Introduction
Recent years have seen a fresh wave of scientific and technical upheaval and industrial change as a result of the rapid development of artificial intelligence (AI) technologies. This cutting-edge technology has altered economic activity to a greater level than ever before by integrating with other technologies, such as big data, machine learning, and robot technology. The integration of AI and robot technologies into our everyday lives has been seen in several industrial settings, changing the character of corporate ecosystems. The Walmart chain of retail stores is one of the biggest investors in the adoption of robots to replace human expertise and labor. It has reportedly installed shelf-scanning robots in 50 sites throughout the United States (Graetz & Michaels, 2018).
These robots are mostly used to check inventory, pricing, and lost products. Worldwide use of robots is increasing, and the global robot market is growing and rising quickly. The international federation of robotics (IFR) reported that the number of robots is increasing internationally at a pace of roughly 7% each year (International Federation of Robotics, 2018). The research seeks to establish the effect of automation on development and employment. The rapid development, expansion, and adoption of robots around the world could results in increased economic growth and disrupt the job market.
The increase in robotization and automation in business contributes to its significant economic growth. According to a report from the international federation of robotics, the volume of robots is growing at a rate of about 6 percent per year globally (Eric et al., 2018). The global demand for robots is projected to grow at a rate of 12 percent per year between 2016 and 2019 (Borjas & Freeman, 2019). The adoption of roots will have a profound impact on global economic growth following the continuously advancing robotics development industry.
Significance of the Study
Due to the increased use of robotics and AI in the production industries, it is imperative to understand the contribution of mechanization in the economic world. Furthermore, anti-robotics campaigners are of the view that the latter decline in economic performance and leads to increased unemployment rates. The proposers of AI and robotics are of the view that robotics and automation increase production, saves time and investments, and, thus, have a long-term impact on economic growth. With the mixed understanding, it is imperative to undertake the present research to understand the relationship between robotics used in production and manufacturing and long-term economic growth. The study’s findings will be pivotal in developing technological policies that will see improvements in AI tools, such as robotics, or regulating the use of the latter in manufacturing and production industries. Additionally, manufacturers and producers will also gain knowledge from this study on how to implement optimal robotic and AI tools to enhance per capita income while at the same time retaining the labor force.
Study Objectives
- To determine the relationship between robotics and per capita output as mediated by machine learning ability.
- To determine the relationship between robotics and per capita output growth rate as mediated by savings invested.
- To determine how robotic and labor learning abilities can influence output growth rate.
Literature Review
Several studies have sought to fathom the impact of the adoption of robots on development and the job market. Some empirical studies back up the claim that advances in information and communication technology have led to greater worker productivity in the context of study into the economic effects of technological progress (Fernald & Jones, 2018). Further reading may be found on the topic of maximizing the benefits of IT to boost business effectiveness. However, it was gloomy about the long-term growth of the United States’ real GDP per capita and the possible productivity increase resulting from technological advancement would decline (Merola, 2022). Economists naturally begin to concentrate on the positive benefits of robots on economic growth as more and more robots are introduced into production for productivity increments and cost savings.
Studies associate the development of the United States with rapid industrialization and technological advancement. According to theoretical models, education and research and development (R&D) spending have been the two primary drivers of economic growth in the United States during the last several decades (Artuc et al., 2022). However, when these drivers begin to weaken, economic growth in the United States is expected to decline. Nevertheless, using AI and robotics raises crucial questions concerning economic development (Akar et al., 2022). Future expansion may be facilitated by the increased use of AI, which will raise the probability that robots may eventually replace human labor.
Intelligent robots and the youthful unskilled work population are in a mutually substitutive relationship, whereas the older skilled labor class is a complement to robots. Young workers find it harder to save money and make long-term investments as their incomes decline. Classifying workers into high-tech and low-tech categories represent the intelligent machine as a mix of capital and code. In particular, a high-tech workforce that can generate codes (Goel & Gupta, 2020). In his analysis, Bordot (2022) finds that introducing robot workers will eventually lead to lower labor costs. In this scenario, the rise of robots exploits a portion of consumers’ income, leading to increased gross demand in the future that cannot be met by decreased aggregated demand.
While there has been some discussion of robots’ effects on the job market and economic growth, most of these studies treat robots as a replacement in manufacturing. The majority of these analyses do not include robot features in their framework. Based on the present trajectory of AI research, some hopeful experts predict that by 2029 AI will be more intelligent than humans (Bordot, 2022). Among impartial experts, there is a 90% chance that AI will surpass general human intelligence in this century (Gasteiger and Prettner, 2022). By using machine learning technology, robots will likely exceed human capacity for self-learning in the following decades, and they will have mastered all knowledge humanity has accumulated, including pattern recognition and problem-solving.
Empirical Findings
Theoretical Model
In developing our theoretical model, we considered the following two primary aspects of robots used in manufacturing. The first is robots’ potential to displace human workers and their unique capacity for machine learning, which sets them apart from human laborers. In this study, a model for economic expansion that considers these two aspects of robots is built. The Prettener model is a standard for evaluating our efforts (Prettner, 2019). Many current investigations into the feasibility of integrating robots into production are predicated on the assumption that robots can be used as a stand-in for human workers. For instance, Bordot (2022), who developed his development model as an extension of Solow’s, classifies the production components used in manufacturing into three broad groups: human labor, mechanical labor, and fixed capital. His model’s production function looks like this Cobb-Douglas one:
Y1=A1 (L1=R1) αk11-a
All symbols used in our model are included in Table 1 of the supplemental material. Workers and capital are imperfect replacements in this production function, whereas workers and robots are fully interchangeable (Mitra & Das, 2022). Expressly, Prettener’s model assumes that technological development is entirely exogenous. Our model is an extension of the abovementioned model, with all other assumptions held constant. Our model’s output function is shown in Eq 2.
Yt= (ARtRt +ALtLt) αKt1-a
Table 1 displays the notations used in our model. In this study, we take it for granted that the robot can pick up new skills automatically. The robot’s productivity will skyrocket as data and resources are amassed (Lankisch al et., 2019). ARt takes its shape from the following, which is based on the “Learning by doing” principle of endogenous development theory:
ARt = BKtθ, B>0, θ >0
Further, we anticipate that the rate of machine learning in robots will be far higher than that of the human workforce. Specifically, ALt would be written as:
Alt =CKtʎ, C > 0, θ > ʎ > 0
Θ > ʎ shows that the robot is more adept at picking up new skills than human workers. Our model’s resultant function of output is hence.
Yt = (BKtθ Rt + CKtʎ Lt) α Kt1-α
Table 1 Model notations.
From the theoretical model developed above, three assumptions were derived considering the impact of automation and robotization on long-term economic growth.
Proposition 1
The first proposition was that when all parameters remain constant, there is an increasingly concave output with the robotics inputs as illustrated below;
Summary of proposition 1:
∂Yt/∂Rt = αBK tθ+1−α(BK tθRt+ CK tλLt)α−1 > 0, (B-1)
∂2Y, α−2
t/∂R = α(α− 1) B2Kt2θ+1−α(BK tθRt+ CK tλLt) < 0, (B-2)
since 0 < α < 1. Proven.
In this case, increasing robotics input will lead to increased output. However, the marginal output will decline, indicating that robots have an optimal investment level in manufacturing and production processes.
Proposition 2
The second proposition is that the learning ability of labor and robots predicts the return gap between robots and labor. In this case, when there is a substantial learning ability of robots than labor, the return gap between robots and labor increases as the capital increases (Sedik & Yoo, 2021). On the other hand, if the learning ability of labor is more potent than that of the robots, then the return gap between labor and robot decreases with the increase in the capital as illustrated in the model below:
Summary of proposition 2:
wR/wL = B/Ct*Kθ−λ. (B-3)
When better learning is seen in robots than human labor, i.e., θ – λ > 0, wR/wL ≥ 0. When human labor is better at learning than robots, that is., θ – λ < 0, wR/wL < 0. Proven.
Data Analysis
Numerical analysis was performed to strengthen further the theoretical models developed and the propositions suggested in line with the impact of automation and robotization on long-term economic growth. Data was collected on parameters aligned with the machine-learning capability of robots (Acemoglu et al., 2020). The parameters were then used to determine the long-term economic growth rate using the varied learning abilities of robots and the varied total savings acquired from the robots. The values on robots’ machine learning capabilities were obtained using the parameters modeled in the theoretical, empirical equations above (i.e., n, s, α, δ, sr, θ, λ, B, C, and t) (refer to table 1. for what the symbols represent in the model).
Comparative Analysis
A comparative analysis was performed between the learning ability of robots to illustrate long-term economic growth. In this case, long-term economic growth was demonstrated using the different learning capabilities of robots and the total percentage of savings acquired from the robots owing to their learning ability. Table 4 below illustrates the findings.
Table 4 Summary of comparative analysis.
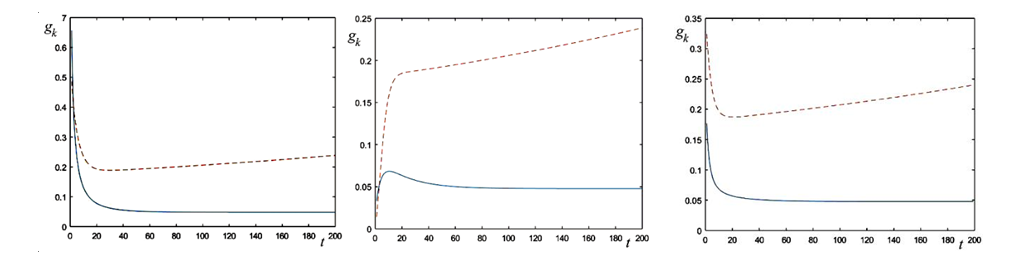
The first graph illustrates the growth rate of capita per capita, while the second graph shows the growth rate of robots per capita. The third graph shows the growth rate of output per capita. The imaginary lines presented in the graph depict a strong learning ability of robots than that of human labor, while the continuous line depicts a situation when there is no machine learning ability in robots; that is, human labor has a strong learning ability than that of robots (Klarl, 2022). The analysis confirms proposition 3, illustrated in the previous section.
Correlation Analysis
A correlation analysis was performed to determine the relationship between machine learning ability and per capita output growth rate. Figure two below illustrates the findings:
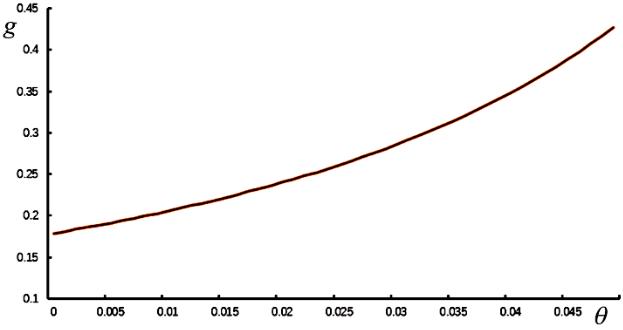
The analysis indicated a positive relationship between machine learning ability and per capita output growth rate. It shows that the per capita output growth rate increases as machine learning gets stronger in robots (Buchholzer, 2022). Consequently, it is projected that the learning ability of robots will keep increasing in the future owing to the increasing advancements in technological trends and artificial intelligence, which will lead to more long-term economic growth.
A correlation analysis was also performed between the total saving invested (in percentage) and the per capita output growth rate. The findings are illustrated in figure 3 below:
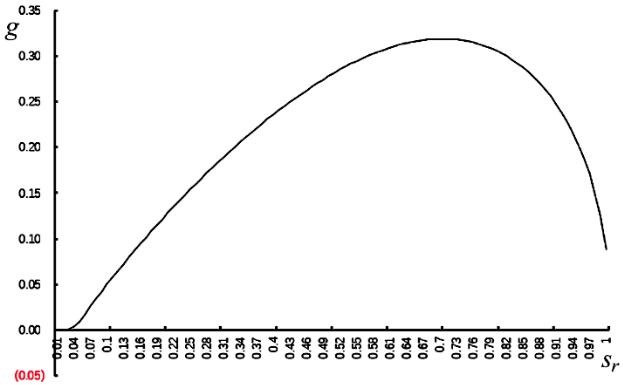
As indicated in figure three, there is an optimal investment ratio in robots to maximize the long-term output per capita growth rate. The findings confirm proposition one in the theoretical models (Ray & Mookherjee, 2022). It is imperative to note that machines such as robots are not always better, if not 100%. In this case, therefore, it is advisable to not only focus on increasing robotics investment but also emphasize robots’ learning ability.
Discussion
From the study, it was realized that not only do we recognize the robot’s potential to reduce the need for human work, but we also recognize the fundamental ways in which it differs from both human labor and conventional forms of capital (International Federation of Robotics (IFR, 2018). We think that artificial intelligence (AI) technology applied to the robot gives it the capacity of machine learning, which may one day make robots more productive than humans (Grau, 2022). It allows the robot to learn from experiences and improve its performance over time. Incorporating this fundamental distinction between robots into our theoretical framework was a natural next step.
There is a positive correlation between robots’ capacity for learning and the pace of increase in production per person; there is an optimum ratio of robot investment that maximizes long-term increase in production per capita (Graetz & Michaels, 2018). Powerful new tools have emerged in several disciplines thanks to advances in artificial intelligence and robotics. For the industrialized world, using robots creates ideal circumstances for “reindustrialization,” reshaping national manufacturing competitiveness and developing innovative sectors. For developing nations like China and other growing economies, this means new chances and difficulties as they work to preserve their competitive manufacturing edge, modernize their industrial base, and maximize the efficiency of their workforce. The research suggests that putting robots to work might help the economy. Nevertheless, it is not always the case that robots are better than human power. Executives should not just ramp up spending on robots. Improving robots’ capacity for learning is also crucial.
Conclusion
The primary aim of the present study was to understand the impact of automation and robotization on long-term economic growth. It was noted that automation and robotics positively impact long-term economic growth, as illustrated in figures 2 and 3, as well as the theoretical propositions and models. Global economic production is expected to increase with the adoption of robots and automation in various industries. The influence of robots on jobs remains unclear with the current data and analysis. However, it was noted that robots could not be 100% better as they have their optimal production threshold. It means that more focus should not be on investing in more robots, but the emphasis should be placed on the learning ability of the equipment as well as their quality. An increase in robotic and labor learning abilities has a direct and positive influence on economic production and growth rate.
References
Acemoglu, D., & Restrepo, P. (2020). Robots and jobs: Evidence from US labor markets. Journal of Political Economy, 128(6), 2188-2244. Web.
Akar, G., Casalone, G., & Zagler, M. (2022). You have been terminated: Robot taxation and the welfare state. WU International Taxation Research Paper Series, 2022-05, 1-16. Web.
Artuc, E., Bastos, P., Copestake, A., & Rijkers, B. (2022). Robots and AI. Routledge.
Bordot, F. (2022). Artificial intelligence, robots, and unemployment: Evidence from OECD countries. Journal of Innovation Economics Management, 37(1), 117-138. Web.
Borjas, G. J., & Freeman, R. B. (2019). From immigrants to robots: The changing locus of substitutes for workers. RSF: The Russell Sage Foundation Journal of the Social Sciences, 5(5), 22-42. Web.
Buchholzer, M. G. J. (2022). Value-ADDED Automation, a Solution for the Future of Work in Automotive Manufacturing in Romania. Revista de Management Comparat International, 23(1), 101-111. Web.
Eric, E., Aldo, G., Marco, G., & Massimiliano, N. (2018). Mapping the evolution of the robotics industry: A cross country comparison. The University of Turin.
Fernald, J. G., & Jones, C. I. (2018). The future of US economic growth. American economic review, 104(5), 44-49. Web.
Gasteiger, E., & Prettner, K. (2022). Automation, stagnation, and the implications of a robot tax. Macroeconomic Dynamics, 26(1), 218-249. Web.
Goel, R., & Gupta, P. (2020). A roadmap to industry 4.0: Smart production, sharp business, and sustainable development. Springer, Cham.
Graetz, G., & Michaels, G. (2018). Robots at work. Review of Economics and Statistics, 100(5), 753-768. Web.
Grau, R. M. A. (2022). International conference on inclusive robotics for a better society. Springer.
International federation of robotics (IFR). (2018). Robots and the Workplace of the Future. Web.
Klarl, T. (2022). Fragile robots, economic growth, and convergence. Economic Modelling, 112, 105850. Web.
Lankisch, C., Prettner, K., & Prskawetz, A. (2019). How can robots affect wage inequality?Economic Modelling, 81, 161-169. Web.
Merola, R. (2022). Inclusive growth in the era of automation and AI: How can taxation help?Front. Artif. Intell, 5, 867832. Web.
Mitra, S., & Das, M. (2022). Exploring the impact of robotization on economic development. International Journal of Economics, Business and Management Studies, 9(1), 13-27. Web.
Prettner, K. (2019). A note on the implications of automation for economic growth and labor share. Macroeconomic Dynamics, 23(3), 1294-1301. Web.
Ray, D., & Mookherjee, D. (2022). Growth, automation, and the long-run share of labor. Review of Economic Dynamics, 46, 1-26. Web.
Sedik, T. S., & Yoo, J. (2021). Pandemics and automation: Will the lost jobs come back? International Monetary Fund.