Introduction
The real estate industry’s growth is evident in the United Arab Emirates as customers buy and sell their properties daily. Customers usually approach real estate agencies to sell or purchase appropriate properties on the market markets. However, the problem is that buyers and sellers find it hard to estimate their houses’ market prices. The absence of a prediction model that considers numerous factors make buyers and sellers underestimate or overestimate their properties’ real value, resulting in significant losses or gains (Stamou et al., 2017). The analysis of the growth rate of the demand for real estate in Dubai is rapid because it almost doubled between 2018 and 2020 (Dahan, 2018). Such rapid growth requires buyers and sellers to have an accurate method of estimating prices and getting their houses’ value. The existence of diverse interests among clients implies that they consider numerous factors in purchasing homes. Some of the critical factors that customers contemplate when buying houses are the number of rooms, space outside, distance from amenities, and security.
The literature analysis shows that customers factor in numerous factors when buying a house for residence or speculation. Ersoz et al. (2018) established that the size, location, age, and popularity of properties are significant determinants of Turkey’s housing prices. In their studies carried out in China, Cui et al. (2018) found out that the nature of the structure, location, quality of the neighborhood, and accessibility to amenities are significant factors that influence houses’ prices. Based on the literature, it is evident that dwellings with many rooms, a high level of security, spacious compound, and additional facilities tend to be more expensive than those without them (Stamou et al., 2017). As one of the real estate companies, ABC helps buyers and sellers to assess and estimate the prices of houses. In this view, the purposed of this assessment report is to create a model that would enable ABC to accurately predict the prices of houses in the market by considering the quality of the neighborhood, the age of the house, the number of bedrooms, the number of bathrooms, the availability of air conditioning, the presence of a pool, and the size of space outside.
By considering the effects of factors, this assessment hypothesizes that the quality of the neighborhood, the age of the house, the number of bedrooms, the number of bathrooms, the availability of air conditioning (ac), the presence of a pool, and the size of space outside are statistically significant predictors. The coefficient of the neighborhood’s quality would be positive to suggest that it increases the price of houses. The age would have a negative coefficient since the price of a house declines with time. Coefficients of the number of bedrooms and bathrooms would be positive because prices increase with the house’s size. Since air conditioning is an accessory, its coefficient would be positive because it increases houses’ prices. The size of the pool would be positive because it is an amenity that increases the price of a house. Space outside would have a positive coefficient since the square feet area increases the price of a house. Therefore, the proposed linear regression model would be:
Prices = β0 + β1 (neighborhood quality) – β2 (age) + β3 (bedrooms) + β4 (bathrooms) + β5 (ac) + β6 (pool) + β7(outspace)
Literature
The growth of real estate in the United Arab Emirates is evident in the past decade, stemming from increases in demand and prices. Dahan (2018) reports that real estate and construction accounted for 13% of the gross domestic product in Dubai because it generated over 50 AED in 2016. In this view, real estate agencies have emerged to help buyers and sellers of houses gain the most out of their investments. The strategic location of Dubai, coupled with the favorable economic environment and security, has attracted people from diverse countries. Typically, buyers and sellers experience the challenge of pricing houses, resulting in significant gains and losses. The existence of numerous factors that determine the prices of homes requires the use of composite models. The application of economic models is essential for accurate prediction and evaluation of customers’ costs to get value for their money.
Given that numerous factors determine the prices of houses in the real estate sector, consideration of the micro-environment is necessary. Cui et al. (2018) explain that house prices in China are subject to micro-and macro-determinants in various jurisdictions. Economic factors, such as land supply, economic status, and movement of people, comprise macro-determinants, while spatial distribution and rent of houses constitute micro-determinants. In their study, Cui et al. (2018) examined the impact of factors at the micro-level on the prices of houses in China. An increasing trend of urbanization increased the demand for houses and augmented prices. Moreover, the diverse interests of customers varied prices of houses in different towns and cities. In the analysis of micro-determinants, Cui et al. (2018) noted that structural features, location, neighborhood attributes, quality environment, and proximity to amenities are major factors that clients consider. In this view, the study recommends considering these factors in evaluating and assessing the prices of houses.
Across the world, factors that determine the prices of houses vary from one country to another or one city to another. In their study, Wittowsky et al. (2020) examined the influence of neighboring apartments, characteristics of houses, and accessibility on the prices of houses in Dortmund, Germany. Adjacent apartments comprise space, security level, ethnic composition, green spaces, and proximity to amenities. Characteristics of homes entailed the size of houses, number of rooms, and type of dwellings. Distances from town, availability of public transport, state of the road, and walkways are some of the features that determine the accessibility of houses (Stamou et al., 2017). The findings of the study indicated that dwelling characteristics, accessibility, and environment are three major factors that determine the prices of houses in Dortmund, Germany. Customers prefer houses that are not only spacious but also accessible to amenities. Moreover, clients have a preference for houses that are in a clean, green, and safe environment.
Further research confirmed that housing characteristics, location, and accessibility play a central role in the pricing of houses. The analysis of the real estate in Turkey was performed to generate information for advising customers on the best prices of houses. Specifically, Ersoz et al. (2018) studied how location, age, and size determine the prices of houses in Turkey by employing a data mining strategy. In the aspect of location, the study sampled data from some houses were located in the urban centers while others were in remote areas. The age of houses varied from five years to over 20 years in various cities and suburban regions of Turkey. The sampled houses had more than one bedroom with at least one bathroom and variable spaces. Ersoz et al. (2018) found out that proximity to an urban area, age, and the fame of houses are statistically significant predictors of prices. These findings suggest that real estate agencies and customers should factor in these significant attributes of houses in estimating their prices.
Analysis
Assumptions
The assumptions of multiple regression were checked using the residual plot, q-q plot, scale-location plot, and variance inflation factor. The residual plot shows that predictors have a linear relationship with the housing prices as per the assumption of regression analysis (Figure 1).
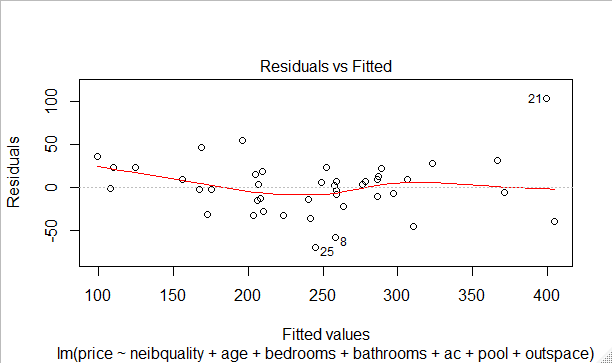
The q-q plot indicates that the standardized residues follow the normal distribution because data points trail the trend of the reference line (Figure 2). However, it has minimal outliers, which do not cause a significant deflection of the normality trend.
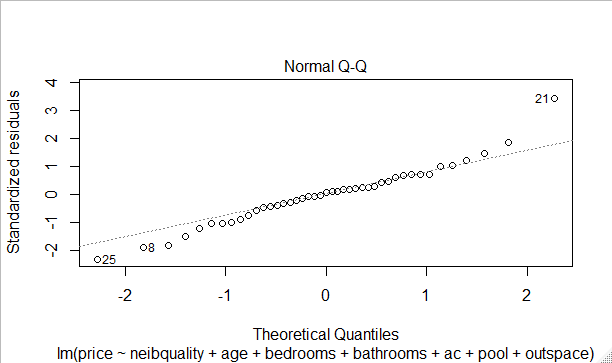
The distribution of the standardized residuals exhibit homogeneity of variance along with the fitted values (Figure 3). The distribution of data points meets the assumption of homoscedasticity since predictors have uniform distribution along the trend line.
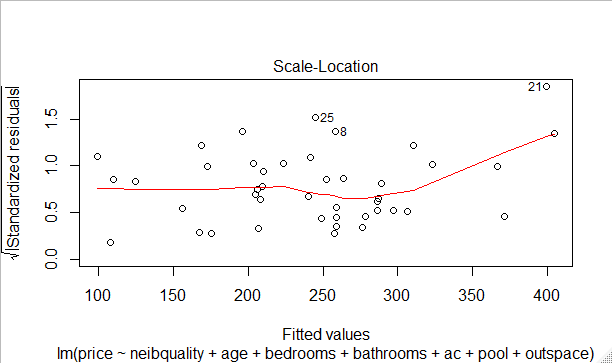
Variance Inflation
Multicollinearity analysis shows that all independent variables have variance inflation factors between 1.29 and 2.31 (Table 1). These values suggest that predictors do not exhibit multicollinearity, hence meeting the assumption of the regression model.

Correlation
Correlation analysis (Table 2) shows that price of houses has negative relationships with neighborhood quality (r = -0.69) and age (r = -0.39). However, price of houses has a positive relationships with the number of bedrooms (r = 0.65), bathrooms (r = 0.76), air conditioning (r = 0.54), the availability of pool (r = 0.10), and space outside a house (r = 0.56).
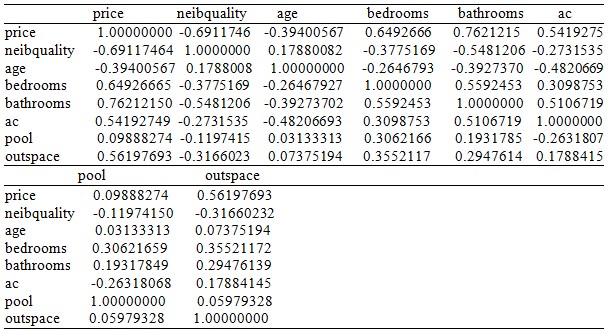
Regression Analysis
The regression model is statistically significant in predicting independent variables’ effect on the housing price, F(7,35) = 27.52, p = 0.000. All predictors in the regression model accounted for 84.63% of the variation in the price of houses (R2 = 0.8463). Coefficients show that neighborhood quality, bedrooms, bathrooms, and space outside are statistically significant predictors (p < 0.05). In contrast, age, air conditioning, and pool are not statistically significant predictors (p > 0.05). These coefficients predict that a unit increase in the quality of neighborhood quality, the age of a building, the number of bedrooms, units of bathrooms, the presence of air conditioning, the availability of a pool, and the size of space outside causes the price of to decrease by 28.33, 0.60, and 0.758, respectively. Moreover, a unit increase in the number of bedrooms, units of bathrooms, the presence of air conditioning, and the size of space outside causes the price to increase by 25.21, 41.59, 22.43, and 0.008, correspondingly.
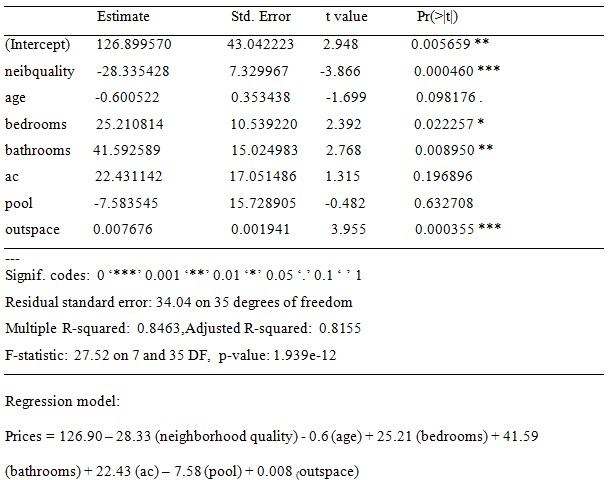
Conclusions and Recommendations
The data assessment revealed that neighborhood quality, the age of a building, the number of bedrooms, units of bathrooms, the presence of air conditioning, the availability of a pool, and the size of space outside account for 84.63% of the variation in the price of houses. The regression model shows that neighborhood quality, bedrooms, bathrooms, and space outside are statistically significant predictors at an alpha level of 0.05. However, the building’s age, air conditioning, and pool are not statistically significant predictors at the same level of significance. Based on these results, the assessment report recommends the following to the ABC management
- Employ the linear regression model in predicting prices of houses based on numerous factors that customers prefer in purchasing houses
- Consider incorporating the neighborhood quality, bedrooms, bathrooms, and space outside as predictors because they are statistically significant predictors.
- Exclude factoring in the building’s age, air conditioning, and pool since they are statistically insignificant predictors of houses’ prices.
References
Cui, N., Gu., H., Shen, T., & Feng, C. (2018). The impact of micro-level influencing factors on home value: A housing price-rent comparison. Sustainability, 10(1), 1–23.
Dahan, A. A. (2018). The future of the real estate industry of Dubai: The demand for real estate (2000-2020). Journal of Global Economics, 6(4), 1–12.
Ersoz, F., Ersoz, T., & Soydan, M. (2018). Research on factors affecting real estate values by data mining. Baltic Journal of Real Estate Economics and Construction Management. 6(1), 220–239.
Stamou, M., Mimis, A., & Rovolis, A. (2017). House price determinants in Athens: A spatial econometric approach. Journal of Property Research, 34(4), 269–284.
Wittowsky, D., Hoekveld, J., Welsch, J., & Steier, M. (2020). Residential housing prices: Impact of housing characteristics, accessibility, and neighboring apartments: A case study of Dortmund, Germany. Urban, Planning and Transport Research, 8(1), 44–70.