Introduction
Innovations, such as AI have a strong potential to enhance contemporary society. Advances in these technologies create the basis for critical applications in manufacturing, promoting future opportunities in manufacturing systems and ultimately challenging traditional conceptions of manufacturing operations in society. There is an increasing debate on the role of artificial intelligence technologies in critical processes, which clashes with the human oversight role. The effectiveness of human decision-making might depend on numerous factors, such as alcohol, current motivations, education, criminal history, and motives. That is why advocates of AI emphasize its ability to resolve issues being free from subjective judgments. The application of such technology has a significant contribution to manufacturing operating systems.
Background
There are many ways in which technology can improve manufacturing. It helps to resolve such issues as unskilled or a lack of skilled workforce, security of data, supply chain, autonomous monitoring, managing factory from a phone, remote access to experts, predictive maintenance, better product lifecycles, quality inspection, and generative designs (Li et al., 2017). However, the sphere of technology is very versatile as many tools exist to resolve the abovementioned issues. In terms of artificial intelligence, the scope of improvements is narrowed down to the following: optimizing the supply chain, autonomous monitoring machines, predictive maintenance, quality inspection, and generative designs.
The application of artificial intelligence to manufacturing processes has reduced the time and resources spent on certain operations. Artificial intelligence can save costs on staff and improve operational cycles by eliminating mistakes related to human fatigue. Competition in the industrial sector is stiff, necessitating manufacturers to produce high-quality items while also reducing wastage. In particular, applying AI to lean manufacturing principles is crucial to realizing effective and efficient productivity (Li et al., 2017). Manufacturing is a process that runs from the assembly of raw materials, inserting essential components, testing, final assembly, final test, and packaging (Li et al., 2017). The production of any final product is curtailed by a set of wastage that needs addressing.
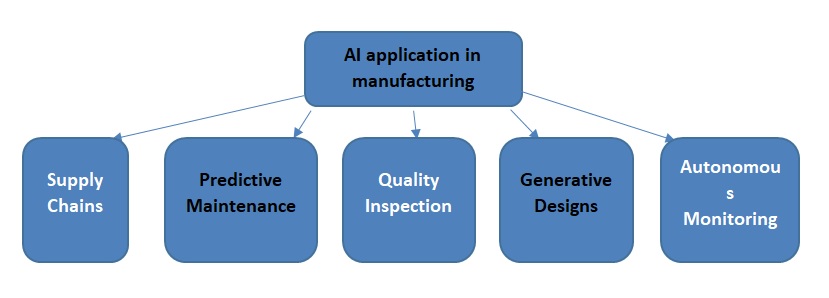
Problems
Artificial Intelligence in Lean Manufacturing
First, there is a wastage of time in terms of cycle time that is below the takt time (Cuesta et al., 2019). Cycle time refers to the time taken to make each unit, and particularly the desired time to complete one step (Mittal et al., 2019). On the other hand, takt time refers to the rate at which a product must be completed to meet the customers’ demands. Notably, the takt time depends on the customers’ demands, while cycle time depends on the required process to manufacture a particular product. Regarding the manual manufacturing process, the time to complete a production step is much longer than the desired timeframe. Concurrently, the packaging time is way above the wanted takt time (Cuesta et al., 2019). Furthermore, waiting represents another observable wastage. In particular, waiting connotes the time wasted while waiting for the next step, as it takes longer than the desired time for the next step to be initiated. In addition, obstruction within the corridors of workstations and transportation is another challenge that reduces efficiency and productivity (Cuesta et al., 2019). The distance between the raw materials warehouse, the production process, and packaging was long enough to slow down the completion of units, as noted by Cuesta et al. (2019). Also, there is a disorder in workstations.
The lean standards of manufacturing are the activities and techniques applied in the production process to identify the bottlenecks and streamline the efficiency of the process while ensuring high productivity. As discussed above, the wastage presented in the packaging section would be improved by eliminating one assembly station and redistributing the workload to the other stations, as there were excess stations that reduced efficiency, hence increasing the efficiency by 4.92 percent (Cuesta et al., 2019). Moreover, implementing the 5s tool would increase cleanliness and liberate space in the corridors, reducing occupational accidents. Also, the 5s implementation would decrease unnecessary movement by placing shelves of required components in front of the operators minimizing the effort to search for items (Cuesta et al., 2019). Besides relocating the raw materials warehouse close to the line and quality control at the end line, the supermarket system reduces movement and time for supply and dispatch.
Solutions
Mieruka, a Japanese term meaning control, is a potent lean tool that allows for synthesis and visualization of the performance. Artificial intelligence could significantly improve this aspect of manufacturing (Sahu et al., 2021). The visual signals present a system standard for production, explaining what people expect. In particular, the system encompasses the four levels of visual management by indicating the area of production, the machines used, and the entire production process. The signals include floor marks that define particular spaces like walkways, resources, tooling areas, and material intake points; safety signs like fire extinguishers and emergency exits (Kinkel et al., 2021). Most significantly, the visual signals constitute the process or machine’s light that indicates the current state of a particular action and documentation related to standardized work (Kinkel et al., 2021). Hence, artificial intelligence algorithms could perform the visualization aspect on-demand to save time and costs.
Artificial Intelligence in Machining
Artificial Intelligence can contribute to manufacturing by increasing its productivity. One of the examples of such improvements can be taken from the machining industry. Machining is the process of cutting raw materials such as metal, wood, or plastic into the desired final shape and size (Wan et al., 2020). Modern machine shops use computer-controlled precision machining tools for cutting, drilling, boring, milling, etc. (Wan et al., 2020). Therefore, machining is an essential part of the manufacturing industry.
Historically, a major challenge of the machining industry is the time-consuming task of cost estimation. For example, when a machine shop takes an order for building parts such as cabinet hinges, plinths, or furniture feet, it needs to determine the individual cuts and holes that need to be made for each item by carefully reading the blueprint provided by the customer (Sahu et al., 2021). When done manually, this process requires machining experts who are dedicated to the cost estimation process, which can act as a bottleneck for the productivity of the machine shop as a whole.
However, a Japanese company called Kabuku has managed to resolve the bottleneck challenge. Kabuku has launched a new solution by handling the data management of their machining process with AI (Wan et al., 2020). Kabuku is a startup focused on modernizing the manufacturing industry with 3D printing, cloud, and AI technologies for major manufacturing customers such as Toyota, Honda, and Olympus (Wan et al., 2020). The company has developed a tool that allows a blueprint to be analyzed for cost estimation. The uploaded blueprint is investigated and highlights every object that demands to process. As a result, a customer receives the number of steps needed for the final product to be done, the cost of each step, and the total estimated cost for the entire part. In addition, the tool provides detailed information for each aspect, and this process takes seconds. Therefore, the time required and the price of cost estimation can be greatly reduced through artificial intelligence technology.
Decreasing Manufacturing Errors
The demand for high-quality and highly customized manufacturing is rising. Machine learning and artificial intelligence technologies are applied to meet the growing demand. As such, batch size 1 is the new manufacturing paradigm. In mass production, predictive maintenance was employed. However, for batch size 1, it is not applicable due to the large variation in the production environment (Wang, 2019). For example, tools can be worn out, which may affect the final product quality. Alien objects can damage manufacturing tools and products. Finally, low-quality parts will be used for the final products. Such problems are often detected too late, so they may impact product quality and productivity.
The current problem monitoring solutions may cost too much time and money. Hence, the Siemens company has developed innovative technology to automate and simplify the process. This technology allows manufacturers to train a neural network and deploy custom models. A technology recognizes abnormal situations without user intervention. The operator receives notifications within seconds, enabling immediate problem resolution (Wang, 2019). This has been shown to reduce non-conformance costs, and expensive tool breaks down significantly. Hence, products are released faster with the highest quality.
AI in Additive Manufacturing
Besides that, artificial intelligence is widely applied in the additive manufacturing (AM) industry, which has experienced significant growth during the last few years due to the COVID-19 pandemic. AI provides inspection benefits for AM systems in the hardware space to ensure greater process control and repeatability (Yao et al., 2017). AI can use pattern recognition to form the best practices for error handling. AI-driven hardware can spot defects and part production and report them to the cloud. Workers on the line immediately pull the defective units before reaching a final assembly, saving time and money in recalls and repairs. All in all, the use of additive manufacturing and artificial intelligence can cut the production time of 3D printed parts from 30 minutes to mere seconds per individual job. AI can increase printer utilization, optimize the material selection, reproduce production errors and catch production defects along the way. Artificial intelligence helps engineers to ensure the rules of 3D printing are followed and helps produce a large amount of data along the production process (Yao et al., 2017). By providing automated assistance, additive manufacturing enhanced by AI can be the manufacturing solution of the future.
Conclusion
In conclusion, AI can extend the sheer reach of potential applications in the manufacturing process from real-time equipment maintenance to virtual design that allows for new, improved, and customized products to a smart supply chain and the creation of new business models (Mittal et al., 2019). Developing artificial intelligence capabilities in industrial manufacturing is not only critical for economic transformation but also a mechanism toward sustainable competitive advantages. The application of artificial intelligence in manufacturing has its benefits in the spheres of predicting maintenance issues, increasing production efficiency, enhancing the quality of a product using computer vision, and faster adaptions to market changes.
Recommendations
In predicting maintenance issues, AI-based sensor technologies can be effective in providing accurate and relevant information about current issues and problems that should be resolved. Artificial intelligence leads to significant improvement in enhancing the effectiveness of manufacturing processes (Mittal et al., 2019). AI also offers data helping managers to improve decision-making and contribute to better goal achievement (Zhang et al., 2020). Artificial intelligence-equipped cameras provide levels of sensitivity to spot the items that need corrections. Machine-vision software uses computer vision to spot microparticles and surface defects while enabling a computer to see, analyze, and learn from the collected data (Cuesta et al., 2019). This not only boosts the inspection process but can also ensure changes in the final product. Faster adaptions to market changes are achieved because artificial intelligence not only plays a role in operational production levels but also in improving manufacturing supply chains, pattern recognition, or consumer behavior analysis (Cuesta et al., 2019). This guarantees an organization can predict shifts in the market, build plans, track metrics, or even automate processes in the output, quality, or cost control of analytics. Finally, with AI algorithms able to formulate estimates on the information gathered, manufacturers can also optimize manpower, raw material availability, inventory management, and many other vital processes for the industry. Hence, while AI has only helped some spheres to improve, it has already succeeded in changing and enhancing numerous manufacturing firms.
References
Cuesta, S., Siguenza-Guzman, L., & Llivisaca, J. (2019). Optimization of assembly processes based on lean manufacturing tools. Case studies: Television and printed circuit boards (PCB) Assemblers. In M. Botto-Tobar, M. Vizuete, P. Torres-Carrión, & S. León (Eds.), International conference on applied technologies (pp. 443-454). Springer.
Kinkel, S., Baumgartner, M., & Cherubini, E. (2021). Prerequisites for the adoption of AI technologies in manufacturing – Evidence from a worldwide sample of manufacturing companies.Technovation, 102375. Web.
Li, B. H., Hou, B. C., Yu, W. T., Lu, X. B., & Yang, C. W. (2017). Applications of artificial intelligence in intelligent manufacturing: a review.Frontiers of Information Technology & Electronic Engineering, 18(1), 86-96. Web.
Mittal, S., Khan, M. A., Romero, D., & Wuest, T. (2019). Smart manufacturing: Characteristics, technologies and enabling factors.Journal of Engineering Manufacture, 233(5), 1342–1361. Web.
Sahu, C. K., Young, C., & Rai, R. (2021). Artificial intelligence (AI) in augmented reality (AR)-assisted manufacturing applications: a review.International Journal of Production Research, 59(16), 4903-4959. Web.
Wan, J., Li, X., Dai, H. N., Kusiak, A., Martínez-García, M., & Li, D. (2020). Artificial-intelligence-driven customized manufacturing factory: key technologies, applications, and challenges.Proceedings of the IEEE, 109(4), 377-398. Web.
Wang, L. (2019). From intelligence science to intelligent manufacturing.Engineering, 5(4), 615-618. Web.
Yao, X., Zhou, J., Zhang, J., & Boër, C. R. (2017). From intelligent manufacturing to smart manufacturing for industry 4.0 driven by next-generation artificial intelligence and further on.2017 5th International Conference on Enterprise Systems, 311-318. Web.
Zhang, J., Arinez, J., Chang, Q, & Gao, R. (2020). Artificial intelligence in advanced manufacturing: Current status and future outlook.Journal of Manufacturing Science and Engineering, 142(11), 110804. Web.