Summary
Species classification is a practice that has gained popularity over the last few years across various disciplines, especially in natural sciences. The classification is vital in that it helps us understand diversity and facilitate the proper identification of different organisms. Categorizing organisms using the classification method has also proven critical in better understanding complex organisms’ evolution. Technological innovation has made it easier for scientists to classify organisms in various ways. One of the technological innovations that have played a significant role in facilitating taxonomy is data mining, allowing scientists to extract and apply essential data about species using mathematical, statistical, and artificial intelligence methods.
Technology provides easy access to biological information about species, demonstrating their structural differences between the past and the future. Unlike human intelligence which is highly prone to mistakes, data mining is more accurate, reliable, and easy to use when communicating biological information. Mining data sets regarding the classification of iris flowers species will be essential in documenting the past, present, and future evolution of the flowers while providing an effective method of communicating this information to future scientists. Data mining will also prove critical in helping scientists understand the structural changes of the flower in its efforts to adapt to the changing information.
Problems and Opportunities
Data mining is increasingly becoming a common and valuable practice among scientists in different fields. The concept refers to the practice of exploring large blocks of data sets using machine learning techniques to extract data patterns, trends, and statistics (Roiger, 2017). The practice aims at discovering only the most meaningful patterns and trends to determine outcomes. Sowmya and Suneetha (2017) also defined data mining as how one identifies anomalies and correlations to predict an outcome. Therefore, the data that researchers discover using data mining processes must be usable and significant to the overall process. Data mining regarding the classification of iris flowers will be important in identifying the existing iris species, outlining the structural differences between the various species, identifying the possible causes for the differences, and underpinning potential future changes and causes of the flower’s evolution.
The iris flower is a genus that consists of various species with distinctive features that set them apart from other similar-looking flowers. However, pattern recognition through speech and images remains a significant limit in the flower’s classification (Choi et al., 2019). The availability of machine learning has facilitated the classification process. Scientists experienced breakthroughs when deep neural networks became applicable to extracting external features from labeled data (Choi et al., 2019). The usefulness of the neural networks in iris flower classification proves that data mining has been crucial in enhancing the classification of the flowers’ species.
Data mining will identify these different species and their distinctive features and structures while facilitating standard scientific naming. Some botanists say that the flowers have sepals, while others disagree with this claim. The naming will reduce miscommunication and potential faults between the scientists when discussing the iris flower classification. Mining the selected data will also create a variety of opportunities. For example, computer experts will have an opportunity to develop and create better classification methods for the iris flower. Future scientists can utilize these methods to improve classification or broaden existing knowledge about the known iris genus and species.
Data Preparation Questions
The quality of data that the researcher utilizes during data mining primarily determines the correctness and accuracy of the data set. Therefore, the data preparation process is a vital step in ensuring the quality of the data set and enhancing the efficiency of the data mining procedures. Data preparation involves cleansing, arranging, and pre-processing raw data to enhance its value by eliminating noise and correcting inconsistencies (Priyanka et al., n.d). Since humans complete the data entry process, the raw information is highly prone to errors. Therefore, the data preparation method seeks to remove these inaccuracies by making suitable corrections, reformatting data, and enriching data sets by adding the missing information. Different data mining methods may also require varying data sets, hence the need for data preparation to ensure that the available information is suitable for the chosen data mining technique. I observed that the answers and data preparation techniques changed with the selected technique.
There were missing values when I selected a different data mining procedure, which called for a different data preparation method. I retained instances of the missing values to enhance the quality of the raw data sets. Retaining the missing values allowed specific coding, hence enhancing target labeling. According to Che et al. (2018), retaining the missing values in time-series prediction and other tasks that involve data mining correlates with informative missingness. The correlation enhances exploiting the patterns and trends from the dataset. Although the work is limited, it enhances imputation and improves prediction performance (Che et al., 2018). Therefore, I did not delete the missing values, but I retained them for specific coding and dataset.
Assigning predetermined ranges to the numeric values proved critical in preventing typing mistakes. Thus, if a field requires values that do not exceed two digits, presetting this numerical value eliminates the risk of entering values that exceed the value. Grouping and coding the categorical variables to reflect a hierarchy is vital in the C4.5 data mining algorithm. Since the algorithm is a classifier that relies on predictors, it produces a decision tree consisting of the different levels of decisions for each action. The primary purpose of the decision tree is to provide an outcome or decision based on certain conditions. For example, certain conditions determine whether an iris flower falls under a particular species category. Grouping and coding the categorical variables to reflect the hierarchy are essential in demonstrating the steps that the algorithm takes to arrive at the final decision regarding the distinct species of the iris flower.
Methods
Data Mining Methods: Neural Networks and Decision Trees
Neural Networks
are common data mining methods in iris flower species classification. Neural network models are nonlinear data modeling techniques used in complex modeling relationships between inputs and outputs (Priyanka et al., n.d.). The model is widely applicable in data mining due to its robustness and scalability, which allows it to accommodate different types of data types. Despite the complexity of neural network structures, long training times, and result representation that proves hard to understand, scientists and researchers still prefer the technique due to its accuracy and ability to handle noisy data (Priyanka et al., n.d.). The network mimics the structure of the human brain and how the organ processes information. However, neural networks are biological tools rather than replicas of brain function (Priyanka et al., n.d.). Neural networks have been widely used in plant classification due to their accuracy in identifying the distinctive features of different species.
The neural networks have varied applications across different fields. Botanists and researchers use the technique in machine learning to recognize patterns, cluster, feature-mine, predict and classify species, while it also serves as an essential data mining tool (Priyanka et al., n.d.). One of the benefits of neural networks is that the user can train them to calculate weights and cumulative calculations through machine learning gradually. The neural network model comprises three primary features: the architecture, learning algorithm, and activation functions (Priyanka et al., n.d.). I would utilize this data mining technique to recognize and retrieve patterns and trends that exhibit associative information. For example, iris flowers that exhibit associative patterns would fall into the same category of either one species or genus. Contrastingly, I would classify flowers that do not exhibit similar associative features under varying taxonomy. Priyanka et al. (n.d.) also recommends neural networks for mining data with combinatorial optimization problems or exhibits noise and other ill-defined problems.
Decision Trees
Another standard classification method in data mining is decision trees, which are structural tools that resemble a tree. The decision tree consists of root, branches, and leaf nodes, which hold different information values depending on the classification. The values on the branches signify the outcome of an action, while the values in the root node symbolize the action done on the attribute (Lachowicz, 2019). The leaves symbolize class labels, which are the most superficial values or attributes that the scientist uses to determine the decision direction. In this case, a leaf holds the class label of the distinctive iris species. Decision trees, like neural networks, provide an easy way of classifying plants. The data mining techniques consist of an algorithm that works recursively at each node (Lachowicz, 2019). The recursive process aids the decision-making process while facilitating the development of an outcome.
The use of decision trees to classify iris flowers has been in existence for a long time in botany. Ronald Fisher was the first person to use the decision tree to classify the iris flower in 1936 (Lachowicz, 2019). Ronald’s classification distinguished the flowers using categories such as petals and leaves, width and length, and color. According to this classification, there are three significant types of iris flowers – Versicolor, setosa, and virginica (Lachowicz, 2019). These categories offer the broadest form of classification of the iris flower. Combining machine learning and decision tree technique helps further to categorize the iris flower into its unique species. This technique would be essential in developing a comprehensive database of the various iris flower species.
Algorithms Chosen
I selected the C4.5 algorithm, which generates a decision tree based on the raw data that the researcher feeds into the WEKA Explorer. The algorithm creates univariate decision trees that help in pattern recognition (Damanik et al., 2019). However, discovering these patterns requires the implementation of machine learning. Thus, the purpose of the algorithm is to teach the machine how to classify items by analyzing the data sets and instances whose class is known (Damanik et al., 2019). When an iris flower’s genus is known, the machine is taught to classify the flower under all iris flowers. If the flower contains distinct features like the shape and color of petals, the machine is taught to categorize it under a particular species. I selected the C4.5 algorithm because it is the most ideal in creating univariate decision trees. The algorithm also allows construction premises that enhance the classification of species. For example, items that fall within the same class are labeled in the leaf part of the tree, making it easier for the algorithm to calculate the potential attributes of each item (Damanik et al., 2019). Therefore, the C4.5 algorithm is crucial in creating the decision tree and classifying the species accurately. Using this algorithm also satisfies my need to explore a new method of interest in classification.
Using Prunning Parameters
Even well-prepared data may contain outliers that can adversely affect the data analysis outcomes. Therefore, pruning helps in identifying and clarifying such outliers, enhancing the quality of the classification. Pruning also enhances the definition of specific parameters that appear to differ significantly from others under similar classification. I used the specific pruning parameters to reduce classification faults and enhance generalization. I limited the activity to particular tests rather than nodes to avoid the classification errors in univariate decision trees. This strategy helped limit the extraction to specific parts of the tree, rather than focusing on the whole tree, which would create classification inaccuracies.
Results
Comparing Alternative Solutions
The literature revealed other alternative solutions to iris flower species classification, including the Recurrent Neural Networks, RNN algorithm. Che et al. (2018) investigated the application of the RNN algorithm in multivariate time series with missing values. Time series is a type of data mining process that we can use to explore classifying iris flower species. Unlike the decision tree, time series consists of data sets regarding a particular sample over some time. Therefore, time series enables the user to identify pertinent factors that influence the appearance of the data set. While the C4.5 algorithm utilizes machine learning to identify patterns and trends, the RNN algorithm relies on sequential data to show the data sequence over time. However, this alternative solution is most applicable in speech recognition and machine translation (Che et al., 2018). Therefore, it would not be ideal in species classification since the process requires specific botanical names.
Cases Identified Correctly
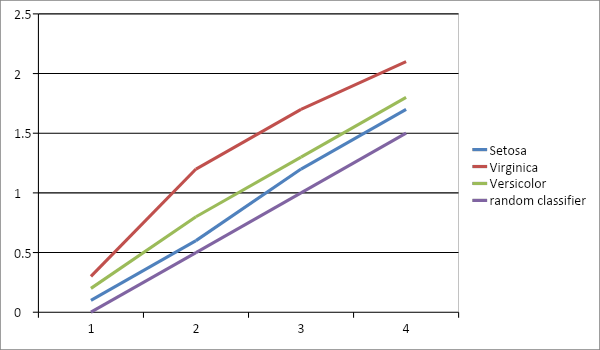
Superior Data Mining Method
The decision trees technique was the superior data mining method for the selected data set. Decision trees provide an easy method of classifying the iris flower species because it allows the user to define input parameters and the corresponding output. Therefore, this technique reduces the risk of misidentification and wrong classification. Decision trees also utilize the C4.5 algorithm, which is the most suitable for classifying taxonomical data like the different species of the iris flowers.
Comparison of Results from Different Recommendations
Alternative methods includes neural networks, hierarchical clustering, genetic algorithms, and time series, among other data mining methods. However, the results would be different from the expected outcome. For example, the time series would only show the changes and contributing factors over some time instead of classifying the species. Neural network models may provide classification, but the technique may compromise the accuracy because it selects the final output based on the highest value. Therefore, the decision tree is the most appropriate method of classification of the iris flower species.
Recommendations/Conclusion
Data mining is a vital aspect of the classification of organisms. Different data mining methods exist, but each method’s efficacy depends on the data sets and type of information that requires classification. Neural network models, decision trees, time series, association rules, regression, and genetic algorithms are examples of data mining methods that facilitate classification. Analysis of the literature revealed that the decision trees method is the ideal data mining procedure for iris flower classification. It is easy to use and has a higher rate of accuracy compared to the other alternatives. The literature also revealed that the C4.5 algorithm is the best for classifying the species. Therefore, I would deploy the decision tree technique and C4.5 algorithm to data-mine and classify iris flower species.
References
Che, Z., Purushotham, S., Cho, K., Sontag, D., & Liu, Y. (2018). Recurrent neural networks for multivariate time series with missing values. Scientific Reports, 8(1), 1-12.
Choi, Y. J., Lee, E. M., & Jeong, H. G. (2019). Design of a neural chip for classifying iris flowers based on CMOS analog neurons.Journal of Sensor Science and Technology, 28(5), 284-288.
Damanik, I. S., Windarto, A. P., Wanto, A., Andani, S. R., & Saputra, W. (2019). Decision tree optimization in C4. 5 algorithms using genetic algorithm. In Journal of Physics: Conference Series (Vol. 1255, No. 1, p. 012012). IOP Publishing.
Lachowicz, M. (2019). Decision tree approach for IRIS database classification. Institute of Mathematics, 1-5.
Priyanka, G. (n.d.). Neural networks in data mining. International Journal of Electronics and Computer Science Engineering, 1(3), 1-5.
Roiger, R. J. (2017). Data mining: a tutorial-based primer. Chapman and Hall/CRC.
Sowmya, R., & Suneetha, K. R. (2017). Data mining with big data. In 2017 11th International Conference on Intelligent Systems and Control (ISCO) (pp. 246-250). IEEE.