Introduction
Today’s business environment is challenging to technology gatekeepers because they have to develop information systems that can effectively manage irregular flow of large volumes of data (Fox & Do 2013, pp. 739-760). Reducing the cost of managing information and ensuring data security are also significant challenges that technology gatekeepers have to grapple with (Marthandan & Tang 2010, pp. 37-55). Other challenges that face technology gatekeepers are summarized in Figure 3. The percentages represent the proportion of the technology gatekeepers who agreed that they had experienced the challenges highlighted in Figure 2 in their organizations.
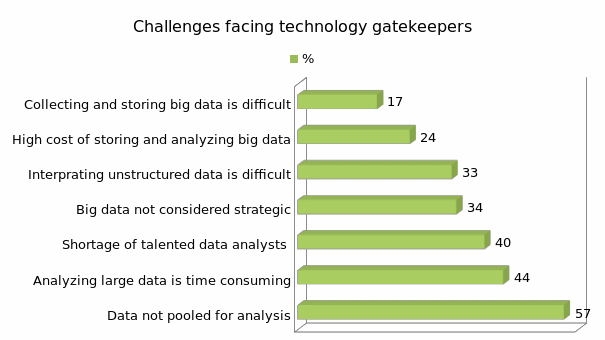
This report will discuss the strategies that can be used to develop Union National Bank’s information system to exploit the potential of big data. Union National Bank (UNB) is a midsize commercial bank that operates in the UAE. The bank was founded in 1982 in the UAE where its headquarters are located (UNB 2014).
UNB is a public company that is owned by the governments of two emirates namely, Abu Dhabi and Dubai. The bank’s products include savings, credit, and investment services. Big data refers to the “exponential growth and availability of structured and unstructured data” (Zikopoulos & Eaton 2011, p. 6).
Big data is associated with four characteristics namely, volume, velocity, variety, and variability. Volume refers to the fact that big data is generated from several sources, which include social media, sensors, and transaction databases among others. The data often streams in at an unprecedented velocity. Big data is also associated with variability in flows because data from various sources is often generated in an inconsistent manner (Marz & Warren 2014, p. 15).
Exploiting big data can help a company to deepen customer engagement, optimise operations, prevent fraud, and develop new revenue streams. UNB can realise these benefits if it integrates big data into its operations support systems (OSS). OSS refers to a computer-based information system that enables managers to manage various business processes effectively and efficiently (Clarke 2012, p. 67). Therefore, the implementation of big data will be discussed in the context of OSS.
Analysis
Challenges
Union National Bank faces the following challenges, which warrant adoption of big data. First, the bank is currently on a growth trajectory, which is characterised by rapid market expansion. Currently, the bank has only 68 branches in the UAE, which it intends to double in the next five years by joining new markets in the Gulf Cooperation Council region (UNB 2014).
However, the challenge to this expansion plan is that the bank lacks the capacity to achieve a high level of customer centricity in order to attract clients in new markets. This challenge is attributed to the fact that the bank hardly analyses its customers’ data such as their social media activities. As a result, the bank lacks an adequate understanding of the needs of its customers.
The bank can overcome this challenge by using big data technologies to retrieve and analyse the huge volume of data at its disposal (Lemieux, Gormly & Rowledge 2014, pp. 122-141). Second, the bank stores customer data in different databases that are used by systems that perform specific functions such as customer relationship management (CRM) and monitoring loan servicing (UNB 2014).
This prevents integration of data to gain a clear 360-degrees perspective about customers’ needs. Therefore, the bank needs big data analytics technologies that will integrate its databases and facilitate seamless analysis of customer data. Third, the company lacks a strategic focus to leverage the potential of its OSS.
Effective management of data requires advanced technologies that facilitate storage, organisation, and retrieval of large volumes of data (McDonald & Leveille 2014, pp. 99-121). However, the bank still uses an outdated OSS that can only facilitate analysis of static and historic data. In this respect, big data technologies will help the company to mine historic and new data in a near real-time manner.
Fourth, Union National Bank lacks an effective data management framework. This challenge is exacerbated by the fact that the company uses ad-hoc analytics tools that are based on the experience of its employees in data analysis (UNB 2014). Consequently, the outcome of data analysis hardly provides adequate insights that are needed to make effective decisions.
Therefore, the bank needs to adopt “more advanced analytics techniques such as predictive and prescriptive analytics that facilitate precise modeling of customer behavior” (Capgemini 2014, pp. 2-15). The bank can access these techniques by adopting big data analytics.
Change
The main stakeholders who will be affected by big data at Union National Bank include the employees, the management, and customers. Employees will be affected in the following ways. To begin with, employees will have to undergo rigorous training in order to use big data effectively.
Organisations can use big data effectively only if they have data scientists who possess advanced quantitative skills (Zikopoulos & Eaton 2011, p. 78). However, the supply of data scientists is very low, whereas the demand for their skills is ever increasing.
For instance, nearly four million data scientists will be required globally by 2015. However, only one third of the demand will be met due to the shortage of data scientists (Zikopoulos & Eaton 2011, p. 81). This means that Union National Bank will have no choice but to train its own data scientists to implement and use big data analytics.
Apart from improving employees’ skills, implementing big data analytics is likely to change staff morale (Schroeck, Shockley & Tufano 2013, pp. 3-20). For instance, improved management and analysis of data is likely to boost the performance of employees. This will lead to improved morale.
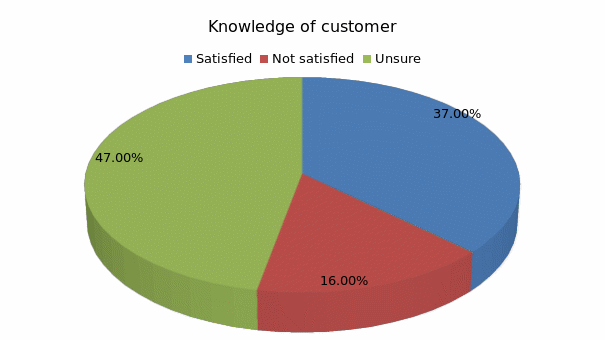
The main effect on customers will be improved service quality. Only 37% of customers in the banking industry believe that banks have adequate knowledge about their needs as shown in figure 1 (Capgemini 2014, pp. 2-15). Moreover, only 43% of customers are satisfied by the distribution channels that are used by banks as indicated in figure 2 (Capgemini 2014, pp. 2-15).
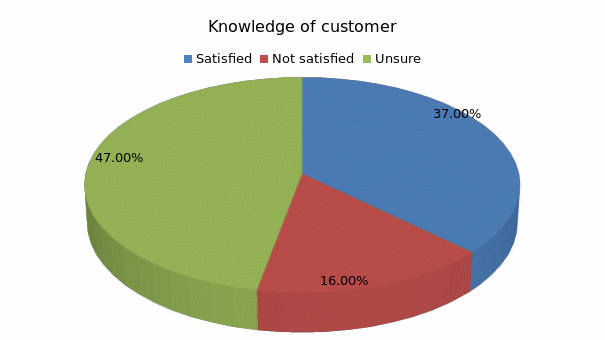
Over 70% of executives in the global banking industry believe that big data analytics can help them to improve service quality by deepening their understanding of customer needs. In this respect, the insights obtained from big data are expected to help the company to align its services to customers’ expectations.
Given these effects, the success of big data at the bank can be enhanced by collaborating with various stakeholders as follows. First, the marketing executives and operations managers should collaborate with the suppliers of the big data analytics system during the development stage.
This will ensure that the system is capable of analysing all the performance indicators that marketers and operations managers expect to measure. Second, the management and all employees should be informed about the function and expected outcomes of the system to prevent resistance during the implementation stage.
Finally, customers should be assured that the security of their data/ information will be maintained. This will encourage “customers to provide the information that is necessary for the successful implementation of big data” (DATASTAX 2013, pp. 3-15).
Value/ Benefits of Big Data
Big data will benefit Union National Bank in the following ways. First, it will allow the bank to maximise its lead generation and acquisition of new customers. The US Bank is one of the companies that have increased their customer base by exploiting the potential of big data. The bank used “big data analytics to integrate data from its online and offline distribution channels to gain a clear view of its clients” (Capgemini 2014, pp. 2-15).
This helped the company’s sales team to identify and contact potential clients. As a result, US Bank’s lead conversion rate increased by 100% (Capgemini 2014, pp. 2-15). This means that Union National Bank can use big data to generate relevant leads that will eventually turn into actual sales.
Second, big data analytics will help the bank to improve its credit risk assessment. Currently, the bank is using the FICO scores that evaluate credit worthiness by taking into account only the customer’s financial history. Big data analytics will allow the “company to conduct a more comprehensive credit risk assessment by taking into account additional variables such as customers’ demographic, employment, and behaviors” (Ohlhorst 2012, p. 88).
Consequently, the bank will reduce its level of non-performing loans by advancing credit only to customers who are capable of repaying their loans. Third big data will facilitate effective market segmentation. Big data facilitates integration of data concerning “past buying behavior, demographics, and sentiments from social media with CRM data” (Capgemini 2014, pp. 2-15).
This helps in gaining insights concerning customers’ preferences, which in turn facilitates market segmentation. Undoubtedly, effective segmentation will facilitate cross-selling and up-selling, as well as, improvement in customer engagement and loyalty. Ultimately, effective segmentation will improve the bank’s sales.
Moreover, the reputation of the bank will improve if it uses big data to develop products that meet customers’ expectations in terms of quality and prices (Hirsch 2013, pp. 36-39). The resulting improvement in customer loyalty will increase the bank’s profits.
Finally, Union National Bank will be able to predict and reduce customer churn rate. In the banking industry, churn rate refers to the percentage of customers that a bank loses within a financial year. In 2012, nearly 50% of customers globally changed their banks (NGDATA 2013, pp. 2-10).
The high churn rate is a significant risk in every bank since it leads to loss of revenue. Big data analytics will enable Union National Bank to gain customer intelligence to avoid a losing its clients. This will help the company to avoid losing market share by taking timely measures to retain its customers.
The benefits discussed in the foregoing paragraphs will be measured using the following metrics. To begin with, maximisation of lead generation will be measured in terms of the lead conversion rate. In this case, the percentage increase in new customers as a result of using big data will be an indication of success.
Improvement in credit risk assessment will be measured by the percentage change in the level of non-performing loans. The percentage change in sales in various market segments will be used to measure improvements in segmentation. Moreover, the percentage decrease in the number of customers lost within a financial year will be used as a measure of the effectiveness of using big data analytics to predict and prevent customer attrition.
These metrics are effective because they provide quantifiable measurement of each benefit of big data. Moreover, the metrics are easy to use since they require only simple calculations. Therefore, they will facilitate accurate and effective assessment of the impact of big data on the bank’s performance.
Time
The big data project will take at least four years to implement. This timeframe is justified by the fact that implementing the project involves several activities that will require a lot of time (Fox & Do 2013, pp. 739-760). For instance, at least one year will be required to conduct a pilot test before the full rollout of the project.
In this regard, the first year will be used to create a new database that will improve access to and analysis of big data effectively. In the second year, the company will focus on acquiring the hardware and software that will facilitate big data analytics.
Moreover, the management and employees will be trained on how to use the new system to analyse big data. The third year will be used to conduct a pilot test in order to identify problems that might negatively affect the usability of the system. The full implementation of the system will be done in the fourth year.
Costs
Table 1 below indicates that the bank will require UK pounds 22.25 million to implement the big data project. Acquiring the software that will be used to query/ analyse the data will cost approximately UK pounds 8.75 million. The high cost is attributed to the fact that the company will have to modify its OSS to accommodate the new software package the will be used to manage big data.
Table 1: Cost of acquiring the system
Source: Estimates based on the costs of similar big data projects done by Oracle
Overall, the high cost of implementing the project is justified by the expected benefits. The company is expected to recover the cost in the first three years after implementation. The cost will be recouped as the bank’s revenue increases.
According to Table 2, the bank will require approximately UK pounds 3.25 million annually to use the big data analytics system. However, the annual usage and maintenance cost will vary in future due to changes in technology and competition among IT service providers.
Table 2: Usage and maintenance costs per year
Source: Estimates based on the costs of similar big data projects done by Oracle
For instance, the cost of storing data is expected to decline in future as cheap technologies become accessible. Similarly, a high competition among IT companies such as SAS and Oracle is likely to reduce the software license fee in future (DATASTAX 2013, pp. 3-15).
The indirect costs of the project include deskilling of the workforce and loss of morale among employees. Deskilling is likely to occur because the project will automate various processes of managing data/ information (Prajapati 2013, p. 67).
Thus, employees will depend on the reports generated by the OSS rather than using their skills to analyse the existing data. This means that data analysis will be a routine process that is likely to cause boredom, which in turn will reduce employees’ morale. Undoubtedly, reduced morale will cost the company dearly in terms of low productivity among employees. Moreover, deskilling the workforce is likely to prevent innovation.
Risks
First, using big data analytics to establish relationships between related and unrelated pieces of data is likely to reveal sensitive customer information. Moreover, outsourcing data analysis processes is likely to compromise the security of customer data (Marz & Warren 2014, p. 92). Generally, lose of data security is likely to cause costly legal battles between the bank and its customers.
Furthermore, the bank is likely to lose the trust of its customers if it exposes their data/ information to third parties. This will lead to a reduction in the number of customers and profits. This risk can be prevented by developing and implementing policies that will facilitate protection of data and the insights generated through big data analytics (Asiata 2010, pp. 308-322).
Second, the high cost of implementing the project is likely to compromise the profitability and growth of the company (Trottier 2014, pp. 51-72). Specifically, the bank might remain with inadequate funds to support business processes such as marketing if it channels a large portion of its resources towards implementation of the project.
However, the risk can be prevented by exploiting external sources of funding to finance the project without compromising the bank’s profitability. For instance, the bank can use a rights issue to raise cheap capital from its shareholders to finance the project. This will allow the bank to use its internal financial resources to support other projects such as product development.
Finally, the project might not achieve the desired outcome if it is not implemented appropriately. Deutsche Bank is one of the companies that are yet to benefit from big data analytics despite using for over two years. The challenge facing Deutsche Bank is that its 46 data warehouses were not integrated during the system design stage (Capgemini 2014, pp. 2-15).
As a result, the company is not able to extract and analyse the large volume of data at its disposal. This means that Union National Bank’s project can also fail to provide the desired output if it is not designed effectively. This risk can be prevented by paying attention to detail and collaborating with the users of the system to improve its design. In addition, the pilot test stage should be used to detect and correct all errors that are likely to compromise the effectiveness of the project.
Implementation
The implementation process should be conducted in three controlled steps to ensure a smooth transition to the new system. The first step should focus on assessing the bank’s analytics capability. The assessment includes conducting system investigations and analyses to identify the information and the analytics techniques that are required by the bank. This will help in identifying the type of software that will be required to analyse the existing and new data (Rouda 2014, pp. 2-15).
In the second step, the bank should acquire a tailored off-the-shelf big data analytics system. This will involve purchasing a prewritten application from a software vendor. However, the application will have to be reconfigured to suit the needs of the bank. This method of acquisition will benefit the bank in the following ways.
First, purchasing an off-the-shelf application will reduce the time that will be required to acquire and implement the system. Reconfiguring an off-the-shelf application is easier and requires less time than writing a new one from scratch (Barlow 2013, p. 97). Second, an off-the-shelf application is cheaper than a bespoke and end-user developed software.
In the last three years, venture firms have invested over $2.5 billion in companies that develop big data applications (Rouda 2014, pp. 2-15). This has resulted into increased availability and reduced cost of off-the-shelf applications. Thus, Union National Bank can reduce the cost of the project by purchasing a prewritten application.
Third, off-the-shelf applications have high quality because they are often feature rich. In this respect, the bank will benefit from advanced analytics capabilities. However, reconfiguring the application may introduce bugs in the bank’s OSS. In this respect, the reconfiguration stage should focus on eliminating the problems that might emerge after the implementation of the new system.
The third step should involve a series of pilot tests to verify the effectiveness of the new system. Rabobank is one of the largest banks in the Netherlands that have successfully implemented big data projects through a series of pilot tests.
The bank began using only internal data to gain insights into its customers’ needs (Capgemini 2014, pp. 2-15). The problems identified at this initial stage were easily resolved and enabled the company to expand the scope of its big data project without significant bottlenecks. Therefore, UNB should focus on testing various aspects of the project and rolling it out gradually to avoid a system-wide failure.
Conclusion
Union National Bank should exploit the potential of big data in order to achieve its market share and profitability objectives. Big data analytics will enable the bank to acquire new clients and reduce customer attrition. It will also enable the bank to reduce its level of non-performing loans and segment its market effectively. As a result, the bank’s profits and market share will increase.
However, implementing a big data project is time and resource intensive. Thus, the implementation stage should focus on reducing costs and addressing the risks that are likely to cause failure. This includes ensuring data security and acquiring effective analytics tools to generate the expected insights.
References
Asiata, L 2010, ‘Technology, individual rights, and the ethical evaluation of risk’, Journal of Information, Communication, and Ethics in Society, vol. 8. no. 4, pp. 308-322.
Barlow, M 2013, Real-time big data analytics: emerging architecture, O’Reilly Media, New York.
Capgemini 2014, Big data alchemy: how can banks maximize the value of their customer data?, Capgemini Consulting, Dallas.
Clarke, S 2012, Information systems strategic management, Routledge, New York.
DATASTAX 2013, Big data: beyond the hype, DATASTAX Corporation, Santa Clara.
Fox, S & Do, T 2013, “Getting real about big data: applying critical realism to analyse big data hype”, International Journal of Managing Projects in Business, vol. 6. no. 4, pp. 739-760.
Hirsch, P 2013, ‘Corporate reputation in the age of data nudity’, Journal of Business Strategy, vol. 34. no. 6, pp. 36-39.
Lemieux, V, Gormly, B & Rowledge, L 2014, “Meeting big data challenges with visual analytics: the role of records management”, Records Management Journal, vol. 24. no. 2, pp. 122-141.
Marthandan, G & Tang, C 2010, ‘Information technology evaluation: issues and challenges’, Journal of Systems and Information Technology, vol. 12. no. 1, pp. 37-55.
Marz, N & Warren, J 2014, Big data: principles and best practices of scalable real-time, Manning Publications Company, Greenwich.
McDonald, J & Leveille, V 2014, “Whither the retention schedule in the era of big data and open data”, Records Management Journal, vol. 24. no. 2, pp. 99-121.
NGDATA 2013, Predicting and preventing banking customer churn by unlocking big data, NGDATA Consulting, New York.
Ohlhorst, F 2012, Big data analytics: turning big data into big money, John Wiley and Sons, New York.
Prajapati, V 2013, Big data analytics with R and Hadoop, Pact Publishing, New Delhi.
Rouda, N 2014, Getting real about big data: build versus buy, Oracle, Redwood.
Schroeck, M, Shockley, R & Tufano, P 2013, Analytics: the real-world use of big data, IBM Institute for Business Value, Beijing.
Trottier, D 2014, ‘Big data ambivalence: visions and risks in practice’, Studies in Quantitative Methodology, vol. 13. no. 1, pp. 51-72.
UNB 2014, About us. Web.
Zikopoulos, P & Eaton, C 2011, Understanding big data: analysis for enterprise class, McGraw-Hill, New York.