Introduction
The case study describes a situation pertaining to Regional Airline’s telephone flight reservation system. The case demonstrates an inbound call center. When a consumer calls the reservation system, the call is routed to an inbound call center (or more generally, contact center), a large, centralized pool of trained agents (contact center employees) who are qualified to address the customer’s inquiry. The management of Regional Airlines by policy believes that minimizing the cost of agents should be minimized.
Main body
As the utilization of the system is 0.8 for a single agent system, we see that calls arrive at the reservation system at some average rate. At any given time, a certain number of calls will be waiting in the queue (zero or more); the average number waiting is w, and the meantime that a call must wait is Tw. How is averaged over all incoming calls, including those that do not wait at all? The agent handles incoming calls with an average service time Ts; this is the time interval between the dispatching of a call to the agent and the departure of that call from the agent.
Utilization, r, is the fraction of time that the agent is busy, measured over some interval of time. Finally, two parameters apply to the system as a whole. The average number of calls resident in the system, including the calls being served (if any) and the calls waiting (if any), is r; and the average time that an item spends in the system, waiting and being served, is Tr; we refer to this as the mean residence time.
If we assume that the capacity of the queue is infinite, then no calls are ever lost from the system; they are just delayed until they can be served. Under these circumstances, the departure rate equals the arrival rate. As the arrival rate, which is the rate of traffic passing through the system, increases, the utilization increases, and with it, congestion. The queue becomes longer, increasing waiting time. At r = 1, the agent becomes saturated, working 100% of the time. Thus, the theoretical maximum input rate that can be handled by the system is:
λmax = 1/Ts
However, queues become very large near system saturation, growing without bound when r = 1. Practical considerations, such as response time requirements or buffer sizes, usually limit the input rate for a single agent to 70-90% of the theoretical maximum.
Considering the basic queuing system, customers who require service are generated over a period of time by input source. The customers enter the queuing system and join a queue. At a certain time, a member of the queue is selected for service and the other person gets disconnected from the connection, as the telephone system does not allow call waiting. In the case of the telephone system which does not allow waiting, we see that there would be 60 minutes of the total time available within a time period of 10 AM to 11 AM.
The agents talk for around 3 minutes with every caller. Considering that the management keeps only one agent to minimize the cost and there is no waiting system available in the telephone system then a single agent can take the following number of calls as shown in table 1. The table shows that there are 60 minutes available to the agent to take the calls. If the historic data shows that a mean of 1 call every 3.75 minutes then there are 16 calls that can be taken by the agent (assuming calls arrive at regular and constant intervals of 3.75 minutes.
The past data shows that there is a time of 3 minutes spent on every call by the agent on average. Then the total time spent on taking 16 calls is 48 minutes. Hence the time an agent remains idle is 12 minutes overall assuming customers call every 3.75 seconds. Hence, there are 0.75 minutes idle for the customer every 1 call.
Table 1: Assuming no waiting system is operational.
So analyzing this situation statistically we see that there is a definite number of calls that a single agent can take in a period of 1 hour. So to serve at least 85 percent of the customers would require a large number of agents as we assume there are a large number of customers. But then there would be few customers who will not be served. Hence an up-gradation of the system was required which helps in queuing the calls. Plotting a multi-agent queue we get the following figure.
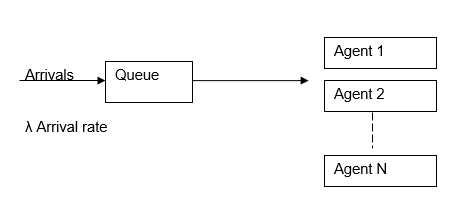
Here (figure 1) λ is the number of arrival per second which is 1/3.75 or 0.2666. Given the mean service time for each call i.e. Ts is 3 minutes. Assuming single server operational for the system, we see that the utilization of the system (ρ) is
Ρ = λTs
Hence, we see that the utilization of the system when the original system is operational is 0.8. Higher utilization rates imply longer delays in the queue, and in managing capacity, call centers trade-off resource utilization with accessibility.
Here it is important to provide a simplified description of the situation that the Vice president devised states that he wants a single agent to serve the customers with an upgraded system. Hence, the customers are more satisfied and the management can minimize the cost of agents for the reservation call center.
The telephonic reservation system for waiting line model assumes a Poisson arrival which meets the following requirements:
- The number of customers in the system is very large.
- The impact of a single customer on the performance of the system is very small, i.e. a single customer consumes a very small percentage of the system resources.
- All customers are independent, i.e. their decisions to use the system are independent of other users.
Assuming Poisson arrivals where the customers call in a sequence of events which are “randomly spaced in time.” Now considering the arrival of telephone calls at the airline call center, we see that:
- The total number of customers that are served by a telephone exchange is very large.
- A single telephone call takes a very small fraction of the system’s resources.
- The decision to make a telephone call is independently made by each customer.
Again, if not all the rules are met, we cannot assume telephone arrivals are Poisson. If the telephone exchange is catering to a few customers, the total number of customers is small, thus we cannot assume that rules 1 and 2 apply. If rules 1 and 2 do apply but telephone calls are being initiated due to some disaster, calls cannot be considered independent of each other. This violates rule 3.
In the current system, the caller gets disconnected as the line comes busy. There is no waiting facility provided in the current reservation system.
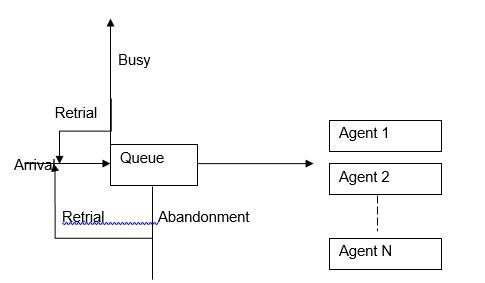
In figure 2 the queuing model follows the M/M/s queue, also known in call center circles as Erlang C. Queuing theory was conceived by A.K. Erlang at the beginning of the 20th century and has become one of the central research themes of Operations Research. In a queuing model of a call center, the customers are callers, the servers are telephone agents or communications equipment, and queues are populated by callers that await service.
The queuing model that is simplest and most widely used in call centers is the so-called M/M/N system, sometimes called the Erlang-C model1. Given arrival rate λ, average service duration µ–1 and N agents working in parallel, the Erlang-C formula, C(λ, µ, N), describes, theoretically, the long-run fraction of time that all N servers will be simultaneously busy, or the fraction of customers who are delayed in the queue before being served. In turn, it allows for the calculation of the theoretical distribution of the length of time an arriving customer will have to wait in a queue before being served.
The main aim of any call center is to achieve a certain service level, i.e., answer X% of calls within Y seconds, while minimizing overstaffing. The Regional Airlines reservation system aims to attain an 85 percent service level with minimizing cost by using a single agent. The simplest and most used performance model is the stationary M/M/s queue. It describes a single-type single-skill call center with s agents, operating over a short enough time period so that calls arrive at a constant rate, yet randomly (Poisson); staffing level and service rates are also taken constantly.
The assumed stagnation could be problematic if the system does not relax fast enough, for example, due to events such as an advertising campaign or a mew-product release. The model assumes out busy signals, abandonment, retrials, and time-varying conditions. The reason for using the M/M/s queue is of course the fact that there exist closed-form expressions for most of its performance measures. However, M/M/s predictions could turn out highly inaccurate because reality often violates its underlying assumptions, and these violations are not straightforward to model.
The most important of these assumptions is that the arrival rate obeys the Poisson distribution, which is equivalent to saying that the inter-arrival times are exponential, which is equivalent to saying that the arrivals occur randomly and independent of one another. This assumption is almost invariably made. Without it, most queuing analysis is impractical. With this assumption, it turns out that many useful results can be obtained if only the mean and standard deviation of the arrival rate and service time are known. Matters can be made even simpler and more results that are detailed can be obtained if it is assumed that the service time is exponential or constant.
As fewer agents cope with a given workload, operational efficiency increases. The latter is typically measured by the system (or agents’) occupancy, namely the average utilization of agents over time. Formally, it is de.ned as
ρ = λ / Nµ
(17) where λ is the arrival rate, E(S) = 1/µ is the average service time, and N number of active agents, either serving customers or available to do so. Thus, the sta.ng level – the number of available agents N – is required to calculate agents’ occupancy. Neither occupancies nor sta. ng levels are explicit in our database, however.
Now we consider single-agent queues as desired by the vice-chairman of Regional Airlines. The single-agent queue is the arrival rate is Poisson and the service time is general. When the standard deviation is equal to the mean, the service time distribution is exponential (M/M/1). This is the simplest case and the easiest one for calculating results.
Assumptions
- Poisson arrival rate.
- Dispatching discipline does not give preference to items based on service times.
- Formulas for standard deviation assume first-in, first-out dispatching.
- No items are discarded from the queue.
When the model followed is M/G/1 the arrival rate is Poisson and the service time is general. Making use of a scaling factor, A, the equations for some of the key output variables is straightforward. Note that the key factor in the scaling parameter is the ratio of the standard deviation of service time to the mean. No other information about the service time is needed. Two special cases are of some interest. When the standard deviation is equal to the mean, the service time distribution is exponential (M/M/1). This is the simplest case and the easiest one for calculating results.
Zero: This is the rare case of constant service time. For example, if all transmitted messages were of the same length, they would fit this category.
A ratio less than 1: Because this ratio is better than the exponential case, using M/M/1 tables will give queue sizes and times that are slightly larger than they should be. Using the M/M/1 model would give answers on the safe side.
A ratio close to 1: This is a common occurrence and corresponds to exponential service time. A ratio greater than 1: If you observe this, you need to use the M/G/1 model and not rely on the M/M/1 model. A common occurrence of this is a bimodal distribution, with a wide between the peaks. An example is a system that experiences many short messages, many long messages, and few in between.
For a Poisson arrival rate, the inter-arrival times are exponential, and the ratio of standard deviation to mean is 1. If the observed ratio is much less than 1, then arrivals tend to be evenly spaced (not much variability), and the Poisson assumption will overestimate queue sizes and delays. On the other hand, if the ratio is greater than 1, then arrivals tend to cluster and congestion becomes more acute. Given this problem, it is important to understand the single agent, though minimizes cost, reduces service level. And hence, we need to consider a system where more than one agent is operational.
The staffing proposition that we have is based on the argument that all calls are immediately answered because that is what we are aiming to achieve. The immediate-answer property means that the usual performance analysis concern about the impact of waiting before beginning service or blocking and retrials after blocking need not be considered; i.e., it suffices to use an infinite-agent model. In this context, the staffing requirement (number of required agents) in the near future can be divided into two parts:
- the number of current calls that will remain in progress in the future and
- the number of new calls that will arrive and remain in service.
Moreover, it is reasonable to regard these two components of future demand as being independent (and we do) and focus on them separately.
If the lead time (the length of the interval until the time for which the prediction is made) is larger than all but a few call holding times, then-current calls in progress will tend not to be significant. However, we are thinking of predicting for lead times less than many call holding times. As in the case of Regional airlines, the lead time is 3.75 minutes and the holding time is 3 minutes. But this holding time cannot be generalized as in many settings; there are different classes of customers with very different holding-time distributions. One class might have a mean holding time of 1 minute, while another class has a mean holding time of 3 minutes.
In order to determine the number of agents for the reservation system, we use the simplest deterministic model (Grassmann 1988). The simplest possible model is as follows: arrivals are deterministic, and the time between arrivals is 1/K. Similarly, there is a constant service time of l/jji. The problem is to find the best value for S, where S is the number of agents. To solve this problem, we assume that the profit per time unit is calculated as follows: Each of the S servers costs c per time unit to maintain, whether busy or not. In a deterministic setting, this cost is minimized if the number of servers is equal to the average traffic flow R = λ /x, rounded to the next higher integer.
Further human servers cannot operate effectively at utilizations approaching 100 percent. If the utilization must not exceed a percent, the least number of servers one needs to meet this requirement is 100R/a, rounded to the next higher integer. For instance, if the servers should not work more than 85 percent of the time, the minimum number of servers needed must be 100R/85 = 1.76R. Given 1/µ as the constant service time, i.e. 3 minutes, we see that the number of customers would minimize the cost of operation, R= 1/λ*3 i.e. 4. Hence, the number of agents required for the system would minimize cost is 7 (after rounding off). This clearly shows that the reservation call center requires more than 1 agent.
To forecast the traffic flow we make the assumption that the arrival rate λ is subject to a forecasting error. However, once X is given, the arrivals are still deterministic. Specifically, we assume that (Linder 1969, Grassmann 1988) X has a normal distribution. Consequently, R has a normal distribution as well, and E(R) and Std(R) are the mean and the standard deviation of R. Of course, if R follows a normal distribution, one always runs the risk that the number of servers cannot handle the average flow and that the queue will increase forever.
In practice, no queue will ever reach infinity. Normally, one of the following will happen: the day comes to an end, new arrivals balk or they are no longer admitted (this happens in banks and supermarkets), or new servers are added, or the setup is made more efficient, thus increasing the service rate. The problem is therefore to find the number of servers that minimizes the expected costs for a finite time span, say from 0 to T.
Since we are now looking at a time-dependent case, we have to decide how many elements are in the system initially. The simplest assumption is that the system starts out empty and that the first arrival occurs at time 0. The first departure will then occur at time 1/µ, and none of the λ/µ arrivals occurring during this period will leave before 1/µ. Consequently, the number in the system will increase at a rate λ until it reaches λ/µ, at time 1/µ.
Normally, the service time is short as compared to T, and this initial period can therefore be disregarded. After time 1/µ, the behavior of the system depends on whether R«S, or R>S. In the case R«S, the expected number of busy servers is S the number of a number of departures per time unit is Sµ, and the number in the queue at time t + 1/µ is t(λ-Sµ). If the period from 0 to 1/µ is again neglected, and if the queue length is initially 0, the length of the queue will be T(λ — Sµ) at time T. The average number in the queue is thus T(λ-Sµ)/2.
The recommendation we have for the reservation system of Regional Airlines is that they use a more general queuing formula, the service time often affects performance measures through its squared-coefficient-of-variation C2 = σ2/E2, E being the average service time, and σ its standard deviation. For example, a useful approximation for the average waiting time in an M/G/N model (Markovian arrivals, Generally distributed service times, N servers), is given by Whitt (1993):
- E [Wait for M/G/N] ~ E [Wait for M/M/N] × (1 + C2)/2
Thus, the average wait with general service times is multiplied by a factor of (1+C2)/2 relative to the wait under exponential service times. In fact, in the approximation above and many of its “relatives”, service times manifest themselves only through their means and standard deviations. Consequently, for practical purposes, if means and standard deviations are close to each other, then one assumes that system performance will be close to that with exponential service times.
However, for large call centers with high levels of agent utilization, this assumption may not hold: simulation studies indicate that the entire distribution of service time may become very significant (Mandelbaum and Schwartz 2002). While ours is a small call center with moderate utilization levels, its service-time distributions are, nevertheless, likely to be similar to those of larger, more heavily utilized systems. Thus, it is worthwhile investigating the distribution of its service times.
The important implication of the excellent to a lognormal distribution is that we can apply standard techniques to regress log (service time) on various covariates, such as time-of-day. For example, to model the mean service time across time-of-day, we can first model the mean and variance of the log (service time) across time-of-day and then transform the result back to the service time scale. Shen (2002)contains a detailed analysis of service times against other covariates, such as the identities of individual agents (servers), as well as references to other literature involving lognormal varieties. Let S be a lognormal distributed random variable with mean ν and variance τ 2, then Y = log(S) will be a normal random variable with some mean µ and variance σ2. It is well known that
ν = eµ+1 2б2
Conclusion
We use the above equation as a basis for our proposed methodology. Suppose we wish to estimate ν = E(S) and construct an associated confidence interval. If we can derive estimates for µ and σ2, then ν = eµ+1 2б2 will be a natural estimate for ν according to the above equation. Furthermore, in order to provide a confidence interval for ν, we need to derive confidence intervals for µ and σ2, or more precisely, for µ + σ2/2.
For our call center data, let S be the service time of a call and T be the corresponding time of day that the call begins service. Let n i=1 be a random sample of size n from the joint distribution of and sorted according to Ti. Then Yi = log (Si) will be the Log (Service Time) of the calls, and these are (approximately) normally distributed, conditional on Ti. We can.t a regression model of Yi on Ti as Yi = µ(Ti) + σ(Ti)_i, where _i|Ti are i.i.d. N(0, 1).
Works Cited
Grassmann, Winfried K. “Finding the Right Number of Servers in Real-World Queuing Systems.” INTERFACES 18: 2, 1988: 94-104.
Linder, R.W. “The development of manpower and facilities planning methods for airline telephone reservations offices.” Operational Research Quarterly Vol. 20, No 1, 1969: 3-21.
Mandelbaum, A., and R. Schwartz. Simulation Experiments with M/G/100 Queues in the Halfin-Whitt (Q.E.D) Regime. Technical Report, Technion, 2002.
Shen, H. Estimation, Confidence Intervals and Nonparametric Regression for Problems Involving Lognormal Distribution. Pennsylvania: Working Phd Thesis, University of Pennsylvania, 2002.
Whitt, W. “Approximations for the GI/G/m queue.” Production and Operations Management 2, 1993: 114–161.