Introduction
General Background
Data science is a discipline that entails the study of information. It cuts across many areas by helping experts in various fields to study and work collectively as well as making sense of data (Newman et al. 2016). Productivities from different works can spawn data in diverse formats. Processing, evaluation and reporting of data outputs are important steps for all economic sectors. In businesses, data science makes it possible to understand everyday problems and business performance on a daily, weekly, monthly or yearly basis. It also helps businesses to predict their performance and make the necessary adjustments.
Data science is important for businesses because it facilitates the collection and analysis of data on business procedures, schemes and general performance. Consequently, businesses can use the outputs of data science to enhance their products, services, or operations. An additional advantage of data science in business is facilitating predictive modeling to enable the timely development of apposite strategies. A business data science (BDS) model is necessary to realize all these benefits.
According to Newman et al. (2016), five key attributes make up data science. These qualities are summarized as the 5 Vs of velocity, volume, variety, value and veracity. Volume denotes the size and amount of data concerned, whereas velocity is the speed at which the data is created and stored for businesses. Veracity refers to the degree of accuracy and precision in the analysis and interpretation of results, which is critical to decision-making and business development. In contrast, variety refers to the different types of data generated and various formats that they can be presented. Value alludes to the additional worth created by BDS models to produce positive outputs for multiple organizations.
Challenge
There are no established ways of implementing BDS because current literature does not have a definite standard or best practice approach. Even though many organizations are interested in data science, they lack the knowledge of how to manage and handle data science. Newman et al. (2016) developed a business data science model in a format that can be adopted by businesses. The model provides an organized standard for the advancement of business projects and services.
The authors focus on pertinent modeling and simulation methods to facilitate the smooth transition to BDS. Modeling and simulation are critical steps that must be done to guarantee that a BDS model can work efficiently with existing business activities, models, processes and functions. Additionally, the empirical design of BDS models should merge seamlessly with areas such as business intelligence and research.
Insights from extensive reviews of the literature were used to develop a conceptual model for BDS. Important aspects that were clarified included modeling and simulation techniques, system dynamics, design features, software issues, experimental design for specific businesses, analytics, privacy and security issues, as well as the contribution of the model to data science. However, several questions remained unanswered, including how business intelligence can combine with BDS to create significant positive impacts in the analysis of large data volumes and transforming outputs into visualization and interactive explorations. Another issue that arose was how business analytics can convey outputs effectively to people without technical know-how.
Solution
Wazurkar, Bhadoria and Bajpai (2017) proposed a way of dealing with large volumes of data using Predictive Analysis (PA) as a theoretical decision-making process for data to maximize the success of handling large datasets. This idea was put to test by Roskams and Haynes (2019) in a bid to comprehend the environmental performance of the workplace. Predictive analysis was used to forecast subjective environmental comfort by combining real-time environmental data with independent assessments. Environmental sensors designed to quantify basic indoor environmental quality parameters were fitted into an open-plan office.
The specific environmental strictures that were measured included temperature, carbon dioxide concentrations, humidity, lighting and sound pressure. In addition, spatial measures such as workspace integration and workspace density were taken for each workspace using building modeling techniques. A total of 15 workers were sampled repeatedly over 11 days. The subjects were asked to give 78 brief ratings of their environmental comfort (Roskams and Haynes, 2019). Various models were used to investigate the extent to which the independent environmental data forecasted subjective environmental comfort.
Definition of the Technology
Business intelligence (BI) refers to methods or practices that use varying technologies to develop applications that evaluate existing business data to guide decision-making based on the forecasts made by the data. BI includes data processing and analytical machinery as well as numerous business-focused practices that apply to different sectors such as healthcare, e-governance, security, market intelligence and e-commerce. The success of BI depends on the quality of data and the efficiency of the data management protocols. The analytical methods used in these systems are based on data mining techniques and statistical computations. Figure 1 shows a schematic representation of various scenarios that are possible in business analytics, which is the core of BI.
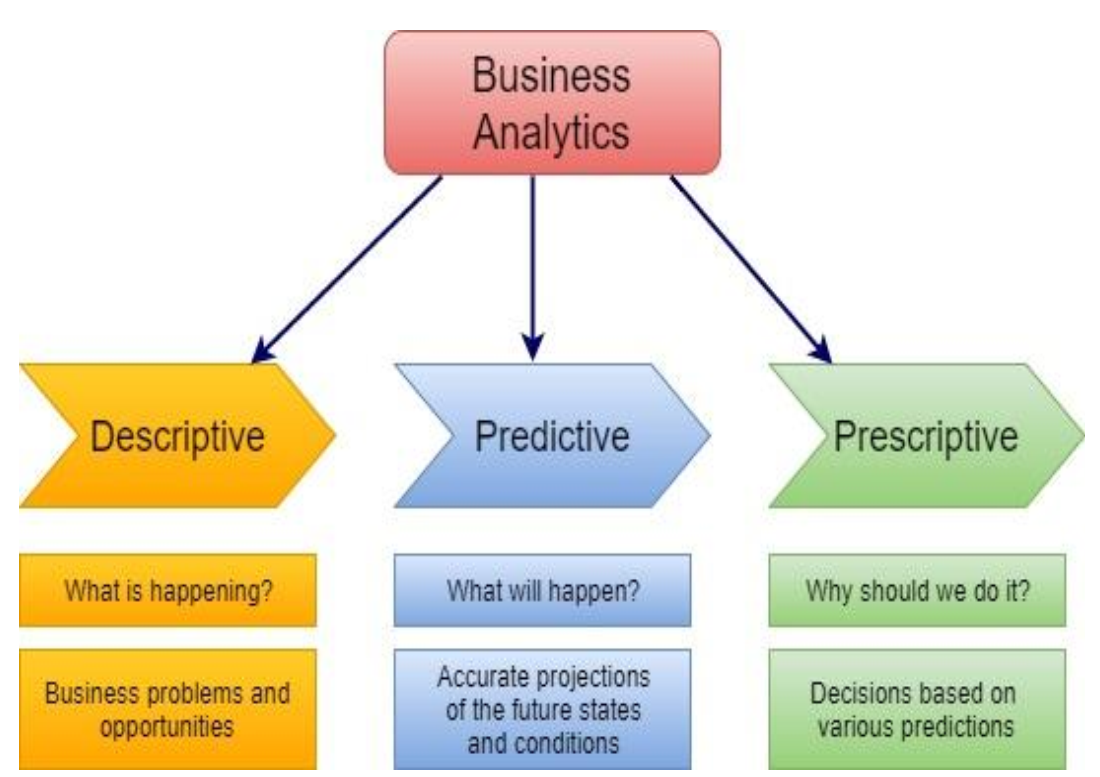
The three pillars of business analytics are descriptive, predictive and prescriptive. The predictive analysis depends on predictive models to establish patterns in data and use them to forecast events. These models make use of various algorithms that may work simultaneously or consecutively. To obtain optimal outcomes from predictive analysis, businesses need to follow six key steps as outlined in Figure 2.
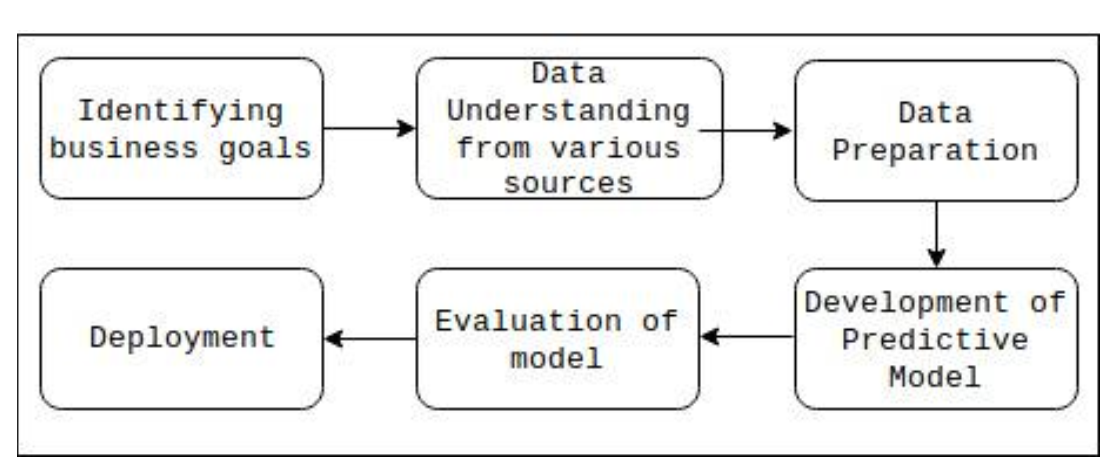
Results
The outcomes of the predictive analysis showed that elevated carbon dioxide concentrations were linked with more negative scores of air quality. In contrast, higher ‘workspace integration’ correlated with higher degrees of distractions, whereas higher workspace density was connected to reduced social interactions (Roskams and Haynes, 2019). Overall, the authors determined that data from sensors helped monitor air quality and ensure occupant satisfaction. Therefore, the predictive analysis could be used to ensure functional workplaces.
Summary of the Research Area
Business models are shifting from cost reduction measures to value-added services as ways of ensuring client satisfaction. The optimization of indoor environmental quality (IEQ) is one way that managers can use to guarantee the comfort of the workplace environment for maximum productivity. Organizations such as the WELL Building Standard have guidelines for IEQ maintenance, which require the physical measurement of parameters to ensure that they fall within predetermined comfort limits (Nag, 2019). However, such an approach has shortcomings such as high costs and a limited ability to monitor specific locations for prolonged periods.
The inception of wireless technology has made it possible to obtain uninterrupted measurements of key environmental parameters in precise locations at definite times. Data from multiple sensors can be superimposed onto a three-dimensional replica of the workplace and envisaged in real-time, thereby permitting the prompt detection and redress of substandard environmental conditions.
Evaluation
The main finding of the study was that wireless sensors were valuable sources of environmental data that could be exploited by predictive analysis to maintain optimum workplace conditions. This was the first experimental study to look into the association between physical environment data from wireless sensors and independent assessments of environmental comfort. The outcomes are useful proof-of-concept of the viability of integrating building and human analytics.
Previous studies have looked into predictive analysis in the management of parking lots (Maheshwari and Sivakumar, 2018), management of pathology facilities in hospitals (Lessard et al., 2016) and safe operation of gas assets (Engelbrecht and Abbaspour, 2017) among other applications. These studies confirmed the usefulness of predictive analysis in planning endeavors. However, none has integrated building and human analytics in predictive analysis. The major missing component of the study was how the collected data on environmental comfort can be used to improve the workplace environment.
The reviewed study had several shortcomings. The three IEQ elements of lighting, temperature and humidity were mostly within the recommended comfort limits for the entire duration of the study (Roskams and Haynes, 2019). This phenomenon could be a drawback because there was inadequate data to evaluate whether non-adherence to comfort boundaries leads to decreased levels of environmental comfort. Furthermore, all workplaces in the study area were similar in terms of floor plan designs and sizes, which reduced variations in workspace integration scores. Few observations were used in the statistical analysis, which could have interfered with the statistical power to detect significant effects.
Future studies could use larger sample sizes and investigate diverse types of workplaces to ensure more rigorous testing of hypotheses concerning the impact of various attributes of the workplace environment. Studies that involve the manipulation of environmental conditions could also be considered to determine the reliability of the predictive analysis in forecasting environmental comfort.
Reference List
Engelbrecht, H. and Abbaspour, N. (2017), ‘Demonstrating good practice in the safe operation of gas assets with predictive analytics’, The APPEA Journal, 57(2), pp. 437-439.
Lessard, L. et al. (2016), ‘Predictive analytics to support real-time management in pathology facilities’, AMIA Annual Symposium Proceedings, 2016, pp. 772-778.
Maheshwari, K.A. and Sivakumar, P.B. (2018), ‘Use of predictive analytics towards better management of parking lot using image processing’, in Hemanth, D. and Smys, S. (eds), Computational vision and bio-inspired computing, Springer, New York, NY, pp. 774-787.
Nag, P.K. (2019), Office buildings: health, safety and environment, Springer, Singapore.
Roskams, M. and Haynes, B. (2019), ‘Predictive analytics in facilities management: a pilot study for exploring environmental comfort using wireless sensors’, Journal of Facilities Management, 17(4), pp. 356-370.
Wazurkar, P., Bhadoria, R.S. and Bajpai, D. (2017), ‘Predictive analytics in data science for business intelligence solutions’, 7th International Conference on Communication Systems and Network Technologies (CSNT) conference proceedings, Maharashtra, India, pp. 367-370).