Introduction
Facial recognition technology is becoming increasingly common in today’s world. The implantation of a chip in video cameras to identify the face of the shown participant is actively used for national security purposes of the state, in the agricultural sector, and in the front camera modules of smartphones for unlocking access (Othman & Aydin, 2018). Thus, there is a serious social demand for improvement in such systems since technical errors are unacceptable. That being said, it is important to consider that computer accuracy is directly dependent on learnability, for which humans are responsible (Guo & Zhang, 2019).
In turn, this generates a reasonable hypothesis: errors are likely in this system’s operation if a human is used as a computer intelligence. In this experiment, a statistical experiment was conducted to investigate a video face recognition system using human short-term memory and comparison abilities.
Method
The central idea of this trial was to use a video in which 4-5 photographs of men were shown to the participant with a duration of 15-30 seconds. The men’s photographic portraits were similar, and the persons depicted were of Hispanic, Caucasian, and light-skinned African American backgrounds between the ages of 21 and 45 years old. The hair color of all the men did not vary much, ranging from brown to black. Thus, for the test was formed the most similar sample of photos, which, according to the study’s idea, was to complicate the recognition of faces. After a set time, the computer screen hid all the pictures shown and then presented the respondent with one picture of a man’s face, asking if this person had been shown earlier. Subsequently, the number of correct answers was counted and analyzed.
Results
The primary outcome of the test was the number of correct responses attempted. Specifically, of the ten questions asked about the man’s presence in the previously shown sample, there were seven correct answers. This implies that 70% of all individuals shown were processed correctly, while 30% of individuals were perceived incorrectly by the respondent (Figure 1).
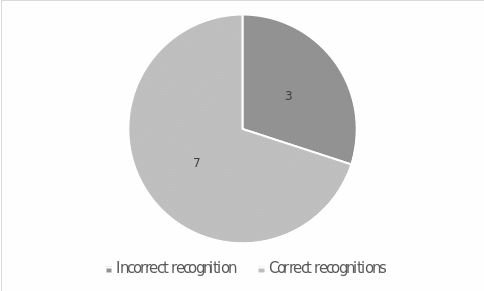
Discussion
The percentage of correctly guessed faces is of key importance for a video recognition system. The final effectiveness of the technology depends on how well and accurately the camera identifies the observed faces with the database. In this laboratory test, the respondent played the computer’s role automatically comparing the recognized faces with the database. A sample of 4-5 photos was offered as a database, and the participant’s task was to experimentally compare the image shown with the one that was present on the screen earlier.
The result achieved of 70% correctly recognized faces might indicate a generally working cognitive comparison system. In particular, correct answers to the questions posed were given for the most part. This implies that for this sample, the recognition performance is above average. These findings correlate with the results of a similar study that evaluated gender patterns of face recognition (Rehnman & Herlitz, 2007).
Specifically, it was shown that men, on average, were worse at remembering faces than women, which is also true for this test. For this reason, recognition of Hispanic, Caucasian, and light-skinned Hispanics may be performed satisfactorily in future studies. Consequently, the hypothesis posited is confirmed because errors did occur in the test. Nevertheless, while this percentage may be acceptable in this test, where the role of the computer was played by a student, it is too low a performance of the recognition system in an industrial video camera environment.
References
Guo, G., & Zhang, N. (2019). A survey on deep learning-based face recognition. Computer Vision and Image Understanding, 189, 1-37.
Othman, N. A., & Aydin, I. (2018). A face recognition method on the Internet of Things for security applications in smart homes and cities [PDF document]. Web.
Rehnman, J., & Herlitz, A. (2007). Women remember more faces than men do. Acta Psychologica, 124(3), 344-355.