- Introduction
- Overview of the Data-Driven Decision-Making Problem
- Justification for the Problem Selection
- Data Requirements, Sources, and Value Proposition
- Solution Deployment Approach
- Data Analytics Tasks, Target Variables, and Features
- Training Data
- Evaluation of Model Performance
- Conclusion and Recommendations
- Reference List
Introduction
Even a small company can benefit significantly from utilizing data related to its industry to make business decisions. Information presents a major opportunity for virtually any market to improve its inner workings greatly. In a highly competitive environment with a low barrier to entry, such as an e-commerce business, the importance of such a source of competitive advantage cannot be underestimated. Sabbeh (2018) suggests that “retaining existing customers is at least 5 to 20 times more cost-effective than acquiring new ones” (p. 273). However, with an abundance of metrics and, therefore, information related to buyers’ behavior, such an approach becomes challenging without an adequate solution. Increasing customer retention for an online store through personalized recommendations is a task that is related to machine learning (Almazora, 2021). In this paper, a problem that online retail stores face nowadays that can be solved with a data-driven decision will be presented and analyzed, and a plan for its fix and evaluation will be proposed.
Overview of the Data-Driven Decision-Making Problem
The rapid expansion of e-commerce companies has made their competitive environment too challenging to handle without extensive analysis tools. With the realization of the customer attrition issue came a shift in perception of marketing-oriented to past and potential long-term customers. Nowadays, there are multiple suggestions on what an online retail store owner can do to achieve an increase in the number of retained customers. However, they often come with little explanation and leave people to implement them via trial-and-error, potentially harming their customer bases (Bao, 2020). Machine learning can be used as a way to diminish unnecessary expenditures, such as failed loyalty programs and inefficient website layouts.
Out of all options, personalization is an easily accessible yet efficient method for retailers to keep a customer engaged for an extended period. This process is defined by AI-driven customization of a company’s marketing mix directed to an individual (Kumar et al., 2019). For example, StitchFix, an online fashion store, achieved a 60% increase in retention due to machine learning (Bao, 2020). However, the way the products may appear to a customer may be inappropriate to the point of becoming a negative factor that leads to a user switching stores due to being overwhelmed by marketing efforts (Hamilton, Rust, and Dev, 2016). Creating a compelling set of personalized recommendations is a challenging task that causes many issues for inexperienced companies and marketing teams.
A part of the problem is the fact that the solution is difficult to establish correctly over the massive amount of data that stems from the nature of online stores. The multitude of metrics available to online store owners leads to the creation of inconsistent strategies, spreading the marketing efforts and accomplishing little to no positive impact (Customer retention measurement: 10 key metrics to track, 2022). Choosing what data to use in this process can cause a wide range of issues. Some data may also be irrelevant or redundant, leading to attempts to influence customers whose buying behavior indicates their inability to commit to a company’s store (Abdalla, 2019; Win and Bo, 2020). There are risks of including data that does not represent the store’s current customer base and market trends. Melendez (2020) states that “the tendency to shortchange the data is a fairly universal problem that often results in misleading or incorrect results and poor outcomes.” Taking only the most actual data is a challenge that many online shops are yet to overcome.
Shopify stores provide an excellent example of how this problem persists throughout customer churn rates. It is difficult to see the exact point at which a customer decides to no longer use a store since there is no subscription to be canceled (Davidson-Pilon, 2017). It makes a marketing team that attempts to assess a user’s intention to continue visiting a store rely heavily on assumptions and hypotheses. Both factors significantly increase the chance of a decision being incorrect or even detrimental to a company.
Justification for the Problem Selection
This problem signifies a necessity to set up a prediction model due to the complexity of data that governs the product recommendations in case of personalization. Providing an attractive plan for a customer is one of the essential steps for increasing customer attrition rates (Lalwani et al., 2021). However, online stores often do not utilize AI-driven solutions, with only 23 percent of businesses successfully achieving this goal (Ringman, 2019). It is possible that companies may fail to see how machine-learning solutions can assist them with reaching out to old customers in an efficient way.
At the same time, the online retail market is knowledgeable about the benefits of customer retention. Returning customers do not only make less investment to make a sale, but they also spend over 60% more on average than new visitors (Olson, 2020; Returning customers pay 67% more than new customers, 2021). Such users are also more valuable when a company introduces new product lines since these customers are more likely to try them and spread the information regarding their qualities (Makad, 2019). Overall, it is only logical for an online retail store to pursue high levels of customer retention.
The issue requires a more in-depth approach to analyzing the data due to the complexity of the situation. High bias or variance may cause severe breakdowns, leading to a necessity to build a data set out of the existing user base to test assumptions in a controlled environment (Mitchell, 2019; Yang et al., 2020). Such an approach makes it necessary to utilize machine learning since empirical experiments will not yield viable results due to the high number of involved variables (Rocks and Mehta, 2022). Selecting appropriate metrics, target retention periods, and suitable prediction model algorithms are the key to a successful machine-learning intervention.
Diagnosing the reasons behind an unsatisfactory customer churn rate is also a necessary step in determining the optimal experience that a store must strive to provide. For example, customers may have issues finding products they desire in a reasonable time frame, receive insufficient support during their visit, or find better experiences and prices elsewhere (Bernazzani, 2022; Campbell, 2020). Their point of view might also be underappreciated, making visitors feel as if they are not being trusted (Makad, 2019). The primary benefit of this solution must be a reduction of one or more reasons behind a high customer churn rate. Personalized recommendations are a fitting way to answer these issues.
Data Requirements, Sources, and Value Proposition
Companies often use pre-made retail systems, such as Shopify, as their platform of choice for an online store. While they give an extensive view of the customer behavior, AI-driven technologies can analyze this data and provide recommendations with accuracy beyond previously possible. Amazon is one such company whose recommendations from its store’s landing page generate approximately two-thirds of all product views (Briedis et al., 2020). The training process of such prediction models requires one to carefully select which data will be used and how it should be trimmed for maximum accuracy with low bias and sufficient variance. Customer behavior is inconsistent in most cases, leading to a significant level of noise in the data used for prediction training (Arora et al., 2021; Smith, Saker and Leano, 2020). Due to this issue, companies are required to use classification methods and separate customers based on their values, despite the possible bias in the results. Avito.ma had an issue with noise in data learning, which resulted in severely decreased accuracy of its prediction model (Rmidi, 2018). Mitigating an improper balance between bias and variances requires one to either seek more data, regularize or categorize it, or change a model structure entirely to reach the irreducible level of errors (Singh, 2018; Tan, 2020). In this case, there is an overabundance of data, implying that supervision is necessary for finding a correct solution.
The sources must be correctly picked to fit into an acceptable bias and variance range. One of the common pitfalls of AI-backed marketing strategies is the creation of information bubbles that stems from feeding algorithms with data that store owners want to see (Faynburd and Simon, 2021). Bias might be inevitable, yet, with acceptable variance, a prediction model will be sustainable within a given environment. For example, Walmart has a multimodal algorithm that groups products based on both products and predicted customer behavior (More, 2017). The goal is to identify and categorize all users from a store as close to their behavior as possible without causing a model to overfit.
The data will be taken from a Shopify store’s current customer base, as well as the platform’s global data bank related to an industry of an example store. Customers must be separated into two groups with additional sub-groups depending on their lifetime value. Two major groups are churners and non-churners, with the latter being divided in accordance with a potential future value (Customer churn prediction using machine learning: Main approaches and models, 2019). This way of defining categories will allow a prediction model to modify the weights of each customer in the final decision for recommendation to ensure that their input indeed does lead to a lower customer churn rate. A proposed solution must increase customer retention in an online store, improving its financial stability and opening up new opportunities for expansion.
Solution Deployment Approach
To implement the solution for the issue of customer retention, it will be essential to focus on keeping customers who fit into a profile of a user with sufficient lifetime value constantly engaged through all possible channels. However, it will be necessary to assess all the users first, after which the products of a store must be analyzed for their relation with recurring visitors. This method may lead to high bias if the categorization is made incorrectly or high variance if no new data can be efficiently added to the model, causing it to be overfitting (Kadi, 2021; Provost and Fawcett, 2013). An ideal solution must be able to cross-reference a store’s user base with products and their related variables and remain applicable for newcomers. Due to the complexity of data, a decision tree with an ensemble method appears to be the most feasible option since it combines classification and regression (Brand, Koch, and Xu, 2020). A tree’s flexibility is sufficient for a proposed problem, as there are several categories involved in assessing the same products.
First of all, it will be important for an online store to consider how one can adequately allocate customers and avoid high bias or variance. This task requires a machine learning team to utilize supervised learning, as datasets must be sufficiently classified and focused through regression algorithms to avoid high variance (Delua, 2021; McAfee and Brynjolfsson, 2012). Such a classification will require setting up decision boundaries that will divide a store’s customer base into segments that will be utilized by a prediction model. Data that will be fed into the system must be correctly assessed. Customers must be separated according to their potential to return to a store. Netflix is one of the example of a successful classification approach, as it continuously updates numerous ‘stereotypical’ profiles for each customer group within a region (Kumar et al., 2019). The Recency, Frequency, and Monetary Value (RFM) technique can be utilized for this purpose, giving an online store a clear view of low-, medium- and high-value targets for personalized marketing (Soto, 2022). It is a commonly implemented method that can separate less loyal customers in a short period (Andi Purnomo, Azzam, and Khasanah, 2020). This part will be later utilized in connection with products.
After the initial classification, the data on product views and sales among said groups of users will serve as a basis for further identification of product recommendations that give maximum retention rates. The next step requires connecting several different components with a time scale. A learning algorithm used for the creation of a prediction model must be able to analyze other groups of customers, extrapolate their interests in products, and provide an optimal path to attracting recurrent visitors. For this purpose, Deep Auto-Regressive (DeepAR) forecasting algorithm can be utilized, as it emulates the behavior and correlation between cross-sectional units within a given period (Mandal, 2020). It will provide machine learning teams the possibility to trace the data points to achieve necessary intermediate data values. Different categorical features can be presented as a dimension that changes over time (DeepAR forecasting algorithm – Amazon SageMaker, no date). Criteria for a data set should be sufficient to create such a space. There are limitations that must be taken into consideration when using this model. DeepAR has issues making predictions with high frequency if the values are too complex and will require setting periods for the forecast with maximum intervals possible (DeepAR forecasting algorithm – Amazon SageMaker, no date). This algorithm, alongside the classification of users, should provide a sufficient basis for a decision tree model.
Data Analytics Tasks, Target Variables, and Features
The primary target variable for this proposition is the number of returning customers in a span of a year. The secondary yet nonetheless critical variable is the customer lifetime value (CLV). However, both variables are not directly affected by the proposed solution, requiring a careful selection of variables that signify the likelihood of a customer returning to a store website. A benefit of a decision tree prediction model is the ability to split the data into a hierarchical structure that clearly shows the relationship between variables (Brand, Koch, and Xu, 2020). However, these variables must be carefully selected for each store’s specifics.
A distinct feature of said variables is their relation with long-standing customers’ shopping activities, such as promotion responses or recommendations to other visitors. There are risks of approximation that stem from overlaying information, yet they are expected in real-world data sets (Knox, 2018). Recurring customers must be reminded of the service on a regular occasion via natural communication. Large companies utilize cause-related marketing for this purpose, as it improves visitors’ perceptions of the value of an online store (Lee and Charles, 2021). The positive effect of this approach can be seen in the example from the available data. Approximately 30% of customers are willing to return to an online retailer whose social position that is shown through such marketing aligns with their views (Polkes, 2020). Machine learning can be used to extract data on the most relevant social issue for customers with high lifetime value and create an efficient cause-related campaign. At the same time, some users may search for the most optimal retailer in terms of discount opportunities. Referral rewards can further complement regular promotions as a means to sustain interest in a brand. Additional discounts per referral reaffirm one’s commitment to a store in addition to generating new visitors (Chiu et al., 2018). Price discounts are essential retaining methods for stores that do not sell ego-boosting utilities.
Predicting churn rates may require assumptions to be made due to inconsistencies stemming from customer behavior (See Figure 1). Their nonlinear decisions can cause a prediction model to allocate non-churn users into churners due to insufficient training information (Pondel et al., 2021). As a result, a data set for this task requires high diversity, proper validation, and must be comparable with relevant real-life examples. Therefore, the first data analytic task for an online retail store is to determine the profile of its typical recurring customer, including the most influential promotion that keeps bringing them back. Consumer expectations derived from such an analysis must then be extrapolated onto potential customers’ preferences (Treiber, 2021). However, this data may not be sufficient if gathered from a single location or a website. Comparing a store’s results with other similar data sets can ensure the validity of information and lower its variance (Dekimpe, 2019). The expected shopping habits among recurring customers must be used as a foundation in creating a new marketing strategy for an online retail store.
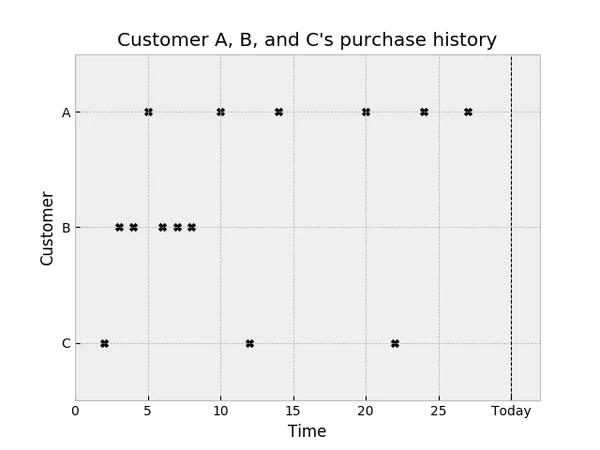
Note: Customer behavior is inconsistent and requires a company to select a scope that will suffice the specifics of its product lines.
Training Data
Relevant information can be collected from samples provided by outside sources, as well as internal archives. Shopify gives an extensive data mining toolset to its users, allowing them to gradually build a solid data set or select from the existing machine learning libraries available for the platform’s users (Vidas, 2022). A large data set is necessary to ensure that a prediction model will know what information to filter (Edirisinghe, no date). This approach gives a model certainty in most real-life encounters.
Selecting metrics that will represent a data set may require additional classification of products. Based on the preferences of the identified groups of customers, specific categories may be more relevant in achieving a higher customer retention rate. Such clusters must be represented among training data by taking a larger space within a data set, as well as guaranteeing diversity of customers for testing, although it will be necessary to clear up noise (Melendez, 2020; Ullah et al., 2019). At this point, cleaning information is essential for achieving the desired outcome.
Finally, it must be understood that the demographics are not constant. A prediction model must be able to adjust itself to a changing environment and different customer bases (Blasinsky and Pressley, 2018). Personalization relies on individual characteristics, yet these parameters are not constant. Customers’ habitual behavior must be used as training data and updated to be reused on a regular basis (Capponi, Corrocher, and Zirulia, 2021). Their browsing patterns, history of purchases, and even email reactions must be documented and represented within a data set (Browning, 2019). While the task may appear insurmountable, machine learning algorithms must be able to accommodate this training data.
Evaluation of Model Performance
To assess the proposed model’s performance, e-commerce businesses will need to gather the following data:
- A customer’s repeated visits over the period of at least six months;
- Total expenditure from a person over that time;
- The amount of potential long-term customers identified and retained due to the proposed changes.
Prediction models can improve a company’s reputation as well by giving it an upper hand in customer interactions. In this case, recommendations will be a way for an online retailer to communicate their understanding of a customer’s desires. A proper model must ensure similarities in outcomes if an input shares many characteristics with existing data (More, 2020). Hypotheses regarding a proposed intervention must be tested on a model. However, both marketing and machine learning teams must take part in assessing said hypothesis by running it through a prediction model generated for customer retention (Tran, 2021). Testing such a model is a necessary step in achieving a higher rate of accuracy.
A compiled network should be able to take a sample data set and fit it within the defined parameters. For this task, it is possible to utilize a visualization method to see a prediction model at work (See Figure 2). This method provides a bridge between business and machine learning teams, improving the likelihood of a successful outcome. The efficiency of forecasting will require input in the form of a new customer who is similar to a preset position in a model, which must then be correctly identified by AI. Inconsistencies must be addressed by adjusting the target bias-variance trade-off and expanding the number of iterations of decision trees, which will cause a higher complexity, yet more accurate outcomes.
Visualization of retention proposed by a prediction model
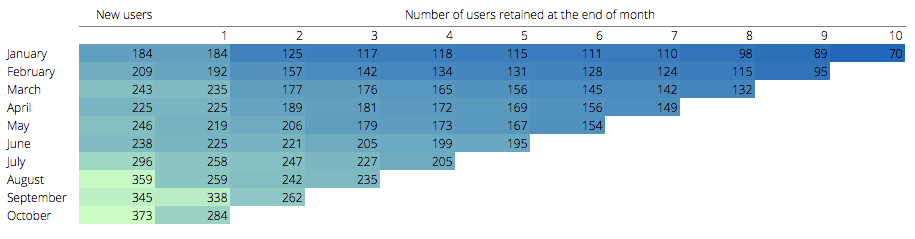
Note: A prediction model can provide a clear forecast of chances of each customer staying with a company over a span of several months.
Conclusion and Recommendations
In conclusion, an e-commerce business that strives to lower its customer churn rate can utilize a prediction model based on the classification of its users and run deep regression algorithms in a supervised environment. This approach gives any retailer an opportunity to define a pre-existing and potential recurring visitor. Understanding that customers’ behavior varies greatly and is often inconsistent over a short span puts a company in a position where an advantage generated by using a prediction model requires long-term planning. However, if such a framework is built correctly, it is expected that a customer retention rate alongside a total value per customer will increase significantly.
It is highly recommended for an online store to implement an AI-based solution for retaining customers. This sector has one of the highest rates of machine learning solutions implemented, as a highly competitive market pushes companies to follow and predict trends among their visitors closely. It is not abnormal for the majority of customers to make a single purchase, yet a company still has to try to change their minds via recommendations (Ching, 2019). A prediction model that will be put to the task of reallocating goals for user experience on a website must be regularized with an input of the most influential ideas.
One of the common errors during an introduction of a prediction model into an online store is improper communication. Machine learning teams must be kept in unison with business managers and owners to avoid making decisions that may lead to unsatisfactory outcomes (Tran, 2021). A model must be as close to real-life situations as possible, although it is understandably challenging to achieve. However, a decision tree method can be smoothed sufficiently to remain compatible with real-life issues (Belkin et al., 2019). Bias is expected to stay, yet its impact must be manageable.
The final recommendation for an online store is to focus on why its long-term customers keep returning. Attention to both positive and negative factors is essential, as the improvement can be achieved from many sides. Nowadays, online retail stores aim to provide frictionless checkout, and recommendations generated by a prediction model can become a valuable method for efficient product placement (Briedis et al., 2020). Capturing the essence of what a customer needs to see to return in the future is the primary goal of modern online retailers.
Reference List
4 must-have cohort analysis for eCommerce (no date) Web.
Abdalla, A. (2019) ‘Increase model performance by… removing data?’. Towards Data Science, Web.
Almazora, E. (2021) ‘5 data-driven strategies for improved customer retention. AdVersity, Web.
Andi Purnomo, M.R., Azzam, A. and Khasanah, A. (2020) ‘Effective marketing strategy determination based on customers clustering using machine learning technique’, Journal of Physics: Conference Series, 1471, p. 012023.
Arora, N. et al. (2021) ‘The value of getting personalization right–or wrong–is multiplying’,McKinsey. Web.
Bao, H. (2020) ‘Repurchase rate: Over-looked metric of thriving eCommerce’, BSS Commerce, Web.
Belkin, M. et al. (2019) ‘Reconciling modern machine-learning practice and the classical bias-variance trade-off’, Proceedings of the National Academy of Sciences, 116(32), pp. 15849–15854.
Bernazzani, S. (2022) ‘22 Examples of customer retention strategies that work’, Hubspot, Web.
Blasinsky, C. and Pressley, E. (2018) ‘Think globally’, NACS Magazine, Web.
Brand, J., Koch, B. and Xu, J. (2020) Machine learning. London, UK: SAGE Publications Ltd.
Briedis, H. et al. (2020) ‘Adapting to the next normal in retail’, McKinsey, Web.
Browning, K. (2019) ‘Personalization: The secret to customer retention, Justuno, Web.
Campbell, P. (2020) ‘Why customers churn: Top 8 causes of churn’, Profitwell, Web.
Capponi, G., Corrocher, N. and Zirulia, L. (2021) ‘Personalized pricing for customer retention: Theory and evidence from mobile communication’, Telecommunications Policy, 45(1), p. 102069.
Ching, C. (2019) ‘Modeling customer churn for an e-commerce business with Python’, Towards Data Science, Web.
Chiu, Y.-L. et al. (2018) ‘Studying the relationship between the perceived value of online group-buying websites and customer loyalty: the moderating role of referral rewards’, Journal of Business & Industrial Marketing, 33(5), pp. 665–679.
Customer churn prediction using machine learning: Main approaches and models(2019) Web.
Customer retention measurement: 10 key metrics to track (2022) Web.
Davidson-Pilon, C. (2017) ‘How Shopify merchants can measure retention’, Shopify, Web.
DeepAR forecasting algorithm – Amazon SageMaker (no date) Web.
Dekimpe, M.G. (2019) ‘Retailing and retailing research in the age of big data analytics’, International Journal of Research in Marketing, 37(1).
Delua, J. (2021) ‘Supervised vs. unsupervised learning: What’s the difference?’, IBM, Web.
Edirisinghe, E. (no date) ‘Reinforcement learning for personalized recommendations’. Web.
Faynburd, A. and Simon, F. (2021) ‘Understanding and Fighting AI Bias’, Retail TouchPoints, Web.
Hamilton, R.W., Rust, R., and Dev, C. (2016) ‘Which features increase customer retention?’, MIT Sloan Management Review, Web.
Kadi, J. (2021) ‘The relationship between bias, variance, overfitting & generalization in machine learning models’, Towards Data Science, Web.
Knox, S.W. (2018) Machine learning: A concise introduction. Hoboken, NJ: John Wiley & Sons, Inc.
Kumar, V. et al. (2019) ‘Understanding the role of Artificial Intelligence in personalized engagement marketing’, California Management Review, 61(4), pp. 135–155.
Lalwani, P. et al. (2021) ‘Customer churn prediction system: A machine learning approach’, Computing [Preprint].
Lee, L. and Charles, V. (2021) ‘The impact of consumers’ perceptions regarding the ethics of online retailers and promotional strategy on their repurchase intention’, International Journal of Information Management, 57, p. 102264.
Makad, S. (2019) ‘6 reasons why customer retention can drive E-commerce growth’, Wigzo, Web.
Mandal, K. (2020) ‘Machine learning applications in eCommerce’, in. State of AI and ML, IEEE Computer Society. Web.
McAfee, A. and Brynjolfsson, E. (2012) Big Data: The Management Revolution, Harvard Business Review. Web.
Melendez, C. (2020) ‘The nurturing and feeding of data: The unsung hero in AI development’, Forbes, Web.
Mitchell, M. (2019) ‘Selecting the correct predictive modeling technique’, Towards Data Science, Web.
More, A. (2020) ‘Product matching in eCommerce’, Medium, Web.
Olson, S. (2020) ‘What is customer retention? 11 examples and strategies to retain customers, Zendesk, Web.
Polkes, A. (2020) ‘The state of brand loyalty 2021: Global consumer survey’, Yotpo, Web.
Pondel, M. et al. (2021) ‘Deep learning for customer churn prediction in e-commerce decision support’, Business Information Systems, pp. 3–12.
Provost, F. and Fawcett, T. (2013) Data science for business: What you need to know about data mining and data-analytic thinking. Beijing Etc.: O’Reilly.
Returning customers spend 67% more than new customers (2021) Web.
Ringman, M. (2019) ‘Approaching AI as a strategic business opportunity, Forbes, Web.
Rmidi, A. (2018) ‘How I used deep learning to optimize an eCommerce business process with Keras’, Towards Data Science. Web.
Rocks, JW and Mehta, P. (2022) ‘Memorizing without overfitting: Bias, variance, and interpolation in overparameterized models’, Physical Review Research, 4(1).
Sabbeh, S. (2018) ‘Machine-learning techniques for customer retention: A comparative study, International Journal of Advanced Computer Science and Applications, 9(2), pp. 273–281.
Singh, S. (2018) ‘Understanding the bias-variance trade-off’, Towards Data Science, Web.
Smith, B., Saker, R. and Leano, H. (2020) ‘How to predict customer churn while maintaining profitability’, Databricks, Web.
Soto, A. (2022) ‘Customer RFM classification’, Artificial Intelligence in Plain English, Web.
Tan, T. (2020) ‘Examples of bias-variance trade-off in deep learning’, Towards Data Science, Web.
Tran, N. (2021) ‘How did machine learning save cost for eCommerce companies’, Towards Data Science, Web.
Treiber, J. (2021) ‘AI innovation: A guide to modern retail customer retention strategy’, Data Clarity, Web.
Ullah, I. et al. (2019) ‘A churn prediction model using random forest: Analysis of machine learning techniques for churn prediction and factor identification in telecom sector’, IEEE Access, 7, pp. 60134–60149.
Vidas, I. (2022) ‘The magic of Merlin: Shopify’s new machine learning platform’,Shopify, Web.
Win, T.T. and Bo, K.S. (2020) ‘Predicting Customer Class using Customer Lifetime Value with Random Forest Algorithm’, IEEE Xplore.
Yang et al. (2020) ‘Rethinking bias-variance trade-off for generalization of neural networks, in Proceedings of the 37th International Conference on Machine Learning. Proceedings of Machine Learning Research. Web.