Introduction and Background of Waymo
Waymo is a self-driving car company that started in 2005 as a subsidiary of the Google Self-Driving Car Project. Sebastian Thun led the team that designed the Stanley Robot car. The company’s technology was advanced by 2010 as it surpassed the initial technology that had humans behind the autonomous cars for emergency control. Waymo introduced its new self-driving car model that removed the need for steering wheels, pedals, and mirrors (Scanlon et al. 1). The company then held its first autonomous drive on public roads in October 2015, after which Google unit of the autonomous car became a division called Waymo.
Waymo has also extended its vehicle supply deal with Fiat Chrysler to produce a fleet of 600 minivans in 2017. During the same time, the company offered residents of the Phoenix area a free ride in the self-driving minivan. The company used Google’s long period of operation on public roads to enter into the transportation of normal passengers. The firm also established self-driving technology on large commercial trucks in April 2017 before signing a contract with Lyft. Waymo also signed a deal with rental car company Avis to assist in maintaining the firm’s minivan fleet in the Phoenix region (Webb et al. 2). Waymo has several strategies that aim to ensure its success in the self-driven autonomous car. Three main case studies of the Waymo division illustrate its success strategies in the market of self-driven vehicles. This report discusses the reconstruction of fatal crashes in autonomous vehicle operations, Waymo’s safety strategies and readiness, and the case study on the firm’s public road safety data analysis.
Case study 1: Waymo Public Road Safety Performance
Waymo’s mission is to limit traffic injuries and deaths and enhance mobility for all users. The firm’s mission has resulted in the deployment of automated vehicles on public roads without a human driver (Schwall et al. 4). The company, therefore, intends to achieve the mission by issuing the public with information and appropriate data on the validated safety of its automated driving system called the Waymo Driver.
Crashes and Slight Contacts
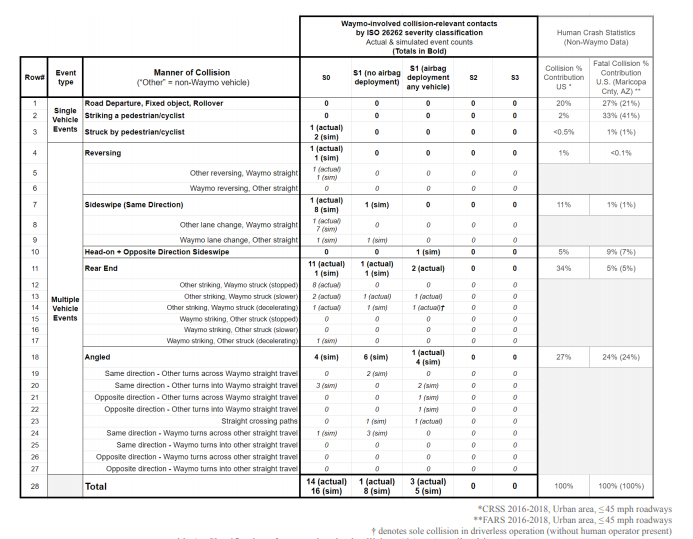
Collision events form significant implications for the public road safety performance of any category of vehicles. Waymo participated in 20 events involving contacts with other objects and resulted in 27 disengagements, which produced contact in post-disengagement simulation for an overall of 47 events as indicated in table 1 (Schwall et al. 6). It is important to have information about driving events suitable and applicable to the Waymo AVs’ safety operating in driverless mode on public roads. Such information is significant in generating extensive learning with the sector, policymakers, and the public while also enhancing public confidence in automated vehicles.
Above 6.1 million miles of automated driving comprising 65,000 miles of driverless activities without a human behind the wheel resulted in 47 crashes or other contact actions (Scanlon et al. 1). There were also 16 rear-end occasions where the Waymo car was struck while stationary or at a gradual deceleration for traffic controls. All three single-vehicle events were non-injurious events involving the striking of a Waymo vehicle by a pedestrian or cyclist while stationary.
Collision Avoidance of Waymo in Management of Human Driver Related Causative Elements
Humans possess a large disparity in driving performances, such as deviations from traffic regulations and safe driving practices resulting in collisions. The case studies above on collisions have one or two rule violations or other driving malpractice from another road user. Table 1 below highlights the contributing components for the forthcoming severe events.
The significance of human deviations from safe driving practices in the table emphasizes the necessity of collision avoidance characteristics in realizing Waymos objective of limiting injuries and fatalities from a collision (Schwall et al. 2). Waymore strategized to eliminate potential collisions resulting from human practices, including aggressive driving, speeding, and negligence. The principal objective of the company’s public road operations is to refine and enhance AV maneuvers in their target environment progressively.
Aggregate safety performance
Lower severity events in both human-operated and automated cars happen at a substantially higher rate than higher severity occasions. The appropriate miles for making statistical implications are therefore fewer. Waymo uses the events that require the 6.1 million miles in self-driving with skilled workers to give an adequate indication to detect average to high differences in SO and S1 occasion frequencies.
In higher severity collision risk, 6.1 million miles fail to offer statistical influence to make significant inferences about the frequencies of severity events (Schwall et al. 4). The statistical noise is large at the 6.1 million milages with low or no event counts giving performance levels and hence the need to consider alternative metrics. Waymo, as a result, adopts the simulation-based and closed-course scenario-based collision avoidance analysis.
Case Study 2: Waymo Safety Methodologies and Safety Preparedness Determinations
Waymo uses its position as the most experienced developer of automated driving systems (ADSs) to develop and apply exemplary high-tech safety methods continuously (Schwall et al. 1). Ways. Approaches help the firm to execute a revolutionary safety model that states its commitment to safety. The firm aims to limit injuries due to traffic and mortalities by ensuring safe and responsible driving and carefully managing risk as the company scales its operations.
Waymo presently operates AVs primarily in California and Arizona while also doing more tests in other states such as Michigan, Texas, Florida, and Washington. The company has a fleet of Chrysler Pacifica in Metro Phoenix that transports passengers in various self-driving services since 2017 (Schwall et al. 2). Waymo has also been providing driverless services in the Metro Phoenix area. Waymo currently has a combined 20 million self-driving miles on public roads with operational experience in over 25 cities, including 74000 driverless miles.
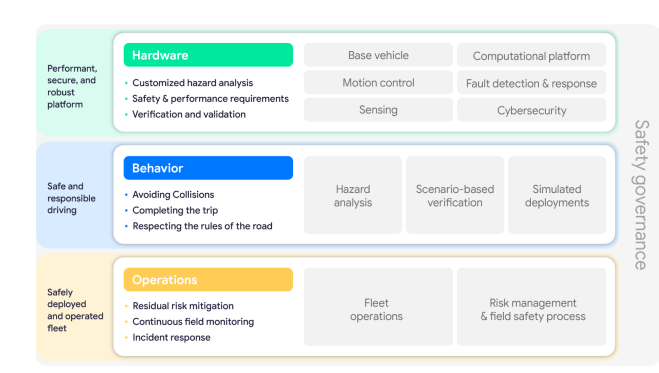
The safety methods emphasize the growth, qualification, positioning, and sustained field operation of an exclusive product that can perform dynamic driving duties without human drivers’ presence in ordinary traffic situations and very complex contexts that one can expect in a specific ODD. The form continues to learn from extensively recognized engineering processes and principles before modifying them using its experience in Level 4 technology (Schwall et al. 13). Waymo uses simulation, closed-course, and public road testing levels to support its method, including the use of various types of elements and subsystem testing. The methodologies are dependent on three phases of technology, including hardware, ADS behavior, and vehicle operations, as shown in figure 2.
Hardware Layer
Waymo purchases safe and completely certified cars from experienced automobile manufacturers. The order has certain specifications such as redundant braking and steering actuators, enabling a safe, driverless vehicle. The technical requirements include widespread authentication of the hardware-in-the-loop and closed-course testing. The company also adds its sensing systems such as a multitude of lidar, radar, camera, and audio units to the vehicle’s base and motion control structures that offer a comprehensive understanding of the driving environment (Webb et al. 13). The system has a set of fault responses that are modified and accorded to any problem. A robust process is also available to identify, select, and prohibit any cybersecurity hazards.
Behavioral Layer
This involves software that directs the safe driverless maneuver of the AVs on public roads. Evaluation of performance capabilities of the ADs behavioral layer is based on three fundamental capabilities and includes crass avoidance, trip completion in the driverless modes, and observance of the existing driving rules. The methodology starts with threat analysis to build robustness before leveraging situation-based substantiation to align the ADs’ behavior with the requirements and expectations (Schwall et al. 4). The final phase involves the large-scale simulation of the system through large-scale log playback or public road operations to permit the empirical estimation of overall performance metrics.
Operation Layer
Waymo has a safety program that enhances the use of leading safety practices in the maneuver of the AVs. The programs include fatigue management procedures for the car operators, event response planning and preparation, and coordination with the law administration and emergency responders to engage the driverless cars appropriately. The company also embraces the seat belt seat in any car and encourages the passengers to belt up while on the ride. The fleet response team is vital for remote support to the ADS when necessary (Webb et al. 24). Waymo’s Field Safety Program is important in identifying and effectuating relevant dispositions of prospective safety aspects depending on the collected data after the release of updated software for driving on public roads. Therefore, the safety process gathers and assists in correcting possible security issues from various sources comprising workers, riders, and the public.
Safety Controls
The control procedure includes a tiered network of security analyses of challenges from the field safety process, risk management, awaiting deployment conclusion, and other sources (Schwall et al. 6). The safety board includes executive leaders from the safety, engineering, and product divisions to intervene in any safety challenges and appraise new safety programs and maintain a current state of the whole safety framework.
Waymo’s Readiness Purpose
Waymo, during certain instances, needs to make a definite determination on its safety methods concerning promptness of a particular configuration of the ADS for a specific arrangement. The firm uses a structured assessment of the important outputs from all the safety methodologies incorporating cautious security and engineering conclusion focused on definite facts applicable to a certain resolve (Schwall et al. 2). The division desires to continuously apply and adapt the methodologies and knowledge from others in the AV sector.
Case Study 3: Waymo Simulated Driving Performance in Rebuilt Disastrous Crashes in an Independent Vehicle Functioning Sphere
The current road traffic mortalities in the United States are at about 36 thousand, while the global deaths are approximately 1.35 million. The operational design domain (ODD) for human-driven passenger automobiles is highly vulnerable to incidences of fatal accidents (Schwall et al. 1). The case study investigates the ADS ability to avoid instigating the collision situation and the effectiveness of AD in reacting to the prospective collision resulting from another party’s initiation.
Severity Assessment
Waymo uses various collision severity dynamics based on various collision dynamics of the first collision incident. The dynamics include avoided collision, mitigated simulations, unchanged simulations, and exacerbated simulations. The collision dynamics were essential, including injury risk models to determine the collision severity from which the resultant maximum party level injury risk forms the total collision severity. Collision severity depends on several situation elements such as the seating position, vehicle occupancy restraint utilization, and inertial car features (Scanlon et al. 1). Waymo Driver uses the ADS sensing and software systems to evade and alleviate possible collisions.
Simulation Safety Impact Performance
Waymo simulated the events that led to a collision with a Driver, representing the fatal collisions common among human drivers. Waymore defined avoidance collision as braking at a deceleration above 0.5 g or steering with a vehicle yaw rate above 8.3 degrees /second (Scanlon et al. 1). The Waymo Driver effectively avoided 82% of the collisions, while 62.5% of the productively avoided responder role simulations, the Waymo Driver used conflict avoidance behaviors without using evasive movement (Scanlon et al. 1). In a residual collision, the Waymo Driver mitigation is possible in numerous actual-world situations related to human driving deviances and mistakes. The mitigation strategy results from a combination of conflict and collision avoidance practices. The graph in figure 3 represents the crash avoidance levels by Waymo Driver.
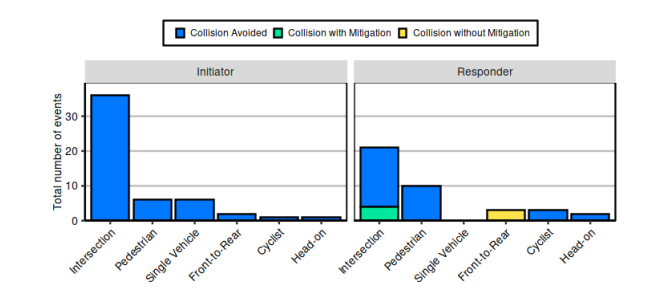
Conclusion and Future Efficiency
Waymo Vehicles have high-tech systems that place them in a better competitive position in the automated vehicle industry. Waymo has implemented various processes that have improved public confidence in its driverless car technology, including safety activities to reduce traffic injuries and deaths through secure and responsible driving. The company has safety methodologies that give a framework of the whole safety process as Waymo advances through development, testing, and deployment in various vehicles.
Waymo has provided information on every actual and simulated collision for missions of miles of automated driving. However, most of the existing data represent the practices of Waymo Driver at an instant, yet the performance is progressively improving. Therefore, Waymo needs to use other tests for the Driver in various instances to show performance in a broad range of environments.
References
Scanlon, John M., et al. Waymo Simulated Driving Behavior in Reconstructed Fatal Crashes within an Autonomous Vehicle Operating Domain, Waymo LLC, 2021. Web.
Schwall, Matthew, et al. Waymo Public Road Safety Performance Data. Cornell University, 2020. Web.
Webb, Nick, et al. Waymo’s Safety Methodologies and Safety Readiness Determinations. Cornell University, 2020. Web.