Abstract
Managing people is one of the most complex activities that leaders have to undertake on a regular basis within an organizational setting. It takes time to recruit and train employees who can meet organizational goals within their departments. It is important to ensure that once such talents are recruited and trained, they should be retained and constantly motivated to improve their performance. In this study, the researcher focused on determining how machine learning and big data can be used to enhance the work of managers. The study relied on two main sources of data. Secondary data was obtained from books and relevant online sources.
Primary data was collected from sampled respondents. Primary data was analyzed both qualitatively and quantitatively to respond to the research questions. The primary and secondary data both demonstrate the importance of machine learning and big data in enhancing the role of managers in the modern business environment. Managers find themselves in situations where they have to process large data and come up with decisions within a limited time. Using the machines has made it easy to make such decisions and to predict the future with higher accuracy than before when the tasks were done by people. The study shows that although some managers are still reluctant when it comes to embracing this new approach to management, machine learning is a very promising area in the field of management that organizations cannot ignore.
Introduction
Machine learning is an emerging concept in the field of big data that is increasingly becoming important in management. According to Murphy (2012), the current business environment is very challenging. People in the management positions find themselves in very delicate positions where they have to deal with both internal and external forces. External forces such as market competition, changing customers’ demands, inflation, and government policies have a direct impact on a firm’s ability to achieve success. Internal forces relating to employee management, operational management, and policy implementation also need the attention of the managers.
As Raschka (2015) observes, management is becoming a more challenging occupation because some of the strategies that managers used traditionally may not be applicable in the current business environment. In the past, managers had a near absolute power when coordinating the work of the employees. The coercive approach to management was a popular and the employees had to follow the directives of the managers without questioning their rationale. However, that is no longer the case in the modern business environment. According to Marsland (2015), stiff competition in the market forces firms to ensure that they hire and retain top talents. Such top talents cannot be retained in a dictatorial regime if they can be hired at other firms. It forces managers to embrace consultative management approaches that involve active engagement of the employees. It means that managers must be very accommodative and understanding of their employees.
Machine learning is a promising field that may help in solving various management problems in the modern business environment. Alpaydin (2016) says that modern-day managers must understand how to embrace diversity in culture and views of their employees. Using machine learning, Bell (2015) says that it is possible to improve the performance of the employees through the creation of an environment that is highly accommodating. Instead of the manager struggling to understand specific needs of individual employees, technology can be used to capture and analyze these diverging needs. After analysis, the machines can help in the development of the most appropriate governance model that should be used.
The model must take into consideration the strategic goals of a firm and the manner in which the current workforce can be used to achieve these goals. Hackeling (2014) says that through such strategies, managers can find it easy to coordinate the activities of the employees. This technology can also be used in improving the skills and capabilities of the employees. Given the fact that such technologies can be used to compare the capabilities of individual employees with their skills, the analysis can help in determining the gap. It can also propose ways through which such gaps can be addressed to ensure that the skills of the employees are aligned with the job requirements. In this paper, the researcher seeks to analyze the concept of machine learning, and the manner in which, it could be used to improve employee engagement and ultimately the performance of an organization.
Rational for the Study
In the current competitive business environment, companies cannot afford to make constant mistakes. Achieving perfection is critical when it comes to managing challenges in the external environment. In such a highly disruptive external environment, managers still have to deal with very demanding employees who must be handled with care to ensure that they do not consider moving to other companies. Management role has become so complex that success is not guaranteed even among the most knowledgeable and experienced individuals. Alpaydin (2016) says that even in such a challenging environment, firms must find ways of achieving success. Machine learning promises to solve serious managerial problems that firms are currently facing. The need to understand the capabilities of individual employees accurately and their needs cannot be done appropriately by a manager. However, using information technology, it is now possible to understand areas of improvement that each employee needs.
The machines are becoming superior management tools with capabilities that are beyond that of a human being. It is true, as Camastra and Vinciarelli (2015) put it, that it may not be possible for the machines to replace people completely in the management role. However, it can make the work simple and more accurate in the current challenging business environment. Machine learning can enable the managers to understand their current environment and accurately predict the future in a way that would enhance the success of the organization. That is why this research is very important. It will explain how modern-day managers can increase their reliance on information technology to enhance their managerial functions. It will explain the new role of managers in an environment where technology has become a critical component of management.
Research Questions
When conducting research, it is important to focus on the goals that must be achieved by the end of the study. Coming up with clear research questions makes it possible to define the research focus. It acts as a constant reminder to the researcher about the data that should be collected and analyzed. It also eliminates cases where a researcher would collect massive amounts of data partially related or completely unrelated to the research topic. The following are the research questions that the study seeks to answer:
- What are the main challenges that managers face in coordinating and controlling the activities of the employees?
- How can companies use the concept of machine learning to improve employee engagement, performance, and motivation?
- How can managers use machine learning to improve their performance?
Theory and Hypothesis
Background
Employees are very critical component of an organization. In fact, Hackeling (2014) says that employees are the wheels of an organization without which it may not achieve the desired goals and objectives. Once the top management has formulated strategic goals and objectives, the mid-managers will translate them into actionable goals to be assigned to the employees. Success of employees in meeting those goals defines how successful an organization would become. Unlike in the past when large corporations monopolized the market, Alpaydin (2016) says that today companies are struggling to manage stiff competition because of the liberalized global market. Customers understand that they have several options to choose from whenever they want to purchase a given product.
As clients become more demanding than they were before, firms find it necessary to improve their operational strategies. They have to ensure that they deliver improved quality products at lower costs than they previously did. They have to embrace efficiency to achieve sustainability in such challenging business environment. Successful firms have learnt how to empower their employees so that they can help in delivering the desired value (Camastra & Vinciarelli, 2015). Managers have to learn how to streamline skills of the employees with job requirement. These employees must also remain regularly motivated to improve their level of performance. It explains why the role of employee management has become a critical area that leaders must take seriously.
People management is not as easy as it used to be in the past. Marsland (2015) says that managers find it difficult maintaining their employees constantly motivated. The problem is caused in part by the diversity in most of the current workplace environment. In large organizations, which employs hundreds of employees, managers cannot give their workers personalized attention on a regular basis. It means that understanding their unique needs and capabilities is not easy. Factors that may be motivating to a section of the employees may not be the same as those preferable to the other section. Bell (2015) says that satisfying the generation Y and millennial employees is posing another serious challenge to the current managers. These employees are very demanding and do not hesitate to move from one organization to the other at the slightest provocation. It means that a firm may invest heavily on training an employee only for him or her to move to another organization with the new skills learned. Hackeling (2014) says that whenever a firm commits its resources to train an employee, it must be capable of retaining him or her for a considerable period to get the returns on the investment. The realities that modern-day managers face have made it necessary for them to look for how technology can be used to improve their performance.
According to Pentreath and Paunikar (2015), the concepts of big data, artificial intelligence, and machine learning have been in existence for several decades. However, more than before these concepts have become very relevant today. The challenges that managers face may not be addressed effectively without the assistance of technology. The decisions that they make have a significant impact on their organization. These managers cannot afford to make decisions that fail to deliver desired results. As such, their decisions must be based on facts. They need information that can help them understand a given pattern. They can then use such patterns to make a decision, having the knowledge of the possible consequences of their choices. Developments made in the field of information technology have improved data collection, processing, storing, and sharing whenever it is necessary.
Managers can now access relevant information that can inform their decisions and guide them whenever it is necessary to make choices. As Alpaydin (2016) explains, machines are not replacing managers. They are only taking over the role of decision-making when such decisions must be based on data that has to be processed and interrelated. The machines are reducing and in some cases, eliminating decisions made without understanding the possible outcome. It creates a scenario where all the possible outcomes for each strategic choice made are made clear before one of them is chosen. It makes it possible to know the most desirable option based on the organizational goals and the prevailing forces. Hackeling (2014) argues that such systems improve efficiency in decision-making, especially when one is presented with statistics that may influence the future of a firm.
Literature Review
In this section of the report, it is important to focus on reviewing what other scholars have found out in this topic. Machine learning is becoming a critical area of research as organizations try to come up with unique ways of achieving success. According to Sugiyama and Kawanabe (2012), the responsibility of managers is increasingly becoming complex in modern organizations, and it is necessary to find ways of making it more effective. Machine learning promises to solve most of the problems that managers face when it comes to making decisions about the future based on large data. Looking at what scholars have found out in this topic will help in identifying research gaps and coming up with hypotheses, which can be analyzed using primary data collected from the field.
Understanding the Concept of Big Data
According to Marsland (2015), big data refers to “a set of data that is so complex and voluminous that it cannot be dealt with using traditional data processing applications.” Data can be classified as such based on three premises, which are the volume, variety, and velocity (Christiano & Zhao, 2016). When the volume of data is very large, then it may not be possible to analyze it using traditional methods. For instance, a company Abu Dhabi National Oil (ADNOC) has over 25,000 employees whose activities must be monitored on a daily basis. Information about the daily performance of each of these employees is very voluminous and a manager using analogue systems cannot collect and analyze it in time to make a critical decision. In fact, it may not be easy to collect data from such employees manually. Collection of such data will need a digital system that is computerized to capture their performance based on the set goals and objectives. Such a manager will need assistance to summarize the daily and monthly performance of each of these employees in a digital format. It is only through that approach that it will be possible to have an accurate understanding of the performance of these employees. At that point, the manager will have a clear picture of the employees who are underperforming and a decision can be made on what needs to be done to address the weaknesses.
Variety is the second factor that defines how big a given data set is within an organization. Large multinational-corporations such as General Electric and Samsung that operates in numerous industries across the world receive a wide variety of data on a daily basis. In most of the cases, the top management unit may be needed to make decisions about the wide variety of data presented to them (Cleophas & Zwinderman, 2015). It may be information about employees, customers, shareholders, strategic partners, competitors, suppliers, government agencies, environmental watch groups, or any other relevant party. The management unit may have a short time to process and respond to the needs in each of these areas. Their decision may have a significant impact on the overall performance of the firm. It is not humanly possible to process such a variety of data and make decisions within that short period. Even if the top management engages their junior officers, serious mistakes cannot be avoided if it is done manually. The digital technology platform makes it possible for these managers to have such data processed within a short time (Zhang & Ma, 2012). By stating the possible choices that have to be made, the manager will be able to predict the consequence of each action plan. It makes it possible to know the most appropriate approach that should be taken within such a short period.
The third factor that makes a set of information be classified, as big data is velocity. The speed with which new information streams in and the urgency with which they have to be processed defines how the management must act (Dehmer & Basak, 2012). Large corporations such as ADNOC constantly have to deal with various issues in its many departments. For instance, customer requests that this firm must deal with comes in very high velocity. It is the responsibility of the sales department to ensure that information coming from the clients is processed and acted upon within the shortest time possible. The information may be about changes in the order, complaint about a previous order that needs to be addressed, cancellation of an order, creation of new order, existence of a new product in the market that is affecting sales of the company’s product, or any other relevant information.
The sales manager must process and act upon that set of information streaming in at high speed within the expected time. It is not possible, even when dealing with the most effective manager, to deliver the expected outcome without the help of technology. It requires the manager to use information technology to processes such data, and give necessary directives on how decisions should be made to address the concerns raised. Bell (2015) warns that when handling customer needs, efficiency and effectiveness are critical factors that cannot be ignored. The speed with which an issue raised by a customer is addressed determines whether they will remain loyal to the firm. Marsland (2015) says that it is not just about a speedy response to the issue raised. The response should be in line with the expectations of the customer for them to see that their needs are addressed.
According to Hassanien and Gaber (2017), big data is gaining popularity in predictive analytics. The market has become very unpredictable as emerging technologies continue to be disruptive in the normal operations of companies. When planning, Alpaydin (2016) says that a number of assumptions are always made about the future. These assumptions must be made because at the time of planning, it is not clear what shall become of the future environment within which a firm operates. The assumptions that a company makes defines the approaches and strategies that it will embrace. If wrong assumptions are made, it is likely that the strategies proposed may fail to yield the desired outcome. In such cases, sustainability of such an organization may be seriously compromised.
It means that managers cannot just afford to make assumptions without any proper basis. The assumptions must be based on a given pattern and must hold true for such a company to achieve success using the set strategies. It explains why companies have now embraced the approach of using data to make assumptions and decisions. Major corporations are now using information technology to process large data to come up with a prediction about the future. By critically analyzing the past and present, Hackeling (2014) says that it may be possible to predict the future. One must understand that most of these trends do not move in straight line. Sudden changes may occur that completely changes the direction expected. Using modern technology, such possible disruptions can be explained, and an alternative approach adopted to deal with it as it emerges.
Machine Learning in Management
According to Farrar and Worden (2012), sometimes artificial intelligence and machine learning are used interchangeably, which should not be the case. Artificial intelligence refers to the ability of machines to undertake tasks in a smart way, while machine learning is a concept that believes in giving machines data access s that they can learn from themselves. In machine learning, a greater freedom is granted to the machines in allowing them to undertake tasks that should be done by human beings. Marsland (2015) considers machine learning as a vehicle that is currently driving artificial intelligence so that machines can become more independent of human control and be able to deliver the expected outcome. Alpaydin (2016, p. 87) says, “rather than teaching computers everything they need to know about the world and how to carry out tasks, it might be possible to teach them to learn for themselves.” Machine learning emphasizes on the need not to teach computers everything that they need to do, but instead code them in a way that they would think and act like a human, and then offer them access to information that would inform their decisions (Sjardin et al., 2016). Scientists believe it is a better way of using technology. Machines have proven to be better than human in undertaking activities entrusted to them in terms of speed, accuracy, uniformity, and other qualitative and quantitative factors. It is believed that they can also be more effective in making decisions if they are allowed to learn and act independently without a significant influence by human.
According to Ivezić et al. (2014), human beings are not perfect when it comes to decision making. A manager may compromise success of an organization because of the desire to please a close friend or a relative. A wrong decision may be made deliberately because a leader is biased in a given manner. Corruption is another critical area that often influences wrong management decision. When a manager is corrupt, it is expected that his or her decisions cannot be for the good of the organization. They will serve personal interest at the expense of the organization and people who deserve better. Compassion is another factor that may impair the decision-making process of managers. Bell (2015) says that it comes a time when difficult choices have to be made.
Such a decision may involve eliminating a very popular employee within the firm because of underperformance of negative influence in the organization. As a human being, it is natural to rethink such decisions, and sometimes one may opt against them. However, Marsland (2015) says that the modern competitive business environment cannot afford weak minds. When hard choices have to be made, then the sooner they are made the better for the organization (Sra et al., 2012). Success in the market requires managers who are only focused on goals, not personal interest. The forces may be so unforgiving that even if the manager’s mistake was caused by misinformation or lack of it other than deliberate decision, the consequence can still be devastating. As Hassanien and Gaber (2017) say, management in the modern business environment requires perfection.
Given the fact that human beings cannot be perfect, machines come in as an alternative, meant to address weaknesses of people. According to Zhang and Ma (2012), when using machines to make decisions, every stakeholder will be aware that the set standards must be met strictly, because no one will be favored by the machines. If it is about performance of the employees, the machines will give accurate data on how each one has delivered based on the set goals. When such an organization has a system where underperformers have to be eliminated, it will be clear to everyone who should be sent home (Bell, 2015). Such systems eliminate blame games when an employee is laid off against their wish. Before embracing such system of people management, everyone should be informed about the new assessment approach and the standards expected of everyone.
At the end of the set period, every employee will see the performance record and the suggestions about their position in the firm made by the machine. It creates a sense of responsibility among the employees because they know that acts of favoritism do not exist. The machine also eliminates challenges associated with corruption, biasness or stereotyping, and mistakes caused by sympathy when it is not necessary. According to Marsland (2015), machine learning promises to address people management challenges explained in McGregor’s Theory X and Y. The problem with Theory X is that it is so pessimistic that managers tend to spend a lot of their managerial time looking for mistakes of the employees so that they can be punished. On the other hand, Theory Y is so optimistic that it ignores the need for the manager to monitor activities of the employees with keenness. The new concept will promote fairness in people management, where every individual is judged based on performance and capabilities.
Hypothesis
The information from secondary sources demonstrates that machine learning and big data offers a bright future to the field of management (Bell, 2015). Currently, people are reluctant to embrace this new concept because it is not properly understood. It is also yet to receive maximum support in many organizations because of the fear that it may replace people in the role of management. As Alpaydin (2016) says, power can be very addictive, and once one gains it, there is always the desire to retain it at all costs.
It is true that the machines will replace people in the decision making process if the concept of machine learning is fully embraced. It is also true that these machines will address human weaknesses such as corruption, favoritism, excessive compassion, and fear when it comes to making critical decisions. These weaknesses must be eliminated in the modern business environment, and that is why it may not be possible to avoid machine learning and artificial intelligence for long (Marsland, 2015). Firms that continue to resist this new concept may find themselves struggling to achieve success in the market. This information from the literature review has informed the formation of the following hypothesis.
H1. The use of machine learning and big data is a solution to improve company’s performance by monitoring, analyzing, and readjusting efforts for employee engagement.
Methods
In this section of the report, the focus is to explain the methods that were used in collecting and analyzing data from various sources. The researcher collected data from two main sources. Secondary sources of data were very important in providing background information and focus for the research. Secondary data came from books, journal articles, and reliable online sources. They formed the basis of literature review and informed the research hypothesis. Primary data was collected from sampled respondents and it helped in answering the research questions and confirming the hypothesis.
Research Design
The overall strategy that a researcher uses in a given study should be based on the goals that should be achieved in the project. According to Sugiyama et al (2012), choosing the right research strategy is critical in ensuring that the right data is collected and analyzed to inform conclusion and recommendations in the study. In this research, the primary goal was to determine how machine learning and big data could be used to improve company’s performance by monitoring, analyzing, and readjusting efforts for employee engagement. To achieve the set goal, the researcher chose to use both primary and secondary data. Primary data was collected from a sample of 100 respondents through survey as discussed below. Once the data was collected, a series of analysis was conducted to come up with findings to confirm the research hypothesis and to respond to the research questions. Descriptive statistics was considered important in explaining the relevance of machine learning in an organizational setting.
Data collected was analyzed mathematically to help bring out the views of the respondents. Excel spreadsheet was used to facilitate the statistical analysis. The findings from the analysis were presented in charts and graphs. Other than the statistical analysis, it was also important to conduct qualitative analysis in the research. Some of the respondents are in managerial positions, and they have experienced the relevance of machine learning in their organizations. A section of them feels that this new concept is very important and can improve their managerial responsibilities, while others are skeptical towards it. Capturing their varying views, through qualitative analysis was considered important. It helped in explaining why machine learning is yet to be widely embraced in the country despite its increasing relevance as a management tool.
Sample and Data Sources
Machine learning is an emerging concept in this country and it was important to collect data from respondents who have been affected by it, or have heard about it in their career. A sample of 100 participants was selected to take part in this study. The researcher used simple random sampling to identify the participants. The respondents were selected based on their willingness to be part of the study, their availability, and their knowledge of the concept of machine learning. After sampling the respondents, the researcher used online survey to collect data from them. It was considered an appropriate approach because of the limited time within which the research project had to be completed.
Procedure
As explained above, the researcher used online survey to collect data from the respondents. The first procedure was to contact the individuals believed to have the needed knowledge about machine learning. They were contacted on phone and the researcher explained their role in the study and significance of the project. They were informed that they had the liberty of withdrawing from the study in case they considered it necessary (Murphy, 2012). The researcher then e-mailed the questionnaires, which had been prepared prior to the data collection process, to them. They responded to the questions and then e-mailed back the filled questionnaire. The researcher then analyzed the data using both quantitative and descriptive statistics.
Measure
In this paper, the independent variable is the use of machine learning. The dependent variable is people management role in an organizational context. The focus is to measure the machine learning (the independent variable) can affect people management (dependent variable) within an organization. By using statistical methods, it will be possible to quantify the relationship. The researcher will be able to explain the level of relationship between these variables. If it is determined that there is a close relationship between these variables, then the study will have confirmed the set hypothesis.
Analysis and Results
Descriptive Statistics
In this section of the report, the researcher will provide descriptive statistics based on the information that was collected from the respondents. The three questions that were set will be answered in this section based on the primary data. The hypothesis will also be tested to determine if the respondents believe machine learning and big data can be used to improve company’s performance by monitoring, analyzing, and readjusting efforts for employee engagement.
What are the main challenges that managers face in coordinating and controlling the activities of the employees?
The first question focused on capturing the main challenges that managers are currently facing in their role of coordinating and controlling employees. In the literature review, it was noted that management in the modern-day organizational setting has changed significantly. Managers face unique challenges that require unique solutions. Figure 1 below identifies major challenges that managers have to deal with in the current organizational setting.
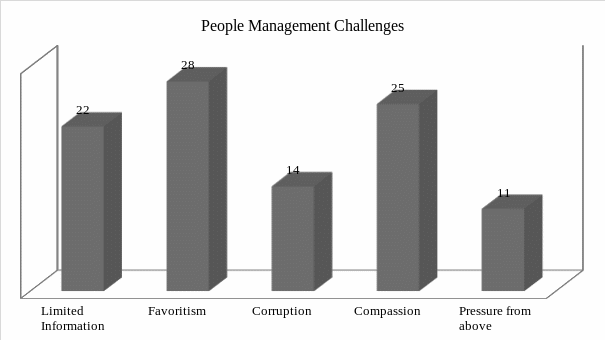
Majority of the respondents felt that favoritism is one of the biggest challenges that managers have to deal with in their organizations. It is common to find cases where a manager feels compelled to offer special treatment to a section of the employees because of various reasons. Sometimes the relationship between the manager and some employees that makes it difficult for the manager to make a decision that would be unfavorable to these employees. The decision and actions of such a manager will be compromised. Instead of issuing warnings or even punitive measures as would be expected when these employees fail to perform, they will prefer to ignore such issues for fear of ruining the relationship. Such actions may have a significant impact on the overall performance of an organization because other employees will be demoralized by these acts of favoritism. Compassion is a desirable attribute that every person should embrace to lead a fulfilling life, but it poses managerial challenges. Managing people is a very tasking role that may force one to make tough unpopular decision for the sake of the firm. When one is so compassionate that he or she cannot afford to lay off an employee, then such a person may not be a good manager.
The respondents also noted that limited access to information is another challenge that managers sometimes face in their respective organizations. For a manager to make a decision, he or she needs information that would guide them in such processes. With limited information, it is possible that the choices or decisions that such a manager would make will not be the most appropriate ones. Such mistakes may be very costly to an organization. Corruption was another factor that was identified in the study. Some respondents felt that when managers allow themselves to be bribed, they become compromised in their decision-making processes. They may ignore organizational values and its interests for personal gains. The respondents also noted that in some cases managers are affected by the pressure from their superiors. They may be forced to circumvent organizational rules and principles in favor of the interest of a section of the board of directions. Given the authority of these directors, the managers may have limited choice but to do as directed.
How can companies use the concept of machine learning to improve employee engagement, performance, and motivation?
The second question focused on determining how companies can use the concept of machine learning to improve employee engagement, performance, and motivation. The respondents were asked to state whether they believe machine learning can address the problem. Figure 2 below shows their response:
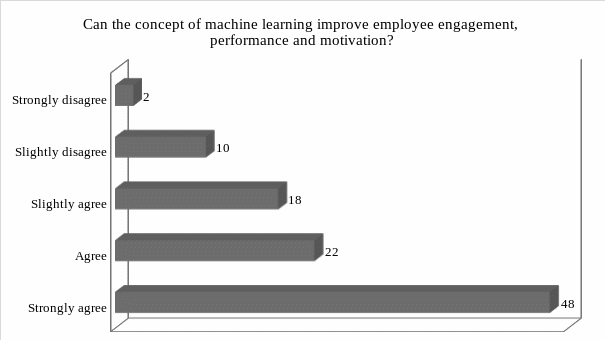
An overwhelming majority (70%) of the respondents agree with the statement that machine learning can improve employee engagement, performance, and motivation. It improves the process of collecting, analyzing, and sharing information that is needed for operational purposes. Employees’ performance will be improved if they are allowed access to relevant information that would guide their actions. It makes it possible for them to engage with the management in consultative debates through improved systems of communication. When machine learning eliminates weaknesses of management and promotes fairness within an organization, employees will be motivated. They will realize that their career growth and future at the firm will be based on their capabilities and effort other than favoritism and corruption. Their improved commitment to the firm will enhance the overall performance of the organization.
How can managers use machine learning to improve their performance?
The last question focused on determining how managers can use machine learning to improve their performance. The respondents were asked to state whether they believe machine learning can improve the performance of managers. Figure 3 below shows their response.
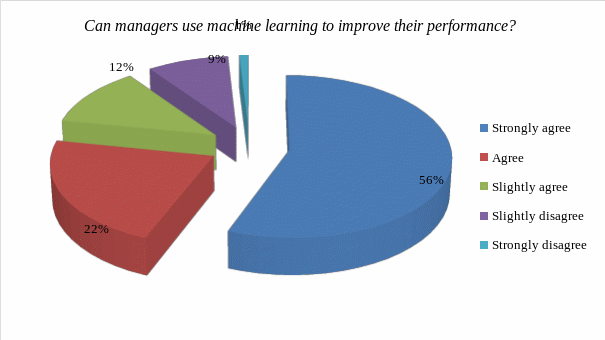
Majority of the respondents (90%) agree with the statement that machine learning can help improve the performance of the managers. They explained that using machine learning, it is possible to eliminate major weaknesses of employees associated with human nature such as greed and favoritism. It also improves accuracy in the decision-making process, especially if it involves predicting the future.
H1. The use of machine learning and big data is a solution to improve company’s performance by monitoring, analyzing, and readjusting efforts for employee engagement.
The researcher then focused on analyzing primary data to confirm or reject the hypothesis that had been set. To confirm the hypothesis, the researcher asked the respondents whether they believe that machine learning and big data can improve a company’s performance. Figure 4 below shows their response:
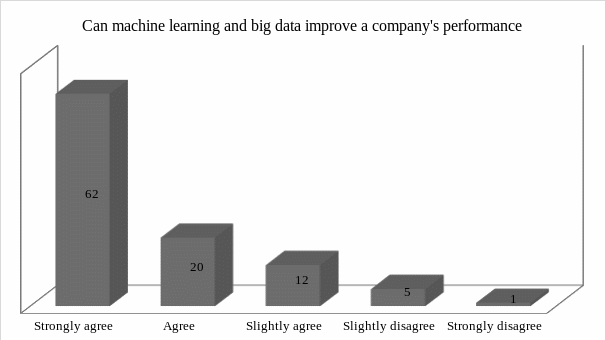
As shown in the figure above, the majority of the respondents (94%) believe that machine learning and big data can improve a company’s performance. They stated that machine learning could improve the process of monitoring, analyzing, and readjusting efforts for employee engagement. Their response means that the above hypothesis is confirmed.
Case Study: Machine Learning at General Electric
General Electric is one of the largest multinational corporations in the world, and it has its headquarters in Boston, Massachusetts (Alpaydin, 2016). The company has highly diversified its product portfolio, and as such, it operates in several industries. Managing hundreds of thousands of people and coordinating all operational activities at this company is a very complex process. The top management unit realized that the large size of the company and its diversification into many industries could not be a justification to underperform. As such, it has been working on ways of improving the performance of managers and that of the firm.
In early 2017, the chief executive officer of this company announced the commitment of GE’s top management to embrace digital transformation. It was announced that the management was keen on building a digital organization to improve the performance of the managers and that of the employees. One of the areas that the machine learning was initiated at that early stage was supplier and client data management. Instead of relying on traditional methods, the company opted to use machine learning and artificial intelligence to ensure that information from clients, suppliers are analyzed within the shortest time possible, and accuracy is maintained when making predictions. The move has significantly improved the performance of GE in these two departments (Raschka, 2015). The success registered at GE in its implementation of machine learning can be replicated in other organizations keen on embracing this new concept.
Discussion
The analysis of primary data and review of literature show that one of the most important areas that one cannot ignore when researching on machine learning is the concept of artificial intelligence. According to Murphy (2012), artificial intelligence refers intelligence that is displayed by machines. Under normal circumstances, intelligence is expected to be displayed by human beings and animals. These living things need some level of intelligence to monitor their environment, get food, and avoid harm. However, advancement in the field of information technology has created a platform where machines can also learn and display intelligence.
The technology of computer programming has made it possible to empower computers with problem-solving capabilities. It is expected that machines must receive instructions from people to undertake a given task. That has been the case for many years that machines have been used to ease the way of life of humankind. However, these machines have never been trusted with the ability to make independent decisions based on the forces in the environment. The fact that they are not living beings makes it impossible for them to make rational decisions. They lack compassion to act in a manner that a human being would (Raschka, 2015). However, advancements witnessed in artificial intelligence promises to change this. It is not possible for the machines to make decisions without getting instructions from people.
According to Hackeling (2014), one of the areas where machines have proven to be perfect in making independent decisions is on room temperature management such as the technology used in the fridge. The system can monitor itself, and ensure that a given temperature is not exceeded. Fully automated cars with the capacity of driving themselves on designated roads have been created. Such machines are empowered, through modern technology, to monitor the immediate environment, collect relevant data, process, and act upon it within the desirable time. Although such smart cars are yet to be commercialized, they point to a future where technology will be used to make decisions that could previously be made only by human beings. Artificial intelligence is also actively used in the control systems of the airplanes. The systems are designed to help pilots in making decisions. They get to warn them when they make wrong decisions and recommend the appropriate decisions based on the prevailing circumstances (Marsland, 2015). In the military, artificial intelligence is currently used actively to monitor actions of enemies and in determining the appropriate actions that should be taken. They help in eliminating cases where erratic decisions are made by the officers due to fear or misinformation.
The success of artificial intelligence in various fields means that it can also be applicable in the field of management. Managers are also facing various challenges in their respective organizations that may require super-brain to come up with an effective answer (Alpaydin, 2016). For instance, employees form a critical component of any organization. Many companies spend many resources to train and improve capabilities of their employees. After spending such resources, it would be necessary to retain all of them for as long as possible so that the organization can benefit from their skills (Murphy, 2012). However, sometimes it may be necessary to eliminate some employees even after spending resources to improve their performance.
Theoretical Implication
It is important to look at theoretical implications of this study. This primary and secondary data demonstrate that machine learning is a critical tool that cannot be ignored in the modern business environment. The study strongly suggests that some theories of management that have been used in the past may not be suitable anymore in the modern business environment (Raschka, 2015). For instance, McGregor’s Theory X and Y may not be suitable in the modern business context. The outcome of this study shows that Theory X is too pessimistic while Theory Y is too optimistic to be applicable in the business environment today. Both theories also tend to generalize employee’s performance, something that machine-learning concept strongly opposes.
Managerial Implications
This study strongly suggests an urgent need for a major shift in management approach in a modern organization. Firms cannot afford to make constant mistakes, especially in relation to employee management. Sometimes it may be necessary for a manager to make a difficult decision that may not be popular with the majority within an organization. A number of factors may determine the need to make such a painful decision, and a manager may need to put into consideration so many factors. Without the aid of artificial intelligence, a firm may end up eliminating an employee that might have become a star within the firm if specific issues were to be addressed (Alpaydin, 2016). For instance, such an employee may be having a problem with his or her family, which in turn affects productivity at work. Using artificial intelligence, a manager can develop a comprehensive analysis of each employee within a short time to determine whether he or she is worth keeping. It eliminates mistakes by presenting a detailed analysis of the key issues, which are of interest to the firm.
Limitations and Future Research
When conducting this research, one of the main limitations faced was the ability to collect data from managers in various top companies in the United Arab Emirates. To understand the current trend in the application of this modern technology in the country, it would be important to have a face-to-face interview with several managers of top companies so that they can explain the internal practices in their organizations, challenges they face, and the plans put in place to embrace machine learning in management. Future research should focus on how machine learning is being applied locally in companies such as ADNOC. Existing studies explain how it has been applied in the United States and European companies, but limited information exists about its application in the United Arab Emirates.
Conclusion
Machine learning is a concept that is increasingly gaining relevance in the modern organizational environment. Machines have been used for a long time to make work easy but in a controlled manner. In the past, machines had to rely on the decisions made by people who operate them. However, time has come when machines can be allowed to make decisions independently without any human influence. Machine learning is a concept where computers are coded to work as a human mind, allowed access to data that can facilitate learning, and enabled to make independent decisions. One of the areas that machine learning is gaining rapid relevance is in the field of management. Managers face numerous challenges in their normal duties.
Sometimes they have to make unpopular decisions for the sake of the company. At times, they make decisions with limited information, leading to mistakes. Managers also have personal weaknesses such as favoritism, greed, and nepotism that sometimes impair their judgment when making critical managerial decisions. It is important to find ways of addressing these challenges to enhance the performance of the managers in their respective organizations. Using machine learning makes it possible to manage people without being affected by the challenges made above. It also enhances accuracy when predicting the future. Machine learning enables managers to process large and complex data within a short time and with high levels of accuracy. The new trend cannot be ignored in the modern competitive business environment.
References
Alpaydin, E. (2016). Machine learning: The new AI. Cambridge, MA: MIT Press.
Bell, J. (2015). Machine learning: Hands-on for developers and technical professionals. Hoboken, NJ: John Wiley & Sons, Inc.
Camastra, F., & Vinciarelli, A. (2015). Machine learning for audio, image and video analysis: Theory and applications. London, UK: Springer.
Christiano, T., & Zhao, L. (2016). Machine learning in complex networks. Cham, Switzerland: Springer.
Cleophas, M., & Zwinderman, A. (2015). Machine learning in medicine: A complete overview. Cambridge, MA: MIT Press.
Dehmer, M., & Basak, S. C. (2012). Statistical and machine learning approaches for network analysis. Hoboken, N.J: Wiley.
Farrar, C. R., & Worden, K. (2012). Structural health monitoring: A machine learning perspective. West Sussex, U.K: Wiley.
Hackeling, G. (2014). Mastering machine learning with scikit-learn. Birmingham, UK: Packt Publishing.
Hassanien, A. E., & Gaber, T. (2017). Handbook of research on machine learning innovations and trends. New York, NY: Cengage.
Ivezić, Z., Connolly, A., Vanderplas, J. T., & Gray, A. (2014). Statistics, data mining, and machine learning in astronomy: A practical Python guide for the analysis of survey data. Princeton, N.J: Princeton University Press.
Marsland, S (2015). Machine learning: an algorithmic perspective, New York, NY: Taylor & Francis Group.
Murphy, K. P. (2012). Machine learning: A probabilistic perspective. Cambridge, MA: MIT Press.
Pentreath, N., & Paunikar, A. (2015). Machine learning with spark: Create scalable machine learning applications to power a modern data-driven business using Spark. Birmingham, UK: Packt Publishing.
Raschka, S. (2015). Python machine learning: Unlock deeper insights into machine learning with this vital guide to cutting-edge predictive analytics. Birmingham, UK: Packt Publishing.
Sjardin, B., Massaron, L., & Boschetti, A. (2016). Large-scale machine learning with python: Learn to build powerful machine learning models quickly and deploy large-scale predictive applications. Birmingham, UK: Packt Publishing Ltd.
Sra, S., Nowozin, S., & Wright, S. J. (2012). Optimization for machine learning. Cambridge, MA: MIT Press.
Sugiyama, M., & Kawanabe, M. (2012). Machine learning in non-stationary environments: Introduction to covariate shift adaptation. Cambridge, MA: MIT Press.
Sugiyama, M., Suzuki, T., & Kanamori, T. (2012). Density ratio estimation in machine learning. New York, NY: Cengage.
Zhang, C., & Ma, Y. (2012). Ensemble machine learning: Methods and applications. New York, NY: Springer.