Introduction
Education is one of the main areas of life where people aspire to scale from one level to the next. While some fail to continue their education and settle for work, family, or personal businesses, many succeed and seek to pursue their careers at more advanced levels. Breakthroughs in education usually occur after passing high school exams with grades that give them entry to the university. Most students are generally confused because they have no career choices, yet they must make critical decisions that can influence their future professionalism and families (Darioshi & Lahav, 2021). According to Lent and Brown (2020), the magnitude of this decision surpasses previous choices ever made by the learners. Consequently, making such a decision is not trivial because the students may not have access to all the crucial information necessary to inform their choices. According to Batool and Ghayas (2020), many student students choose their courses based on peer influence, which eventually becomes burdensome to them at a later stage in life. Moreover, some students change their courses after some years in the course they chose while they were uninformed about the programs that suited their potential.
Apart from choosing courses, students are also faced with the challenge of selecting the institutions where they can study. Higher educational institutions, students, and parents recognize the significance of pursuing education outside the home. From a student’s perspective, studying abroad may mean they are exposed to an entirely new way of living, communicating, studying, learning, and socializing. This may be beneficial as they become independent, have a more comprehensive worldview, and become better communicators. Other benefits may include better career, job, and immigration opportunities. However, deciding on an institution because it is based in a specific region may mislead a student into selecting a course that may not help the learner in the future. Consequently, it is crucial to furnish learners, especially high school graduates, with enough information that assesses their past, behaviors, aspirations, and interests and use the gathered data to recommend a course for the students.
Background of the Study
University and course selections have challenged students for several years despite the availability of online resources. According to Malik, Rana, and Bansal (2020), the unfiltered information provided through the Internet has been one of the primary causes of misleading information that high school graduates use to make information regarding their chosen courses. Consequently, it is critical to design a system that considers students’ preferences and interests to guide them in selecting their courses. For instance, some learners have fixed attitudes and only explore specific content on the web regarding their education. Others consider courses that are easy for them to obtain high grades, while others may want to study guided by professors with experience. Therefore, it is crucial to analyze the opinions that could make the learners gather enough data to help them recognize their interests and course choice. This can be achieved through an assistive technology for supporting student admission through course selection discovery or a Recommendation System (RS), which directs the learners from one point to the next and uses the collected data to give the students their unique preferences.
The primary role of such an assistive technology for supporting student admission through course selection discovery is to deliver customized information meeting the needs of various users based on their preferences. These can be achieved through using Collaborative Filtering (CF) or Content-based Filtering (CBF), each other which uses unique algorithms that help in making the final recommendation (Thannimalai & Zhang, 2021). CF reaches a choice based on past ratings coinciding with that of the user, while CBF relies on the contents relating to the same items of the user profile. Hybrid systems combining the two have also been implemented to account for the benefit of the two mentioned system design approaches.
Problem Statement
Various stakeholders have implemented several information and communication technologies and systems to support the university course application process. However, in most of them, students meet an overwhelming chunk of data that they must individually go through one by one to help them select a preferred institution and course to study. Some learners must outsource the task of finding suitable colleges to agents or consultants. In contrast, others must spend longer hours searching for tailor-made information, which may yield fruitful results or render the individual’s efforts futile. Still, others settle for courses other than their original choice because they fail to get what they were searching for and yield to the pressure from their parents to select a particular college based on sentiment or personal biases. Moreover, other learners gather and rely on misleading or erroneous information from blogs and websites whose identity and integrity are questionable.
Using third-party agents may be a significant factor in a student’s choice of university. When guided by such persons alone, several factors must be considered and weigh the positive and negative impacts of such services. For instance, agents have years of experience and may be the distinguishing factor when applying to specific institutions. They can assure sources in reviewing the institutions and provide insightful feedback. They can also offer realistic chances of acceptance to quality programs and guide the form of communication in the mother tongue of the prospective student, who may find it to be a comforting and assuring method for making decisions.
On the other hand, such consultants may introduce bias based on their previous work experiences, such as application successes and failures, personal convictions and assumptions, and sentimental attachments. They can also choose to mismatch the candidate with available university programs. Moreover, they may have a limited scope of awareness as they may not know existing universities, government policies, and scholarships. They can be affiliated with some universities, thus providing information about the institutions they represent. Still, the students or their parents must pay some fees to get assistance.
Problem Solution
The problems mentioned above call for designing and implementing a dependable and cost-effective system for the users. Such a system must address the users’ needs, mainly by sifting relevant information, serving it to the students, and suggesting and guiding them to a final decision. The system also must create a baseline for consensus among all the stakeholders, including the public, the university, and the authorities concerned. Consequently, the application must provide solutions to the users searching for information regarding courses and universities and help with how such information relates to their potential and interests. The solution to the problem identified will ensure that the system gives the learners access to institutions, their courses, and valuable information surrounding their services on one platform. Students will have little or no need to seek information about institutions from third-party agents only, if necessary, as a supporting source.
Even though a similar technology has been implemented and used in different business applications, the current one has been given a more novel approach. It gathers global information and synthesizes it to fit an individual’s needs. It achieves this through the following specific functions:
• Allows customers to register on the platform
• Allows customers to create a profile
• Allows customers to view their profiles
• Allows customers to update their profiles
• Allows customers to sign out safely from the platform
• Provides a graphical user interface (GUI) for the various end users.
• Allows customers to search for university, and course information
• Allows customers to filter search results
• Allows customers to save desired institutions and or courses for a later review
• Allows administrators unique to each institution to create a university portfolio
• Allows administrators to view institution details as well as make changes as they see fit
• Provides sign-in and sign-out functionalities for designated end-users
• Produces insightful reports from activities taking place on the platform to super administrators
• Identifies if a customer is a right fit for an institution or meets some of the requirements of the institution
• Provides account recovery options to users subscribed to the platform’s services
Moreover, the system will have three classes: public users, administration, and university. The general users are either potential students or persons who assist prospective students in seeking valuable, insightful, and current information about institutions and courses. The administrator will be the management staff with super admin access to the web application. The university will encompass the staff and management of institutions that will manage data disseminated on the platform to prospective students. These various classes of end users are expected to utilize the web application to their advantage efficiently. They may require familiarity with the terminologies used on the platform’s GUI.
Aims and Objectives
The current study aims at creating assistive technology for supporting student admission through the course and university discovery. The following specific objectives help in further exploring the present research and seek to understand the approaches used to realize such a system:
- To implement a communication system for extracting student information requests via textual analysis, which will serve as a query for presenting insightful data relative to universities or colleges.
- To provide timely, concise, and clear information tailored to the needs of an individual seeking admission by giving answers to queries and guidance for each stage of the application process.
- To provide a step-by-step approach to delivering data to inform prospective students on their choice of institutions, career paths, and potential job opportunities
- To explore the most effective way of providing information tailored to an individual’s inquiry about university programs in the UK.
- To assess the impacts of the implemented software model on the decision of choice college by an individual.
- To explore the insights that could be derived from utilizing such an assistive technology for university institutions.
Key Research questions
The following research questions are addressed to achieve the current project’s objectives.
- What design approach can be used to implement a communication system for extracting student information requests via textual analysis that can serve as a query for presenting insightful data relative to universities or colleges?
- How can the designed system provide timely, concise, and clear information tailored to the needs of an individual seeking admission by giving answers to queries and guidance for each stage of the application process?
- What implementation process can help achieve a step-by-step approach to providing data to inform prospective students on their choice of institutions, career paths, and potential job opportunities?
- What way will be more effective in providing information tailored toward an individual’s inquiry about various university programs in the UK?
- What is the impact of such a software model on the decision of choice college by an individual?
- What insights could be derived from utilizing such an assistive technology for institutions?
Improving application choices is important because these choices impact college enrolment, degree attainment, and labor market outcomes. This project aims to provide a low-cost software model to support students’ decision-making by providing personalized admissions information.
Scope of the Project
The present research assesses the available technologies and existing systems and fills the gap by providing solutions to the identified problems. Consequently, the project will create a web application that supports various mobile and desktop devices with browser capabilities. It is also geared towards achieving a web application optimized to run on several computing platforms, including Apple iOS, google android, and Microsoft windows. Moreover, the project also aims at creating a mobile-first approach to the design and development of the final product. In addition, it makes a prototype to illustrate the proposed system’s look, feel, and design approach. Furthermore, the project also covers requirements engineering, analysis, design, implementation, and testing of the web application and presentation of a minimum viable product for stakeholders’ perusal. However, the project does not cover aspects of advertisement and marketing and membership subscription plans.
Relevance of the Project
The current project addresses critical concerns in the high education sector, especially regarding admissions and choice of courses. It creates a system that can be accessed from any internet and browser-enabled device, making it easier for users to search and access the necessary information to make decisions regarding their courses and universities. Even though there are some existing systems, the current project focuses on providing an application explicitly used for university courses. Moreover, the current project also provides an in-depth analysis of systems and applications that have been implemented, hence identifying existing gaps and those that subsequent studies can fill. As innovation continues and more advanced technologies suffice, new knowledge areas emerge to solve past problems, which is why such an extensive review is considered necessary for continued progress. Furthermore, the project also provides a feasible solution, which upon implementation, solves the problem as expected. This enables the project to create a scenario in which the proposed system can be tested against various issues it is supposed to address and other emerging challenges.
Legal and Ethical issues
Retrieving data from various government websites, education sectors, and social media platforms may raise security concerns. How is the integrity of the client performing the request? These concerns may be in denial of service, which occurs due to repeated web page requests, thus, slowing down the web server, causing a hindrance in the network bandwidth, and affecting the speed of downloading web pages. To resolve such a challenge, one may request permission from site owners to download data, utilize APIs, retain the same content after it has been downloaded once to avoid bandwidth wastage, and reduce the speed of crawling data from those web pages.
Another issue to consider is government policies, such as compliance with the current Global Data Protecting Regulation (GDPR), which is concerned with managing the data collected. This must be considered as the privacy of stakeholders is of utmost priority. If not adequately handled, it may render the application useless and tarnish any sense of worth and integrity already established in the minds of its users.
Project Outline
The project has been organized into the following sections: introduction, literature review, methodology and work plan, implementation and technology, and conclusion and future work. The introduction gives the study background and states the existing problems and the proposed solution. The section also provides the research’s aim and objectives and defines the research’s scope and project. The second chapter gives an extensive literature review on the theories regarding assistive technologies, the concepts of data mining, and the Internet as a source of infinite information. The literature review also presents past research on recommender methods and their various classifications. Moreover, chapter two also reviews relevant existing systems, including collaborating filters, association rules, clustering methods, and similarity methods. These parts explain the course recommendation steps in the existing systems. Furthermore, the section presents the proposed system approaches, including the general requirements.
The third chapter documents the techniques used in the research and the development of the proposed system. It explains the agile methodology used, the methods of elicitation requirements, and the breakdown of the structure. The section also presents the product details, flow diagram, and work schedule. Moreover, the risks and contingency plans are also presented in the third chapter. The fourth chapter describes the implementation approaches and the technology and resources used. It explains the system developed in detail, including the customer portal, the university admin portal, and the recommender systems used to give the system its fuller functionality. Moreover, the chapter also explains the potential impacts of the system and the project, including its benefits and demerits. The last chapter concludes the research, reflects the overall projects and suggests future work.
Review of Related Literature
This section of the study provides an extended background of theories and concepts explored by other researchers in the past. It builds on the scientific findings regarding innovations and assistive technologies used in education. While most assistive technologies have been primarily concerned with students with various disabilities, the current research gives an in-depth understanding of computer and software-based assistance using algorithms. The chapter is divided into a literature review, a related software application review, a review of the relevant existing system, and the proposed system.
Literature Review
The literature review section explores the theories and concepts of assertive technology and the relevant studies that have shaped the development of systems in the past.
Relationship between Assertive Technology (AT) and the Human Activity Assistive Technology (HAAT) Model
The teaching and learning environments use three different types of technology. Consumer technology is available to the public and can be purchased from any shop, such as a smartphone. Instructional technology is a system used to facilitate learning and consequently improve the student’s performance, such as interactive whiteboards. Students with some disabilities mainly use assistive technology to achieve the learning outcomes as those without such disabilities. AT gives the cognitive or physical ability to those who lack them so they too can successfully meet their learning needs. In addition, AT used in the learning environment enables the students to navigate existing barriers they encounter, thereby helping them to actively involve themselves in the lessons, thus maximizing their educational outcomes. According to Zilz and Pang (2021), the ATs adopted for a learning purpose must be student-centered, addressing each learner’s needs. AT designed must have a framework critical in the decision-making process in evaluating and selecting the system.
The Human Activity Assistive Technology (HAAT) model is utilized by occupational therapists (OT) to integrate AT into their services. The model considers human interaction with various tasks and AT. Technology is applied where the OT and the system user can manipulate the surrounding factors or environment to maximize the participation and achievement of the individual. Consequently, this assertion indicates that the environment should be adjusted, not the individual. Including the AT in the learning process enables the user to access the environment to achieve the educational outcome. The Human Performance Model (HPM) also reveals that a system involves a person, an activity, and a context. Consequently, the HAAT model extends the HPM by stating that humans, an activity, and assertive technology all interact in a given context. Therefore, the HAAT model reveals that assistive technology applies not only in cases of a student with disabilities but is also used by any other human who does an activity.
The Concept of Data Mining
The amount of information stored in various electronic forms has grown faster over the last decade. Several companies collect multiple kinds of information about their processes and customers and relate such data to make critical decisions in the successful operation of the businesses. Such data form the core asset of the firms as they make the organizations track their performances and forecast future outcomes. According to Rao et. (2019), the scientific community indicates that an experiment can produce terabytes of information. Consequently, there has been an increased demand for tools and techniques for analyzing large volumes of data collected by different entities. More significant challenges have resulted from storing such data, let alone the analysis. Data mining has emerged as a necessity for analyzing large chunks of data, making them useful in the daily operations of businesses. Ray and Saeed (2018) define data mining as getting specific trends from a large amount of stored information. The term “process” in the definition of data mining is crucial because it encompasses a vast array of methods, tools, and algorithms from statistics, machine learning, and database management.
While data mining is a critical process, it is a step-in knowledge discovery. Several other processes occur before and after data mining, including data cleaning and visualization. These supportive processes in data mining have also advanced over the years. According to Lu and Holubová (2019), structured data, for instance, relational data, was used in most cases. For example, a system would be designed to discover the correlation between goods a customer purchases from a store and the likelihood of buying another product. Such a system can reveal that a user who purchases diapers also purchases kids’ toys. However, the emergence and widespread of the Internet and integration of different heterogenous data sources have resulted in extensive data amounts that are not rigid, known as semi-structured data. According to Sabri Ahmad and Man (2018), semi-structured data is data with some implicit regularity but lacks a schema to conform to such data. Most databases use semi-structured data, usually in the form of eXtensible Markup Language (XML).
The Internet has increased the number of data sources made available and accessible to users. In most cases, these sources of data are stored, requested, and exported in different formats that are different from others in terms of structure, consistency, and regularity. Such data types sometimes fit the specifications of the relational model, though they also have their unique implicit structures, hence referred to as semi-structured data. In educational internet systems, for example, the home pages of student portals of different universities vary greatly, even though they have some basic similarities. For instance, they will all have the name and contact details of the profile owner. Some home pages introduce other links to departments, activities, past events, upcoming events, or other critical notices. Consequently, these pages do not have a specific schema as expected in the relational data structure. Moreover, the pages are mostly interconnected using links, though there exists some inherent structure making the individual pages. For example, each student page may be created from a given template, exist in a similar setting, and have some converging interests.
The other example of semi-structured data is the integration of information. Online shopping has become significant activity on the Internet, and the vendors have made it possible to compare different products, in a concept referred to as comparison shopping. While it is possible to assume that each shopping website may have a regular structure, the information that results from integrating such online shops becomes irregular. This happens due to the different products and information provided on each site, which consequently infinitely increases the points of irregularities. For instance, an online site selling shoes may give different information from another site selling similar types of shoes. However, other information about the product is likely identical in each shop selling the items, even though such data must not necessarily be identical. Integrators may find great value in identifying the products with standard information as they can use the mined data to present the features common to each item in the same categories. This makes it easier for clients to compare similar products from different vendors.
The two examples above explain why it is critical to discover the implicit structure of any given semi-structured data. The discovery of structure is an essential aspect of data mining in semi-structured data, whether the structure discovered is considered approximate or imprecise. Consequently, there is a compromise between the conciseness and precision of the structure discovered during the data mining process. The tradeoff relies heavily on the specific application and the semi-structured data. For instance, grouping together all the items sold on the web, some of them will exhibit similar features such as the price, name, or even image.
In recent years, the eXtensible Markup Language has emerged as a method for certain data descriptions and exchanges. Spoth et al. (2018) indicate that most of the XML data can be grouped as semi-structured data though they have their unique Data Type Definition (DTD). Such data are considered semi-structured because DTD primarily encodes all the possible occurrences of similar data types even though specific data instances have unique features defined by the DTD. Consequently, DTD is usually more prominent than the actual data instance.
The Impact of the Internet on Data Mining
Data mining is the basis for the present study, which uses information provided to the system to make recommendations. The Internet has played a crucial role in providing the needed information through databases and search engines. However, Ferri, Grifoni, and Guzzo (2020) reveal that the information available on the Internet is not sufficiently structured, hence can make students feel overwhelmed by the amount of information overload. Apart from the large volumes of unstructured data, the Internet is filled with irrelevant, biased, or false information that has misled many in other areas (Ferri, Grifoni, and Guzzo, 2020). Consequently, such spaces rarely offer complete information and sometimes even provide inaccurate information as they are designed to exploit weak mental points that may present judgmental traps or promote weak reasoning. While a lot of effort has been put into assisting students in securing college or university admission through third-party agency companies’ web applications, only a few projects have attempted to help learners get insightful resources about universities and career opportunities.
The major projects that have been used in achieving this insightful student information have been associated with large companies that require significant amounts to be owned. These include Google and Microsoft Bing search engines, which have also been used in general cases. The closest project relating to a course and university search engine was recently developed by the Universities and College Admission Service (UCAS) in a project known as “discoveruni,” aiming to provide students with information about colleges and academic programs. The project was meant to provide a different model for capturing candidates’ queries and communicating and displaying the necessary information needed.
Recommender Methods
Humans rely on choices and recommendations to perform various tasks each day. However, as the information on the Internet continues to grow, it becomes challenging to search and decides what to choose. Consequently, new technologies must emerge to help Internet consumers easily navigate the information they need. Recommender systems are critical applications that recent studies and manufacturers have tried to explore. Several recommender systems are currently used on the Internet to help users select items systematically based on their preferences. A recommender system involves steps such as user data analysis, information extraction, and prediction of the items to users (Kulkarni, Rai, and Kale, 2020). RS combines several user data such as item rating, item features, transaction history, user demographic data, comments, and contextual data. Kulkarni, Rai, and Kale (2020) reveal that RS should enable the users to get the items they are looking for as quickly as possible and to remove all other information that overloads the system. The RS used by websites, such as online shops selling electronics, makes it possible for a user to find a given electronic product following recommendations in various categories.
Recommender Methods Classifications
Four groups are used to classify recommender methods: collaborative, knowledge-based, content-based, and hybrid. A content-based recommender (CBR) method primarily proposes the items that a user is likely to target based on their affinity between various items and user profiles while ignoring the data collected from other system users. On the other hand, in collaborative ones, the system recommends the items to the targeted users based on data similar to the users’ preferences while ignoring any features of such items. Knowledge-based ones exploit the points of reference using artificial intelligence, thus reducing any match between the targeted items and the users. This method uses extensive knowledge regarding item features and the ratings provided by the users. Consequently, hybrid approaches are used to combine knowledge-based and content-based recommender methods and the collaborative recommender method.
Content-Based Recommender Methods
CBRs propose the items to the users based on similar features between the unseen contents and the users’ preferences or past interactions. A system may attempt to correlate the frequency of keywords in a given site with the users’ preferences. The system creates a user’s interest by analyzing item contents the user has rated and saves the information within the database in the form of cookies. When the user interacts with the system later, it proposes similar items to those the user rated. Various techniques such as machine learning, heuristics, and approximation theory are used to analyze the items’ contents and create some form or regularities that become critical in recommending potential desired items. The methods used include Bayesian classifier, Association Rule Mining, and nearest neighbor formation.
A system designed purely for a content-based recommender does not provide total efficiency. According to Javed et al. (2021), such a system can only become effective when used with textual data only. On the contrary, a pure content-based recommender has proved challenging in non-textual information such as video, images, or audio. Javed et al. (2021) reveal that CBRs can only become effective when closely related item contents are attached to the users’ profiles, making the user only get items similar to those searched in the past. Therefore, the system becomes ineffective when the user wishes to find new items that have not been explored in the past. However, Lops et al. (2019) indicate that filtering can be done to help solve the issue posed by CBRs. Moreover, the problem can be addressed by using diversity to help rank items recommended to the user. Furthermore, this also proves challenging for new users because the system does not have any reference to recommend the items needed by the new user. This issue is usually referred to as a new user problem because the user has insignificant ratings that can be used to make judgments and recommendations for new items.
Collaborative Recommender Methods
Collaborative recommender (CR) methods recommend its items to users by comparing their past preferences and those of similar users. Thus, CR predicts items based on the system users’ ratings and not the machine analysis of the contents. This indicates that the users are grouped into clusters based on their past preferences. Upon creating the clusters, the ones with the strongest correlations with the users become critical to the recommendations made for the user. Various analytical methods such as association rules, correlation-based, and Bayesian network techniques are usually used to represent the affinities combining the outcome of similar users in the system.
CR can fix some of the problems associated with CBRs because CR does not depend so much on machine-filled content errors. The CR methods recommend items to users based on the user preferences and quality of items instead of focusing on the item properties, which makes them suggest items to the user that can be different from what the user had liked in the past (Rivera, Tapia-Leon, and Lujan-Mora, 2018). Moreover, CR can also handle various types of content, including videos, images, audio, and artwork, which tend to enhance the quality of recommendations to the user. Even though their excellent outcomes compared to CBRs, CRs suffer some issues such as problems associated with early rater, sparsity problems, and the grey ship problem. According to Zhu et al. (2019), an early rater, also known as the cold start issue, occurs when a user requires recommendations for a new item with no ratings or for a new user who has not registered any information that can be compared with peers. Such a scenario makes the CR not work as expected as it lacks the necessary information to recommend an item for the user. Yang, Zhou, and Cao (2020) define the sparsity problem as an issue that happens when the items available in the system are not enough to identify which ones are similar and which users have similarities, resulting in poor recommendations. The grey sheep problem results from poor recommendations to users whose historical data are added compared to other users within the system. Thus, these users whose data are utilized to make the recommendations do not have similar preferences.
Knowledge-Based Recommender Methods
Knowledge-based recommendation (KBR) methods are used primarily in domains that require a profound knowledge of the product to identify and justify the solutions needed by the users. For instance, if a user wishes to purchase books or other items and use their financial services, the system may need more information and intelligence mechanisms to support the solutions that help complete the transactions. Consequently, the system needs a wide range of information regarding the product, marketing, and sales information to complete the transaction. KBR systems utilize inferences that use AI to refer to a match between the product and the buyer based on the product features rather than the ratings from users’ preferences.
Hybrid Recommender Methods
Each of the pure recommender systems has a deficiency that other addresses. Consequently, hybrid recommender systems have been proposed to combine the benefits of content-based, knowledge-based, and collaborative methods. The primary goal of such a method is to enhance the accuracy of recommendations and limit the drawbacks associated with traditional or pure recommender systems. According to Sharma, Rani, and Tanwar (2019), hybrid recommender systems are built based on several taxonomies, which include meta-level, weighted, feature combination switching, mixed, feature augmentation, and cascade. The research by Sharma, Rani, and Tanwar (2019) found that most hybrid recommender systems combined two methods, especially content-based and collaborative approaches, or used data mining methods.
Other hybrid systems have also been used to select courses targeting universities using affinity between the programs a particular student wishes to undertake based on the courses other learners take. Sharma, Rani, and Tanwar (2019) developed a collaborative recommender method utilizing association rule mining to find specific course trends and suggest relevant courses for students. The study initially used clustering to categorize users based on their course grades and applied the nearest neighborhood technique to select learners’ groups closely related to the target users. Lastly, the system applied association rule mining to give the course recommendations to the system targets.
Review of Relevant Existing System
One of the most relevant existing systems related to the project described in this paper is the automated recommender system used in course selection. Several companies use such systems and operate using the principles utilized in the project described in this paper. Consequently, this section reviews the techniques used by these systems, specifically collaborative filtering (CF), association rules mining (ARM) concepts, clustering methods, and similarities techniques.
Collaborating Filtering
CF methods have been used for years and have resulted in satisfactory recommendations to the target user. These techniques are based on the preferences of users classified together by the system by suggesting the items that have been selected by other users whose interests coincide with the target users’. Consequently, the primary goal of the algorithms used in any CF system has mainly been associated with predicting item ratings from a specific user based on the items’ ratings of similar users. The data from users closer to those targeted then become strongly relevant to the targeted ones. According to Zriaa and Amali (2020), this is achieved because the algorithms used with CF systems use k-nearest-neighborhood programs to suggest the items. Zriaa and Amali (2020) indicates that such systems employ the following steps to reach the final decision:
- Construction of a profile vector used in line with the target user by gathering the ratings of the user’s past items.
- Computation of the pair-wise similarity between the profile created and that of any other nearby user.
- Construction of target users’ closest neighbors, which are sorted descending using the same value and taking the k-users with similar data features as the user.
- Use the nearest neighbor list to compute the prediction rating for a new item recommended to the user.
Association Rules Mining
Several scholars and companies have explored ARM for a few years. ARM is mainly used to get the correlations between elements in a dataset by getting the items that appeared together in several transactions. Each dataset must have confidence and support, some of the most essential quality heuristics utilized in measuring the association rule interestingness. Confidence is used to measure how valid the rule X ⇒Y is, where the lesser the exceptions, the greater the validity of the rile. On the other hand, support is used to measure the rule’s efficiency (Ghafari & Tjortjis, 2019). According to Sulova (2018), rules with support and confidence higher than the target user’s minimum corresponding values are known as interesting rules. Scholars and designers have devoted significant work to ensure that the algorithms used in association rules have become more efficient. ARM has been used to recommend items to the target users. Neysiani et al. (2019) recommended a method to offer a personalized web experience using association rule to discover users’ past transactional information. The items are recommended by matching a user’s session with the trends discovered through the association rules on the user’s transaction information.
Clustering Methods
Clustering techniques involve grouping information into different classes to ensure that the objects within a given cluster have similar points of comparison but are dissimilar to those in other clusters. The dissimilarities are most identified using the values that describe objects, especially distance measures. A designer may choose from several clustering algorithms based on the data types available and the specific application used. Kalra, Lal, and Qamar (2018) indicate that the k-mean clustering method is utilized because it is simple and suitable for supervised numerical data such as those for student course grades. The technique is considered the most straightforward unsupervised algorithm, which does not depend on predefined classes to address specific issues. Its primary idea is to create k centers for each of the clusters within a dataset. Each point of a set is taken and associated with the available nearest centers. A new k is then re-computed based on the clusters obtained earlier (Kalra, Lal, & Qamar, 2018). The new k centers and new bindings are done to create an association between similar data points and the nearest created centers, which generates a loop. The loop results in a series of k center changes until there is no more room for change.
Similarity Methods
Similarity methods are used to reflect how strong two features or objects are related to each other and usually range from -1 to +1. It is possible to measure the similarity between two items based on the data type used. Such measures are usually used to compute the similarity of items as denoted by the interval scaled variables, which are continuous measurements of linear scale, including grade, weight, temperature, and height.
Course Recommendation Steps in Existing Systems
Existing recommendation systems using collaborative methods recommend courses to the learned utilizing the information obtained from the historical data of other students. Such systems recommend the courses and specify the expected marks obtained to attain the programs. Adams and Wilson (2020) indicate when a student selects a course suggested by the system, the learner must have obtained a grade corresponding to the program. This makes it possible for the students to choose from a variety of elective courses and provides data on other students taking the same course, thus creating an association between them. Getting course association rules involves building course datasets and mapping them to each other based on whether the courses are elective or compulsory (Amrutkar, Mahakal, & Naidu, 2021). The system then uses each student’s transaction containing all the courses the learner takes. The system uses unique minimum support, the course dataset, and the minimum confidence as the input. However, it produces course association rules satisfying the constraints for confidence and support to act as the reference values. The system utilizes the created rules to recommend courses for the users.
The steps used by the system include clustering, finding related students, mining for courses, and finally, recommending courses. Clustering step groups course datasets for each student into the same class. The k-means algorithm is used, where the students’ mean value represents the cluster. Wu, Song, and Zhang (2022) indicate that one of the most familiar distance measures is the Manhattan distance measuring how far two data objects are from each other. The clustering step categorizes students with the same clusters into one group.
The second step for finding related students involves selecting a group with the most similarities to that of the active user obtained by comparing the learner’s previous grades with the mean of other students in the same cluster. The n-nearest neighborhood method is used to choose the most similar groups generated from the clustering step. This results in representing all the p-dimensions based on their specific pattern spaces. In the case of a new student, the n-nearest neighborhood seeks and finds the pattern space that can help suggest a cluster closest to that of the new user. The next step involves course mining, which uses association rules mining to get the courses based on the algorithm for finding similar users.
The last and most complicated and vital step is the course recommendations. This is the step that the students want to interact with, even though other critical steps and processes are happening in the background. This stage involves using the course association rules from the courses mining step to suggest elective courses for the students. Liu (2018) suggests the rules used to generate the association rules are usually written as [c1:g1] … [cn:gn] [cn+1:gn+1] … [cm:gm], where [c:g] is a course c with a grade g.
Proposed System
There are several internet-enabled devices in the present age, most of which can be used with a web browser to access information from a given site. The proposed web application is expected to run on several computing devices such as mobile phones and personal computers with browser capabilities. In most cases, the hardware components are not very critical if they can be used to access the web. However, there are concerns for some other devices, which, even though they can access the web, do not give a fuller understanding of pages visited. Consequently, the proposed system can only be used by the mentioned types of devices.
General System Requirements
The system developed in this project is expected to have the features in Table 1 below:
Table 1. System Requirements
The project uses the MoSCoW prioritization technique to fine-tune user requirements, as shown in Table 2 below. In the table, the users defined what they expected to get from the system, which informs the development of the system.
Table 2. MoSCoW Prioritization
High-Level Use Case Diagram
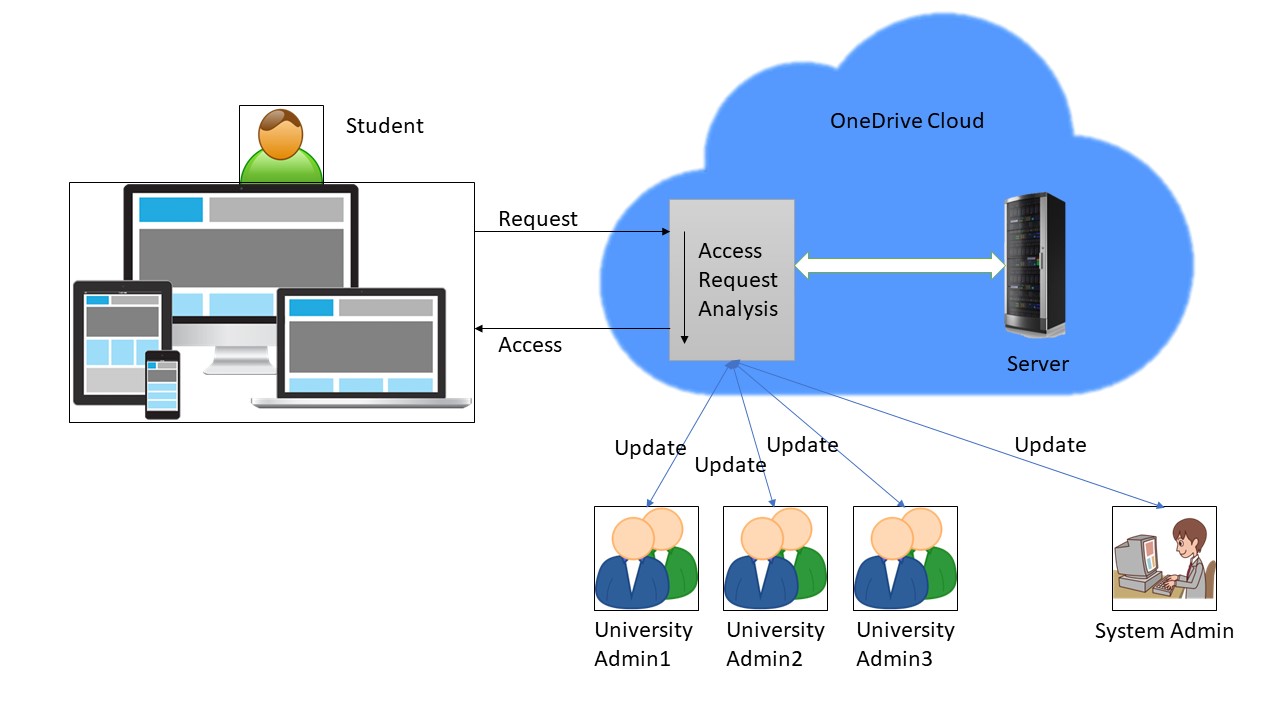
The diagram in Figure 1 above illustrates the system’s use case interpretation by depicting the proposed system’s various actors and their respective interactions with the system. The system’s actors consist of the super administrator, whose roles are identified in the requirements specification. On the other hand, the use case points out the system’s functionalities. The actors of UniSeek systems will perform operations such as login, search, save, delete updates, create a profile, add university, and others listed in the requirements specification document.
Method and Work Plan
This section accounts for the methods and plans followed to complete the project. The literature and the identified gaps have informed the techniques adopted in the process. Software development requires a straightforward method to ensure everything runs according to schedule to realize the final minimum viable product and, eventually, the entire product. In view of the proposed project, the agile framework provides a suitable methodology for achieving a minimum viable product within the shortest time while maintaining high-quality standards.
Agile Methodology
Regarding the project, the desired approach to the development process is the agile methodology. Let us briefly consider the rationale behind this decision. Firstly, we must define the agile methodology. This strategy involves breaking work down into smaller units of tasks that may be worked on independently, learning from mistakes, using testing aspects for evaluation and validation, and adopting new technology as it becomes readily available (Schwaber, 2004). There are several reasons why the agile framework is the preferred choice for the proposed project. Its characteristic features set it apart from other methodologies.
The agile framework places more emphasis on individual communication rather than huge chunks of documentation. It focuses on the business need by ensuring a minimum viable product fit for purpose. It encourages strong collaboration between stakeholders of the project, thus ensuring that at every given time, the stakeholders are kept abreast of the current state of the project and its deliverables. This dramatically reduces the risk of producing an undesirable product. Agile strategy never compromises quality as it sets quality criteria from the outset of the software development life cycle. Another feature is that agile focuses on developing iteratively. This means that change is greatly embraced during the development process, and feedback from stakeholders is built into every iteration, thus ensuring a robust and improved product. Agile also stands on the premise of building incrementally from firm foundations; in other words, proper planning and evaluation of project resources and expertise are needed.
A huge factor of the agile approach is that small-sized groups can exercise it on projects of varying sizes, whether small or large. The proposed system is common, but a constant factor is that customer requirements are constantly changing, and systems evolve. This is one of the biggest strengths of agile as it suits systems whose problem spaces are continuously evolving. There is no doubt that universities are constantly changing their methods of advertising their brands to prospective students. On the other hand, individuals are continually looking for better ways to find insightful information to help them make the right choice as they step into another phase of their lives. The principles of agile include:
• Focus on the business need: Ensures that the product is fit for purpose and guarantees a minimum viable product (MVP).
• Deliver on time: Focuses on the project’s priority timeboxes the work and always hits deadline.
• Collaborate: Promotes a one-team culture and strong teamwork among stakeholders.
• Never compromise quality: Sets and adheres to quality criteria from the outset. Build quality into a project and its deliverables by constant review.
• Build incrementally from firm foundations: Sound planning, including evaluation of required resources and skillset needed for the project.
• Develop iteratively: Embraces change to build the right solution and constructs business feedback into each iteration.
• Communicate continuously and clearly: Encourages honesty and transparency to manage stakeholder expectations during the project’s life cycle. Keeping documentation lean and timely.
• Demonstrate control: Monitors each phase of the development process while evaluating the project’s viability.
We will utilize the Scrum technique with respect to the agile methodology. This technique mainly concerns short iterative and incremental developments known as sprints.
A benefit of the agile methodology is that the risk of constructing the wrong solution is significantly reduced, and the final project deliverable will likely meet the user’s specifications. The above statement is only possible due to a continuous iteration and delivery and persistent review and retrospect of the project’s products and processes. Another benefit is that agile encourages small teams and supports small to medium-scale projects like the intended project stated in this document.
Methods of Elicitation Requirements
The current project was informed by the information gathered from the students and other users who seek course recommendation services. Several tools and methods can be used to collect data on user requirements for any technological product. According to Tam et al. (2020), one of the primary methods is the survey of stakeholders, including users, administrators, service providers, policymakers, and legal experts. The current project used a survey as its primary technique for gathering valuable information about the project’s problem space and business feasibility as it concerns individuals who intend to study abroad. The approach was considered beneficial because many stakeholders provided valuable data to probing questions, which ultimately gave clarity to the business justification of the project and its feasibility in today’s marketplace.
The survey was conducted on students and professionals at different levels. Eight participants were selected and responded to the survey questions. They comprised 2 females and 6 males, with 1 aged below 20 years, 1 between 21 and 24 years, 4 between 25 and 30 years, and 2 being over 30 years old, as shown in Figure 2. All the participants revealed they were international students or professionals. The participants also indicated that they used the Internet and Google search engine daily, occasionally, or never using the UCAS system, as shown in Figure 3. The results derived from the survey indicated that students who want to study abroad consider several factors when deciding their choice of university and course, as shown in Figure 4. The significant factors included entry requirements, living and tuition costs, and academic majors. The survey also provided results emphasizing the need for a university and course discovery system that readily provided insightful data to candidates on time while delivering nuanced technological solutions, as shown in Figure 5.
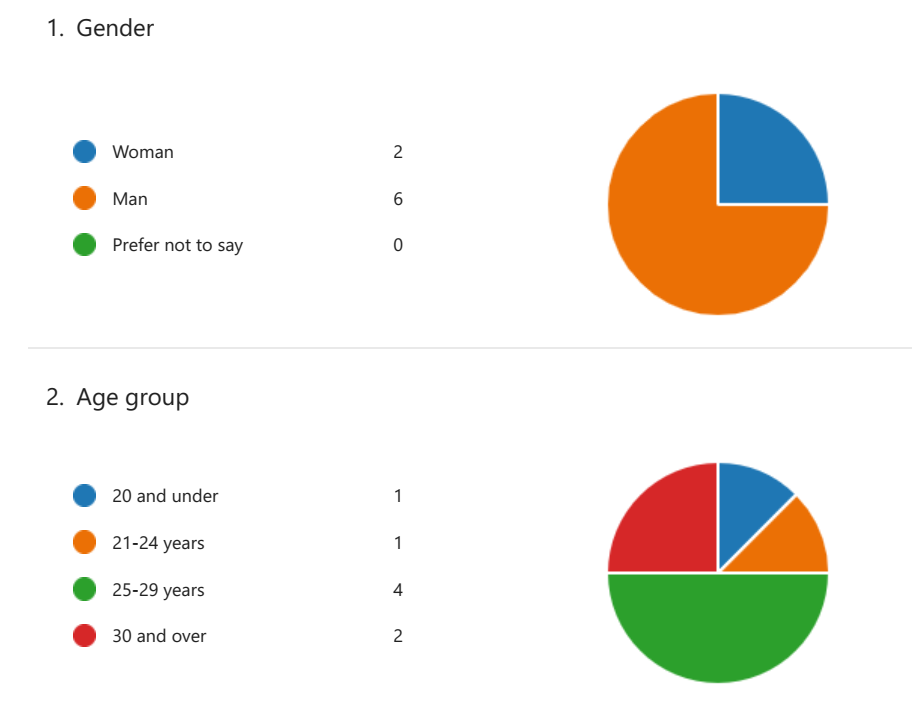
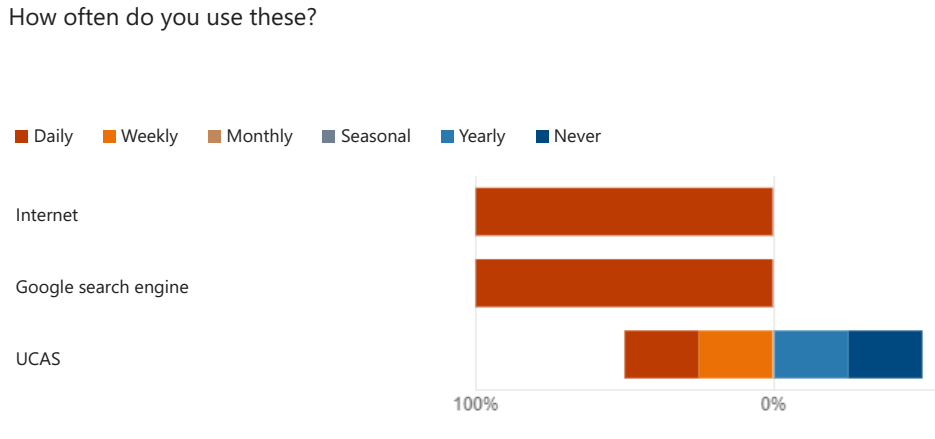
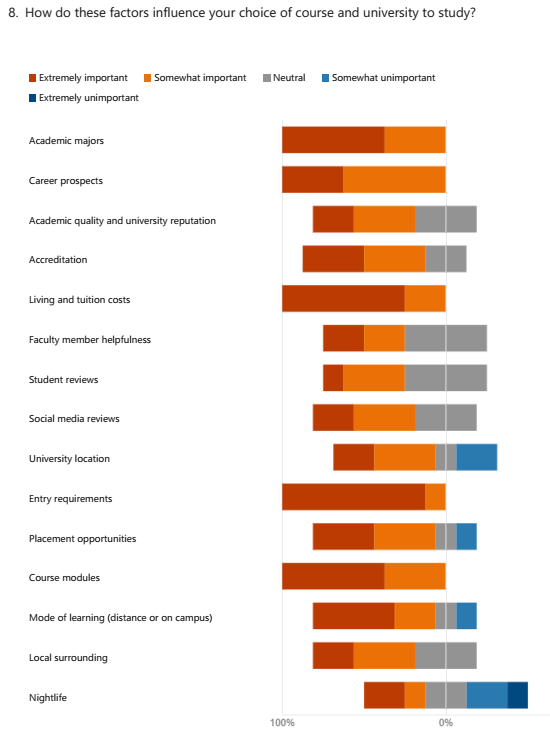
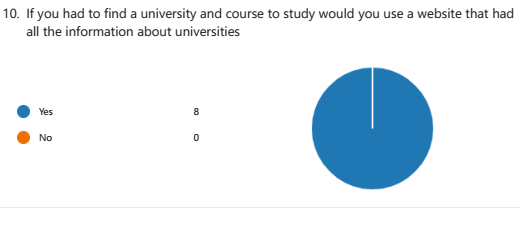
Another technique we employed in eliciting requirements for the proposed system was brainstorming and interview sessions. The essence of brainstorming and interview sessions was to communicate tacitly between a diverse range of stakeholders, including product owners and subject matter experts. One importance of brainstorming is that some requirements may not be clearly stated but inferred. During such deliberate sessions, experts and stakeholders clarify ambiguous requirements, reducing the risk of poor design outcomes and undesirable project deliverables. Interviews also served as a follow-up to survey results, thereby re-evaluating and validating previous assumptions and decisions.
Product Breakdown Structure
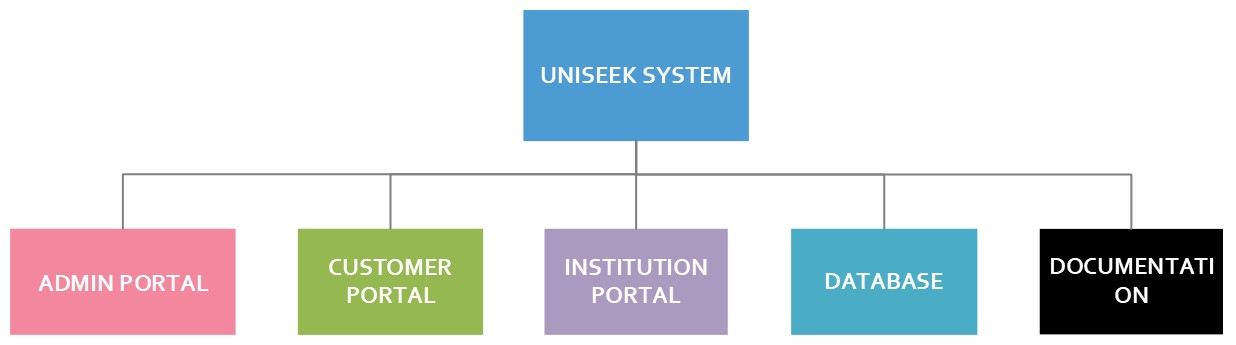
Figure 6 above represents a high-level breakdown structure of the proposed system. It will consist of an administrative portal broken into two parts; firstly, the admin represents a client administrator solely responsible for managing university information specific to their institution. Secondly, a super administrator with complete control and a helicopter view of the entire system and its features. The customer portal provides secure services for customers to find universities and courses and save, sort, and filter for specific information tailored to their needs. The institution portal also provides a platform for client institutions to showcase valuable and current information about their products and services, courses, facilities, and other significant information available. The database will contain the necessary dataset for the entire system. At the same time, documentation provides reports, requirement documents, design documents, and other textual materials containing information about the project, processes, and deliverables.
Development Skills Used in Development
The proposed project deliverable required considerable technical expertise and advice on the cloud technologies and infrastructure to support it. The solutions team consisted of full stack, frontend, and backend developers. The frontend developer was well grounded in frontend technologies such as HTML, CSS, BOOTSTRAP, JavaScript, and jQuery. In contrast, the backend developers had considerable experience using the.NET framework, C#, MVC, and SQL.
Quality Criteria
The project’s resources are housed externally by a third-party cloud service for a long-term period, expected to exist beyond the current project’s life span. The following are the specific quality criteria used in delivering the project.
Compatibility: The system must be accessible on various platforms and devices, including (mobile and desktop devices). The code base was developed using an N-tier architecture with object-oriented and component-based approaches.
Functional Completeness: The proposed web application is fit for purpose according to the end-user’s requirements.
Performance: Web page response time did not exceed the standard maximum of 2.11 seconds.
Usability: The web application provided support for learnability, operability, and responsiveness, reducing the cognitive load of its users as well as protecting users from making apparent errors.
Reliability: The proposed system was simulated to be available 90% 24/7 for customers to access, considering service downtimes
Recoverability: The system must have an existing backup to manage version control in case of an incident or system failure.
Maintainability: The programming paradigms used during the entire development life cycle of the product must account for easy maintenance, ensuring loose coupling.
Scalability: The system must afford a great demand of consumers up to the tone of 20,000 users when the need arises
Security: A secure login must be made available to the system’s users, and proper methods must be implemented to defend against Denial of Service (DOS) attacks.
Detailed Product Breakdown Structure
The essence of illustrating the product breakdown structure is to provide a hierarchical distribution of the system’s components. The approach removes any ambiguity that may arise, provides clear boundaries for the project’s deliverables, creates a foundation for the work packages, and provides a precise requirement specification of the project. As shown in Figure 7 below, each branch has specific functions that users can access through the system. The major components include the admin portal, the customer portal, the university portal, and documentation. The admin portal enables the overall system admin to manage all other users and create updates to the software. The university portal allows university administrators to update details of their specific institutions, thus providing up-to-date information regarding the courses offered by their schools. The customer portal contains pages that enable students to update their data and search for courses and universities. The customer can also read more information regarding the courses a university offers.
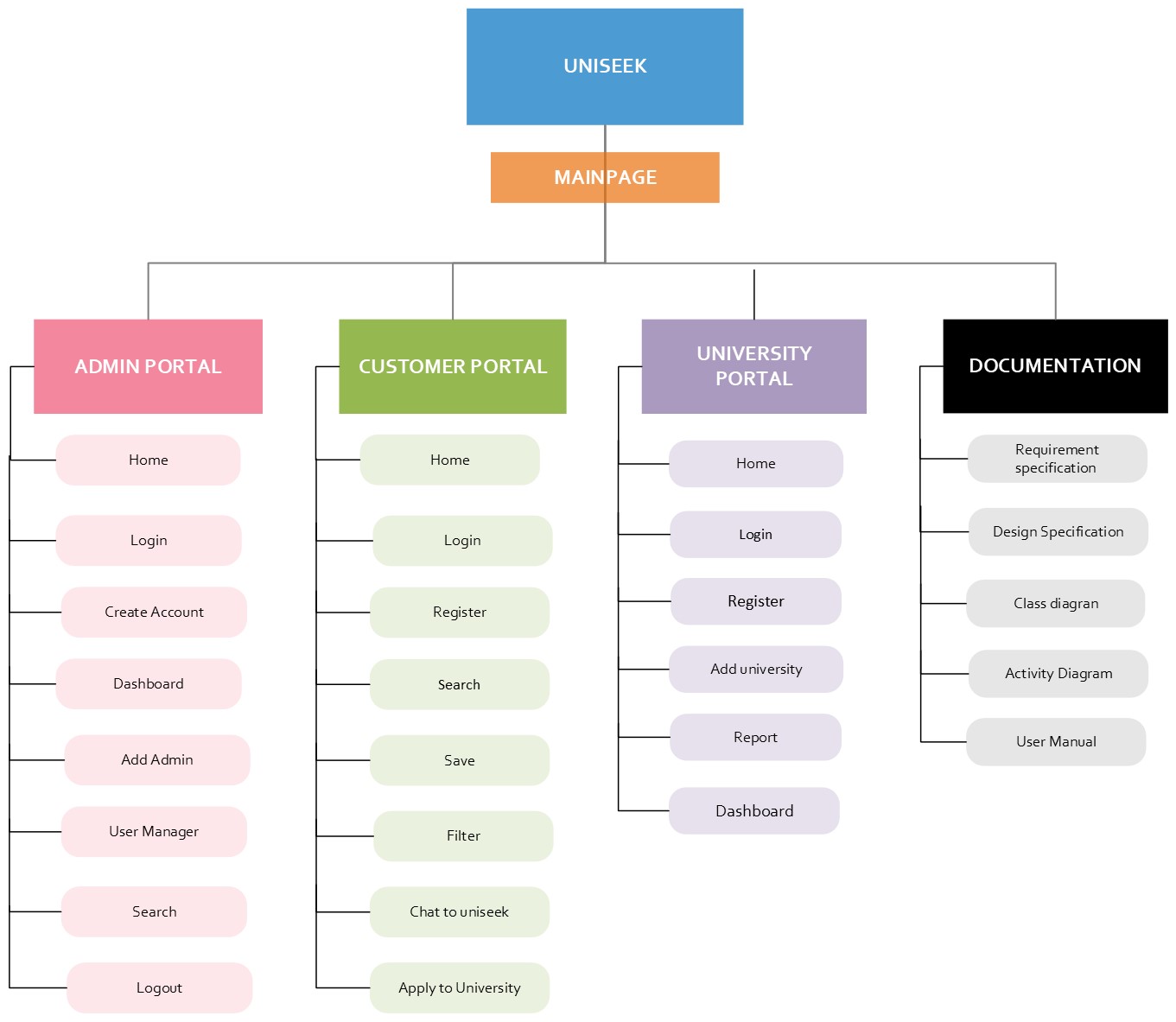
Product Flow Diagram
The following of the system is critical as it shows how different operations occur as driven by the software. As indicated in Figure 8, the project uses an iterative design, enabling every bit of the project to continue simultaneously. This is possible because the developers are working on different modules and testing them before updates. This ensures that the final major update sent to the frontend works harmoniously with all the modules, thus making the system continuously deliver its intended solutions.
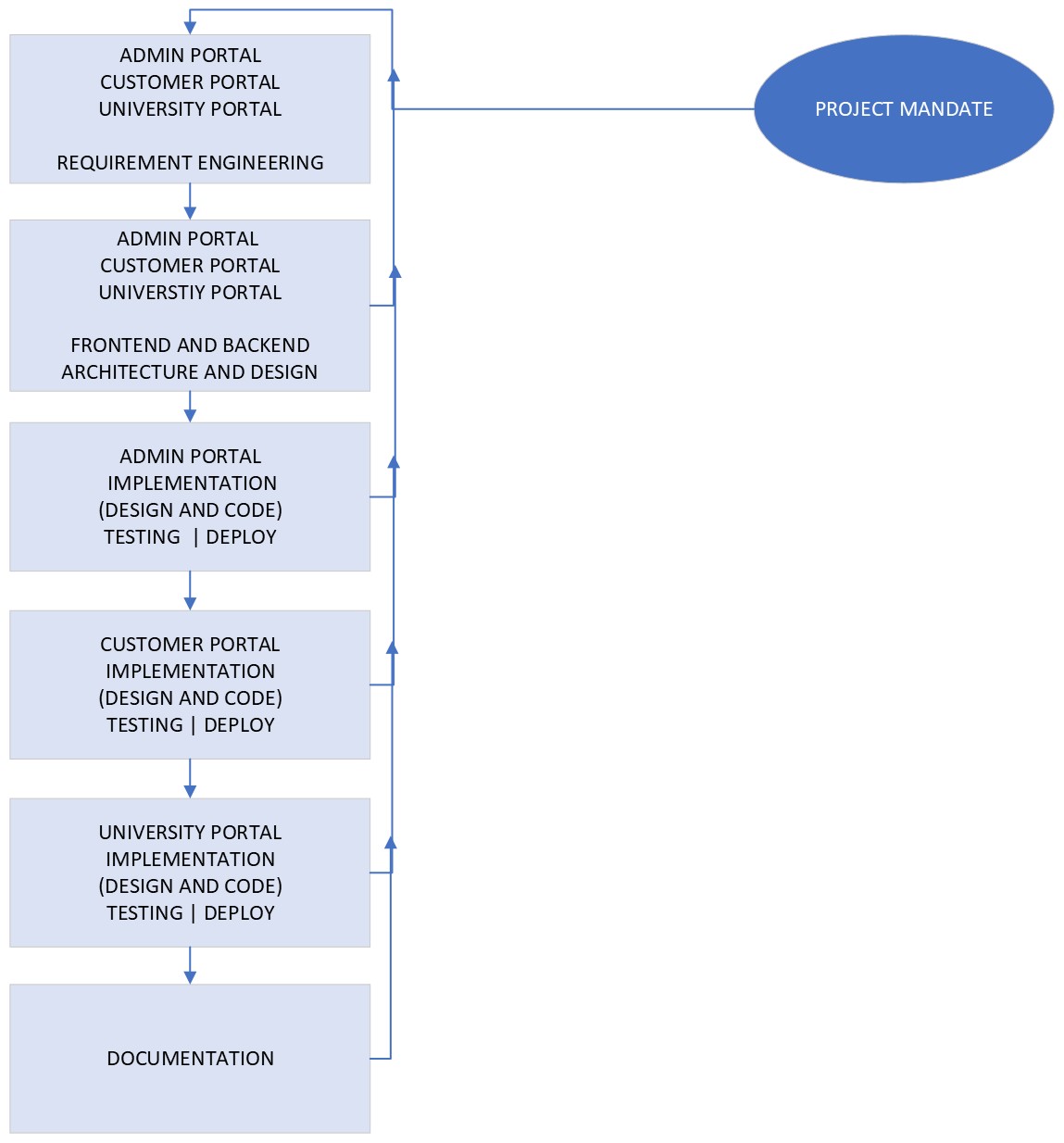
Gantt Chart
Figure 9 below illustrates a high-level workflow schedule of the project. It is essential to note that the various stages of the software development life cycle will be implemented using the agile approach to cater to high-quality deliverables within time, costs, and budgetary constraints. The typical Software development life cycle consists of various stages, including Requirements engineering, system, and software design, implementation and testing, operation and maintenance
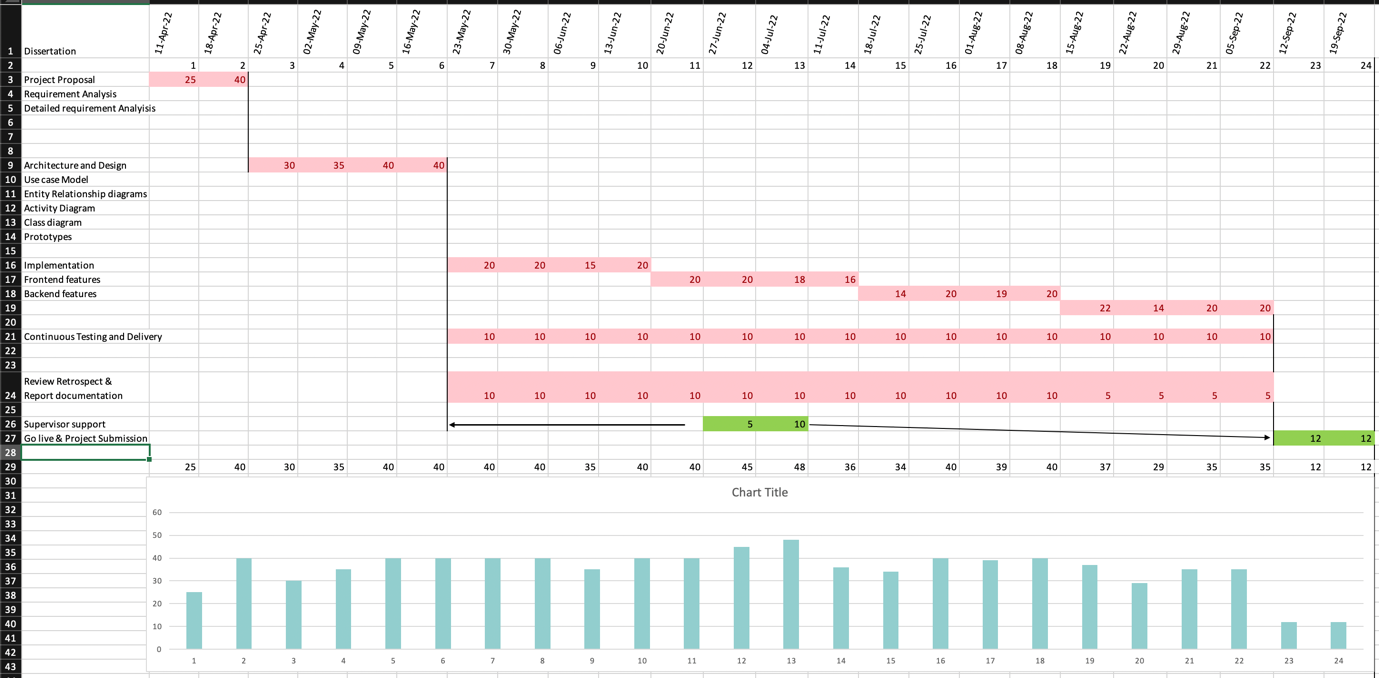
Risk Analysis and Contingency Plans
As important as it is to have a working plan for the project, it is equally essential to identify factors that may impede the smooth running and success of the intended project. Table 3 below seeks to identify potential risks to this project and contingency plans to mitigate them.
Table 3. Potential Projects Risks
Implementation and Technology
This chapter gives the details of how the system was implemented and the technology and resources used to complete the project. The first section presents the technology and resources, detailing how the system development was achieved using various languages and frameworks. The section part of this section gives the flowcharts of two portals: the customer and the university admin’s portals. These two are presented as they are the major frontend sections of the system. This part does not present the developer or admin portal and database sections of the system. Moreover, the flowcharts are presented as the reflection of what goes behind the system, thus summarizing what happens in the code.
Technology and Resources
The answer to the question of the choice of technology is “it depends.” There is no one size fits all, and as a software engineer, the choice of technology to utilize in software development is critical as many factors are considered. Such factors may include the project’s scope, time, resources, and budgetary constraints. In addition, software quality factors such as performance reliability, scalability, maintenance security portability, interoperability, and usability influence the selection of suitable technology. Concerning the intended project, we will consider using technologies such as Microsoft.NET and.NET Core, platforms for building running on experiencing distributed applications. It spans clients, servers, and services. A vital benefit of the.net framework is its two major components, namely.
The common language runtime (CLR) defines a common programming and standard type system for cross-platform, multi-language development. Also integrated with the CLR is the extensive set of framework class libraries (FCL). A rich class library support provides developers with various core functionality such as ASP.NET, web server, ADO.NET, and XML to build different types of robust applications with complex functionality.
In addition, the.NET framework provides an enhanced application development model – ASP.NET Model View Controller (MVC) supports rapid application development (RAD) and object-oriented programming techniques, which support the build of dynamic sites by clean segregation of concerns. It also allows full access to working with HTML, CSS, and JavaScript elements, essential in delivering aesthetically pleasing applications. We will also consider using other frameworks such as JavaScript and cloud technologies like Amazon Web Services AWS, Google Cloud Platforms, and Microsoft Azure.
Below are a few quality factors concerning the.NET framework:
- Performance: Before being executed, all ASP.NET pages are compiled. Overall, compiled code performs significantly better than interpreted code. Furthermore, ASP.NET supports caching, allowing ASP.NET to reuse the compiled version of the source file that has not been changed since the last compilation. Caching improves ASP.NET performance by reducing the time required to compile a page repeatedly.
- Security: The.NET framework provides access to all the built-in security features, such as code access security and role-based user access security. It also offers various authentication options.
- Manageability: the clean separation of concerns in the model view controller pattern supports the ease of maintaining and updating web applications as a developer can concentrate on different layers of this software architecture without interfering with the normal functioning of the other layers.
- Scalability: ASP.NET allows web applications to be run in a web garden and a web farm, providing high availability, performance response, and load balancing.
While person-hours are saved as the.NET framework takes care of small tasks so one can focus on the objective logic of the program, the application’s stability is also ensured due to garbage collection and interoperability rules such as Common Language Specification (CLS).
ASP.NET MVC development service provides substantial, patterns-based progress to build dynamic sites that allow clean segregation of considerations, providing complete control over markup for pleasant, agile development.
Testing: The benefit of writing and running unit tests helps ensure the efficiency and effectiveness of code.
The System
As noted in the survey conducted and whose results led to the implementation of the current project, customers are the key beneficiaries of the system. The surveyed participants reported they needed a system that can recommend a course based on the information available on the Internet and those they share with the system. The response to such needs is implementing a system that factors in significant needs and uses them to find the best match for each user. The customer portal is where the students interact with the system. They are expected to feed the system with the needed information to help it understand the user and utilize its algorithms for recommending a course for the learner.
The Customer’s Portal
As shown in Figure 10, the customer has several input requirements for predicting courses and relevant universities. During the student records phases, the system requires the customer to enter information such as their major subjects, secondary subjects, grades obtained, interests, and past courses, if available. The significant subjects item is used in this case because education systems require students to take mandatory subjects and select others from those provided by the curriculum. In most cases, such subjects are considered foundational subjects, which most universities include as essential requirements during application. Since the system can be adopted globally, this part is left for the students and university admins to populate.
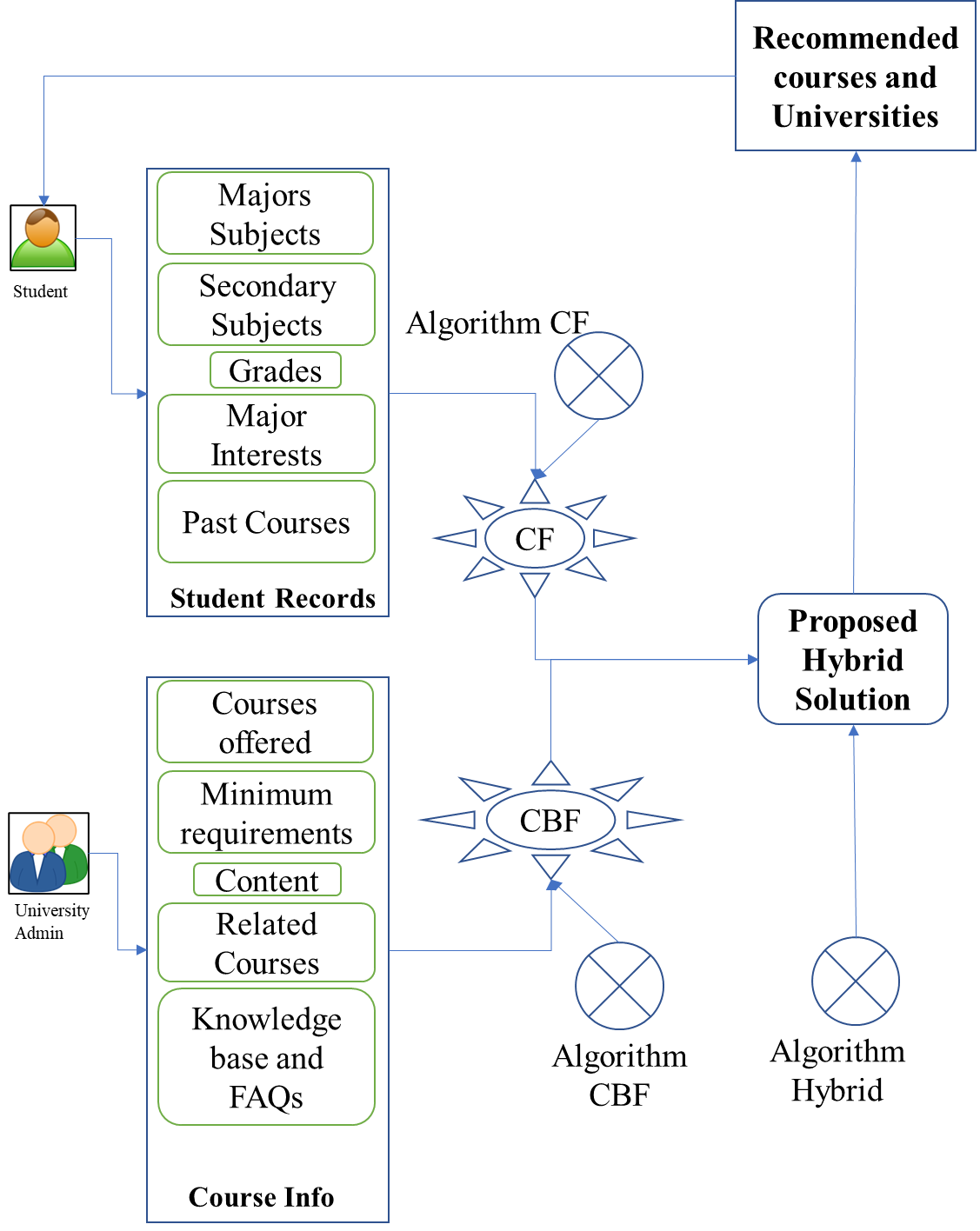
Secondary subjects are mostly the basis for the selection of courses. The system is implemented to understand subjects based on whether they are sciences, arts, crafts, languages, or other categories. When universities update their courses on their portals, they give the subjects associated with such courses. Consequently, when learners select their secondary subjects, the system compares them with the data provided by the universities. The customer then inputs the grades scored in each of the chosen subjects since these also form the basis for the points used to get into different programs offered in various universities.
The interests portion is an added feature that enables the customers to provide other areas that can help the recommendation algorithm suggest the best courses for the learner. For instance, in this case, a customer who indicates that they are interested in cooking will likely take a course in the hotel industry or become a nutritionist. This information is used in line with the additional knowledge provided by the universities. Finally, the past courses module is also an added part that aims to provide the system with more information on the customer so it can recommend a better course and university for the student. For example, a customer with a bachelor’s in business administration may look for information on a Master of Business Administration or another undergraduate business course.
Apart from the information about the customer, during the registration process, the customer is expected to provide some personal data, which is also crucial in the recommendation process. The system recommends courses and universities, making it essential to consider the distance between the university suggested and the customer. For instance, a student in Nigeria may wish to pursue a course in electrical engineering. The customer may achieve all the prerequisite courses in hundreds of universities worldwide. Even though the client meets all the entry requirements, the system must recommend the most appropriate university the student will select. Consequently, the system considers the distance between the customer’s location and the universities and suggests the courses from the nearest location to the furthest one. Moreover, the diagram does not show the chats module, though the system is made such that customers can chat directly with system administrators.
The University Admin Portal
The university admin portal is where most of the work occurs regarding populating the system. The institution’s admin is responsible for updating the details pertaining to their institutions from time to time, ensuring that such information is current. Due to its peculiarity, the system gives university administrators a wide range of functionalities, including managing staff and other information related to the institution. In Figure 10 above, the university admin has various inputs to the system, including the courses offered, minimum requirements for each course, the content of the courses, related courses, and frequently asked questions and knowledge areas. The system uses all these necessary items to index the courses and the university and later recommend the programs to the customers.
In the courses offered module, the administrator must enter all the courses provided irrespective of their schools, faculties, or departments. All the courses enumerated are then indexed such that the university admins can provide more information in subsequent sections. The system is designed not to dictate the order of entry but to present the entered courses in alphabetical order. This allows for work flexibility, including further updates to the university course items. The minimum requirements section allows admins to update information regarding the entry points required for each course. This is the point at which the subjects become critical and results in the definition of the support and confidence values used to calculate the values for the recommendation of a particular course to a qualifying customer. The updater indicates the points for both the core and secondary subjects and the overall score. The three sets of values entered in the minimum requirements are used to calculate the weighted average for each course.
The course contents module enables the admins to add critical information regarding what the course covers. This section is structured so the updater can choose between the modules framework and the years’ framework. Some courses are modules, and others are in year levels. Sometimes, this is an institution’s policy, and such choices are left black for the admins to decide. The admin can provide the units taken and the expected pass marks depending on the chosen framework. They can also suggest readings and resources the customers may need to complete the course or module.
The related courses section is an additional module that the admins can populate depending on their goals. Some universities offer several associated courses that the students may consider as alternatives to what they hope to find. This module is also included to help institutions increase their chances of being recommended to customers. Similarly, the frequently asked questions and knowledge areas module offers the administrators a way to add relevant information regarding selected courses and the university at large. This may act as a blog section where the university writes about all that can be relevant for prospective customers. They can also use the section to answer some of the questions that students have asked them in other fora or direct chats, thus increasing the knowledge available to the public regarding the institution.
Individual Recommender Systems
The current system uses a collaborative filtering technique to estimate related students’ interests and past courses. If a user adds any, the method uses the two similarity measures: interests and past courses. The similarity by interests is used to compute the closeness of the customer’s information with those provided by others with similar inputs. It is expected that students interested in the same lines are likely to take the same courses or related ones. Considering the two similarity measures, with student i and j, the following are determined:
- Similarity by major subjects Mij: This is the measure that calculates the major subjects that each student submitted. The system then computes the linear correlation using Pearson coefficients.
- Similarity by interests Iij: This is the measure that calculates the values for interests entered by students and determines the distance between them based on linear correlation and Pearson coefficients.
The similarity measure is critical and flexible because it is manipulated to fit the relevance levels required to fit each criterion, making it easier for the system to suggest courses using weights that give the most accurate results. It is also worth noting that the CF approach has not been used to determine the relationship between students’ major subjects, secondary subjects, and grades obtained. The three items are computed based on the university’s information regarding their course requirements. Therefore, the current system measures similarities between students and subject weights provided by the institutions and harmonizes the two sets of data to recommend courses and universities.
The CBF is used at the universities’ end to suggest courses to the customers based on the information obtained from the customers’ input and similarity measures. Therefore, the measures used by the university module include courses offered, minimum requirements for each course, and related courses. Specifically, the module compares the related courses of similar students using the customers’ grades and the weighted score to determine the courses to suggest to the learner. The system design makes the measures provided by the universities to be fixed based on the values filled by the administrators, Consequently, the algorithm does not alter such values to meet those provided by the customer. This policy ensures that only qualified students can apply for universities and courses whose weighted scores match those of the customers.
The Hybrid Recommender System
The proposed system combines the effects of CF and CBF to give more advanced recommendations to the customers. The hybrid recommender system adds another layer of course details and trends to find the best course and university for the student. It uses the interest measures and the keywords generated from all the information provided by universities to suggest a final preference p, which is closest to the student’s needs.
Impacts of the Implemented System
The recommended system will have benefits and demerits for both internal and external users. The system will result in reducing the time to get valuable information for several clients at the same time. The administrators and their universities will have access to insightful reports to derive opportunities to improve business operations and customer satisfaction. Moreover, customers can easily keep track of choice institutions, their courses, and the situations surrounding them. However, the system led to less communication between staff and customers, hence may not provide the needed feeling. Moreover, the proposed system may impact users’ data and privacy, though such concerns have been considered. Other risks associated with the system have been shared in section 3.9 under risk analysis and contingency plans.
Conclusion and Future work
This section summarizes the work done, reflects on the project, and suggests more work that can be done in the future.
Conclusion
The issue of course recommendations and university selection has been a major one over the years. While some businesses and institutions have implemented recommendation systems, most have not addressed customers’ critical needs. The proposed system was created with this understanding based on survey findings. The participants indicated that they would prefer getting course recommendations with added information. This finding reveals one of the major issues that has impacted the education sector: inclusive access to all the resources. Primarily, scholars have focused on students with disabilities, which has resulted in the assumption that those without physical disabilities are better placed to meet their academic needs. Thus, assistive technology has often been associated with those with physical disabilities.
The literature analysis reveals that assistive technologies do not only apply in the case of students with disabilities. It is a system needed by an individual doing a task within a given environment. Thus, the current project proposed an assistive technology to help students and other internet users seeking to apply for a course to get the most helpful recommendation that ensures they can have high chances of joining their chosen courses and universities. This project is created to understand the users’ needs and ensure that the required information is always the latest.
The current study and the resulting project were done with the following specific objectives:
- To implement a communication system for extracting student information requests via textual analysis, which will serve as a query for presenting insightful data relative to universities or colleges.
- To provide timely, concise, and clear information tailored to the needs of an individual seeking admission by providing answers to queries and guidance for each stage of the application process.
- To provide a step-by-step approach to deliver data to inform prospective students on their choice of institutions, career paths, and potential job opportunities
- To explore the most effective way of providing information tailored to an individual’s inquiry about university programs in the UK.
- To assess the impacts of the implemented software model on the decision of choice college by an individual.
- To explore the insights that could be derived from utilizing such an assistive technology for university institutions.
Each of the objectives above has been achieved in different phases of the study and the project. The project has proposed a solution that can extract student information from textual analysis and use its various tools to provide insights based on various educational institutions offering different courses. The proposed solution also ensures that the information presented to the customers is timely, concise, and clear and meets the needs of the individual seeking admission since the system provides the specific answers the students desire in each step of the application process. The students can easily follow and understand their actions based on the system’s prompts and suggestions. In addition, the proposed system uses deep algorithms that ensure each customer gets information that enables them to choose their courses and universities and inform them about the possible careers they can pursue and potential jobs they can seek. Moreover, the research through the proposed system has successfully implemented a way of providing information tailored toward an individual’s inquiry about various programs offered in different universities locally and globally. Furthermore, the impacts of the system have also been explored, and the adverse scenarios are effectively addressed through legal and ethical approaches.
Reflection
Completing the current project has been one complex task yet worth the effort. The design of the proposed system has given the developers a wide range of knowledge regarding the actual project management and development of industrial applications. The initial phases of the system design did not provide many insights regarding the entire visualization of the project. The more the team worked on the design, the better they managed to create a system that effectively competes against the existing ones and solves real problems faced by customers and universities. The project has borrowed several concepts from existing ones, including CF and CBF, which are used in businesses. However, the project team also appreciated the work done by other hybrid system manufacturers, which made it easier for them to explore current gaps and fill them with the most needed solution. The initial survey was crucial as it gave the developers an idea regarding the problems users face, thus making it easier for the team to visualize the final project that could solve the problems. Moreover, the project team also appreciates that more work should be done in the future, and this should make better and more advanced systems.
Future Work
The current research has met its primary aim of creating assistive technology for supporting student admission through the course and university discovery. However, the present research and the proposed solution do not provide the ultimate solution for course recommendations and university selections. Future systems designers need to work on more advanced algorithms that integrate with other systems, including social media, to give a better recommendation to the users as suggested by Ranganathan and Tzacheva (2019). Such systems can employ big data from companies such as Google, Facebook, Twitter, and others, thus making it easier to understand a user and suggest.
Reference List
Adams, B. and Wilson, N. S. (2020) ‘Building community in asynchronous online higher education courses through collaborative annotation’, Journal of Educational Technology Systems, 49(2), pp.250-261.
Amrutkar, S., Mahakal, S. and Naidu, A. (2021) Recommender Systems for University Elective Course Recommendation. In Applications of Advanced Computing in Systems (pp. 247-257). Springer, Singapore.
Batool, S. S. and Ghayas, S. (2020) ‘Process of career identity formation among adolescents: components and factors’, Heliyon, 6(9), p.e04905.
Darioshi, R. and Lahav, E. (2021) ‘The impact of technology on the human decision‐making process’, Human Behavior and Emerging Technologies, 3(3), pp.391-400.
Ferri, F., Grifoni, P. and Guzzo, T. (2020) ‘Online learning and emergency remote teaching: Opportunities and challenges in emergency situation’, Societies, 10(4), p.86.
Ghafari, S.M. and Tjortjis, C. (2019) ‘A survey on association rules mining using heuristics’, Wiley Interdisciplinary Reviews: Data Mining and Knowledge Discovery, 9(4), p.e1307.
Javed, U., Shaukat, K., Hameed, I.A., Iqbal, F., Alam, T.M. and Luo, S. (2021) ‘A review of content-based and context-based recommendation systems’, International Journal of Emerging Technologies in Learning (iJET), 16(3), pp.274-306.
Kalra, M., Lal, N. and Qamar, S. (2018) K-mean clustering algorithm approach for data mining of heterogeneous data. In Information and Communication Technology for Sustainable Development (pp. 61-70). Springer, Singapore.
Kulkarni, P. V., Rai, S. and Kale, R. (2020) Recommender system in elearning: a survey. In Proceeding of International Conference on Computational Science and Applications (pp. 119-126). Springer, Singapore.
Lent, R. W. and Brown, S. D. (2020) ‘Career decision making, fast and slow: Toward an integrative model of intervention for sustainable career choice’, Journal of Vocational Behavior, 120, p.103448.
Liu, Y. (2018) ‘Data mining of university library management based on improved collaborative filtering association rules algorithm’, Wireless Personal Communications, 102(4), pp.3781-3790.
Lops, P., Jannach, D., Musto, C., Bogers, T. and Koolen, M. (2019) ‘Trends in content-based recommendation’, User Modeling and User-Adapted Interaction, 29(2), pp.239-249.
Lu, J. and Holubová, I. (2019) ‘Multi-model databases: a new journey to handle the variety of data’, ACM Computing Surveys (CSUR), 52(3), pp.1-38.
Malik, S., Rana, A. and Bansal, M. (2020) ‘A survey of recommendation systems’, Information Resources Management Journal (IRMJ), 33(4), pp.53-73.
Neysiani, B. S., Soltani, N., Mofidi, R. and Nadimi-Shahraki, M. H. (2019) ‘Improve performance of association rule-based collaborative filtering recommendation systems using genetic algorithm. International Journal of Information Technology and Computer Science, 11(2), pp.48-55.
Ranganathan, J. and Tzacheva, A. (2019) ‘Emotion mining in social media data’, Procedia Computer Science, 159, pp.58-66.
Rao, T. R., Mitra, P., Bhatt, R. and Goswami, A. (2019) ‘The big data system, components, tools, and technologies: a survey’, Knowledge and Information Systems, 60(3), pp.1165-1245.
Ray, S. and Saeed, M. (2018) Applications of educational data mining and learning analytics tools in handling big data in higher education. In Applications of big data analytics (pp. 135-160). Springer, Cham.
Rivera, A. C., Tapia-Leon, M. and Lujan-Mora, S. (2018) Recommendation systems in education: a systematic mapping study. In International Conference on Information Technology & Systems (pp. 937-947). Springer, Cham.
Sabri Ahmad, I. A. and Man, M. (2018) Multiple types of semi-structured data extraction using wrapper for extraction of image using DOM (WEID). In Regional Conference on Science, Technology and Social Sciences (RCSTSS 2016) (pp. 67-76). Springer, Singapore.
Schwaber, K., 2004. Agile project management with Scrum. Microsoft press.
Sharma, R., Rani, S. and Tanwar, S. (2019) Machine Learning Algorithms for building Recommender Systems. In 2019 International Conference on Intelligent Computing and Control Systems (ICCS) (pp. 785-790). IEEE.
Spoth, W., Xie, T., Kennedy, O., Yang, Y., Hammerschmidt, B., Liu, Z.H. and Gawlick, D. (2018) SchemaDrill: Interactive semi-structured schema design. In Proceedings of the Workshop on Human-In-the-Loop Data Analytics (pp. 1-7).
Sulova, S. (2018) ‘Association rule mining for improvement of IT project management’, TEM Journal, 7(4), pp.717-722.
Tam, C., da Costa Moura, E.J., Oliveira, T. and Varajão, J. (2020) ‘The factors influencing the success of on-going agile software development projects’, International Journal of Project Management, 38(3), pp.165-176.
Thannimalai, V. and Zhang, L. (2021) December. A Content Based and Collaborative Filtering Recommender System. In 2021 International Conference on Machine Learning and Cybernetics (ICMLC) (pp. 1-7). IEEE.
Wu, Z., Song, T. and Zhang, Y. (2022) ‘Quantum k-means algorithm based on Manhattan distance’, Quantum Information Processing, 21(1), pp.1-10.
Yang, X., Zhou, S. and Cao, M. (2020) ‘An approach to alleviate the sparsity problem of hybrid collaborative filtering-based recommendations: the product-attribute perspective from user reviews’, Mobile Networks and Applications, 25(2), pp.376-390.
Zhu, Y., Lin, J., He, S., Wang, B., Guan, Z., Liu, H. and Cai, D. (2019) ‘Addressing the item cold-start problem by attribute-driven active learning’, IEEE Transactions on Knowledge and Data Engineering, 32(4), pp.631-644.
Zilz, W. and Pang, Y. (2021) ‘Application of assistive technology in inclusive classrooms’, Disability and Rehabilitation: Assistive Technology, 16(7), pp.684-686.
Zriaa, R. and Amali, S. (2020) A comparative study between k-nearest neighbors and k-means clustering techniques of collaborative filtering in e-learning environment. In The Proceedings of the Third International Conference on Smart City Applications (pp. 268-282). Springer, Cham.