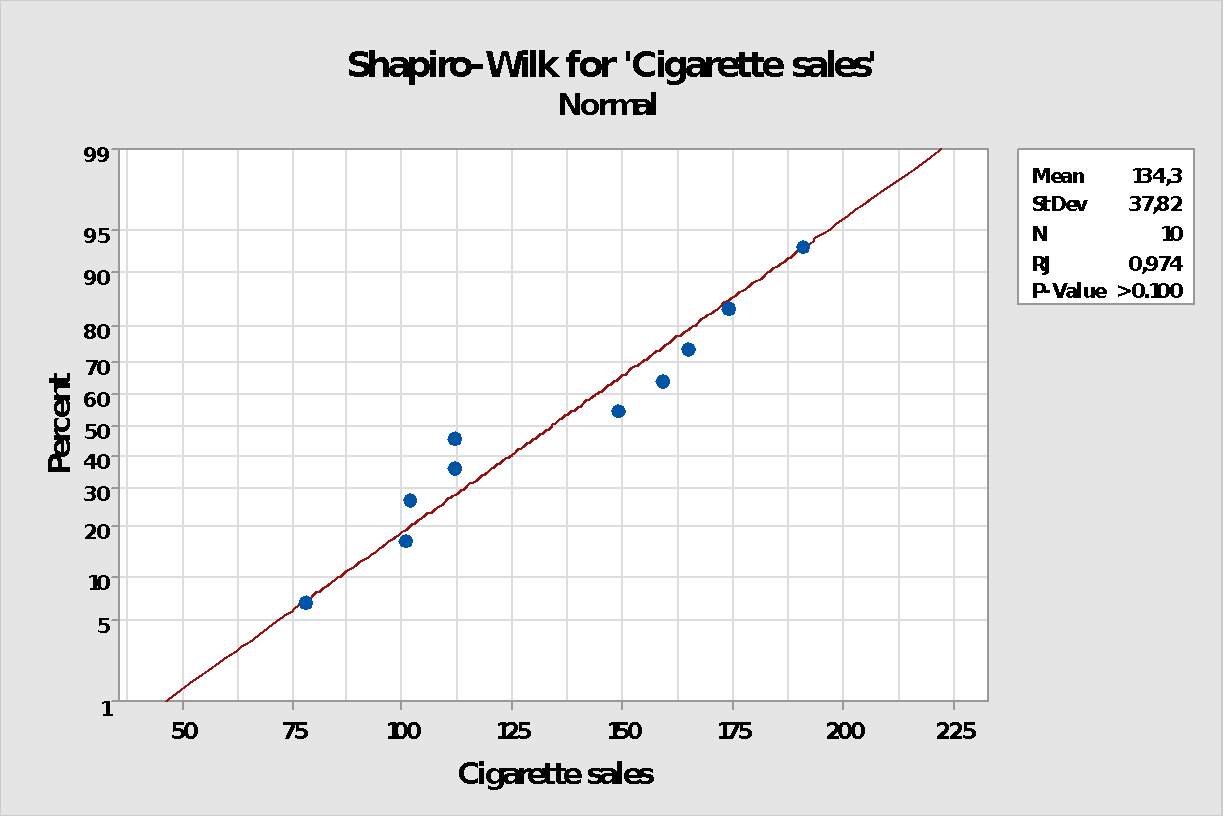
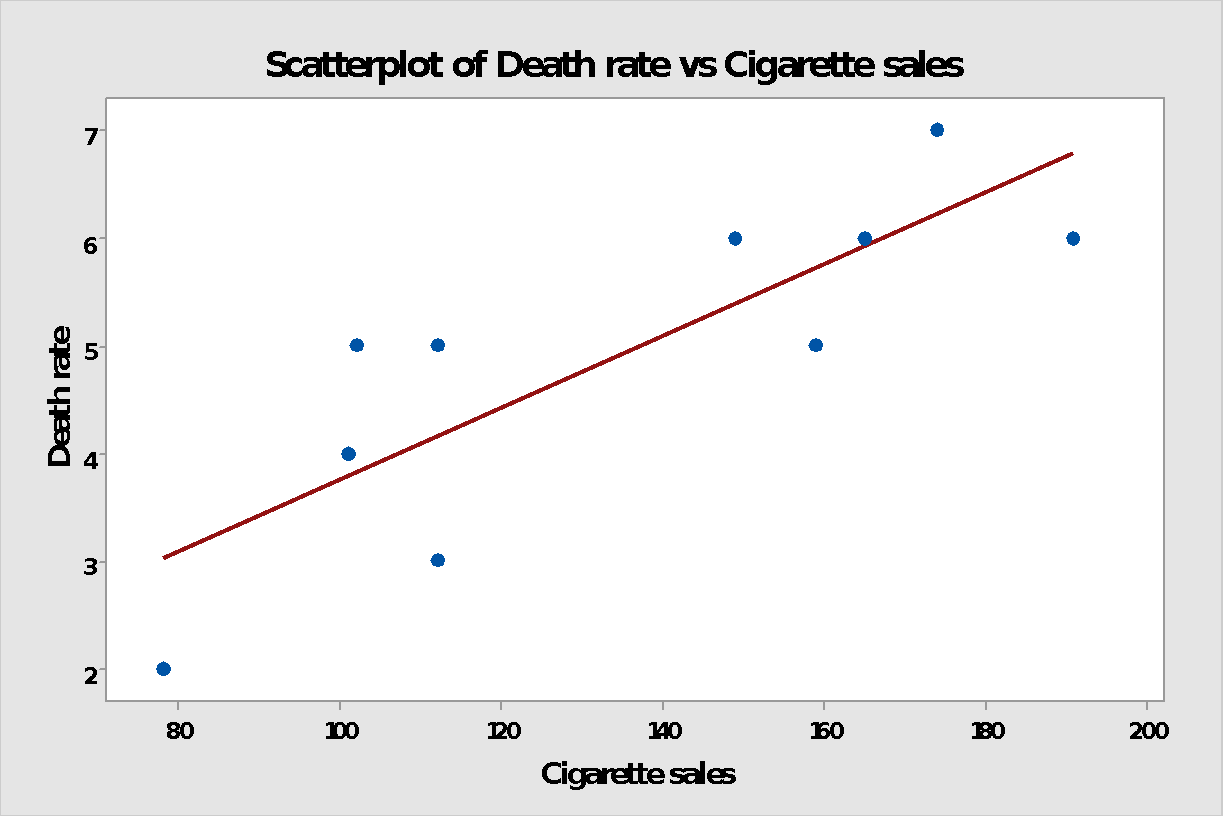
ANOVA
The researcher is interested to explore if high or low fat intake affects changes in blood pressure. The sample of 20 participants was chosen to validate if the mean blood pressure is the same between the two groups with either high (n = 9) or low (n = 11) fat intake. A one-way ANOVA was applied to identify if the level of blood pressure was different for the aforementioned groups. It was found that there is no statistically significant difference between the groups, F(1,18) = 1.68, p = 0.211. Hence, it could be concluded that the mean level of blood pressure does not differ depending on the low or high fat intake.
Analysis of Variance
Source DF Adj SS Adj MS F-Value P-Value
Fat Intake 1 149,5 149,46 1,68 0,211
Error 18 1599,7 88,87
Total 19 1749,2
As the next step, the F-test for overall comparison of means was performed to identify whether any differences are significant. Based on the analysis, it was found that there is a statistically significant difference among all means, F(1,38) = 3646.97, p <.05. Hence, we reject the null hypothesis and conclude that there is no consistency between the average values of blood pressure and fat intake.
Analysis of Variance
Source DF Adj SS Adj MS F-Value P-Value
Factor 1 168351 168351 3646,97 0,000
Error 38 1754 46
Total 39 170105
Least Squares
The researcher is interested to explore the relationship between the presence of doctors per 100,000 individuals and the number of premature births across the world based on the sample of 16 countries. Considering the non-availability of additional input variables, the least-squares analysis was applied to fit a regression line to the data. Figure 4 further shows the graphical output of the model. Based on the model output, there is a statistically significant difference between the intercept-only model and fitted model for the F-test, F(1,14) = 167.35, p <.05, and the t-test, t(1) = 26.82, p <.05. The goodness of fit test also shows that the significant proportion of variance in the number of prematurely delivered newborns is explained by the number of doctors, R2 = 0.92. Hence, it could be concluded that early births per 100,000 inhabitants in countries with fewer doctors available per 100,000 inhabitants occur more frequently.
Model Summary
Coefficients
Analysis of Variance
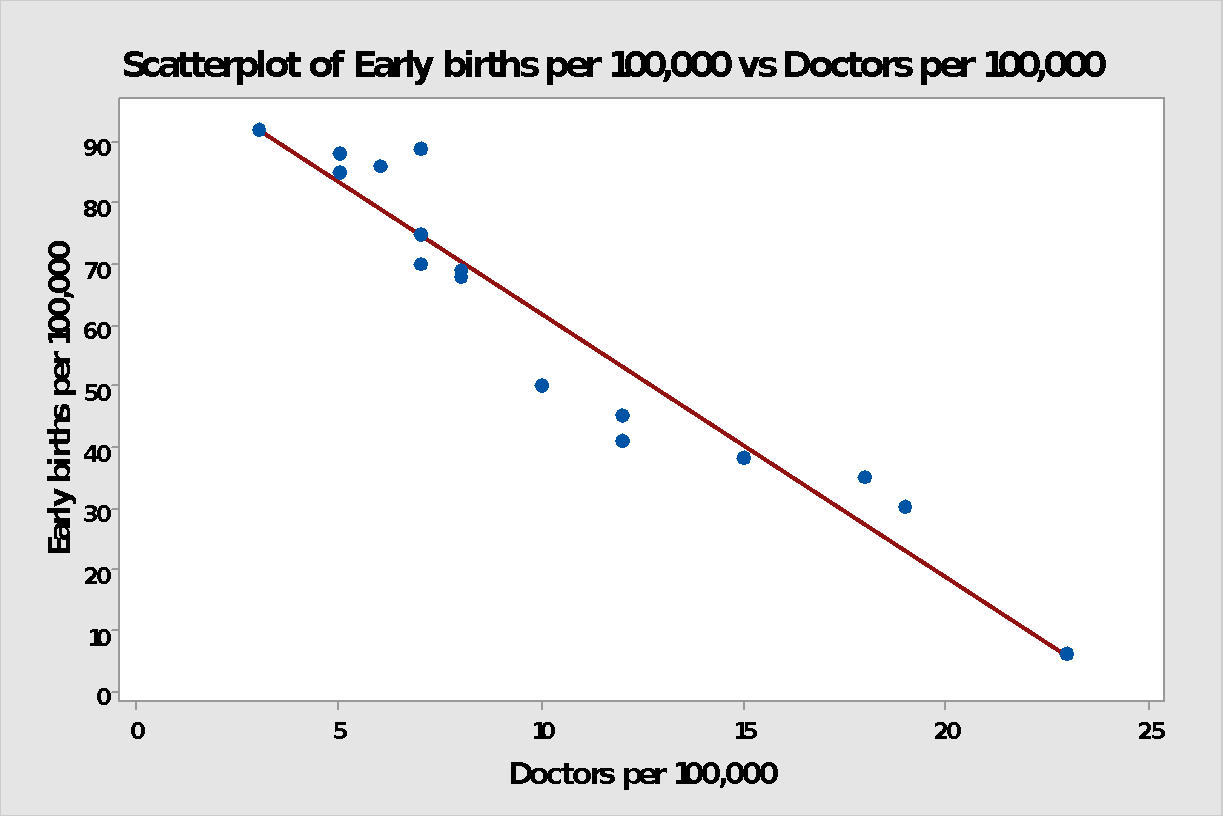
Reference
Rosner, B. (2016). Fundamentals of biostatistics (8th ed.). Cengage Learning.