Introduction
The general population’s health is a significant concern not only because of the aims to reduce mortality and improve the well-being of individuals but also because of the risks correlating with a lack of effective policies. Currently, the opportunities to address such societal challenges are more extensive, so extra effort is needed to combat the crisis in which individuals cannot access the necessary physical and informational resources.
Obesity is one of the key concepts that have become a topic of discussion due to the increased rate of people who fall under the obese category based on the BMI chart. While obesity correlates with increased weight, the condition is linked to multiple health problems and limitations in regard to federal and state efforts to manage the health issue. Namely, inevitably, any health problem that affects a large number of the population is both difficult to address and costly in terms of resources. As a result, it is essential to understand the groups of people who are vulnerable to the condition, the causes, and the effects it generates. It is essential to mention that obesity is a complex concept that cannot be viewed from a narrow perspective.
Multiple factors lead to such health outcomes that are to be discussed in order for the risks to be minimized. Generally, increased weight is merely considered an outcome of inadequate dietary behavior in which an individual consumes more calories than required. On the other hand, such an outlook is limiting since it does not address the socio-economic implications of the lifestyles of individuals with obesity. Namely, living in a food desert, having a low income, not being informed on the topic, having a family history of obesity, and similar concepts may affect a person’s ability to avoid health concerns related to increased weight. Social aspects have also been linked to the prevalence of obesity or lack thereof. Research, however, is to examine the topic in-depth for a better understanding of the issue. For example, evidence shows that educated men are more likely to be obese, a situation contrasting with the female participants whose low education correlates with increased weight (Liao et al., 2018). The concept is yet to be identified in regards to the reasons why the disparities exist. Moreover, the same research has found a positive relationship between marital status and obesity. On the other hand, other researchers have provided evidence that low education is a facilitator of obesity in all demographics (Hsieh et al., 2020). Hence, married males and females are more likely to be classified as obese according to the BMI standards.
Similarly, researchers have also pointed out that low income and obesity are linked despite differences in demographics (Ogden et al., 2017). The different findings correlate with the idea that more research is needed to identify the links between the multiple aspects, which is a limitation that is to be combated. The concept is also yet to be examined in-depth, highlighting the gap in research on the topic. While the implications are clear, it is yet to be determined which exact factors are interconnected with vulnerability to becoming obese. On the other hand, evidence is clear that the challenge is complex, and considering it to be a monolith would imply a disregard for multiple factors influencing it. Thus, there are specific links between income, education, gender, age, knowledge, marital status, and similar characteristics with increased weight.
The current research aims to identify the factors that potentially lead to obesity. Hence, the research question is, “what are the individual characteristics and socio-economic traits that make individuals vulnerable to becoming obese? Moreover, how do knowledge of obesity as a condition and the risks linked to increased rate impact people’s decision-making? The questionnaires will facilitate a comprehensive conclusion on both questions and provide an overview of how people view obesity and the factors that may generate the outcome of increased weight. The initial set of questions, namely 14, is designed to determine patterns in obesity-related concepts such as marital status, age, income, education, etc. the second questionnaire consisting of 16 questions, will provide an overview of how people perceive obesity. As a result, the findings will highlight whether individuals who are informed are more likely to adhere to a healthy lifestyle.
The scope of the research is to determine the factors that may increase the risk of obesity. If said factors are identified, it can be less challenging to address the causes in order for effect to be avoided. Thus, in case a correlation between a lack of information and an unhealthy lifestyle is determined, an informational campaign linked to nutrition and sports can address the challenge. On the other hand, in case other factors such as the number of children, marital status, income, and education are found to be linked to increased weight, the challenge is to be addressed in a more complex manner. As a result, the condition will not be viewed as a choice, an unhealthy habit, or a portrayal of laziness. Instead, the medical outcome will become valid in regards to the multiple levels it has, including an individual’s mental health, family life, knowledge, opportunities, and living situation.
Materials and Procedure
The methodological basis for the present study consisted of a survey questionnaire administered to respondents to identify key trends and patterns associated with the development of obesity. Because the study assessed patterns prevalent among participants at a specific point in time, the study design was identified as quantitative and cross-sectional (Thomas, 2022). The experimental design of the study involved random sampling, which should minimize the effects of bias and bias in either direction of the results. The sample was formed by inviting independent, unfamiliar volunteers — the link to the online questionnaire was distributed via social media — to complete a survey questionnaire pre-created on the Google Forms website. It was not necessary to register on the platform or to enter any data that would unequivocally attest to the identity of the participant; in other words, even the author of this study does not know who exactly filled out the questionnaire.
The questionnaire consisted of 30 multiple-choice questions. This included eight sociodemographic block questions, six behavioral habits questions, ten questions exploring individuals’ awareness of the topic of obesity, and six questions related to the respondents’ environment and clinical characteristics. Responses to the questions were distributions ranked on a categorical nominal scale, which means they had to be coded appropriately before statistical processing. Participation in the questionnaire was implemented between June 8 and 10, 2022: these were the three days respondents could complete the online survey and send in their results anonymously. When responses were closed, the data were uploaded to an Excel file, followed by preparations for statistical analysis. More specifically, the preparation involved removing rows with blank or meaningless responses, allowing only valuable and meaningful results to be considered. After the extra rows were removed, the final sample size was reduced by 15%, from 23 records to 20. This file was successfully loaded into IBM SPSS v. 25, which was used to perform statistical analyses. Specifically, the analysis was implemented at two levels, descriptive and inferential.
Descriptive statistics involved measuring common patterns typical in the sample: mean, standard deviation (SD), and frequency table. In terms of inferential statistics, chi-square tests and nonparametric tests were used since the distributions did not demonstrate a tendency toward normality. A critical alpha level of.05 was used to test the statistical significance of all tests — accordingly, a calculated p-value above this value postulated the need to accept the null hypothesis.
The chosen method of data collection was justified by the desire to obtain real primary data that is responsive to the health agenda and the perception of obesity in the minds of the respondents. Additionally, the use of surveys allows for a cross-section of the sample and potentially uncovers unpopular but existing opinions and beliefs (DeFranzo, 2018). Using a demographic approach to data analysis also proves to be a useful strategy, as it allows one to explore the relationship between the factors being studied and demographic characteristics, even if no overall statistical significance was found for the entire sample.
The ethical considerations of the study included inviting voluntary participation in the test without affiliation or other inducements. Prior to taking the test, participants were asked to check a box in the informed consent box, confirming their honest intentions and introducing them to the basic meaning of the current study. In addition, the survey questionnaire included quite sensitive questions related to clinical history, illness, income, and medications taken. To ensure complete confidentiality of such data, no information directly or indirectly identifying the individual was collected. In other words, all responses were completely anonymous, which respondents were aware of before they were tested.
Results
Respondent Profile
In terms of sample demographics, most were represented by respondents between the ages of 18 and 49 (total: 90.0%, n = 18); in terms of gender, the majority were female (50.0%, n = 10), followed by male investigators (40.0%, n = 8) and non-respondents (10.0%, n = 2), as shown in Figure 1. Figure 2 shows the distribution of participants by marital status and number of children: most of the sample (40%, n = 8) were single, with half of the participants (n = 10) being childless. In terms of education, the majority of respondents (40%, n = 8) were in college but did not have a degree; with 50.0% (n = 10) of the sample stating middle-income (Figure 3). Finally, in terms of geographic and religious distribution, 30.0% (n = 6) of the respondents were from Europe-the same number of participants stated affiliation with Judaism (Figure 4). Overall, the profile of the respondents shows the impossibility of unifying the sample, as it was represented by completely different, at some points, diametrically opposed people in large numbers.
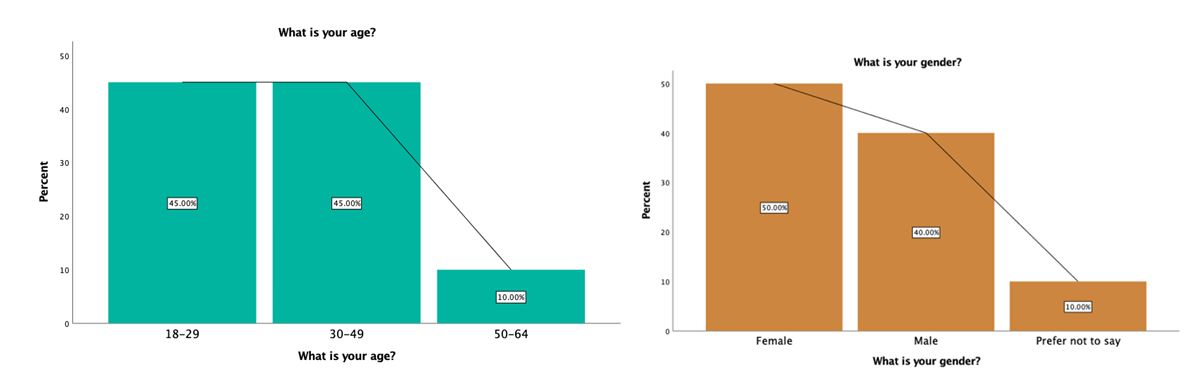
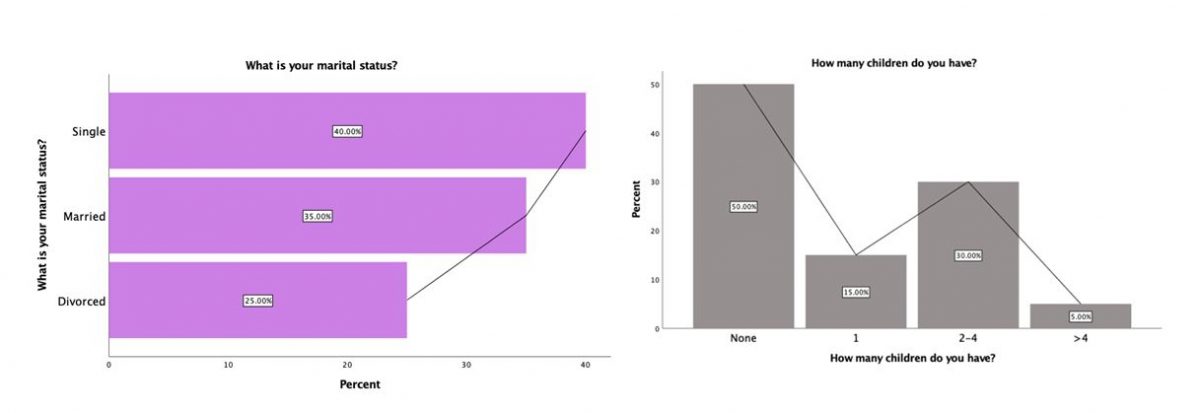
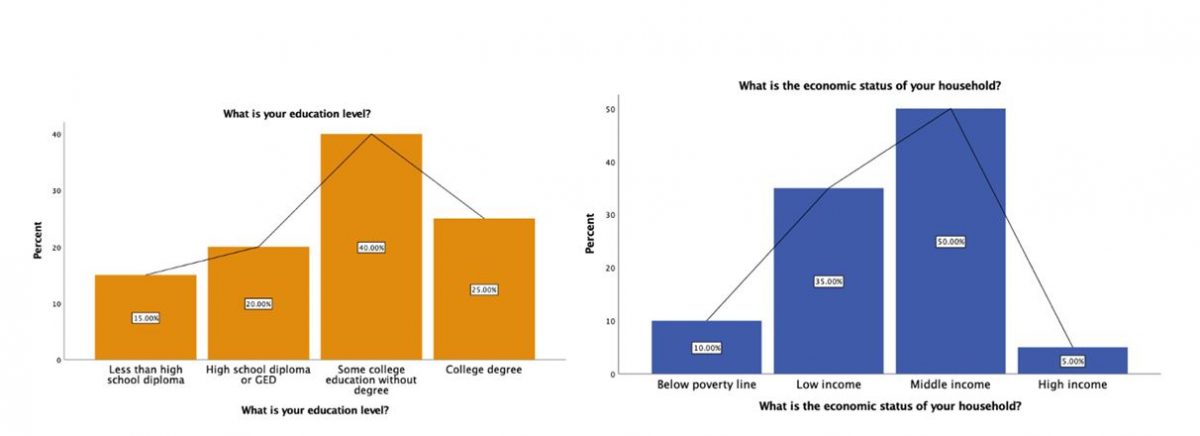
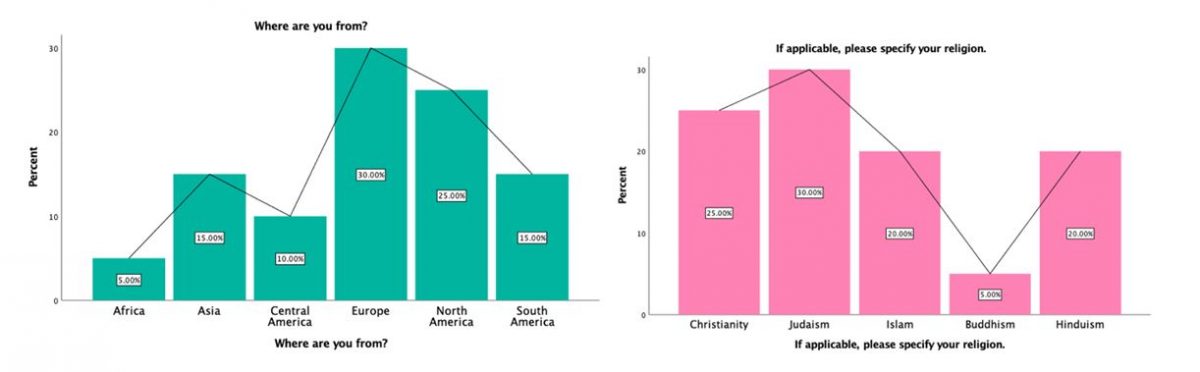
Frequency Distribution
An analysis of the trends prevalent in the sample was also conducted using frequency distribution studies. One of the most intriguing results of the sample collected was the identification that none of the respondents reported having health problems or taking medication — thus, the last two questionnaire questions were not meaningful for research because they did not provide diversity. Differences were found in the remaining questions. The general characteristics of the central tendency for each distribution, including the mean — if applicable — median, and index of distribution normality according to the Shapiro-Wilk test (van den Berg, 2022). The results showed that all variables were non-normally distributed, and thus the choice of parametric tests for their analysis was inappropriate. This included the use of a chi-square test for a single sample — this test was designed to check for equality of distribution within each variable. Based on the test results, it was determined that equality of distributions was not achieved in the following questions:
- Number of children (p =.027)
- Economic status (p =.013)
- Whether obesity leads to diabetes (p =.011)
- Does obesity lead to reproductive health problems (p =.022)
- Does obesity lead to cancer (p =.000)
- Exercise helps control complaints (p =.000)
- Need for weight loss (p =.022)
Chi-Square Criterion for The Independence of Categorical Variables
Because the distributions obtained were measured on a categorical nominal scale, in their case, using Pearson’s correlation and linear regression is invalid. For this reason, the chi-square test of independence of categorical variables was chosen to judge the effects of some variables on others (UDAYTON, n.d.). Since BMI was the only indicator of obesity in the sample, BMI is the dependent factor for evaluating the effects of the other variables. Table 1 below reflects the results of this test: as can be seen, none of the parameters had a statistically significant effect on the BMI choice. In other words, there is insufficient evidence that the choice of specific answers to the following questions had a statistically significant effect on the respondent’s BMI.
Table 1: Results of the Chi-Squared Independence Test for Categorical Variables
Nonparametric Tests
In addition, inferential statistics allow the use of nonparametric tests to assess the relationship between variables that do not exhibit a normal distribution. For example, the Friedman criterion allows assessing whether there are statistically different values between groups — it is analogous to a one-way ANOVA (Laerd Statistics, 2020). The Friedman criterion was applied to the demographic block categories concerning the respondent’s body mass index. Table 2 shows the results of this test: it can be seen that statistically significant results were found for some relationships. The values marked with an asterisk in Table 2 show that there are statistically significant differences in how respondents answered the BMI question for a particular variable. However, Friedman’s test, like the ANOVA, did not provide insight into exactly where differences exist within a single variable.
Table 2: Results of the Friedman Test with BMI as the Dependent Factor (* — p <.05)
Discussion
The central objective of this study was to determine the predictors associated with obesity and the perception of obesity in people’s minds. For this purpose, an experimental questionnaire survey approach was used, in which twenty individuals of different sociodemographic, cultural, and religious affiliations participated. Statistical analysis at the descriptive and inferential level was used to detect a potential relationship between predictors and obesity as measured by BMI.
The results of the statistical data processing allowed us to assess that the sample formed is multidisciplinary and cannot be reduced to a single respondent portrait. Although, as the analysis showed, some of the answers were chosen by the majority, there was quite a substantial discrepancy in the choice of the remaining options as well. In fact, this effect may be quite acceptable for academic practice, as this sample can be representative of different communities without being tied to specific characteristics. On the other hand, Friedman’s nonparametric test showed that eleven variables had statistically valid (p <.05) differences in BMI choices. In particular, respondents from different gender and age groups showed a difference in their choice of BMI. These findings can be compared to evidence from Liao et al. (2018), who showed that men and women are differentially prone to obesity. In terms of tying to the academic literature, both Liao et al. (2018), Hsieh et al. (2020), and Ogden et al. (2017) showed that education and economic status are significant predictors of obesity. In fact, the survey did not support these findings, as respondents, regardless of education and income, tended to answer the BMI question the same on average. This leads to the conclusion that education level and economic status are not sufficient predictors, and their association with obesity should be subject to a more thorough analysis.
Statistical processing also revealed that the propensity to be obese (BMI) varied according to what the respondents answered on the information block. In other words, different awareness of obesity affected obesity, but in the absence of an a posteriori test, it is not possible to determine what these differences were. This understanding is perfectly consistent with the finding in the Introduction that there is a need for additional testing. More specifically, the scale used measured variables only as nominal, which creates barriers to practical statistical analysis, including regression and correlation analyses. For this reason, the results of the present study do not allow for reliable causal relationships but instead indicate the location of statistically significant differences. A more in-depth study of the locations found will allow us to understand the nature of the differences in order to develop different strategies to address the problem of obesity in the population. Other limitations are the small sample size (n = 20) — despite the multisampling nature of the experimental group formed, the small size prevents normality of the distribution and creates the potential for reliability bias. For this reason, there is a need to expand the sample in the future and measure performance on ordinal (Likert scale) scales rather than nominal ones.
In conclusion, it is worth emphasizing that obesity is a severe challenge to the public health agenda and poses a threat to public health. Combating this problem should be a priority because obesity can be eliminated by taking the necessary measures to inform and prevent the population. In this paper, a survey was conducted to identify the critical predictors of obesity. It was shown that the propensity for obesity depends on gender and age, as well as the degree of awareness of the respondents about the problem. Future research should further explore these relationships and formulate causal associations for them.
References
DeFranzo, S. E. (2018). The 4 main reasons to conduct surveys. Snap Survey. Web.
Hsieh, T.-H., Lee, J. J., Yu, E. W.-R., Hu, H.-Y., Lin, S.-Y., & Ho, C.-Y. (2020). Association between Obesity and education level among the elderly in Taipei, Taiwan between 2013 and 2015: A cross-sectional study.Scientific Reports, 10(1), 1-9. Web.
Laerd Statistics. (2020). Friedman test in SPSS statistics. LS. Web.
Liao, C., Gao, W., Cao, W., Lv, J., Yu, C., Wang, S., Li, C., Pang, Z., Cong, L., Dong, Z., Wu, F., Wang, H., Wu, X., Jiang, G., Wang, X., Wang, B., & Li, L. (2018). Association of Educational Level and marital status with obesity: A study of Chinese twins. Twin Research and Human Genetics, 21(2), 126–135. Web.
Ogden, C. L., Fakhouri, T. H., Carroll, M. D., Hales, C. M., Fryar, C. D., Li, X., & Freedman, D. S. (2017). Prevalence of obesity among adults, by household income and education — United States, 2011–2014. MMWR. Morbidity and Mortality Weekly Report, 66(50), 1369–1373. Web.
Thomas, L. (2022). Cross-sectional study | definitions, uses & examples. Scribbr. Web.
UDAYTON. (n.d.). Using SPSS for nominal data: Binomial and chi-squared tests. UDAYTON Academic. Web.
van den Berg, R. G. (2022). SPSS Shapiro-Wilk test – quick tutorial with example. SPSS Tutorial. Web.