Abstract
Falls in elderly patients represents a significant healthcare challenge because they have a direct impact on the quality of life. At the project site, there was no effective fall risk screening mechanism. The purpose of this quantitative, quasi-experimental quality improvement project was to determine if or to what degree the implementation of the Centers for Disease Control and Prevention (CDC) Stopping Elderly Accidents, Deaths and Injuries (STEADI) algorithm for Fall Risk Screening, Assessment, and Intervention would impact physical therapy referrals when compared to current practice among adults aged 65 or older in a primary care clinic in urban Florida over four weeks. The theoretical underpinnings supporting this project were Dorothea Orem’s self-care theory and John Kotter’s 8-step model for change. The project included N = 274 participants (n = 121 in the comparative group and n = 153 in the implementation group). Data was extracted from the site’s electronic health record (EHR). A chi-square test was conducted to analyze the comparison and implementation group data and showed a clinical and statistically significant increase in physical therapy referrals, X2 (1, N = 174) = 22.31, p =.001. Based on the results, the implementation of the CDC STEADI algorithm may improve physical therapy referrals to aid in fall prevention for this population. Therefore, the intervention is recommended to continue and extend to a larger sample over a longer time period.
Keywords: Fall risk, Orem self-care theory Kotter 8-step model, CDC, Stopping Elderly Accidents, Deaths and Injuries [STEADI], algorithm, falls, patient safety, fall screening, physical therapy
Introduction to the Project
Numerous individuals aged 65 and older are subject to falls, making it a significant issue in the health care industry (Durgun et al., 2021). Patient falls, particularly among older adults, occur in multiple settings and are associated with injuries, reduced quality of life (QOL), and significant healthcare expenses (Perrot et al., 2019). In 2019, the Centers for Disease Control and Prevention (CDC) reported that around 25% of older people experience a fall every year; however, the problem of falls could be prevented, for example, by implementing the Stopping Elderly Accidents, Deaths and Injuries (STEADI) toolkit. The STEADI toolkit incorporates an assessment algorithm developed by the CDC (2019) to be used by healthcare providers to prevent falls in older adults, particularly those aged 65 and older. Over the past years, the STEADI protocol for assessing fall prevention has been shown to be a well-established and reliable tool (Eckstrom et al., 2017). Applying the STEADI toolkit to reduce falls is a current topic for which more research has been introduced in recent years (Casey et al., 2017). As a result, the implementation of the STEADI tool within a specific setting that currently does not use it presented an opportunity for this quality improvement project.
There remains a significant need to examine the means of fall prevention, including risk screening methods (Patterson et al., 2019). Risk screening could be considered a primary element of ensuring the safety of patients (Lohman et al., 2017). Given that older patients have specific needs, especially in terms of fall risks, the need to investigate useful and effective interventions for them is especially significant (Durgun et al., 2021). There is significant recent research on falls, which shows that the subject of falls and fall prevention remains an essential and developing field (Gell & Patel, 2019).
The present quality improvement project focused on introducing a method of fall risk assessment (the STEADI algorithm) in primary care. The STEADI algorithm follows specific guidelines represented in its evidence-based structure. After introducing the subject of falls and fall prevention this project tackles, this chapter discusses the background of the problem the project investigates. This chapter then introduces the purpose of the project, followed by the clinical question guiding the project. The chapter discusses how this project advances scientific knowledge, including the project’s significance. Following a discussion of the project’s rationale for its methodology and design, this chapter presents applicable definitions of terms. The chapter then the project’s assumptions, limitations, and delimitations, concluding with a chapter summary and an overview of the remainder of the project.
Background of the Project
Patient falls have been a significant issue in the health care industry, which is especially important for older patients who are demonstrably more likely to fall than younger ones (Shahrbanian et al., 2021). According to the CDC (2019), more than one out of four people 65 and older fall each year (p. 1). Perrot et al. (2019) explained that multiple settings must deal with the issue of people falling. Applying the STEADI fall risk assessment algorithm for this project’s intervention was important to determine the impact of the STEADI algorithm on patient Referrals in a primary care setting (Lohman et al., 2017).
The STEADI Algorithm for Fall Risk Screening, Assessment, and Intervention by the CDC (2019) contains five elements. The first one is screening, for which it is specifically highlighted that screening should be done yearly or when a person falls. The STEADI protocol includes using specific questions and a tool for assessment. The next element is the interventions for persons not at risk of falls; they are meant to be educated on the matter and provided with relevant referrals and reassessments as required. The third element (which is considered the second step for at-risk individuals) discusses the assessment of the modifiable risk factors, which might include comorbidities, issues with vitamin D intake, environmental concerns, gait, strength, balance, and so on. The fourth element is intervening, which is meant to reduce the risks through specific strategies like referrals for physical therapy and others (the STEADI algorithm recommends nine specific categories of interventions and the development of a care plan and health goals). Finally, the fifth element is the follow-up element with recommended intervals of one to three months. The STEADI algorithm by the CDC (2019) is a part of many STEADI tools for older patient fall prevention.
At the project site, a small outpatient clinic in Florida, the issue of falls outside of the clinic was among the common concerns for healthcare professionals. Specifically, the problem examined was that of patients experiencing risks for falls and, as a result, experiencing falls in their lives. The patients were asked whether they had fallen in the previous year or presents with acute fall, and the data were synthesized to demonstrate the need for this direct practice improvement project. Data on falls at the project site were collected from the practitioners’ reports and interviews of the patients visiting the facility. According to currently available information, based on routine questions directed at patients aged 65 and older, any number of falls or risk for fall outside of the clinic had been reported at the rate of 21-23% for each of the years 2017-2019, lower than reported as an average by CDC (2019). However, the number of falls or risk for fall were still fairly large, especially since the fall data were based on self-reporting, and the people reporting the issue might omit some fall instances.
Falls had been identified and officially recognized as a problem since the site’s institution and the healthcare providers, particularly nurses, had dedicated time to assist with risk identification and related referrals. The need for risk assessment was determined on a per-case basis, but for older adults, it was mandatory, and intervention plans along with scheduled follow-ups were offered to check the changes in patients’ well-being. Before this project, the clinic at the project site did not use the CDC STEADI tools for supportive services to reduce fall as physical therapy which indicated a gap in practice. This project sought to fill that gap with an evidence-based best practice (EBP), and the CDC STEADI toolkit, particularly its algorithm, had been supported by evidence (Casey et al., 2017). The CDC STEADI protocol had been regularly adapted and reviewed and was available for download from the CDC website for everybody, including healthcare providers. The openness of the source made it easier to implement for this project’s practice improvement intervention.
Risk assessment has been identified as a crucial element of fall prevention for different populations, particularly older adults (Patterson et al., 2019). In this project, the CDC STEADI algorithm for screening, planning, and referrals was important to implementing EBP to prevent falls in patients aged 65 and older. The intended outcome was an increase in relevant referrals for the older population served at the project site. Offering the providers at the project site use of the CDC STEADI tool for fall risk assessment among patients aged 65 and older was intended to improve the identification of fall risk to improve the use of PT supportive referrals; with this intervention, there could be a corresponding reduction in the incidence of falls in this population.
Problem Statement
Age-related changes, multiple comorbidities, polypharmacy, impaired vision and balance, and other aspects put elderly patients at increased risk for falls and fall-related injuries (Penning et al., 2021). According to Penning et al. (2020), the use of screening algorithms could assess individual patients’ fall risks and help develop individual strategies to decrease those fall risks. The choice of the screening algorithm and program depends on the needs and potential of a particular site.
It was not known if or to what degree the implementation of the CDC STEADI ALGORITHM FOR FALL RISK SCREENING, ASSESSMENT, AND INTERVENTION would impact physical therapy referrals compared to current practice among adults aged 65 and older. This project attempted to identify whether the CDC STEADI algorithm could affect the physical therapy referrals of patients at the project site. If the answer is yes, the project would assess the effectiveness of this intervention compared to the consequences of implementing usual standards of care at the project site, contributing to the literature on the topic (Johnston et al., 2018). The results would be conclusive, and they would offer additional insights on the topic while introducing an evidence-based intervention into a healthcare setting (Eckstrom et al., 2017).
The identified problem has affected a specific population significantly. This project addressed older patients at an outpatient clinic since they appeared in unusual conditions that subjected them to potential threats; specifically, it involved patients appearing outside of a tightly controlled environment (the clinic) while still running rather high risks of falling as a result of numerous causes (Dhalwani et al., 2017). Therefore, the cases under analysis required greater insight.
Furthermore, the focus was placed on the general population of people aged 65 years and older. This was because many of these individuals’ health conditions had deteriorated because of natural processes, which subjected them to more falls (Dhalwani et al., 2017). Every fall could have more dangerous consequences for older people than for younger ones (Durgun et al., 2021). For this reason, strategies for managing falls in the elderly must be designed.
The given project has addressed the role of conducting physical therapy referrals among older adults and offer project results that contributed to solving the problem of falls among this population. By analyzing the STEADI algorithm’s impact on physical therapy referrals at the project site, this project could confirm whether the intervention was effective in protecting older patients from falls, which would suggest that evidence-based fall risk assessment is important for patient health (Mark &B Loomis, 2017). Overall, the project’s goal was to contribute to the wealth of data on a particular EBP while implementing it at a site that recognized the importance of the issue of physical therapy referrals, particularly among older adults. The main positive outcome of this project being successful would consist of the site providers having a new EBP and tool for on physical therapy referrals (Lohman et al., 2017).
Purpose of the Project
Proper risk assessment requires choosing the most appropriate prevention strategies and tools. Screening patients’ health parameters and living conditions should include estimating the potential risks of falls, particularly for older adults. The implementation of the CDC STEADI algorithm in the chosen setting depended on a variety of co-factors and was intended to determine the impact of the tool on physical therapy referrals. The purpose of this quantitative, quasi-experimental quality improvement project was to determine if or to what degree the implementation of the CDC STEADI Algorithm for Fall Risk Screening, Assessment, and Intervention would impact physical therapy referrals when compared to current practice among adults aged 65 or older in a primary care clinic in urban Florida over four weeks. The CDC STEADI algorithm was the independent variable, defined as the STEADI Algorithm for Fall Risk Screening, Assessment, and Intervention (Mark & Loomis, 2017). The dependent variable was physical therapy referrals compared between the comparative and implementation groups. Referral was defined as the instances of referring patients to physical therapy as a result of fall risk assessment. The relationship between the variables was determined based on a quantitative methodology and a quasi-experimental design. The population was specifically outpatients aged 65 and older attending a primary care clinic in urban Florida.
The project’s purpose indicates that the project sought to improve services provided to older adult patients in one outpatient primary care clinic in urban Florida. By examining the impact of the CDC STEADI Algorithm for Fall Risk Screening, Assessment, and Intervention on physical therapy referrals at the project site, the project would show whether it was possible to affect the referrals to relevant services for patients who were defined to be at risk of falls (Lohman et al., 2017). The findings would also demonstrate whether other clinics and health care settings should draw their attention to fall assessment and EBP and related tools to address the problem under analysis. The findings of this project would have immediate value for the patients while also contributing data to the field of study in fall risk assessment, providing the healthcare workers at the site with new evidence-based tools and signaling to other healthcare workers the opportunities possible with applying the EBP of using the CDC STEADI algorithm for fall risk assessment.
Clinical Question
This project included the analysis of measurable variables to evaluate the potential benefits of implementing the CDC STEADI algorithm for physical therapy referrals among patients aged 65 and older at the project site. The project established the relationship between the independent variable, the CDC STEADI algorithm, and the dependent variable, identification of physical therapy referrals. It was estimated that the relationship would be significant, statistically, clinically, or both, and the project was implemented to answer the following clinical question:
To what degree did the implementation of the CDC STEADI ALGORITHM FOR FALL RISK SCREENING, ASSESSMENT, AND INTERVENTION impact physical therapy referrals when compared to current practice among adults aged 65 and older in a primary care clinic in urban Florida?
The CDC STEADI Algorithm for Fall Risk Screening, Assessment, and Intervention was implemented as EBP for this intervention. Before this project, the standard fall prevention at the project site did not use the STEADI tool to assess the risks of falling and educate patients or caregivers (Johnston et al., 2018). The population included patients aged 65 and older in the outpatient clinic at the project site. The selected timeframe of four weeks was the most feasible for this project.
Like the problem statement, the clinical question implies different variables. The STEADI Algorithm for Fall Risk Screening, Assessment, and Intervention was the independent variable. Physical therapy referrals represented the dependent variable, which denoted that it was necessary to measure it. The Electronic Health Record (EHR) data were extrapolated and used to quantify the referrals, which also used ratio data. The predictive statement was that there might be a statistically significant difference between the instances of physical therapy referrals before and after the intervention for fall prevention.
Advancing Scientific Knowledge
This project resulted in improvements concerning population health outcomes at the project site. The project findings demonstrated whether it was adequate to rely on the CDC STEADI algorithm for improving physical therapy referrals. Anticipating positive results from implementing the EBP of the CDC STEADI algorithm, the advancement in scientific knowledge would be a small step forward in a line of current quality improvement projects, demonstrating if the CDC STEADI algorithm could be used effectively in practice to improve fall risk assessment and related referrals (Eckstrom et al., 2017; Lee, 2017). Additionally, the project would contribute to addressing an important research topic, that is, fall risk screening and assessment in patients, especially older patients, a rapidly developing field (Patterson et al., 2019; Phelan et al., 2017; Snooks et al., 2017; Vincenzo et al., 2020). Overall, the project contributed to the improvement of practice in the project site’s clinic and advanced knowledge in an important field related to fall risk assessment.
This project focused on Orem’s (1985) self-care deficit theory (SCDT) as a theoretical underpinning supporting the project. Younas (2017) stipulates that worsened health outcomes are often the result of self-care deficit. This concept implies that a person lacks sufficient ability, knowledge, or desire to care for themselves. From the perspective of nursing, Orem’s (1985) SCDT implies that healthcare providers are capable of affecting self-care deficits through diverse interventions. It could not be denied that fall risk assessment remains one such intervention; fall risk assessment provides the patients with information about their health. Patients are empowered with health knowledge, which assists them in reducing self-care deficits (Patterson et al., 2019). Furthermore, the CDC (2019) STEADI algorithm explicitly aims at reducing self-care deficits. The CDC STEADI identifies specific fall risk issues at hand and offers potential solutions to them through referrals and other changes. Therefore, Orem’s (1985) SCDT could be used to frame the key elements of this project, providing a justification for the selected intervention. This project demonstrated the theory’s use in practice and its viability for empowering patients and preventing falls.
Equally important as a theoretical underpinning for this project was Kotter’s 8-Step Change Model, based on steps to reduce injuries associated with falls in older people. Following Kotter’s model involves developing the most effective actions for treating and caring for older people, suggesting that the eight steps should be followed and used as a template for improving health care. Therefore, Kotter’s (1995) model looks at both the steps of care and the collection of analytical data and how it could be interpreted to prevent a recurrence of the problem in the future. Each step is unique and explains a different reason for a person’s behavior when treating an older person after a fall. Looking at Kotter’s method holistically, it was seen as a critical aspect supporting this project’s practice change.
Significance of the Project
A quality improvement project gap at the project site indicated the need for this project’s intervention to improve fall risk assessment and Physical therapy referrals. There are empirical studies that support the effectiveness of the CDC STEADI Algorithm (Eckstrom et al., 2017; Johnston et al., 2018). The literature supports that the CDC STEADI Algorithm is an EBP that has been studied extensively (Lohman et al., 2017; Mark & Loomis, 2017). The CDC STEADI Algorithm represents a topical area that could benefit from additional exploration, especially in the form of a quality improvement project implementing this EBP as an intervention at a site that currently does not use the STEADI Algorithm tool for risk assessment. In turn, risk assessment is a common approach to fall prevention and is often considered a requirement for healthcare programs that aim to prevent falls, another topical area extensively studied (Gell & Patel, 2019). The given project would contribute to the current literature on the topics and add to the literature on the CDC STEADI Algorithm program and its impact on fall risk assessments on related referrals, particularly in primary care.
This project also was significant because it generated theoretical implications related to the connection between patient falls and Orem’s (1985) SCDT. In particular, the project investigated the elimination of self-care deficit through patient referrals based on fall risk assessment conducted with the evidence-based CDC STEADI algorithm. This project demonstrated the ability of Orem’s SCDT to be applied to an investigation like this one into fall prevention.
Finally, it was reasonable to address the project’s practical implications. The results were helpful for practitioners as it enhances their understanding whether using the CDC STEADI algorithm for fall prevention was useful. The project’s results could influence how health care delivery was conducted within the stated setting of primary care, particularly related to fall assessment and prevention. Attention to the need for risk assessment, referrals, and follow-ups was brought about by the results of this project. In the long run, the project might contribute to the improvement of the whole medical industry since the project tried to improve older patients’ health outcomes by preventing falls. By this project demonstrating the ability of the CDC STEADI algorithm to facilitate increased physical therapy referrals, the relevant literature on the topic was supported and expanded (Johnston et al., 2018). As a result, the project highlighted and promoted the use of the CDC STEADI algorithm in outpatient settings. Thus, the project’s main objective was the application of the CDC STEADI algorithm within the specific setting of primary care to demonstrate how the STEADI algorithm could facilitate referrals to improve outcomes in patients aged 65 and older.
Rationale for Methodology
The quality improvement project sought to identify how the specific intervention influenced the referrals to various services meant to assist older patients experiencing a risk of falls. The research methodology entails work with figures and make appropriate calculations to identify whether the proposed solution was significant, which required a quantitative methodology. Creswell and Creswell (2018) explained that the quantitative methodology type is necessary when there is a need to test for a relationship between different variables. Furthermore, Rutberg and Bouikidis (2018) stipulated that the reasons to choose this methodology address in case of lack of positive improvements in the project or certain quality aspects remain unaddressed. As for the given project, the quality improvement project gap at the project site and the unanswered clinical questions denoted that the quantitative methodology was correctly chosen.
A quantitative methodology was the best option for this project because other variants, including qualitative and mixed methods, were not suitable. A qualitative methodology is used when it is necessary to explore a poorly understood problem (Rutberg & Bouikidis, 2018). Qualitative methods and projects tend to organize semi-structured interviews to allow participants to disclose their feelings and attitudes toward the problem under analysis. Qualitative investigation would not be able to respond to the stated clinical questions of this project, which sought to establish relationships between variables, although it could be helpful in other circumstances (Polit & Beck, 2017). A mixed methodology combines the previous two features of qualitative and quantitative methods. The mixed methodology is used in cases when scientists want to calculate statistical indicators and identify participants’ feelings (Rutberg & Bouikidis, 2018). Finally, Polit and Beck (2017) admit that quantitative methods are more feasible since they require less time to answer clinical questions than qualitative and mixed approaches. In conclusion, the given project’s quantitative methodology was chosen to best address the problem statement, clinical questions, and purpose.
Nature of the Project Design
This project followed a quasi-experimental design to address the purpose and answer the clinical question. The quasi-experimental design included the intervention (CDC STEADI algorithm), and two groups, the comparative and implementation groups. The comparative group and implementation group were observed to determine the impact of the independent variable, the CDC STEADI Algorithm for Fall Risk Screening, Assessment, and Intervention, on the dependent variable, physical therapy referrals, when compared between the two groups undergoing fall risk identification. Such projects are suitable to identify a relationship between an intervention and its outcomes (Polit & Beck, 2017). Furthermore, the quasi-experimental design was appropriate to assess the intervention’s impact while also being relatively easy and quick to implement, compared to, for example, a true experiment (Polit & Beck, 2017). Thus, a quasi-experimental design was the best design for the given project as compared to other options; the quasi-experimental design was more feasible than an experiment and implied fewer ethical concerns, but also was sufficiently capable of responding to the clinical questions.
The given project did not have an extended sample because it was implemented in one Florida outpatient clinic that was not large. That is why a sample size included 150 patients based on a quasi-experimental design analysis. The inclusion criteria were age 65 and older and assessment for fall risks using the CDC STEADI tool. The project investigator did not contact the patients, and data were accessed through the facility EHR, so no signed consent was required.
The project used two groups, a comparative and implementation group, and all the practitioners at the clinic were provided with the CDC STEADI algorithm (Polit & Beck, 2017). The referral data were extracted from the facility’s EHR. The baseline data for the comparative group consisted of previously reported fall risk assessments and
PT referrals for patients from the comparative group over four weeks before the project. Implementation group patients were assessed during the project with the CDC STEADI algorithm and data examined post-intervention from the EHR. Polit and Beck (2017) explained that pre-intervention and post-intervention data are sufficient to assess the effectiveness of an intervention. Consequently, the report included referrals to identify whether the STEADI algorithm was useful for increasing physical therapy referrals when comparing the comparative and implementation group data.
Definition of Terms
Providing the definition of term enhances the clarity of the language used in the project. This section below explains terms, variables, and other specific terms that might be unknown to a layperson. Terms are defined in lay terms and in the context in which they are used. The following terms were used in the project.
Falls
Falls are completed events that occur when patients have collapsed (Hill et al., 2015). These events imply adverse health consequences but can be preventable. Various factors, including health conditions, external factors, the lack of education, and others, can make patients collapse.
Referrals
The CDC (2019) program recommends introducing referrals to varied services meant to promote the healthcare of individuals based on their needs identified through STEADI, which is the definition of referrals within this project. They are the dependent variable to be considered.
The Concept of Self-Care
The concept of self-care refers to a person’s ability and desire to take care of themselves (Younas, 2017). Self-care deficit could result in individuals neglecting their health condition and well-being. The current project considers Orem’s self-care theory and its relation to the occurrence of falls, specifically from the perspective of healthcare providers who can reduce self-care deficits, among other things, through risk assessments.
Stopping Elderly Accidents, Deaths and Injuries (STEADI)
The CDC (2019) STEADI protocol is intended to prevent falls in older patients and offers providers important fall prevention tools. For this project, the STEADI Algorithm for Fall Risk Screening, Assessment, and Intervention was the independent variable. The CDC STEADI tool guides providers on how to assess the risks in older patients and intervene through care plans and relevant referrals, and the implementation of the program has been studied comprehensively.
Assumptions, Limitations, Delimitations
This quality improvement project included several assumptions. The participants’ interest in the project was an underlying assumption. This fact denoted that the staff would be willing to undergo the intervention (that is, learn to use the CDC STEADI algorithm). Furthermore, it was assumed that providers would use the CDC STEADI algorithm correctly, using all of its stages in their practice and reporting the data. Research indicated that the rates of adhering to interventions vary, but they tend to be rather high, specifically for STEADI, which is estimated to be at around 75% (Vincenzo & Patton, 2019). It was believed that the CDC STEADI algorithm would be applied to all patients meeting the criteria.
The project had several limitations. A small sample size of 150 patients was objectively a weakness, but it should be sufficient for the specified methodology based on a quasi-experimental design analysis. In addition, the fact that the participants would only represent one clinic was important; it was a limitation because patterns needed to be studied across different environments (Polit & Beck, 2017). The providers’ workload might impact the visit time and thus might affect the time spent using the CDC STEADI algorithm, impacting its effectiveness. The short timeframe of four weeks admittedly was a limitation (Polit & Beck, 2017).
Finally, a delimitation also must receive specific attention for the given project. This project focused on older patients because they are more subject to falls than younger individuals. Individuals younger than 65 years but at increased risks of falls due to certain circumstances, such as working in risky environments, or due to certain conditions not related to aging changes, including substance abuse, were not included in the project sample.
Summary and Organization of the Remainder of the Project
Patient falls at healthcare facilities and outside of them remain a significant issue in the healthcare industry (Eckstrom et al., 2017). According to the CDC (2019), numerous individuals older than 65 are subject to the problem of falls, making it necessary to find an effective intervention. Risk assessment is a crucial element of fall prevention, and some scientific articles have assessed the impact of the CDCSTEADI algorithm (Eckstrom et al., 2017). This fact determines the significance and purpose of the project, which focuses on falls outside of the clinics.
This quality improvement project was necessary to address a gap in practice at the project site, which did not include EBP for fall risk assessment before this project. This project aimed to study the impact of implementing the CDC STEADI tool to improve physical therapy referrals. A quantitative method with a quasi-experimental design as used for this project to examine participants (N = 274 participants, n = 121 in the comparative group and n = 153 in the implementation group). The project compared physical therapy referrals between the baseline and implementations group, a between-group design that was suitable for the current project (Rutberg & Bouikidis, 2018). Orem’s (1985) SCDT provided the theoretical underpinning guiding the project. Over four weeks, the project’s intervention could lead to significant theoretical and practical advancements in the health care industry by investigating the effects of an evidence-based intervention. The small sample was a limitation, as well as the four-week timeframe.
Chapter 1 provided an overview of the project. Chapter 2 presents the extensive literature review conducted to support the project, including its theoretical underpinnings. Chapter 3 focuses on the project’s methodology, including its purpose, clinical question, and applicable methodology and design. Chapter 4 presents the results of data analysis, which is necessary to understand how the project reached and interpreted its results and contributed to the nursing field. Chapter 5 offers a project summary and conclusion along with recommendations based on the project’s findings.
Literature Review
This quality improvement project focused on fall prevention in older populations that included educating providers and caregivers to facilitate the implementation of the CDC STEADI Algorithm for Fall Risk Screening, Assessment, and Intervention (CDC, 2017; CDC, 2019). This literature review synthesizes the results of a systematic review of the literature on the topic of falls, fall education, and some findings of non-systematic reviews of the literature on falls in general. The purpose of this quantitative, quasi-experimental quality improvement project was to determine if or to what degree the implementation of the CDC STEADI Algorithm for Fall Risk Screening, Assessment, and Intervention would impact physical therapy referrals when compared to current practice among adults aged 65 or older in a primary care clinic in urban Florida over four weeks.
This project confirmed with its literature review that the problem of falls in or outside of healthcare settings remains a serious issue, especially among patients aged 65 years and older (Durgun et al., 2021). Falls could cause injury, resulting in additional healthcare expenses and reduced QOL, and the issue is associated with multiple factors that should direct efforts aimed at preventing falls (Dhalwani et al., 2017). Research has shown that educational solutions meant for patients, caregivers, and professional caregivers are a viable option for helping to prevent falls (Chang et al., 2019). Specifically, interventions for patients are rarely researched, and those for informal caregivers are seldom studied, although the need for them has been established in the literature, as patients and caregivers have requested additional information on falls and fall risk identification and prevention (Schoberer et al., 2016). Therefore, the topic of caregiver education in fall prevention was a clear research gap this project addressed since few recent sources mentioned it (Schoberer et al., 2016). This project contributed to the literature and to practice by introducing the CDC STEADI intervention at the project site to examine the impact on physical therapy referrals to improve patient and caregiver education and patient outcomes.
This project’s literature review identifies three themes and subthemes; the first one is based on systematically reviewing all the literature on the topic of education-based fall prevention published within the past five years in peer-reviewed journals found in large healthcare databases, including MEDLINE, CINAHL, and PubMed. The decision to limit the literature to recent articles was made to ensure the most pertinent information was included about up-to-date approaches to fall risk identification and prevention. The named databases are among the sources most often employed in healthcare research (Polit & Beck, 2017). The second section involves finding predominantly recent articles on the topic of falls, including fall prevalence, fall prevention, fall education, and provider and caregiver involvement in fall prevention. Additionally, some seminal works were incorporated related to the theoretical frameworks supporting this project, but in most cases, the project looked into recent literature only. Inclusion criteria were English language, availability, and published date within five years of this project. The keywords included fall prevention, education, and older patients. Articles could be excluded for not being relevant to the project.
This chapter is structured to present the project’s literature review, guided by related themes and subthemes supported by scholarly sources. This literature review demonstrates that significantly more research is required on the topic of fall risk identification and prevention, especially since recent sources on the topic are rather scarce, and certain subtopics, like the education of caregivers, are rarely considered. This chapter highlights the presented literature in support of the project’s topic, methodology, and design, including its theoretical underpinnings. After synthesizing applicable literature related to the theoretical foundations supporting this project, this chapter presents a thorough literature review guided by the mentioned themes and subthemes, concluding with a chapter summary and an overview of Chapter 3.
Theoretical Foundations
As people age, their bodies become frailer, and they gradually lose stability. This process leads to a high number of fall-related injuries and deaths among adults 65 years and older, costing over $30 bill. annually for U.S. citizens alone (Stevens, 2013). The two articles by Stevens and Phelan propose the Stop Elderly Accidents, Deaths, and Injuries (STEADI) tool kit, which a list of resources essential for healthcare providers to consider when dealing with older patients.
The impact of falls on the well-being of older adults cannot be underestimated for the sake of public health. Not only does this problem strain the healthcare system, but it also causes the loss of life among older people, with over 20,000 deaths occurring in 2012 (Stevens & Phelan, 2013). There is a growing percentage of older adults in the United States who suffer from at least one fall in a year, indicating that the existing measures were insufficient (Stevens & Phelan, 2013). The change in direction is necessary, and the tool kit provides a risk assessment to understand the exact impact of the issue on a patient’s wellbeing.
Articles regarding falls often discuss possible ways to reduce the occurrence of this type of incident. Steven’s works provide a solid basis for action, yet more recent studies reveal additional evidence for alleviating the problem. For example, Carlucci et al. (2018) propose a program that promotes joyous physical exercises to prevent older adults from associating movement with pain, injury, or embarrassment (p. 2). Patients’ knowledge also plays a vital part in reducing their chances of falls. A short education course can decrease the chances of suffering from fall-related trauma by over thirteen percent (Jie & Deng, 2019). Promoting such practices is one of the key goals of STEADI, which can be further modified in accordance with the latest evidence for maximum efficiency.
The tool kit also highlights that it is vital to provide necessary assistance to people at risk of fall-related traumas. This goal can be achieved by referring high-risk individuals to physical therapy sessions designed to upkeep sufficient body movement capabilities before balance and coordination issues cause any traumas (Carlucci et al., 2018; Stevens, 2013). Referrals to local community-based therapies satisfy the need for physical activities among older adults, but physicians must be knowledgeable about such opportunities.
The STEADI tool kit is a comprehensive solution to a major healthcare crisis and needs to be incorporated into all facilities that deal with older adults. Stevens, alongside numerous experts, has built a system that considers all recognized factors affecting the change of fall traumas, as well as eliminates barriers to efficient fall prevention. The tool kit is easily modifiable and can be updated with numerous new evidence-based practices that are proven to be efficient in their proposed task.
Orem’s (1985) self-care theory is used to support this project. The concepts of self-care and self-care deficits have been explained and help justify this project’s intervention (Younas, 2017). Since this project is focused on fall risk identification and prevention, including educating patients and caretakers as well as Orem’s (1985) theory helped to frame the clinical question and assist in understanding the nursing practice needed to support the project’s intervention.
Orem’s (1985) general idea is that nursing is the act of assisting someone with self-care, which, in turn, is the ability to take care of oneself, meaning the ability to maintain one’s well-being. A nurse needs to have the knowledge and training required to understand and assist other people with their self-care. Humans are the objects of nursing, as a result, and the subjects carrying out the care are nurses. The environment is also taken into account, including physical and social aspects (Orem, 1985). Orem’s (1985) view of health as soundness is slightly outdated, but it takes into consideration both the health of individuals and groups, which is helpful for the present project. A self-care deficit implies that a person cannot ensure effective care of themselves for any reason, which often includes a deficit of resources, but might also incorporate a variety of factors, including demographics (for example, age), cultural and social factors, and environmental factors (for example, access to healthcare) (Orem, 1985). One of the primary reasons that justify the present project is the lack of knowledge of evidence-based fall identification and prevention at the project site. The same could be said about the lack of knowledge in caring for another person. Improved knowledge should reduce a self-care deficit in the participants, providing them with improved means for self-care, which, in turn, should lessen the negative outcomes of self-care deficits (falls). Thus, the theory supports this project’s intervention and related variables and the reason for investigating the possible relationship between them.
Self-care is a complex concept that incorporates universal requisites (something that is required for a person to be able to perform self-care, including the intake of air, water, food, excretion, rest, interaction, development, and management of hazards), as well as the requisites that become important when a person is in the state of health deviation (Orem, 1985). Teaching another person how to self-care and ensure the fulfillment of all these requisites is one of the methods of helping a person to self-care (Orem, 1985).
It is important that for Orem’s (1985) theory, the interactions between humans are critical. According to the theory, humans form connections for the sake of their well-being and exchange the means of self-care and organize the care for the people who cannot take care of themselves. This perception of self-care and human attempts to provide care to those who cannot perform it is also helpful in understanding the variables of the project, in particular, the idea of exchanging fall prevention knowledge, like through physical therapy, for improved self-care.
Finally, a change theory was needed to advance this project’s goal at the project site to encourage a positive shift in managing falls among the elderly, specifically, implementing the STEADI algorithm to facilitate related physical therapy referrals. To ensure that the proposed change was integrated promptly and seamlessly into the specified clinical context, Kotter’s 8-Step Change Model was needed. Applying Kotter’s 8-Step Change Model helped the project successfully integrate change into the clinical context to sustain the practice change and enforce it as a form of continuous improvement.
Kotter’s 8-Step model should be seen as a template for incremental change in the healthcare setting. According to Shammas (2017), Kotter’s model suggests that change should be advanced in a manner that allows for creating a cycle of continuous improvement. The authors present a Specifically, the first stage of Kotter’s framework involves establishing the sense of urgency required for the change. In the context of the project site, the assessment of the current healthcare setting, with the focus on the self-care deficits observed within it, helped accomplish the specified goal. Afterward, a team of experts had to be built to promote practice change. Specifically, a multidisciplinary group of experts deployed the STEADI algorithm at the project site to promote more effective management of falls by improving fall risk assessment and making physical therapy referrals assisted by the evidence-based STEADI tool (Shammas, 2017). The next step was building a vision of an environment in which collaboration and the adoption of an advanced data management system allowed reducing the threat of falls and improving the quality and efficacy of patient referrals within the current healthcare system.
The key goals for the project participants were to align their actions with and become engaged in the process of change communicated by implementing the STEADI algorithm. The specified objective was accomplished by improving the current communication channels between nurses and patients and among nurses and healthcare experts. As a result, comprehensive data for each patient was collected and arranged accordingly so that proper decision-making could be made and effective fall prevention strategies could be deployed. Specifically, all participants were informed and instructed about applying the STEADI model in the clinical context to address the observed fall risk issue and improve the quality of patient referrals.
To empower action as the fifth step of Kotter’s Model, incentives for improved performance were introduced. Namely, nurses received active support and training options to apply newly developed skills to manage the issue of falls and address the referral situation. To create short-term wins as the sixth phase of the model implementation, minor milestones such as the reports identifying improvements in the public health levels within the target community were introduced. Finally, to ensure that the change continued and was accepted, thus, implementing the seventh and the eighth steps, the project established a reporting system for nurses to use to offer feedback concerning the efficacy of the system’s performance and the challenges in applying the STEADI tool. The eight-step approach of Kotter’s model offered simple but effective planning that assisted with this project’s intervention (Kotter, (1995).
Review of the Literature
This section is guided by the primary themes and subthemes noted earlier in this chapter and found with the help of a systematic literature review, as well as a non-systematic review, on the topic of educational interventions for falls and related fall identification and prevention. The approach consisted of reviewing the major healthcare databases according to Polit and Beck (2017) and finding the literature that could be found to test or discuss the intervention, especially the STEADI algorithm and its impact on fall identification and prevention, although all other information-based interventions regardless of their application to patients, nurses or caregivers were considered as well. The articles were included only if they were in English; also, the goal was to exhaust the literature on educational fall interventions examined within the past five years, but since the project had been going on for a while and since certain literature from other years proved to be helpful, some additional older literature was included. Thus, the literature includes a systematic review of the sources in English in the past five years dedicated to educational fall interventions and some older sources and general fall sources.
The first theme is interventions needed for falls, while the sub-themes included falls and reduced quality of life and complex causes of falls. The second theme is educational interventions with sub-themes such as educational interventions and positive outcomes and methods of establishing intervention effectiveness. The third theme is STEADI studies with sub-themes such as care quality improvement and caregivers and fall prevention.
Interventions Needed for Falls
Falls are a common problem in the elderly population, which could lead to serious injuries and complications. Due to the age-related changes in the body systems, older individuals might suffer from impaired balance and poor body control, leading to higher risks of falls and higher risks of fall-related injuries (Shahrbanian et al., 2021). Furthermore, the psychological factors, including the fear of falling and additional tension caused by that fear, could also contribute to falls in individuals aged 65 or older.
Falls could be prevented or at least minimized through risk screening in primary care and other settings. Whereas all individuals older than 65 are at risk of falls, individuals who have additional risk factors, such as impaired vision or orthopedic disorders, are also at increased risk. Timely detection of fall risks and referrals to physical therapists, conducted in the frame of a comprehensive screening offered by an evidence-based tool such as the STEADI algorithm, is essential for reducing the risks of falls in high-risk groups of elderly patients. This section on interventions needed for falls is supported by subthemes on falls and reduced QOL, complex causes of falls, and addressing falls through information.
Falls and Reduced Quality of Life
First and foremost, falls are dangerous and capable of diminishing a person’s QOL (Durgun et al., 2021; Shahrbanian et al., 2021). Multiple quantitative studies have demonstrated that falls could cause issues, including associated injuries. Burns et al. (2020) presented a statistics study based on the Vital Statistics and Behavioral Risk Factor Surveillance System data, demonstrating the importance of falls for older men and women in the U.S. Specifically, the authors highlighted the importance of falls (713/1000 in 2018), fall injury (171/1000 in 2018), and fall deaths, which increased 16% between 2012 and 2018 to 64/1000, a statistically significant increase). Additionally, the authors found an increase in fall injuries among men, who reported fewer falls than women. In summary, the article offers useful if broad findings on falls in older adults in the U.S. There were no findings in the article regarding Florida. Still, the U.S. rates remain important, and they show the rates and changes in rates of falls, which justify the present project as one dedicated to an important issue of physical therapy referrals to prevent falls.
Gazibara et al. (2017) carried out an epidemiological study to determine the frequency of falls in older people, n = 354 older Serbians, and a number of other characteristics of falls that would be useful for fall analysis and prevention. The sample consisted of people older than 65, and they came from one community health center. The main data collecting method was detailed interviews and the Falls Efficacy Scale. The frequency of falls amounted to 15.8%, and half of them occurred during walking; moreover, almost half of all falls were injurious (49.1%). The most common injuries sustained included head hematomas and soft tissue contusions (Gazibara et al., 2017, p. 215). The majority of the people experiencing falls were female and had a fear of falling (Gazibara et al., 2017, p. 215). The article focused on the population of one Serbian health center; still, the findings provided an idea about the characteristics of falls that make them dangerous.
Hill et al. (2015) carried out a randomized controlled trial (RCT) with 3,606 inpatients from Australia to determine the effectiveness of a fall prevention intervention, finding that roughly 30% of the falls were injurious. The authors justified their attention by highlighting that falls were very common events in hospital settings and established the aim of examining the effectiveness of a specific program meant to reduce falls in patients through the education of both patients and staff. The project was multicenter, which improved the generalizability of the findings; it also took place over 50 weeks, which made it approach a longitudinal research method. The allocation to the control and intervention groups was random, as is required for an RCT, and their comparison showed that no significant differences between the groups could be found. The only important difference was that the number of falls (based on a patient-days fall registration system) was smaller in the intervention group, implying the rates of falls were reduced in that group, so the program was clinically effective. The project involved only Australian patients, but the number of patients was large, and they came from different centers, which improved the generalizability of the results. Furthermore, the use of the RCT as a research design improved generalizability. Overall, this was a very solid source that assisted in an improved understanding of the mechanisms behind reducing falls in hospital settings. In addition, the article showed that the intervention also reduced the number of injurious falls.
In an effort to make their project more longitudinal, Hill et al. (2015) performed a follow-up to an RCT to determine the effects of a fall intervention on post-discharge falls, finding that more than half of all the falls were injurious. The project’s goal was to determine the effects of an educational program on post-discharge falls, which the authors explained by the fact that older people tended to experience falls after hospital discharge. The methodology was an RCT once again, and the settings were Australian; the duration of the study involved six months of following the participants. The intervention presupposed using a video and a workbook, and a discussion. Only 382 participants were involved over 65 years of age, with 188 people in the control group assigned randomly. The results suggested that significant differences between the groups in fall rates did not exist, but the participants reported falls, and half of them were injurious (49.7%). The program was tested with a rather small number of participants coming from one country; as a result, the findings were not very generalizable. Still, the findings regarding the number of injurious falls seemed to be in line with the rest of the cited research. However, admittedly, more investigation was required to determine the effectiveness of this specific program and other programs. This source could be considered counter to the rest of the articles on fall prevention interventions in that it did not find a statistically significant difference between the two groups. However, the findings should only be applied to the specific intervention used by Hill et al. (2015).
In summary, the literature on the topic suggested that falls, especially in older adults, were likely to be injurious and reduce the QOL of patients, which is why it remains important to address the issue. This subtheme included the important source that contradicted the findings discussed in other studies, including an older source that was included because of its connection with the critical contradicting source. Overall, the literature presented in the following themes supports the exact same idea: all the authors on the topic of falls, fall assessment, and fall prevention, whether in qualitative or quantitative sources, demonstrated that falls remain a significant issue that should be addressed.
Complex Causes of Falls
Falls are associated with numerous factors, especially commonly related to a combination of factors working in concert. Both qualitative and quantitative studies provided relevant information, suggesting that knowledge might be among the factors of interest (Dhalwani et al., 2017; Gomez et al., 2017; Haines et al., 2015; Kiyoshi-Teo et al., 2017; Kuhirunyaratn et al., 2019; Mota de Sousa et al., 2017; Yoo et al., 2016). In this section, the potential risk factors of falling in elderly patients are reviewed, focusing on psychological factors and other ones that could be affected by education.
Hopewell et al. (2018), in a Cochrane systematic review, included 62 trials with almost 20,000 community-dwelling older adults, most of them women. The research involved multifactorial interventions that typically incorporated exercise, technological interventions, psychological ones, medication review, and multiple component interventions that typically included exercise and education. The authors concluded that either option could be effective, and all of them addressed diverse fall risk factors, including medication, frailty, instability, gait and balance problems, as well as problems with vision and the presence of several chronic diseases; additionally, environmental factors were identified, for example, insufficiently secure rails, or slippery surfaces and bad footwear. Typically, an interaction of factors was relevant, and additionally, knowledge of risk factors was shown to be effective in reducing falls. It was also noteworthy that among the studied outcomes were fall rates, number of people experiencing falls, number of people sustaining several falls or fall-related injuries, and number of people experiencing hospital admission or requiring medical attention as a result of their fall. Overall, the project was helpful in contextualizing the current project. However, the limitations of the project were noteworthy, specifically highlighting that many of the projects had issues with quality, which might have affected the quality of the results of their review. Additionally, not many countries were represented in the project. Still, the findings were important.
Pua et al. (2017) studied a connection between postural balance and fall risks in the elderly; they recruited 247 adults who visited a specific emergency department (ED). The outcomes were fall rates and gait speed, as well as falls efficacy and postural balance. The participants were observed for six months. Based on the findings determined with the help of a multivariable proportional odds analysis, with confounding variables taken into account, the authors concluded that falls efficacy affected the relationship between balance and fall risk, and low falls efficacy was also associated with low falls and reduced gait speed. In other words, falls efficacy was considered among the modifiable features that could reduce the risk of falls in older adults. Admittedly, the sample was not very large, and it was mostly drawn from one location, which might have affected the outcomes. However, the analysis was rigorous, with confounding variables taken into account, and the methodology was that of a prospective cohort study, which guaranteed a high level of quality. Overall, the findings could be used to highlight the complexity of the interactions of risk factors for falls and demonstrate that education that targets specific risk factors could be useful.
Mota de Sousa et al. (2017) used the approach of a systematic literature review to identify risk factors for falls in older adults who resided in a community. The authors highlighted that they identified 50 risk factors, of which only 38 were listed on the Taxonomy of the NANDA International. The authors explicitly meant to update that taxonomy. The following key risks were identified: physiological factors (most typically, difficulties with seeing, walking, balancing, as well as pains, lowered strength and mobility, and several specific illnesses or conditions, for instance, urinary urgency), environmental ones (for example, the absence of grab bars or anti-slip materials in strategic areas), pharmacological agents (various medications, especially polymedication), cognitive factors (different reasons for changes in cognitive function), psychological factors (for instance, the fear of falling and depression), socioeconomic factors (in particular, low education), and personal factors (most commonly, age and gender). This was a comprehensive, nurse-oriented review that provided findings based on 62 sources, all of which had their own limitations but could still be used due to the focus on primary sources with predominantly cohort or correlational and descriptive studies, as well as a few RCTs and quasi-experiments. It was noteworthy that the evidence was gathered from all over the world, but, admittedly, the U.S. and Australia were the most well-represented ones. Overall, the review provided some good grounds for understanding the causes of falls. It could be used to contextualize this project and its intention of considering and adjusting the psychological factors of falling.
Schoene et al. (2019) provided a systematic review of specifically the issue of the fear of falling. The authors investigated the association between this factor and the QOL in older people. A total of 30 studies were identified with roughly 29,000 people, the majority of them women, and through a literature review, the authors demonstrated that fear of falling did appear to be associated with QOL, lowering it, while falls themselves did not have a similarly profound effect on QOL. Fear of falling was associated with falls as one of the relevant risks (Perrot et al., 2019); however, the study specifically demonstrated that fear of falling in itself was a major issue that deserved to be addressed. Falls were not shown to have an impact on fear of falling. The authors highlighted the importance of interventions that address the fear of falling, which would be expected to affect the risks of falling while also improving the QOL in older patients.
In summary, the number of sources on the causes of falls was substantial and suggested that there are diverse causes, several of which could act simultaneously. As a result, the primary intervention that the sources suggested was a holistic intervention. However, admittedly, the intervention that targets education would also be appropriate based on the presented findings.
Mamani et al. (2019), in an interview-based study (n = 97; Brazil), investigated caregiver practices and found that their knowledge of the topic of fall prevention was superficial. In fact, almost half of the participants exhibited little to no knowledge of the topic, and only one-fourth of them practiced different fall prevention methods. Despite using qualitative methods, the project was focused on quantitative analysis, and it showed that more knowledge and improved attitudes toward fall prevention could be developed within the population. The authors concluded that insufficient knowledge and inappropriate perceptions of fall prevention might have been among the reasons for the low percentage of practicing fall prevention methods, but their study was not correlational, so they could not assert that based on their data. The project had a small sample of only Brazilian people; however, the research on caregivers was limited, although the findings provided at least some information on the topic.
Singh et al. (2020) evaluated the state of fall prevention at Canadian facilities and demonstrated interesting conclusions about the educational component of fall prevention. The project studied a number of specific facilities, and it used the documentation to report the results, finding that pre-fall policies and procedures were intelligence-focused. To be more precise, they included understanding what a fall meant, establishing risk assessments, and, eventually, fall prevention approaches, which incorporated environmental and educational components. Overall, the authors concluded that similarities were found between the different facilities, but more evidence-informed approaches were required.
Similar to Mamani et al. (2019), Lim et al. (2018) prepared an interview-based study (n=100; patients from Singapore) that looked into patient fall experiences and uncovered multiple negative attitudes that could increase patient risks and might need unlearning. Lim et al. (2018) conducted a qualitative, not quantitative, study and uncovered several key themes. For one, many of the participants appeared apathetic and uncaring about falls. They shared the perception of the inevitability of falls and considered them less important than having hygiene issues. Furthermore, the participants blamed themselves for falls, did not want to “impose” on nurses, and were prone to overestimate their abilities, which resulted in falls. They also experienced difficulties with remembering fall-related advice. The project participants were not representative of any specific population, although most of them were male and over 60 years old. Additionally, the sample was relatively large for qualitative research but insufficient for generalizations. Overall, the research was not generalizable, but it provided some information about the possible specifics of fall experiences, including the attitudes that need unlearning and that more attention needs to be paid to teaching fall prevention.
The fact that falls remain a major concern justifies this project (Müller et al., 2019). Research into the causes of falls is important for their prevention. As could be seen from the qualitative studies, patients and caregivers recognize the need for education as well. While there are limitations to qualitative research, in terms of the subjective needs of participants, they are capable of producing relevant data. Since the voices of patients and caregivers are important to consider, the presented literature was critical for understanding the issue important to this project.
Educational Interventions
Educational interventions work, a conclusion made after scientific investigation of many studies (Frith, 2017). The analysis of this project interested in the literature is based on various unique outcomes, certain of which shall be reflected in the current project. Additionally, it is essential to note that aspects of educational interventions had been studied, again supporting the need for this project, particularly in patient education on fall risk identification and physical therapy referrals (Schoberer et al., 2016). The following subthemes support this second primary theme of educational interventions that work: educational interventions and positive outcomes; methods of establishing intervention effectiveness; STEADI studies; fall risk identification and physical therapy referrals.
Educational Interventions and Positive Outcomes
According to the literature, educational interventions work (Frith, 2017; Lyons & Hall, 2016). However, it might be more accurate to state that many educational interventions to prevent falls appear to have positive outcomes specified by the relevant literature. Nevertheless, it is also important to remember the work by Hill et al. (20159), suggesting that different interventions might have different levels of effectiveness. This section presents the findings related to educational interventions that do appear to be working.
Perrot et al. (2019) carried out an RCT of a complex fall prevention intervention (n=30), and they found that it reduced the fear of falling. It was important that the intervention was not just education; it was augmented with physical activities. However, the RCT was carried out specifically to compare the education program with physical activities against physical activity alone, which means that it was the educational program that made the difference between the intervention and control groups. The project had a very small sample, with only 30 people involved, most of them women. There also were significant differences between the control and intervention group pre-test. The assignment was random, though, which increased the quality of the project and made it an RCT. The participants were specifically older adults who had fallen at least once in the year before the project, which used the Timed Up and Go test, the Tinetti test, and the Falls Efficacy Scale, all of which are accepted methods. The findings suggested multiple outcomes, but the most important one for this project was that patient education was shown to affect the fear of falling by reducing the fear positively. More research with a bigger sample would be required, but the project offered some insight into the effects of training on fall prevention.
Ueda et al. (2017) conducted a pilot RCT to test an educational fall prevention program (n=51), which effectively reduced fall rates. To be more specific, the program was meant for older orthopedic patients who had experienced at least one fall in the year preceding the project, and it was based on tailored interventions (educational programs) that incorporated floor plans. The sample size was small for an RCT, but the project was a pilot study. Even with the small sample, it was obvious that the intervention group had fewer falls and near-falls. However, the limitations of the sample were crucial, as well as the fact that the project employed an innovative and never-before-tested program. More research definitely was required to make conclusions on the topic.
Carlucci et al. (2018) conducted a quasi-experimental project (n=215) implementing an intervention to improve falls efficacy and functional reach. The intervention incorporated “joyful movement,” a complex intervention incorporating education, psychological intervention, and retraining of biomechanics. Only one group of older adults was involved in the project, with only 86 providing feedback and only 102 completing all the assessments before and after the intervention. Still, the results suggested that the intervention improved both the mobility and falls efficacy of the participants. The project incorporated both educational and other elements, which might have affected the study’s outcomes; furthermore, the sample admittedly was small and drawn from one facility. Still, the research clearly suggested benefits to interventions with an educational component relevant to this project. The authors also recommended more research on the topic to determine the program’s effects on fall rates.
Ott (2018) focused on community-dwelling patients, making the article suitable for this project. Ott (2018) conducted a pilot study to investigate an educational session and its effect on knowledge, fall prevention behavior, and falls. The design was quasi-experimental, which was helpful for this project, but the sample was small, with only eight participants completing all activities related to the project, making the tracking of falls difficult. The authors could only report one fall in the group, which was not enough for statistical analysis. Still, fall knowledge and prevention behaviors were both demonstrated to increase after the intervention. Overall, the article was similar to the current project design-wise, and it also provided some information on the ability of educational interventions to improve fall knowledge and prevention.
Kiyoshi-Teo et al. (2019) developed a multi-method research project that focused on veteran inpatient fall prevention recommendations and how they were implemented based on the Plan-Do-Study-Act cycle. Using the fall rates as their outcome and multiple qualitative and quantitative methods, the authors studied two units within one healthcare system in Portland. The use of fall rates could be considered a recommendation for the project on selecting an appropriate outcome. As for the findings, it was relevant for this project that despite the carrying out of educational interventions, mostly connected to communicating information on the topic, the patient participants still reported a lack of knowledge on falls. From this perspective, it was apparent that there were more and less effective education methods, and simple communication of information might not be effective.
Kuhirunyaratn et al. (2019) focused on the urban elderly, making the article especially relevant for this project. The aim consisted of studying the effects of an education program, and the settings were urban Thailand. The study was quasi-experimental, with two different communities involved; two groups were formed with roughly 100 people in each, all over 60. They were all registered at one healthcare unit, and the intervention group was subjected to an educational buddy intervention. The pre-experimental fall risks were slightly smaller for the intervention group, but the intervention helped to decrease it to a statistically significant level. The findings implied that educational interventions could affect fall risks through medicine usage and other factors, which was a helpful finding for this project.
Lyons and Hall (2016) focused on Latin America and the Caribbean, which might have limited the applicability of the article to this project, but which also showed that the issue of falls is worldwide. Furthermore, the article focused on community-dwelling older adults, aligning with this project. The article’s goal was to determine the feasibility of an educational intervention implementation meant to prevent falls in Grenada. The article sample was small, 62 older people, but it showed that older people in Grenada might be interested in learning more about falls and require fall knowledge, which further supported the idea that educational interventions are a helpful and empowering option for fall prevention.
Ferreira et al. (2019) aimed to construct a fall prevention nursing process to apply to the elderly with Parkinson’s disease. The target population was rather specific and different from the target population of this project. However, it was clear that both projects aimed to study the older population (over 65), which brought them close. Only nine older people with the disease were involved, and through interviews and workshops, they helped to produce two games, and one educational booklet meant for fall prevention. The authors highlighted empowering the patients and improving their ability to exercise self-care with the help of the specified new interventions. The article was in line with the present project, particularly from the perspective of improving self-care. It also introduced ways in which caregivers, specifically formal caregivers, could assist patients with fall prevention. However, the population of the article was specific. While the approach toward the development of the materials could be used outside of the article, the materials themselves had not been tested in terms of their effectiveness. Thus, the article had its limitations, although it showed that educational materials could be developed in ways that empower the target population.
In summary, there exists sufficient research on the topic to suggest different educational interventions for fall prevention could work. Admittedly, the studies typically had small samples or otherwise limited samples. It was rare for one and the same intervention to be tested several times. In this light, the work by Hill et al. (2015) was especially important because it contradicted the rest of the evidence suggesting the usefulness of educational interventions or interventions with educational components (see below). Overall, it could be suggested that more research is still required, specially dedicated to one particular intervention. Additionally, it was clear that different methods of establishing intervention effectiveness could be discovered, and the next subtheme discusses this topic in detail.
Methods of Establishing Intervention Effectiveness
The effectiveness of prevention interventions could be established with the help of fall numbers, likelihood, frequency, and so on. Overall, there are a lot of valid methods for determining the quality of an intervention. In this section, some quantitative options are presented; qualitative ones are outside of the realm of this project because it is quantitative.
Nakagami-Yamaguchi et al. (2016) conducted a pre- and post-test study in which an animation movie-based prevention method reduced fall frequency and likelihood. The animation was supposed to provide education, which explains the study’s relevance for this project. The study was a pilot one, and it involved both patients and caregivers, which is why this not very recent study was included in the review. However, the project mostly implied nurses as caregivers, which makes it less relevant. Still, the research showed a clear need for educational intervention, with only 30% of the patients understanding the instructions on nurse interaction in terms of fall prevention before the intervention. Furthermore, the findings implied that the 269 patients involved and 304 nurses involved managed to reduce the patient falls to 8.6% from over 15%, which proved statistically significant. Furthermore, the likelihood of falls decreased in older patients in a statistically significant way. Overall, the animation proved to be an effective educational tool, and it was specifically more effective for older patients. The study was a pilot one, which explained its limited sample. More research on the topic might be required for additional conclusions.
Dykes et al. (2017) studied falls in acute hospitalization facilities, two of them located in the U.S., with a focus on an intervention called TIPS (Tailoring Interventions for Patient Safety) and its effects on mean fall rate and injury fall rate. The goal was to test the intervention, which could be considered a clinical decision support tool, and the findings showed that one of the facilities showed significant improvements in both desired outcomes, while the other one only showed improvements in one. The study’s strong feature was that it tracked the compliance of the intervention, but other confounding variables were not considered in the pilot implementation project. More research clearly was required for conclusive statements, especially research in different settings. Still, the project clearly showed that certain measures of falls could be used in the research for fall prevention interventions.
Jie and Deng (2019) tested fall education (n=178 female patients with osteoporosis) in a pre- and post-test study, showing a reduction in fall risks and fall likelihood. The topic was rather specific in that the authors focused on osteoporosis patients receiving zoledronic acid, which was explained by a high rate of falls in the population and the negative outcomes associated with falls in the population, specifically, morbidity and mortality. The project involved controls and random assignment to the respective groups (86 intervention cases with a fall prevention education at the time of zoledronic acid administration and a telephone follow-up, and another session a month later). The data were collected with the help of a survey before the intervention and after it one year later. The project had a relatively small sample, and it only recruited female patients with osteoporosis, which limited the findings’ applicability. However, the results implied that it is possible to reduce fall risks with the help of fall prevention education.
Kelley (2022) reported the findings of a practice project meant to test an intervention bundle for fall prevention with two educational elements out of five, including daily education of patients and educational handouts. Other elements included patient risk assessment, ensuring the carrying out of previously established fall prevention strategies, and a “safety agreement” with the patients meant to facilitate their interactions with nurses. The fall rate decreased significantly by half, and the authors highlighted an increase in staff compliance with fall prevention strategies. They indicated issues with staff turnover as an obstacle to the project but pointed out that communication between the nurses and patients was of utmost importance. The findings suggested that interventions with an educational component could be helpful and that fall rates could be used to assess such interventions and their success.
Shim and Kim (2019) carried out a quasi-experiment to investigate the effect of fall prevention education in older patients. The project had an experimental and control group, both about 30 people, which was a small sample. It still allowed for the use of parametric tests meant to compare the fall knowledge and fall prevention behavior in patients. The intervention consisted of an educational DVD and leaflets; they demonstrated improved scores in both parameters. The information about falls in either group was not provided. The authors highlighted that the desired outcomes were achieved with the help of this complex education system, implying that it could be further researched. From the perspective of this project, the article was a relevant methodology and demonstrated the effects of educational fall prevention interventions.
In summary, there are a lot of sources that could be used to help direct a new project on the topic of educational fall prevention interventions. It is especially true since they are sufficiently diverse, with different approaches to measuring the same variables. Overall, this literature assisted in producing the methodology for the present project.
STEADI Studies
The STEADI intervention has been studied and written about in professional literature (Casey et al., 2017; Eckstrom et al., 2017). However, not all studies are relevant to this project. In fact, none of them considers the educational component of the STEADI from the perspective of caregiver education. Among these, the article by Eckstrom et al. (2017) could be considered the basis for the CDC guidelines concerning the implementation of the STEADI algorithm. The following projects were used to gain information about the STEADI algorithm intervention.
Eckstrom et al. (2017) incorporated the STEADI algorithm into a clinic’s routine. The STEADI algorithm that were used to that end included the EHR, training, and workflow tools. Three-fourths of the providers were involved, and they screened more than half of the patients (773) throughout six months, most of which required and, therefore, received STEADI algorithm interventions. The authors concluded that the screening burden was reduced by the STEADI algorithm, although the number of patients considered high-risk increased as a result. The only intervention that was not carried out often was reducing the medications that affect fall rates. Overall, the project provided an example of STEADI algorithm implementation, which could apply to this project’s intervention.
Vincenzo and Patton (2019) aimed to determine adherence to the STEADI algorithm intervention among older patients. They performed a set of semi-structured interviews, showing that only half of the forty participants who fully participated in the study followed the STEADI algorithm recommendations after six months, and only about three-fourths remembered any recommendations. Additionally, 32% of them fell, and out of these people, more than half did not follow the STEADI algorithm recommendations at all. Overall, the authors unearthed an important issue of long-term STEADI algorithm effects. While this project cannot track long-term effects, this issue informs the project’s STEADI algorithm implementation.
Casey et al. (2017) carried out a study meant to apply the STEADI algorithm in one specific clinic with the help of the Kotter framework. Over 400 people were screened during the entire project. The researchers reported that the successful implementation needed relevant EHR tools, a workflow incorporating the STEADI, algorithm and appropriate leadership. The researchers described the project as successful, but there was no direct assessment of the STEADI algorithm in the project, which was not its aim. Therefore, more research on the topic is required.
Taylor et al. (2019) focused on using the STEADI algorithm initiative through an interprofessional education approach. The justification for the research consisted of highlighting the importance of being trained in working with older adults and fall prevention for healthcare providers. The article involved healthcare students, faculty, and older adults (all below 32 people) in training the former and involving the latter in carrying out a STEADI algorithm intervention. Student knowledge increased statistically significantly, especially regarding fall prevention and applying the STEADI algorithm, showing that the latter could be used for geriatric health education. The sample size was not very large, but it could be used to demonstrate the STEADI algorithm utility, especially in fall prevention education.
Care Quality Improvement
Wongrakpanich et al. (2019) carried out a quality improvement project to investigate the STOP-FALLING checklist, which was developed specifically for one long-term care facility. The project used a pre- and post-test approach (three months before and after checklist introduction) with a rather small sample of 32 patients. The findings showed an improvement in fall rates and staff satisfaction, reduced falls with minor and major injuries, and reduced frequent fallers. The project’s approach could be considered a pilot study, which was why the small sample was acceptable, but the authors proposed further advancing the study of the checklist. This article was relevant for this project because it showed how a fall prevention intervention could be studied while also highlighting the importance of tools like the ones included in the STEADI fall prevention initiative.
Urban et al. (2020) commented on the lack of literature on fall prevention in primary care and introduced the STEADI algorithm program with a focus on its education materials in a primary care clinic. The staff involved included 29 people, and they were educated on the topic of falls with the help of the STEADI algorithm materials, resulting in a statistically significant increase in the mean knowledge score before and after the test, although no statistically significant difference between the control group and education group in terms of STEADI algorithm use was found. The authors concluded that the use of the STEADI algorithm was recommended from the perspective of knowledge improvement, but the project was not successful in implementing the STEADI algorithm. The article could be used to demonstrate the value of the STEADI algorithm, but it did not provide directions on how to implement them.
Lohman et al. (2017) focused on investigating the STEADI algorithm initiative and its validity and adaptability. The article used the National Health and Aging Trends Study to gather information about a large, representative sample of older adults of over 7000 people. The information was used to apply the STEADI algorithm to adults and determine its ability to predict outcomes, including falls and mortality. The findings suggested that the STEADI algorithm was valid in that it was able to predict adverse outcomes.
Mark and Loomis (2017) focused on the implementation of the STEADI algorithm into clinical practice. The article was theoretical, and it consisted of a literature review and a commentary on the STEADI algorithm. It concluded that the main issue with STEADI algorithm implementation likely involved the actual utilization of the toolkit, evidenced to be effective based on the literature review. The authors highlighted that the STEADI algorithm could prevent falls and save funds, but they recommended more research on the validity and reliability of the STEADI algorithm. Overall, the article could be used to support the use of the STEADI algorithm and support the need for this project to investigate the STEADI algorithm impact on fall risk identification and physical therapy referrals at the project site.
Johnston et al. (2018) implemented the STEADI algorithm to determine its impact on a primary care system. The article was especially relevant for this project since it was carried out in the U.S. and involved four outpatient clinics. The number of people involved exceeded 12000 older than age 64, which was large for a study that only involved a part of New York as its setting. The STEADI algorithm was used to develop a fall plan of care for a group of participants who were at risk for falls; a control group with fall risks and no plan of care was introduced, as was a group classified as having no risk of falls. The plan of care based on the STEADI algorithm was capable of reducing the likelihood of fall-related hospitalizations in a statistically significant way compared to the group with no plan of care; in fact, the people with no plan of care showed a similar likelihood of fall-related hospitalization as people who were classified as having no risk of falling. Therefore, the STEADI algorithm was shown to be effective in preventing fall-related hospitalization, which implies that it was also helpful in reducing the likelihood of falls. Naturally, the article described a study that took place only in one region of the U.S., which implies that the results for Florida might be different. Still, the article offered a lot of evidence for the present project.
In summary, the presented literature suggested that the STEADI algorithm was a valid and helpful tool, and even though there was little investigation of its effectiveness, research suggested that it was very capable of bringing along positive outcomes in terms of fall prevention. Recent sources showed that the issue remains topical, but the findings also suggested that more research is required. Overall, the literature could be considered to justify this project by indicating that the intervention is an evidence-based solution in need of further investigation.
Caregivers and Fall Prevention
Caregivers are rarely considered in fall prevention, especially informal and domestic caregivers, which makes every recent article on the topic critical and relevant for the current project (Schoberer et al., 2016). However, there also are limited relevant scholarly sources. A number of relatively recent articles have been identified that considered caregivers, although their contribution to the topic is not equal.
Xu et al. (2017) conducted a qualitative project to develop a fall prevention program for stroke survivors based on the survivors’ opinions (nine people), as well as caregivers and helpers (eight people). Through interviews and thematic analysis, the project determined that both survivors and their caregivers would benefit from fall prevention interventions, and they determined that caregivers were often overprotective of the survivors they cared for. These findings suggested that caregivers are an important element of fall prevention; The qualitative study means that its level of evidence is not very high (Polit & Beck, 2017). Overall, this study was only included because there was little to no information on caregivers’ role in fall prevention.
Schoberer et al. (2016) is an older source, but it provides a qualitative study of the perspectives of patients and caregivers, including family caregivers, regarding an educational fall prevention intervention (specifically, a fall prevention brochure). The participants (25 residents, 12 family caregivers, and 14 nurses) reported they were interested in learning about fall risks and methods of recovering after one, and family members also wanted to know more about strategies to prevent falls. The study’s information suggested that education for caregivers would have been helpful, which justifies the present project.
Hoffman et al. (2017) presented an analysis of different categories of caregivers from the perspectives of their association with falls and injurious falls. The findings suggested that different formal and informal care levels were associated with a reduced risk of falls and injurious falls, although to a smaller degree. The total sample was almost 8000 people, 20% of them with caregivers. The project also took place over several years, further boosting its quality. The sample did have the limitation of being only applicable to the U.S. The project suggested that caregivers are important for fall prevention and that the amount of effort they offer along with their qualifications could improve the quality of care.
Nakagami-Yamaguchi et al. (2016) carried out a study to develop an educational movie as a method of fall prevention. The evaluation involved implementing the movie in a pre- and post-test fashion. Both patients and caregivers were involved in movie evaluation, with pre-intervention surveys showing that before the movie, not all patients understood the fall prevention instructions correctly (for one type of instruction, only 33% of the surveyed patients had a correct understanding). After the intervention, understanding improved, and the number of falls decreased significantly in older patients. The findings suggested that novel education methods could improve participants’ understanding of nurse instructions, and it also suggested involving caregivers in the process of fall prevention.
Wilkinson et al. (2018) presented a literature review on fall prevention strategies outside of hospitals among older adults and their caregivers. The 17 studies, only two of which explored exclusively caregiver perspectives (four additional ones explored those of caregivers and patients), included a sample of about 600 people, 102 of them caregivers. The findings suggested that the caregivers were mostly using the strategy of discouraging independence, highlighting that they might be ill-equipped to provide care and required additional education.
Zhao et al. (2019) collected information about acute care hospitals, limiting the article’s applicability to this project, but the article was a thorough and well-documented investigation of the implications of factors associated with falls (including injurious falls) for the prevention of falls. A major issue of the study was that it was a literature review and not a primary source, but literature reviews are also useful in developing arguments (Polit & Beck, 2017). Regarding the present project, the study justified it by discussing educational interventions as an existing method of preventing falls and engaging patients and their caregivers in fall prevention. The authors concluded that there remains a need for more than single-component interventions, but they also recognized the usability of educational programs.
Vonnes and Wolf (2017) focused on falls in oncology, limiting the applicability of the findings. Still, the project tested a specific fall prevention technique (Fall Prevention Agreement), which involved formal caregivers and older adults. As a result, the falls demonstrated a reduction over the course of two- and eight-quarters, the former demonstrating a sharper decrease of 37%, and the latter still showing a substantial decrease of 58.6%. The authors’ explanation concerned itself with the ability of the Fall Prevention Agreement to improve adherence and participation both for the team and patients. Additionally, the article commented on the ability of Fall Prevention Agreements to involve informal caregivers and families. Thus, the article highlighted the importance of participation in fall prevention activities among informal and formal caregivers and patients, demonstrating that a reduction in falls was observed when a measure meant to enhance fall prevention was implemented. The article could be used to confirm the importance of the contribution of different groups to fall prevention, including informal caregivers.
Ximenes et al. (2019) presented a study that began with constructing and ended with assessing a booklet developed by nurses and patients to prevent falls in medical institutions. As a result, the topic was not fully consistent with the one selected for the project, but it could still be useful from the perspective of educational materials being implemented in fall prevention. The booklet was validated and demonstrated a Content Validity Index of 0.98-1. Patients deemed it understandable. The main weakness of the project was the lack of actual testing of the booklet, but the authors justified the need for educational materials in fall prevention, and they highlighted the ability of patients to contribute information relevant to educational material design. Thus, the article supported the importance of educational materials and offered information about empowering patients and their families through their involvement in fall prevention.
Ang et al. (2019) carried out a qualitative study to gather information about the concerns exhibited by caregivers regarding the risk of falls in their patients. The size sample was small (22), and the authors only used one tertiary hospital from Australia. The goal was to interview (semi-structured) the participants and determine the themes emerging from the collected data. The authors found that there were issues related to caregiver awareness of fall risks and their knowledge and ability to support their patients. Furthermore, a separate theme was connected to the support provided by healthcare professionals. The authors concluded that caregiver knowledge and awareness were critical to fall prevention and well-being. Their recommendation was for healthcare professionals to offer caregivers sufficient information about falls to improve fall prevention and their well-being as well as that of the patients.
Black et al. (2018) offered a model of fall prevention that focused on several elements, including patient mobility, injuries, fall prevention, and ensuring caregiver safety, especially from the perspective of musculoskeletal injuries. The article focused on critically ill patients and ensuring their mobility. The article was relevant to older people and fall risks since those were taken into account. Additionally, the caregivers in question predominantly were formal caregivers, but informal ones also were considered. Finally, the authors’ methodology consisted of a literature review; the article was not a primary source. The article demonstrated the importance of viewing safety and other features of the well-being of caregivers while preparing fall prevention strategies. Some issues with the relevancy of this article could be used to also highlight the lack of the literature on the topic. Caregivers are not studied as extensively as patients when it comes to fall prevention.
Juckett and Poling (2020) focused on home- and community-based services, specifically, their ability to perform fall risk screens and referrals to relevant services. The article used a qualitative approach, involving 26 staff members and administrators with the help of interviews, including focus group interviews. The results suggested that the staff of such services was able to perform fall-related services but was not trained to do it and had even less knowledge on actual fall prevention. Additionally, the service administrators reported a lack of connection with fall prevention providers. The authors recommended rectifying the named issues. The article discussed professional caregivers, but it highlighted the different types of services that such caregivers could offer. Additionally, it showed that even professional caregivers could require help in fall prevention education. Therefore, the article was useful for the project by demonstrating the importance of fall prevention education.
Meyer et al. (2019) focused on people with dementia and the difficulty of engaging them in fall prevention strategies, highlighting the importance of caregiver involvement in such instances. The authors developed a discussion tool to engage both patients and caregivers in fall prevention. Aside from carrying out a literature review to support the tool, the authors also trialed it for six months with 25 patients, who had increased fall risks, and their caregivers. The findings concluded that the tool facilitated communication and engaged participants (both patients and caregivers) in fall prevention. The research was aimed specifically at people with dementia, which provided additional motivation for including caregivers in fall prevention. However, the authors’ goal was to engage the patients as well. Therefore, the project highlighted the importance of empowering both patients and caregivers through the communication of fall prevention information.
Montgomery and Smith. (2020) focused on a program to train non-clinical caregivers to prevent falls outside of clinics, which made the article relevant for this project. The authors examined the importance of falls among older adults and the lack of routine incorporation of fall prevention into nursing practice. The authors proposed the solution was the involvement of non-clinical caregivers with the help of the STEADI algorithm, making the article further relevant for this project; the use of pre- and post-test design also directed the project’s design. Statistically significant increases in knowledge and confidence were identified, and the participants showed good retention of the information during the follow-up. However, the sample was small (less than 30 people), and it was only from the U.S., which was relevant for this project but might not be very generalizable. The findings were important, highlighting the importance of caregivers in fall prevention, as well as gaps in knowledge they exhibited before the project.
In summary, there were a few sources on caregiver involvement in fall prevention, including prevention that was meant to be carried out with the help of education, that were published in the past few years and were in English. This seemed to be a major drawback of the current literature on the topic, especially since the presented sources, as well as the previously discussed qualitative sources, suggested that caregivers in fall prevention were important. Thus, it was critical to produce more literature on the topic, which justifies the present project. Additionally, the need for education and the potential positive outcomes of educating caregivers received some support in the recent literature. Therefore, this project could be considered justified.
Summary
The purpose of this quantitative, quasi-experimental quality improvement project was to determine if or to what degree the implementation of the CDC STEADI ALGORITHM FOR FALL RISK SCREENING, ASSESSMENT, AND INTERVENTION would impact fall risk with physical therapy referrals when compared to current practice among adults aged 65 or older in a primary care clinic in urban Florida over four weeks. Research has suggested that the specific topic of this project is not well-researched, leaving a gap in practice this project could fill. Indeed, fall prevention education was a common topic in research, but specifically, STEADI algorithm education was less often investigated.
Fall prevention education for caregivers remained rather rare in the literature. The topic could be considered a particular form of research gap, exacerbated by the fact that most studies presented individual interventions that needed to be studied more than once and preferably with a larger sample. Since sample limitations were the most common limitations for the literature described so far, it was a major issue. However, other limitations were also noteworthy, such as the specifics of the methodology (for example, the lack of randomization), the drawbacks of the methodology (for example, statistically significant differences between the two groups being compared pre-test), and other issues. Overall, the research was based on the literature that exhibited some limitations and was occasionally not very recent, specifically because there was not enough literature on the topic of caregivers and fall prevention.
However, the findings still produced themes within the literature that could be helpful for this project. Thus, it became apparent from the evidence that falls remain a dangerous issue; that much was established in every piece of literature, but it was especially clear from the statistical sources included precisely for this reason. Consequently, it was apparent that falls still need to be addressed, and one of the methods for doing so is fall prevention education, which follows some of the causes and risk factors of falls. Education has been studied in a variety of circumstances, showing that it could achieve positive outcomes, including a reduction in falls, near-falls, and fear of falls, among a few other things. The STEADI algorithm as an intervention focused on education was generally supported by the literature, although more research would be helpful that would not focus on its implementation above all else.
It also was noteworthy that the current project uses some of the parameters that previously have been established as potential outcomes of educational interventions for falls. Orem’s (1985) theory and Kotter’s 8-Step Model were used to support this project, with the former explaining the key terms and variables and the latter assisting in the process of STEADI algorithm implementation. Research clearly demonstrated that the topic of physical therapy referrals for fall prevention could still be investigated in a variety of ways, including qualitative and quantitative ones. Controlled trials and RCTs were among the options that could be considered. They were employed to determine the effectiveness of an intervention, which made the suggestion appropriate for the present project.
Chapter 3 presents this project’s methodology and design after stating the problem and clinical question guiding the project. The chapter also discusses the project’s population and sample selection and instrumentation or sources of data, including validity and reliability. Next, Chapter 3 presents the project’s data collection and analysis procedures, including potential bias and mitigation, ethical considerations, and limitations. The chapter concludes with a chapter summary and an overview of Chapter 4.
Methodology
Falls during hospitalizations are an important safety concern, leading to increased healthcare costs and higher risks of fall-related morbidity and mortality. According to the CDC (2019), about 25% of elderly patients experience at least one fall per year, with more than 50% of those who fall not telling their provider about the incident. As one of the most common incidences after hospitalizations, falls and following traumatic injuries could and should be prevented. The CDC STEADI initiative has proven to be an effective tool for screening elderly patients, assessing their risks of falls, managing the underlying reasons whenever possible, and reducing the number of falls in elderly patients in recent years.
However, the STEADI algorithm still has not been adopted in some healthcare settings for several reasons, such as lack of resources or competing tasks or requiring time and attention of the administration and practitioners. The significance of and the potential risks from falls in elderly patients and resulting consequences could be underestimated and underreported. The objective of this project was to evaluate the potential benefits of the STEADI algorithm compared to current practice at the project site. The purpose of this quantitative, quasi-experimental quality improvement project was to determine if or to what degree the implementation of the CDC STEADI Algorithm would impact physical therapy referrals when compared to current practice among adults aged 65 or older in a primary care clinic in urban Florida over four weeks.
This chapter provides a statement of the problem and clinical question guiding this project, followed by a discussion of the project’s methodology and design. Next, the project’s population and sample selection are discussed, followed by instrumentation or sources of data, including validity and reliability. Next, the data collection and analysis procedures are presented, including potential bias, mitigation, and ethical considerations. Following a discussion of project limitations is a chapter summary, concluding with an overview of Chapter 4.
Statement of the Problem
Falls are a common problem among elderly individuals both in the hospital and at home. The significance of the problem is often underestimated, as elderly individuals do not always report their falls to their doctors and consider these accidents insignificant. Addressing the problem of falls in elderly patients through effective risk screening in clinical settings could potentially reduce the risks, but effective practices and protocols are necessary.
It was not known if or to what degree the implementation of the CDC STEADI Algorithm would impact physical therapy referrals when compared to current practice among adults aged 65 and older. The implementation of the CDC STEADI initiative in a healthcare setting requires continuing education of health professionals and additional resources for making fall prevention a part of clinical practice. Therefore, before changing protocols and operations, the efficiency of the STEADI program should be assessed and compared to the existing clinical practice. The measurement and assessment of the patient outcomes and updated clinical practices were important to evaluate the effectiveness and appropriateness of the chosen intervention in the clinical setting at the project site.
Clinical Question
To demonstrate the statistically significant relationship between the intervention and practice improvement, this project collected numerical data on the intervention implementation and patient outcomes to assess if there was a statistically significant relationship between the intervention and practice improvement. This project sought to determine if fall risk screening with an evidence-based tool resulted in increased referrals to physical therapists and other specialists to address detected impairments and reduce the risks and fall incidence. The effectiveness of using the CDC STEADI algorithm for risk screening was measured by identifying fall risks and related physical therapy referrals in the implementation group compared to the comparative group not receiving the intervention. The following clinical question guided this project:
To what degree did the implementation of the CDC STEADI ALGORITHM FOR FALL RISK SCREENING, ASSESSMENT, AND INTERVENTION impact physical therapy referrals when compared to current practice among adults aged 65 and older in a primary care clinic in urban Florida?
Compared to implementing the CDC STEADI algorithm, current practice at the project site does not include the risk assessment of falls in patients using an evidence-based tool or education on fall prevention for healthcare professionals or patients. The independent variable in this project was the clinical practice change in the form of the CDC STEADI algorithm implementation, and the dependent variables were the identification of fall risk rates and physical therapy referral rates after the STEADI algorithm was put into practice. The number of physical therapy referrals was measured for the implementation group over four weeks and compared with baseline data from the comparative group also collected over four weeks. The data were retrieved from the EHR. The post-intervention number of fall risk assessments and physical therapy referrals represented the outcome variable, the dependent variable, with nominal level of measurement. The impact of the independent variable, the CDC STEADI algorithm, on the dependent variable allowed this project to evaluate the viability of the project and its possible limitations.
The data were collected between groups from the EHR for comparative data evaluation between the comparative and implementation groups to determine the impact of the intervention. The choice of this approach to collecting data was explained earlier in Chapter 1 with the project’s convenience sampling to examine the risks of experiencing falls in two different groups, the comparative group pre-intervention and the implementation group post-intervention. Thus, opportunities for minimizing costs were pursued associated with the data collection process while also avoiding biases in data collection.
Project Methodology
The quantitative research method was used in this project to collect measurable data and establish a causal relationship between the independent and dependent variables. The choice of the quantitative method was best considering the nature of the variable tested, physical therapy referrals could be quantified. The project sought to establish a causal relationship, requiring statistical analysis and measurable variables. The emphasis of this project was placed on measurement and establishing a relationship through variables, as Pope and Mays (2020) had suggested. The clinical question required numeric data to answer it for statistical analysis. The project used deduction, first conducting a preliminary literature search, selecting the existing theoretical framework, and further testing its applicability in the real-life hospital setting (Zhao et al., 2021). By contrast, the qualitative research method implies the use of induction, in which the analysis starts by collecting data and only later looks for the appropriate theoretical explanations, which could potentially justify the collected evidence.
Quantitative research uses deductive reasoning and generalization. Therefore, an established tool of the STEADI algorithm was used to analyze the process of fall prevention, its effectiveness and its outcomes. The concepts were refined into dependent and independent variables, and then evidence was collected to conclude whether the STEADI algorithm was effective and supported the given clinical question and setting (Frieson et al., 2018). Next, generalizations were to be made to conclude to what extent the findings of this project could be applied to a larger population.
Project Design
A quasi-experimental design was chosen for this project. The project did not include randomization of participants and used only a limited sample size. The project was implemented in a partially controlled setting, in which the practice change was initiated, but some other criteria, such as patients’ and providers’ engagement and setting schedules, could not be manipulated. Whereas this quasi-experimental design was useful for testing the effectiveness of the STEADI algorithm as a fall prevention method, the lack of randomization of participants increased the risks of exposure to a greater number of internal and external validity threats (Rockers et al., 2017).
The relationship between an independent (predictor) variable, the implementation of the STEADI algorithm, and the dependent (outcome) variable, the physical therapy referral rates, expressed in numbers post-intervention, were quantified and further evaluated in order to conclude whether the project was statistically significant. The causal relationship between the intervention and the number of reported fall risk assessments and physical therapy referrals among participants could be established through the quasi-experimental design, but the collected evidence and its analysis could be insufficient for making generalizations. Furthermore, the STEADI method was recommended by the CDC, and thus, there was evidence that the approach was beneficial and assigning a control group not using this method would be unethical. Instead, the participants and their data before the intervention were their own convenience sample.
Pre-implementation data for the comparative group were collected from the project site EHR to evaluate the number of physical therapy referrals generated over four week before the intervention (Durgun et al., 2021; Khairat et al., 2018). The three stages of the STEADI approach included screening, assessment of risks, and further intervening to decrease them with referrals for related therapy. The data on the main risk factors, including poor gait, strength and balance, vitamin D deficiency, visual impairment, and other comorbidities, were collected from EHR (Alabdullgader, & Rabbani, (2021). The key measurable outcome was the change in the number of referrals for falls in elderly patients, which, in turn, should signify reduced instances of falls among the target population members.
Population and Sample Selection
This project was conducted in an outpatient clinic in urban Florida. The total population of this clinic is estimated at 4,000 patients. The clinic size and convenience are the main factors explaining the choice of a non-randomized convenience sample, which is associated with several limitations, but which could be applied in this setting. The total population of the clinic is comprised of middle-class urban citizens living in the clinic neighborhood. The total clinic population includes diverse ethnic and age groups, including non-Hispanic Whites, Hispanic citizens, and African American citizens as the most substantial groups. The older patients aged 65 or older comprise over 40% of the total clinic population
This project included N = 274 patients, n = 121 in the comparative group and n = 153 in the implementation group, and descriptive data were collected for the implementation and control group participants to include age, gender, ethnicity, and highest educational levels. The only inclusion criteria used for this project’s sample were the participants’ age and risks of falls (Dhalwani et al., 2017; Meyer et al., 2019). Whereas convenience sampling was used for this project and is considered non-representative, by including all elderly patients who were available for the project, without excluding individuals based on comorbidities, this project presented results that could be further generalized for a larger population.
Based on the power analysis of this sample, it could be concluded that the sample size of at least 150 participants allowed for the 65 and older population of the clinic. The use of a non-randomized sample was an essential limitation of the project, associated with restricted opportunities for generalizations. The specified approach helped minimize the resources and, thus, reduce costs while offering accurate information in the analysis output.
This project included N = 274 patients, n = 121 in the comparative group, and n = 153 in the implementation group, both males and females, aged 65 or older. The only inclusion criterion used for this sample was the participants’ age and risks of falls. The participants were not excluded based on their past experiences of falls, mental conditions, substance abuse status, or impairment of the musculoskeletal system, which could increase the risks of falls. Exclusion criteria were any adult patients younger than 65 years. Based on a power analysis of this sample, it could be concluded that the sample size of at least 150 participants allowed for making assumptions concerning the efficacy of the suggested measure for the 65 and older population of this clinic, but not all the elderly population of the country in general.
Instrumentation or Sources of Data
The data collected for this project included data on the number of physical therapy referrals before the intervention for the comparative group, retrieved from EHR, and data on post-intervention fall risk assessments and physical therapy referrals for the implementation groups. Demographic data and health history of the participants were collected from EHR for analysis and assessment of the risks of falls. Additionally, risk assessment required physical exams and balance tests, including a 30-second chair stand test and four-stage balance test (CDC, 2019).
For ascertaining stages of risk assessment, practitioners needed to fill in the CDC STEADI risk factors form. This form, complimented by physical balance tests, allowed for evaluating the risks of falls in patients and further referring them to specialists who could help reduce their risks through therapy by addressing their weak points. The detected problems with gait and balance required physical rehabilitation, which would improve the patient’s physical fitness and reduce the risks of falls.
Fall risk patient referral was a questionnaire that specified the patient’s deficits and recommended therapies. This documentation should be used to assess the number of prevention strategies implemented in the program. These questionnaires were an important source of information to be input into the HER regarding the stages for risk assessment evaluation and the outcome of physical therapy referrals.
A major outcome of this project was evaluated though counting the number of patient referrals for physical therapy after the STEADI intervention was implemented compared to the number of physical therapy referrals reported in the comparative group. The independent variable was the STEADI algorithm for fall risk screening for adult patients aged 65 and older. The dependent variable was represented by the number of physical therapy referrals.
Validity
The internal validity, referring to the integrity of experimental design, assesses whether the intervention had an impact on the outcome or not. In terms of internal validity, this project collected substantial data on the quality of risk assessment and related referrals, which allowed for determining whether the intervention played any role in changing the outcome. Factors threatening the internal validity included factors other than the project intervention that could influence the independent variables. Potential internal validity threats in this project included mortality and response bias, when patients might be unwilling to share the information on falls or fall-related risk factors or forgetful about instances, considering falls or impairments increasing their risks of falls as insignificant or not worth mentioning (Farelly, 2013).
The external validity is defined by the degree to which the intervention could be generalized and used in other settings (Farelly, 2013). The external validity of this project was restricted due to the non-randomized sampling method and collection of data in only one isolated primary care setting. Therefore, the project had limited potential in terms of external validity because its findings could be applied to non-project patients in the project clinic, but not to other patients outside the clinical setting under analysis.
Methods to control for validity threats in this project included extracting data from the same team of medical providers over four weeks. All providers had identical access to the STEADI algorithm reminder template. The quality improvement was to be increased fall risk screening and prevention measure through physical therapy referrals.
Nithman & Vincenzo (2019) feature the predictive and discriminate validity of the STEADI, considering prospective 6-month falls and retrospective 12-month falls. It has been found that STEADI algorithm has a more pronounced sensitivity than specificity and was effective in “identifying fallers retrospectively (68.6%) and prospectively (68.4%) with the combined cohort” (p. 191). STEADI algorithm was found to predict 80 per cent of future falls as well as was instrumental in determining past falls in 72.7% cases (Nithman & Vincenzo, 2019).
The STEADI fall risk algorithm had demonstrated predictive validity for fall risk among U.S. older adults. The specificity of the STEADI fall risk algorithm was based on differentiating between risk factors specific to fall prediction versus risk factors attributed to poor physical health in general. There was evidence that STEADI fall risk categories had specificity in predicting the risk of falls, independently of mortality, disability, and other health-related risk factors (Lohman et al., 2017). Therefore, the STEADI initiative had been indicated to be valid in measuring what it aims to measure.
Reliability
The reliability of the project refers to its consistency in measurements and data collection (Farelly, 2013). The project uses STEADI algorithm, the reliability of which has been established both statistically and in scientific literature. Statistically, the reliability of STEADI algorithm was measured through the usage of continuous variables presented as meansstandard deviations and percentages. The distribution of demographic and health-related variables across risk groups was measured before the implementation of STEADI algorithm and after that. The statistics showed that out of 100% percent pf people who received STEADI, 85% commented on a significant reduction in falls (Eckstrom, 2017). Furthermore, logistic regression models were applied to calculate the marginal probability of falling in those people who received STEADI support and those who did not. It was found that STEADI algorithm significantly reduced the risk of falling reducing the marginal probability by approximately 37 per cent across all groups of patients (Nithman & Vincenzo, 2019). The reliability of this project’s findings depends on the appropriateness of the conditions and the presence of non-controlled and unpredicted factors and variables. One of the major disadvantages of a project is the inability to control and predict every variable. Unlike lab experiments, quasi-experimental projects in real-life settings are associated with continuous changes and unpredictable circumstances, interfering with data reliability. For example, one of the important factors interfering with the project outcomes was the patient’s failure to report a fall, considering it insignificant but seriously affecting the data necessary to measure outcomes.
The chi-square test of independence could be applied for evaluating distributions in data (Shi, 2018). This test is robust and informative, and it offers detailed information to be extracted from this statistical method. The strength of association is evaluated on a scale of 0 to 1, with values closer to 0 signaling weak association and values closer to 1 signaling stronger association (Shi, 2018).
Data Collection Procedures
This project started from an educational presentation to providers on the importance of fall risk screening and the main principles of the STEADI algorithm, conducted during lunchtime. In the next step, within four weeks, patients age 65 and older visiting the healthcare facility for any health concerns at the project site were screened for fall risks using the STEADI algorithm. Next, patients screened for risks of falls were recommended for physical therapy according to the risk level identified and the most appropriate intervention for decreasing those risks (Chang et al., 2019). High and moderate-risk patients were referred to physical therapists to address their risk factors whenever appropriate. Next, baseline measurements will be completed for the patients who have been screened with the STEADI tool. The information on pre-intervention falls is retrieved from EHRs. After the risks are assessed, the high-risk patients are referred to specialists and therapists who could address their deficits and reduce the risks (Cho & Jang, 2020). Pseudonymization was used for all data to prevent bias in the stages of data analysis (Bruland et al., 2018).
After GCU IRB approval, this project began by educating the clinical staff on how to use the STEADI algorithm to identify high fall risk patients and how to use the algorithm to refer patients to the appropriate discipline using the algorithm. Data will be collected from the EHR the 4 weeks prior to the project start date on identified high fall risk patients and referral rates. During the project implementation periods, fall risk will be identified using the STEADI algorithm and referral rates will be tracked. Whenever appropriate, high, and moderate-risk patients will be referred to physical therapists for addressing the risk factors. After 4 weeks of project implementation, referral rates will be extracted from the EHR. Demographics will also be collected from the EHR and include age, gender, race, ethnicity, history of falls, and primary diagnosis. The data will be stored on a password protected computer at the project site. Data will be destroyed 12 months after project completion
Data Analysis Procedures
To arrive at a reliable conclusion on the influence of STEADI algorithm on physical therapy referrals, the clinical question has been formed. The clinical question is: To what degree did the implementation of the CDC STEADI ALGORITHM FOR FALL RISK SCREENING, ASSESSMENT, AND INTERVENTION impact physical therapy referrals when compared to current practice among adults aged 65 and older in a primary care clinic in urban Florida? A chi-square test was conducted to compare the physical therapy referral rates in the comparative and implementation groups to address the project’s clinical questions
After four weeks of project implementation, data extraction was carried out with a chart review. The results were collected and numerically coded within an Excel Spreadsheet ordered, and visualized through graphs and tables using Microsoft Excel software. Data for physical therapy referrals were collected for the comparative and implementation and compared in IBM SPSS version 28 using chi-square tests. A chi-square test was the most appropriate type of test for this project because it certified for identifying statistically significant differences in the groups (comparative and implementation). The p-value was less than.05, indicating the increase in the referral rate was statistically significant. Clinical significance was supported by substantial increases in referrals for those identified with a fall risk.
The data was stored on my personal laptop, protected with logins and passwords. After the project was completed, the data would be deleted from the hard drive per site policy. This project used the chi-square test to analyze the collected data and detect the relationships between the intervention’s independent variable, the STEADI algorithm, and the dependent variables of physical therapy referral outcomes (Farrelly, 2013).
Potential Bias and Mitigation
The use of a convenience sampling method could be a source of bias and a threat to the internal validity of the project (Hopewell et al., 2018). Despite the restricted opportunities for generalizations, the nonrandomized sampling method was used for convenience sampling and due to the limitations of a single setting used, this method could be associated with a number of restrictions, such as the inability to generalize findings to other locations or the general population (Shi, 2018). Another important limitation and source of bias could be sampling response bias. The incidents of falls could be underreported by the patients, who might consider them insignificant or feel fearful of practitioners’ criticism. Specifically, the choice of wording for a particular question in the questionnaire might cause the participants to respond in a certain way. Moreover, the practitioners’ reports might lack information on falls due to the lack of time necessary for filling the reports or technical errors. Despite major limitations of a non-randomized sample and the possible failure of some patients to report their falls, this project was expected to answer the clinical question inquiring if or To what degree did the implementation of the CDC STEADI Algorithm for Fall Risk Screening, Assessment, and Intervention impact physical therapy referrals when compared to current practice among adults aged 65 and older in a primary care clinic in urban Florida?
Ethical Considerations
The evaluation of potential ethical issues in this project required considering levels of risk, coercion, and rationale of the project. The project complied with the core principles of the Belmont Report, including those of respect, justice, and beneficence, implemented to not cause any harm to patients or healthcare practitioners emphasizing the importance of fairness and trust, and respecting the rights of all the participants (Hopewell et al., 2018). All personal identifiers were coded with numbers, which also reduced possible bias.
Due to ethical considerations and the fact that the effectiveness of the STEADI algorithm had been indicated in previous projects, it would be unethical to have a control group, for which fall prevention was not used (Gomez et al., 2017). For this reason, subjects from the main group are their own convenience sample. Additionally, some of the risk assessment procedures could lead to patient’s discomfort and stress.
The principles of ethical research practices and ethical protections were of paramount importance in this quality improvement project. There were no added risks associated with project participation. All personal health identifiers, variables, and project data were coded, protected, and shared only with me who are involved in the project implementation and data analysis.
Limitations
This project was guided by the quantitative methodology with a quasi-experimental design. Threats to internal and external validity could cause possible limitations because the data were collected in only one primary care site by a limited number of medical care providers who voluntarily agreed to participate in the project. Other limitations included the failure to randomize the project sample and the selection of a pretest-posttest design. The project also could be weakened by the lack of a control group, as the patients were selected by convenience sampling.
Major limitations of this project were the use of a convenience sample, a relatively small sampling size, and the patients’ failure to report their falls. The use of a convenience sample, lack of randomization, and sample size were discussed above and were dictated mainly by the outpatient clinic size and type of clinical question. The project had only limited potential for generalization of the project findings to a broader patient population.
Summary
This chapter presented a detailed discussion of the project’s quality improvement, data collection, and analysis methods. The method chosen for this project was a quantitative quasi-experimental design. The project needs and convenience explained the choice. The numbers and statistical data were used for establishing whether statistically significant relationships existed between the intervention and project outcomes, that is the application of the STEADI algorithm and increased fall risk assessments and physical therapy referrals. Due to the limited size of the healthcare facility, only a limited number of patients aged 65 and older could be invited to participate in this practice improvement. However, manipulation of variables was restricted, and the occurrence of additional variables, such as unwillingness to report falls, interfering with the project outcomes was possible. The data were retrieved before the intervention from the facility EHR for the comparative group and again post-intervention for the implementation group. A chi-square test was used to analyze data and detect relationships between the dependent and independent variables. Despite major limitations of a non-randomized sample and the possible failure of some patients to report their falls, this project was expected to answer the clinical question inquiring if or To what degree did the implementation of the CDC STEADI Algorithm for Fall Risk Screening, Assessment, and Intervention impact physical therapy referrals when compared to current practice among adults aged 65 and older in a primary care clinic in urban Florida?
Chapter 4 presents the project’s data analysis and results. The chapter also includes descriptive data capturing the project’s sample demographic data in narrative and chart form. After presenting the project’s descriptive data, Chapter 4 discusses the project’s data analysis procedures, followed by results. The chapter concludes with a summary of the results based on the project’s finding and an overview of Chapter 5.
Data Analysis and Results
Patient falls remain an important healthcare concern for many healthcare sites, particularly primary care settings. The risks of falls are high for outpatient primary care clinics because healthcare professionals cannot control the lifestyle and environmental factors of the patients outside of the clinic (Patterson et al., 2019). The outpatient primary care clinic in Florida was chosen as a setting for this project because the project site might benefit from implementing the CDC STEADI Algorithm for Fall Risk Screening, Assessment, and Intervention to increase physical therapy referrals. It was not known if or to what degree the implementation of the CDC Stopping Elderly Accidents, Deaths and Injuries (STEADI) algorithm would impact physical therapy referrals when compared to current practice among adults aged 65 and older.
This quality improvement project used a quantitative methodology with a quasi-experimental design. The purpose of this quantitative, quasi-experimental quality improvement project was to determine if or to what degree the implementation of the CDC STEADI Algorithm for Fall Risk Screening, Assessment, and Intervention would impact the number of physical therapy referrals when compared to current practice among adults aged 65 or older in a primary care clinic in Florida over four weeks. The CDC STEADI algorithm was the independent variable, defined as the STEADI Algorithm for Fall Risk Screening, Assessment, and Intervention. The dependent variable was the physical therapy referrals in the control and implementation groups. The chi-square test was used to determine if an association existed between the implementation of the CDC STEADI algorithm and subsequent physical therapy referrals.
This chapter thoroughly reviews the project’s data analysis and results. The chapter also includes a presentation of the descriptive data for the project sample in both narrative and chart form. Following the descriptive data is a discussion of the project’s data analysis procedures and results. The chapter ends with a summary of the project findings and an overview of Chapter 5.
Descriptive Data
The target population selected for this project involved patients aged 65 and older in an urban primary care clinic in urban Florida. The sample population was chosen because the risks for falls were significantly higher during their clinic visits and immediately post-discharge. The primary care clinic at the project site provides family care, including care for chronic conditions, which are more common in adults aged 65 or older. The comorbidities and resulting polypharmacy might have adverse effects on patient balance and risks of falls in the older patient population (Vincenzo et al., 2020). All patients who visited the primary care facility were included in the project based on the main inclusion criterion of age 65 and older. Descriptive data were significant to this project to detect patterns in the demographic characteristics and the levels of fall risks. While some ethnic and gender groups were at higher risk of developing certain chronic conditions, affecting the fall risk assessment (such as higher risks of osteoporosis in postmenopausal women of Hispanic origin), demographic data were essential for this sample. The educational level was essential for defining the background, whereas living alone or with family and partners status was significant for assessing the assistance availability and social support level.
This project included N = 274 patients, n = 121 in the comparative group and n = 153 in the implementation group. Descriptive data were collected for the project participants. Gender, race, marital status, highest educational levels, and chronic condition are documented in Table 1. All participants were aged 65 years and older. In the comparative group, there were 50 males (41.3%) and 71 females (58.7%). The race was 74.4 Hispanic (n = 90), 8.3% African American (n = 10), and 17.4% Caucasian (n = 21). The marital status was never married for 11.6% (n = 14), married for 71.1% (n = 86), and divorced for 1.4% (n = 21). For education, 20 (16.5%) had a Master’s or Doctoral degree, 40 (33.1%) had a bachelor’s degree and 61 (50.4%) had education listed as “other”. There were 44 (36.4%) with no chronic conditions and 77 (63.6%) with a chronic condition. The risk score was < 4 for 43.8% (n = 53) and > 4 for 56.2% (n = 68).
In the implementation group, there were 89 female participants (58.2%) and 64 male participants (41.8%). The majority of the participants were Hispanic (n = 114, 74.5%), while there were 23 Caucasian participants (15.0%) and 16 African American participants (10.5%). Among the 153 participants, 104 participants were married (68%), 16 participants never married (10.5%), and 33 participants divorced (21.6%). For the education of participants, there were 35 participants who had completed a bachelor’s degree (22.9%), while there were 30 participants who had completed a master’s or a doctoral degree (19.6%). About 66% of the participants had chronic conditions (n = 101), and 71.2% had a fall score of above four at pre-intervention in the comparative group (n = 109). There were also 85 participants who were referred for physical therapy and had a fall score of above four at post-intervention in the implementation group (55.6%).
Table 1: Demographic Characteristics of Project Sample
Data Analysis Procedures
The data collection for this project addressed the following clinical question: To what degree did the implementation of the CDC STEADI ALGORITHM FOR FALL RISK SCREENING, ASSESSMENT, AND INTERVENTION impact physical therapy referrals when compared to current practice among adults aged 65 and older in a primary care clinic in urban Florida? The CDC STEADI algorithm was the independent variable, defined as the STEADI ALGORITHM FOR FALL RISK SCREENING, ASSESSMENT, AND INTERVENTION. The dependent variables were physical therapy referrals compared between the comparative and implementation groups.
The project’s intervention included routine fall risk screening using the STEADI algorithm and referrals to fall prevention programs comprised of muscle strengthening and balance challenging training exercises and home-based exercise programs assigned based on the fall risk assessment. The next stage of data collection included measuring the physical therapy referrals in adults who completed the screening in the implementation group, compared to the number of referrals over four weeks for the control group when the STEADI algorithm was not applied. The second variable of the number of physical therapy referrals also was compared between the comparative and implementation groups.
The project investigator was responsible for data extraction and analysis. An Excel spreadsheet was used for creating a retrospective chart analysis to compare the number of fall risks identified and physical therapy referrals generated over four weeks for the comparative group compared to the implementation group that received the STEADI algorithm. The patient identification data was not collected within the Excel spreadsheet. Instead, numerical codes were used to secure anonymity and protect patient confidentiality.
The reliability and validity of the quantitative methodology were achieved through the use of the STEADI algorithm. The STEADI algorithm has been used in hospitals to measure fall risk. Therefore, the measure has been pre-validated and has been tested for reliability. The data were analyzed using the chi-square test of independence. The Chi-square test could be applied to analyze distributions in data, and its advantage is that the test does not require equality of variances in compared groups, which was the case in this project. The Chi-square test could be applied to handle data sets when parametric assumptions could not be conveyed (Farrelly, 2013).
Considering that the project was comparing two dissimilar groups of charts before and after the intervention, the project required a sufficient population sample to improve the internal validity of the project. The p-value, related to the level of significance, was set at 5% or p<.05. If the p-value was lower or equal to the set significance level, the conclusion would be that the collected data was inconsistent, and the null hypothesis would be rejected (Melnyk & Fineout-Overholt, 2015). A p-value lower than the chosen significance level would indicate a strong association between the planned intervention and the anticipated outcome. Higher power, effect, and sample size would be associated with increased internal validity of the project.
Potential sources of error limiting the validity and reliability of the project included the doctors’ not using the screening tool accurately. In 9% of cases, the screening tool was completed with the answers ‘NO’ to all questions, but the patient was referred to a physical training program. This inconsistency could have had a negative impact on the project’s internal validity, overestimating the association between screening and project outcome variable.
Results
Measuring the application of CDC STEADI ALGORITHM FOR FALL RISK SCREENING, ASSESSMENT, AND INTERVENTION across groups that vary in term of race, gender, and education allowed to arrive at credible results. The results of this project addressed the following clinical question: To what degree did the implementation of the CDC STEADI ALGORITHM FOR FALL RISK SCREENING, ASSESSMENT, AND INTERVENTION impact referral rates when compared to current practice among adults aged 65 and older in a primary care clinic in urban Florida? There was an increase in the referral rate from 69.1% in the comparative group (n = 47 out of 68) to 95.3% in the implementation group (n = 101 out of 106), X2 (1, N = 174) = 22.31, p =.001. The p-value was less than.05, indicating the increase in the referral rate was statistically significant. Clinical significance was supported by the 26.2% increase in referrals for those identified with a fall risk. The results are displayed in Table 2.
Table 2: Chi-Square Results for Referral Rates
Summary statistics (n, %) were computed physical therapy referrals. Figure 1 displays the identification and referral rates in the comparative and implementation groups. The physical therapy referral rate was 69.1% in the comparative group and increased to 95.3% in the implementation group.
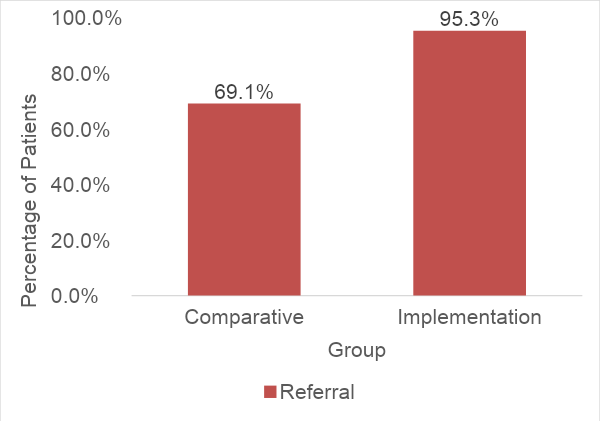
Data analysis was conducted on physical therapy referrals in the comparative and implementation groups of patients. The chi-square test results for physical therapy referrals showed p-values of less than.05, indicating the differences were statistically significant. The results also supported the clinical significance of the project as reflected in increases in physical therapy referral outcomes after the STEADI algorithm intervention.
Summary
This project aimed to evaluate the impact of the intervention (STEADI algorithm) on the outcome variable (physical therapy referrals). The project included N = 274 patients (n = 121 in the comparative group and n = 153 in the implementation group). Descriptive data were collected for the project participants. The gender, race, marital status, highest educational levels, chronic condition, and risk score categories are documented in Table 1. All participants were aged 65 years and older. In the control group, there were 50 males (41.3%) and 71 females (58.7%). The race was 74.4% Hispanic (n = 90), 8.3% African American (n = 10), and 17.4% Caucasian (n = 21). In the implementation group, there were 89 female participants (58.2%) and 64 male participants (41.8%). The majority of the participants were Hispanic (n = 114, 74.5%), while there were 23 Caucasian participants (15.0%) and 16 African American participants (10.5%).
Data for physical therapy referrals were collected for the comparative and implementation patients and compared in IBM SPSS version 28 using chi-square tests. Additionally, there was an increase in the referral rate from 69.1% in the comparative group (n = 47 out of 68) to 95.3% in the implementation group (n = 101 out of 106), X2 (1, N = 174) = 22.31, p =.001. The p-value was less than.05, indicating the increase in the referral rate was statistically significant. Clinical significance was supported by substantial increases in referrals for those identified with a fall risk.
Chapter 5 discusses a summary of the findings and conclusions based on the data analysis. The implications of the collected data, including clinical, practical and future implications of the findings, are outlined. Additionally, future recommendations for improved research validity and generalizability to be achieved in future projects are included with recommendations for practice.
Summary, Conclusions, and Recommendations
Patient falls are an important health care concern, associated with essential risks of injuries or even deaths, reduced QOL, and substantial healthcare expenses (Durgun et al., 2021; Shahrbanian et al., 2021). The risks are higher in older individuals due to age-related changes in different systems, prevalent chronic conditions, and polypharmacy (Dhalwani et al., 2017). According to the CDC report (2019), at least one out of four individuals aged 65 or older experiences at least one fall per year. In some cases, patients underestimate the significance of the incident and fail to report it to their health care provider. However, falls could have significant negative consequences, potentially leading to long-term negative effects on health. Furthermore, the risks of patient falls are even higher outside of the project site clinic when the patients are out of provider control and have no professional support from the medical staff, and the environment could be less safe than it should be. The current practice in the chosen outpatient clinic in Florida lacks effective patient screening on the risks of falls. However, there is evidence that effective physical therapy referrals for reducing fall risks could be effective in preventing patient falls and their consequences (Khairat et al., 2018).
The evidence demonstrates that the CDC STEADI algorithm, consisting of referrals to physical therapists to reduce the risks, could be effective for reducing the incidence of patient falls and related consequences (Casey et al., 2017). Routine screening of patients aged 65 or older could be helpful for identifying high-risk patients and their deficits, which might be compensated through patient referrals to physical training programs to improve muscle strength and balance (Johnston et al., 2018). The literature review that was conducted for this project identified the need for fall prevention and the effectiveness of fall prevention programs (Mark & Loomis, 2017). However, the current practice in the chosen outpatient clinic in Florida did not include screening for fall risks in patients aged 65 or older as a part of routine interviews; therefore, before this project, the project site did not use the opportunities available for fall risk reduction.
The purpose of this quantitative, quasi-experimental quality improvement project was to determine if or to what degree the implementation of the CDC STEADI Algorithm for Fall Risk Screening, Assessment, and Intervention would impact the number of physical therapy referrals when compared to current practice among adults aged 65 or older in a primary care clinic in urban Florida over four weeks. Identifying elderly patients at increased risk of falls could facilitate timely referrals to physical therapists and other strategies used for fall risk reduction and prevention (Dhalwani et al., 2017). Current research supports that referring elderly patients at increased risk of falls to physical therapists and encouraging them to continue exercising at home could help advance timely fall risk recognition, prompt therapeutic intervention, and reduce the incidence of falls, decreasing mortality and morbidity related to outpatient falls (Frith, 2017; Lyons & Hall, 2016). This project implemented the CDC STEADI Algorithm for Fall Risk Screening, Assessment, and Intervention and encouraged medical practitioners to use it as part of a patient’s routine visit. Therefore, even if a patient denied the fear of falling and/or past experiences of falls, the patient should be screened for fall risks. This project might serve as a foundation for future projects exploring evidence-based fall risk reduction opportunities and subsequent prevention of fall-related morbidity and mortality. Future prospects should consider implementing evidence-based fall risk assessment toolkits, like the STEADI or its alternatives, in a primary care clinic to improve patient health outcomes in patients with moderate to high risk for falls, particularly patients aged 65 and older.
Summary of the Project
This project used a quantitative methodology with a quasi-experimental design to assess if or to what degree the implementation of the CDC STEADI Algorithm would impact physical therapy referrals when compared to current practice among adults aged 65 and older. This multi-modal interventional approach was selected because it suited the requirements of a quality-based improvement project among primary care providers.
This chapter summarizes the project findings and conclusions, discusses future clinical and practical implications, and includes a retrospective review of the framework used to guide the project. Next, a critical evaluation of the strengths and limitations of the project is included, along with the analysis of credibility and internal validity of the chosen methodology, project design, and collected data. Finally, the chapter discusses the applicability of the project findings to current primary care practice, addressing the needs of patients aged 65 or older.
Summary of Findings and Conclusion
The current project found that implementing the CDC STEADI algorithm was effective at increasing referrals for physical therapy in Florida among patients aged 65 years or older. This quantitative, quasi-experimental quality improvement project addressed a significant gap in the literature. Few studies in the literature had examined the effectiveness of implementing evidence-based standards of care that address the fall risk of adults 65 years or older (Eckstrom et al., 2017). The findings of the current project could be utilized by practitioners to inform their standard of care when working with adults aged 65 years or older. There was an increase in the referral rate from 69.1% in the comparative group (n = 47 out of 68) to 95.3% in the implementation group (n = 101 out of 106), X2 (1, N = 174) = 22.31, p =.001.
Consistent with previous literature, the current project established the impact of CDC STEADI ALGORITHM FOR FALL RISK SCREENING, ASSESSMNET, AND INTERVENTION on physical therapy referrals when compared to current practice among adults aged 65 and older in a primary care clinic in urban Florida. Previous studies had provided evidence that incorporating the CDC STEADI algorithm into a clinic practice reduced the number of patient falls (Mark & Loomis, 2017; Wongrakpanich et al., 2019). Previous studies also indicated that reducing patient falls with evidence-based tools like the CDC STEADI tool resulted in reduced injury from falls (Johnston et al., 2018; Lohman et al., 2017). Implementing the CDC STEADI algorithm also should not create an additional screening burden on clinics (Casey et al., 2017). The current project expanded on the current literature by examining the effectiveness of the STEADI algorithm in a population not yet examined in the current literature. Additionally, the current project contributes further evidence that the CDC STEADI algorithm could be incorporated into standards of care to help prevent falls among adults 65 years and older.
Implementing interventions to reduce falls among adults aged 65 years and older is an important addition to a clinic’s standard of care. Falls are dangerous experiences for older adults and could cause severe injury and significantly reduce QOL (Durgun et al., 2021; Shahrbanian et al., 2021). Outpatient clinics are ideal for implementing fall prevention efforts as they see a greater number of older adults (Urban et al., 2020). The current project was supported by previous literature and contributed to a body of evidence that an effective intervention could be incorporated into a clinic’s standard of care without significant cost. Medical practitioners who work with adults aged 65 years or older should consider incorporating the CDC STEADI algorithm into their standard of practice.
This project expanded upon the current literature by examining how demographics, including gender, race, marital status, education, and chronic illness, were associated with fall risk pre- and post-implementation of the CDC STEADI algorithm. Although previous research on the CDC STEADI algorithm had not examined how demographics were associated with fall risk after the implementation of the algorithm, demographics had previously been found to be associated with fall risk (Mota de Sousa et al., 2017). After implementing the STEADI algorithm, race, marital status, education, and chronic illness were associated with fall risk. It has been found that less educated people are more fall-prone, and these are females that tend to fall more than men. Chronic illnesses make people more susceptible to falls, and should be treated to alleviate this factor. The implementation of STEADI allows to significantly reduce the risks of falls among all population groups, notwithstanding their race, gender, and the level of education. Understanding how these demographics are associated with fall risk could provide practitioners with information on groups of patients who might need additional intervention or follow-up appointments to address fall risk.
Gender previously was identified as associated with fall risk (Mota de Sousa et al., 2017); however, the current project found that after implementing the STEADI algorithm, gender was no longer associated with fall risk. As such, it appears that the implementation of the STEADI algorithm was effective in addressing the risks of falls. Future research might consider examining how the STEADI algorithm reduces the impact of gender on fall risk.
This project found that fall risk differed by race, marital status, education, and chronic illness after implementing the CDC STEADI algorithm. The project did not identify which groups were at significantly higher fall risk post-intervention. Future research might consider examining these differences in further detail. Identifying which groups of patients are at higher risk of a fall post-STEADI intervention could provide practitioners with information on patients who might need additional intervention beyond the STEADI algorithm to reduce their fall risk.
Implications
In the following sections, implications for the findings of the current project are discussed. Theoretical implications explore the findings within the context of the current literature. Practical implications include how the findings of the current project might influence how professionals work. Future implications examine information the profession needs to consider for future improved understanding of the phenomenon of interest, in this case, fall risk identification and prevention in adults aged 65 and older.
Theoretical Implications
The findings of this project are consistent with the project’s theoretical framework, Orem’s (1985) self-care theory, and Kotter’s 8-step model for change. Orem (1985) asserts that increasing individual knowledge could reduce self-care deficits and improve individual well-being. Screening for adults’ fall risk using the STEADI algorithm increases the individual’s knowledge regarding their physical health and provides opportunities for physical therapy interventions. This increased knowledge and opportunity for self-care through physical therapy improved adults’ well-being by reducing their fall risks.
Besides Orem’s self-care theory, Kotter’s 8-step model for change also provided the theoretical underpinnings supporting this project. Kotter’s 8-Step model could provide a template for incremental change in the healthcare setting. By following Kotter, (1995) 8-step change model, it was possible to promote consistency in decision-making related to the risk of a fall in the elderly and prevent a recurrence with the help of recommending physical therapy. As mentioned earlier, the steps given were consistent and patterned for most cases associated with the problem. It also allowed for acquiring the necessary skills when working with the elderly. By conducting a thorough analysis with the patient, it was possible to learn how to interpret the STEADI algorithm data correctly and develop communication skills through communication with the affected person. The increased communication and fall education using the STEADI algorithm allowed caregivers at the project site to strengthen existing fall risk assessment knowledge and gain a new understanding of the area.
Practical Implications
The findings of this project provide additional evidence for the standard use of the STEADI algorithm in outpatient clinics. The project contributes to previous research on fall risk identification and prevention (e.g., Sarmiento & Lee, 2017; Vincenzo & Patton, 2019; Urban et al., 2020). Supported by previous research, this project found that the STEADI algorithm was effective for reducing fall risk among adults aged 65 years and older (e.g., Sarmiento & Lee, 2017; Vincenzo & Patton, 2019; Urban et al., 2020). Additionally, implementing the STEADI algorithm did not increase medical providers’ work or resource burden (Casey et al., 2017; Eckstrom et al., 2017). Practitioners working in outpatient clinics with adults aged 65 years and older should consider implementing the STEADI algorithm to reduce the fall risk among their patients, particularly older patients.
Future Implications
This project provided evidence that the CDC STEADI algorithm was effective in reducing the fall risk for adults aged 65 years and older. However, the project did not compare the relative effectiveness of the STEADI algorithm to other screening tools. Future practice improvement projects could assess the effectiveness of STEADI implementation in various primary care settings or focus on other alternative fall risk screening toolkits, comparing the intervention outcomes with the STEADI tool.
This project’s potential sources of limitations could include inaccuracies in practitioners using the STEADI screening tool, caused by human factors, lack of time, or both. Additional limitations could include the relatively small sample size, only one setting, non-randomized sampling method, short intervention time (four weeks), and potential bias in patients, who might be trying to conceal their balance issues, fall risks or past experiences of falls, fearing to lose their independence. The strengths of the project included the ease of the screening toolkit use, its convenience, and its ability to cover the different most common fall risk factors. The participating doctors admitted that using the screening tool and further referrals to physical therapists did not cause expressed anxiety in patients. Therefore, the potential value of the project’s intervention outweighed the potential risks and did not create any significant hazards for the patients.
Recommendations
Both researchers and practitioners might find the findings of the current project useful for their work. As such, the findings of this project could be utilized to inform future research and practice. In the following sections, recommendations for future research and practice are discussed.
Recommendations for Future Projects
The findings of this project could be used to inform future research. First, the findings of the project provide additional evidence that the STEADI algorithm is an effective fall risk screening tool. However, the project and much of the evidence examining the STEADI algorithm only examine the effectiveness within one outpatient clinic (e.g., Taylor et al., 2019). Future research might consider evaluating the effectiveness of the STEADI algorithm as a screening tool on a larger scale, for example, across a hospital system as a whole or across a county.
Second, the project found that certain demographics were associated with increased fall risk after implementing the STEADI algorithm. However, the current study did not compare groups within those demographics to determine what group was at a higher fall risk. Future research might consider comparing demographic groups regarding their fall risk to ascertain which groups of individuals might need additional follow-ups after the STEADI intervention.
Third, this project and previous studies (e.g., Casey et al., 2017) examined the STEADI algorithm and the change in fall risk over the short term (e.g., one month) after implementing the program. However, there is some evidence that adults are not following the STEADI recommendations six months post-intervention (Vincenzo & Patton, 2019). Future research might consider examining the effectiveness of the STEADI algorithm long-term (i.e., > six months post-intervention).
Finally, few studies have qualitatively examined the experiences of adults who have been screened using the STEADI algorithm. Understanding an individual’s experience with the screening might provide important information that could be utilized to improve the screening process and reduce adults’ fall risk. For example, some patients might find the screening overwhelming or might struggle to understand some of the information provided. Future research should qualitatively examine the experience of the STEADI algorithm from the perspective of adults aged 65 years or older.
Recommendations for Practice
Based on the findings and conclusions of this project, the STEADI fall risk screening algorithm should be implemented in the primary care setting. The current project was supported by previous research (e.g., Sarmiento & Lee, 2017; Vincenzo & Patton, 2019; Urban et al., 2020). This project contributed to the literature by finding that the STEADI algorithm was effective at reducing fall risk among adults aged 65 years and older (e.g., Sarmiento & Lee, 2017; Vincenzo & Patton, 2019; Urban et al., 2020). Additionally, implementing the STEADI algorithm did not increase medical providers’ work or resource burden (Casey et al., 2017; Eckstrom et al., 2017).
Additionally, the project found evidence of some demographic factors associated with increased fall risk, even when the STEADI algorithm had been implemented. Practitioners might consider developing standards of care in which they follow up with patients who fall into those demographic categories more frequently regarding their fall risk. Alternatively, practitioners might consider more intensive fall risk screening for these individuals. This additional support might reduce the fall risk for adults aged 65 and older who are at the highest risk for falls.
References
Ang, S. G. M., O’Brien, A. P., & Wilson, A. (2019). Understanding carers’ fall concern and their management of fall risk among older people at home. BMC Geriatrics, 19(1), 1-12.
Black, J. M., Salsbury, S., & Vollman, K. M. (2018). Changing the perceptions of a culture of safety for the patient and the caregiver: Integrating improvement initiatives to create sustainable change.Critical Care Nursing Quarterly, 41(3), 226-239. Web.
Bruland, P., Doods, J., Brix, T., Dugas, M., & Storck, M. (2018). Connecting healthcare and clinical research: workflow optimizations through seamless integration of EHR, pseudonymization services and EDC systems.International Journal of Medical Informatics, 119, 103-108. Web.
Burns, E., Kakara, R., Moreland, B., & Henry, A. (2020). Changes in the age-adjusted rate of older adults dying from a fall and reporting a fall and fall injury, 2012–2018.Innovation in Aging, 4(Suppl 1), 774. Web.
Carlucci, C., Kardachi, J., Bradley, S. M., Prager, J., Wyka, K., & Jayasinghe, N. (2018). Evaluation of a community-based program that integrates joyful movement into fall prevention for older adults. Gerontology and Geriatric Medicine, 4, 233372141877678.
Casey, C., Parker, E., Winkler, G., Liu, X., Lambert, G., & Eckstrom, E. (2017). Lessons learned from implementing CDC’s STEADI falls prevention algorithm in primary care.The Gerontologist, 57(4), 787–796. Web.
Centers for Disease Control and Prevention [CDC]. (2017). Checklist. Web.
Centers for Disease Control and Prevention [CDC]. (2019). Algorithm for fall risk screening, assessment, and intervention. Web.
Chang, K. O., Lee, T. J., & Jung, M. Y. (2019). The effect of knowledge, attitude and perceptions of patient safety culture on fall prevention activities in mental hospital nurses.Journal of the Korea Academia-Industrial cooperation Society, 20(5), 372-383. Web.
Cho, M. Y., & Jang, S. J. (2020). Nurses’ knowledge, attitude, and fall prevention practices at South Korean hospitals: A cross-sectional survey.BMC Nursing, 19(1), 1-8. Web.
Creswell, J. W., & Creswell, J. D. (2018). Research design: Qualitative, quantitative, and mixed methods approaches (5th ed.). Sage Publications.
Dhalwani, N. N., Fahami, R., Sathanapally, H., Seidu, S., Davies, M. J., & Khunti, K. (2017). Association between polypharmacy and falls in older adults: A longitudinal study from England.BMJ Open, 7(10), e016358. Web.
Durgun, H., Turan, N., & Kaya, H. (2021). Relationship between fall behavior and quality of life of elderly individuals.Psychology, Health & Medicine, 1-8. Web.
Dykes, P. C., Duckworth, M., Cunningham, S., Dubois, S., Driscoll, M., Feliciano, Z., Ferrazzi, M., Fevrin, F. E., Lyons, S., Lindros, M. E., Monahan, A., Paley, M. M., Jean-Pierre, S., & Scanlan, M. (2017). Pilot testing fall TIPS (tailoring interventions for patient safety): A patient-centered fall prevention toolkit.The Joint Commission Journal on Quality and Patient Safety, 43(8), 403-413. Web.
Eckstrom, E., Parker, E. M., Lambert, G. H., Winkler, G., Dowler, D., & Casey, C. M. (2017). Implementing STEADI in academic primary care to address older adult fall risk.Innovation in aging, 1(2), igx028. Web.
Farrelly, P. (2013). Issues of trustworthiness, validity and reliability.British Journal of School Nursing, 8(3), 149-151. Web.
Ferreira, J. M., Hammerschmidt, K. S. D. A., Siewert, J. S., Alvarez, A. M., Locks, M. O. H., & Heidemann, I. T. S. B. (2019). Gerontotechnology for fall prevention of the elderly with Parkinson.Revista Brasileira de Enfermagem, 72, 243-250. Web.
Frieson, C. W., Tan, M. P., Ory, M. G., & Smith, M. L. (2018). Evidence-based practices to reduce falls and fall-related injuries among older adults.Frontiers in Public Health, 6, 222. Web.
Frith, J. (2017). Fall prevention: Empowering people through online education. The Annals of Family Medicine, 15(5), 482-482. Web.
Gazibara, T., Kurtagic, I., Kisic-Tepavcevic, D., Nurkovic, S., Kovacevic, N., Gazibara, T., & Pekmezovic, T. (2017). Falls, risk factors and fear of falling among persons older than 65 years of age.Psychogeriatrics, 17(4), 215-223. Web.
Gell, N. M., & Patel, K. V. (2019). Rehabilitation services use of older adults according to fall‐risk screening guidelines.Journal of the American Geriatrics Society, 67(1), 100-107. Web.
Gomez, F., Wu, Y., Auais, M., Vafaei, A., & Zunzunegui, M. (2017). A simple algorithm to predict falls in primary care patients aged 65 to 74 years: The international mobility in aging study.Journal of the American Medical Directors Association, 18(9), 774-779. Web.
Haines, T. P., Lee, D. C. A., O’Connell, B., McDermott, F., & Hoffmann, T. (2015). Why do hospitalized older adults take risks that may lead to falls?Health Expectations, 18(2), 233-249. Web.
Hill, A. M., McPhail, S. M., Waldron, N., Etherton-Beer, C., Ingram, K., Flicker, L., & Haines, T. P. (2015). Fall rates in hospital rehabilitation units after individualized patient and staff education programs: A pragmatic, stepped-wedge, cluster-randomized controlled trial.The Lancet, 385(9987), 2592-2599. Web.
Hoffman, G. J., Hays, R. D., Wallace, S. P., Shapiro, M., Yakusheva, O., & Ettner, S. L. (2017). Receipt of caregiving and fall risk in US community-dwelling older adults.Medical Care, 55(4), 371. Web.
Hopewell, S., Adedire, O., Copsey, B. J., Boniface, G. J., Sherrington, C., Clemson, L., & Lamb, S. E. (2018). Multifactorial and multiple component interventions for preventing falls in older people living in the community. Cochrane Database of Systematic Reviews, (7), 1-262. Web.
Jie, E., & Deng, J. (2019). Fall prevention education reduces the falling rate on the osteoporosis patients treated with zoledronic acid.Case Reports in Clinical Medicine, 08(08), 222-230. Web.
Jie, E., & Deng, J. (2019). Fall prevention education reduces the falling rate on the osteoporosis patients treated with Zoledronic acid. Case Reports in Clinical Medicine, 8(8), 222-230.
Johnston, Y. A., Bergen, G., Bauer, M., Parker, E. M., Wentworth, L., McFadden, M., & Garnett, M. (2018). Implementation of the Stopping Elderly Accidents, Deaths, and Injuries Initiative in primary care: An outcome evaluation.The Gerontologist, 59(6), 1-10. Web.
Juckett, L., & Poling, R. (2020). Staff and administrator perceptions of fall risk management in home-and community-based service settings. Innovation in Aging, 4(Suppl 1), 237. Web.
Khairat, S., Coleman, G. C., Russomagno, S., & Gotz, D. (2018). Assessing the status quo of EHR accessibility, usability, and knowledge dissemination. eGEMs, 6(1), 9. Web.
Kelley, R. J. (2022). Preventing Falls in the Surgical Setting by Implementing a Fall Prevention Bundle.
Kiyoshi-Teo, H., Carter, N., & Rose, A. (2017). Fall prevention practice gap analysis: Aiming for targeted improvements. MedSurg Nursing, 26(5), 332-335.
Kotter, J. P. (1995). Leading change: Why transformation efforts fail. Harvard Business Review. Web.
Kuhirunyaratn, P., Prasomrak, P., & Jindawong, B. (2019). Effects of a health education program on fall risk prevention among the urban elderly: A quasi-experimental study.Iranian Journal of Public Health, 48(1), 103-111. Web.
Lee, R. (2017). The CDC’s STEADI Initiative: Promoting older adult health and independence through fall prevention. American Family Physician, 96(4), 220.
Lim, M., Ang, S., Teo, K., Wee, Y., Yee, S., Lim, S., & Ang, S. (2018). Patients’ experience after a fall and their perceptions of fall prevention.Journal of Nursing Care Quality, 33(1), 46-52. Web.
Lohman, M., Crow, R., DiMilia, P., Nicklett, E., Bruce, M., & Batsis, J. (2017). Operationalization and validation of the Stopping Elderly Accidents, Deaths, and Injuries (STEADI) fall risk algorithm in a nationally representative sample.Journal of Epidemiology and Community Health, 71(12), 1191-1197. Web.
Lyons, B., & Hall, R. (2016). Outcomes of a falls prevention education program among older adults in Grenada.Journal of Community Health, 41(5), 1021-1026. Web.
Mamani, A., Reiners, A., Azevedo, R., Vechia, A., Segri, N., & Cardoso, J. (2019). Elderly caregiver: Knowledge, attitudes and practices about falls and its prevention.Revista Brasileira De Enfermagem, 72(suppl 2), 119-126. Web.
Mark, J. A., & Loomis, J. (2017). The STEADI toolkit: Incorporating a fall prevention guideline into the primary care setting.The Nurse Practitioner, 42(12), 50-55. Web.
Meyer, C., Hill, S., Hill, K. D., & Dow, B. (2019). Inclusive decision making for falls prevention: A discussion tool for use with people with dementia and their caregivers.Journal of Aging and Physical Activity, 27(5), 711-718. Web.
Mota de Sousa, L. M., Marques-Vieira, C. M., Caldevilla, M. N., Henriques, C. M., Severino, S., & Caldeira, S. M. (2017). Risk for falls among community-dwelling older people: Systematic literature review.Revista Gaucha de Enfermagem, 37(4), e55030. Web.
Müller, C., Lautenschläger, S., Dörge, C., & Voigt-Radloff, S. (2019). A feasibility study of a home-based lifestyle-integrated physical exercise training and home modification for community-living older people (Part 2): The FIT-at-Home fall prevention program.Disability and Rehabilitation, 43(10), 1-11. Web.
Nakagami-Yamaguchi, E., Fujinaga, K., Batard, A., Baba, N., Nakamura, K., Miyazaki, K., & Nakatani, T. (2016). The effect of an animation movie for inpatient fall prevention: A pilot study in an acute hospital.Safety in Health, 2(1), 1-10. Web.
Nithman, R. W., & Vincenzo, J. L. (2019). How steady is the STEADI? Inferential analysis of the CDC fall risk toolkit.Archives of gerontology and geriatrics, 83, 185-194. Web.
Orem, D. (1985). A concept of self-care for the rehabilitation client.Rehabilitation Nursing, 10(3), 33-36. Web.
Ott, L. (2018). The impact of implementing a fall prevention educational session for community-dwelling physical therapy patients.Nursing Open, 5(4), 567-574. Web.
Patterson, B. W., Engstrom, C. J., Sah, V., Smith, M. A., Mendonça, E. A., Pulia, M. S., Repplinger, M. D., Hamedani, A. G., Page, D., & Shah, M. N. (2019). Training and interpreting machine learning algorithms to evaluate fall risk after emergency department visits.Medical Care, 57(7), 560-566. Web.
Penning, M. L., Blach, C., Walden, A., Wang, P., Donovan, K. M., Garza, M. Y., Wang, Z., Frund, J., Syed, S., Syed, M., Del Fiol, G., Newby, K. L., Pieper, C., & Zozus, M. (2020). Near real time EHR data utilization in a clinical study.Studies in Health Technology and Informatics, 270, 337-341. Web.
Perrot, A., Ayad, A., Gernigon, M., & Maillot, P. (2019). The impact of therapeutic patient education and physical activity programs on the fall risk of elderly people.Movement & Sport Sciences – Science & Motricité, 109, 3-10. Web.
Phelan, E. A., Pence, M., Williams, B., & MacCornack, F. A. (2017). Telephone care management of fall risk: A feasibility study.American Journal of Preventive Medicine, 52(3), 290-294. Web.
Polit, D. F., & Beck, C. T. (2017). Nursing research: Generating and assessing evidence for nursing practice (10th ed.). Lippincott, Williams & Wilkins.
Pope, C., & Mays, N. (Eds.). (2020). Qualitative research in health care (4th ed.). Wiley Blackwell.
Pua, Y. H., Ong, P. H., Clark, R. A., Matcher, D. B., & Lim, E. C. W. (2017). Falls efficacy, postural balance, and risk for falls in older adults with falls-related emergency department visits: Prospective cohort study.BMC Geriatrics, 17(1), 1-7. Web.
Rockers, P. C., Tugwell, P., Røttingen, J. A., & Bärnighausen, T. (2017). Quasi-experimental study designs series — Paper 13: Realizing the full potential of quasi-experiments for health research. Journal of Clinical Epidemiology, 89, 106-110. Web.
Rutberg, S., & Bouikidis, C. D. (2018). Focusing on the fundamentals: A simplistic differentiation between qualitative and quantitative research. Nephrology Nursing Journal, 45(2), 209-213.
Sarmiento, K., & Lee, R. (2017). STEADI: CDC’s approach to make older adult fall prevention part of every primary care practice.Journal of Safety Research, 63, 105-109. Web.
Schoberer, D., Breimaier, H., Mandl, M., Halfens, R., & Lohrmann, C. (2016). Involving the consumers: An exploration of users’ and caregivers’ needs and expectations on a fall prevention brochure: A qualitative study.Geriatric Nursing, 37(3), 207-214. Web.
Schoene, D., Heller, C., Aung, Y. N., Sieber, C. C., Kemmler, W., & Freiberger, E. (2019). A systematic review on the influence of fear of falling on quality of life in older people: Is there a role for falls?Clinical Interventions in Aging, 14, 701. Web.
Shahrbanian, S., Hashemi, A., & Hemayattalab, R. (2021). The comparison of the effects of physical activity and neurofeedback training on postural stability and risk of fall in elderly women: A single-blind randomized controlled trial.Physiotherapy Theory and Practice, 37(2), 271-278. Web.
Shi, D., DiStefano, C., McDaniel, H. L., & Jiang, Z. (2018). Examining chi-square test statistics under conditions of large model size and ordinal data.Structural Equation Modeling: A Multidisciplinary Journal, 25(6), 924-945. Web.
Shim, S. M., & Kim, E. (2019). Effect of fall prevention education for older patients in comprehensive nursing care service ward.Journal of Korean Public Health Nursing, 33(2), 200-213. Web.
Singh, H., Flett, H. M., Silver, M. P., Craven, B. C., Jaglal, S. B., & Musselman, K. E. (2020). Current state of fall prevention and management policies and procedures in Canadian spinal cord injury rehabilitation.BMC Health Services Research, 20, 1-10. Web.
Snooks, H. A., Anthony, R., Chatters, R., Dale, J., Fothergill, R., Gaze, S., & Russell, I. T. (2017). Support and assessment for fall emergency referrals (SAFER) 2: A cluster randomized trial and systematic review of clinical effectiveness and cost-effectiveness of new protocols for emergency ambulance paramedics to assess older people following a fall with referral to community-based care when appropriate.Health Technology Assessment, 21(13), 1-218. Web.
Stevens, J. A. (2013). The STEADI tool kit: A fall prevention resource for health care providers. IHS Primary Care Provider, 39(9), 162-166.
Stevens, J. A., & Phelan, E. A. (2013). Development of STEADI: A fall prevention resource for health care providers. Health Promotion Practice, 14(5), 706-714. Web.
Taylor, D., McCaffrey, R., Reinoso, H., Mathis, M. W., Dickerson, L., Hamrick, J., & & Klein, C. M. (2019). An interprofessional education approach to fall prevention: preparing members of the interprofessional healthcare team to implement STEADI into practice.Gerontology & Geriatrics Education, 40(1), 105-120. Web.
Ueda, T., Higuchi, Y., Imaoka, M., Todo, E., Kitagawa, T., & Ando, S. (2017). Tailored education program using home floor plans for falls prevention in discharged older patients: A pilot randomized controlled trial.Archives of Gerontology and Geriatrics, 71, 9-13. Web.
Urban, K., Wright, P. B., Hester, A. L., Curran, G., Rojo, M., & Tsai, P. F. (2020). Evaluation of an education strategy versus usual care to implement the STEADI algorithm in primary care clinics in an academic medical center.Clinical Interventions in Aging, 15, 1059. Web.
Vincenzo, J. L., & Patton, S. K. (2019). Older adults’ experience with fall prevention recommendations derived from the STEADI. Health Promotion Practice, 1524839919861967. Web.
Vincenzo, J. L., Shubert, T., Brach, J. S., Tripken, J., Schrodt, L., Sidelinker, J. C., Hazan, P., Hergott, C., Shirley, K., & Rhorer, B. (2020). 4025 Fall risk screening and referrals to community-based programs among physical therapy professionals. Journal of Clinical and Translational Science, 4(s1), 132-132. Web.
Vonnes, C., & Wolf, D. (2017). Fall risk and prevention agreement: Engaging patients and families with a partnership for patient safety.BMJ Open Quality, 6(2), e000038. Web.
Wilkinson, A., Meikle, N., Law, P., Yong, H. J., Butler, P., Kim, J., & & Hale, L. (2018). How older adults and their ihttps://www.sciencedirect.com/science/article/abs/pii/S0020748918300531?via%3Dihubnformal carers prevent falls: An integrative review of the literature. International Journal of Nursing Studies, 82, 13-19. Web.
Wongrakpanich, S., Danji, K., Lipsitz, L., & Berry, S. (2019). STOP-FALLING: A simple checklist tool for fall prevention in a nursing facility.Journal of the American Medical Directors Association, 20(7), 916-918. Web.
Ximenes, M. A. M., Fontenele, N. Â. O., Bastos, I. B., Macêdo, T. S., Neto, N. M. G., Caetano, J. Á., & Barros, L. M. (2019). Construction and validation of educational booklet content for fall prevention in hospitals.Acta Paulista de Enfermagem: Página Inicial, 32(4), 433-441. Web.
Xu, T., O’Loughlin, K., Clemson, L., Lannin, N., Dean, C., & Koh, G. (2017). Developing a falls prevention program for community-dwelling stroke survivors in Singapore: Client and caregiver perspectives.Disability and Rehabilitation, 1-11. Web.
Yoo, J., Kim, C., Yim, J., & Jeon, M. (2016). Factors influencing falls in the frail elderly individuals in urban and rural areas.Aging Clinical and Experimental Research, 28(4), 687-697. Web.
Younas, A. (2017). A foundational analysis of Dorothea Orem’s Self-Care Theory and evaluation of its significance for nursing practice and research. Creative Nursing, 23(1), 13-23. Web.
Zhao, P., Ross, K., Li, P., & Dennis, B. (2021). Making sense of social research methodology: A student and practitioner centered approach. SAGE Publications, Incorporated.
Zhao, Y. L., Bott, M., He, J., Kim, H., Park, S. H., & Dunton, N. (2019). Evidence on fall and injurious fall prevention interventions in acute care hospitals.JONA: The Journal of Nursing Administration, 49(2), 86-92. Web.