Introduction
This study will explain how Artificial Intelligence (AI) and Machine Learning (ML) could improve clinical trials in the pharmaceutical industry. The contents of this paper will give details on how the two technologies could help advance the efficacy of clinical trials using data and statistics generated from companies that have adopted them. At the same time, to draw contrasts on the application of AI and ML in the health sector, the limitations of the technologies will also be elucidated to highlight areas of improvement that could be explored for future improvements and integration in clinical practice. To understand these areas of research in detail, in this analysis, emphasis will be made to highlight the role of the technology in addressing poignant challenges associated with clinical trials, such as the recruitment and selection of participants. This area of the investigation will encompass most of the analysis included in the present text but the role of AI in optimizing dosing regimens and improving the design of effective interventions will also be explored as supplementary analyses. Before embarking on these areas of analysis, it is important to understand some of the most significant challenges clinicians experience when completing their trials.
Cost and Time Associated with Clinical Trials
Recruiting patients for clinical trials is marred by challenges relating to the incompletion of tests and rising costs of sustaining volunteers throughout the investigation. This is why pharmaceutical companies pay a lot of money in research and development before they develop and present new drugs to the market (11). The costs associated with developing such drugs could stretch into millions or even billions of dollars, depending on the design and site selection protocol of adhering to clinical research guidelines (10). These findings mean that pharmaceutical companies have to invest many resources in minimizing the time taken to conduct clinical research and present safe drugs to the market. Part of the process involves making sure that a participant who is willing to engage in a clinical research trial is committed to staying in a program for its full length. However, clinicians do not always achieve this objective because of the high failure rates associated with past assessments and the costs of keeping the participants engaged throughout different stages or phases of clinical research (11). Figure 1 below shows the average cost of maintaining a volunteer, across different phases of a typical clinical research trial.
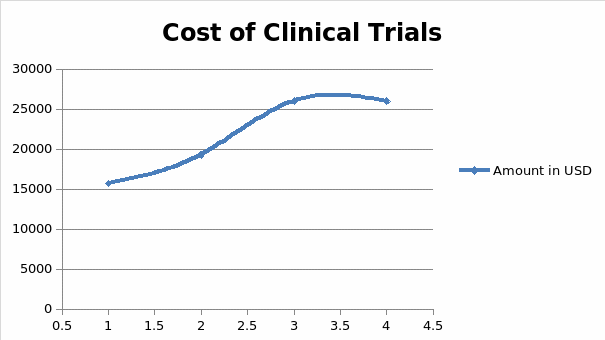
According to figure 1 highlighted above, the cost of maintaining the participation of research participants rises across four phases of clinical trial development. On average, the first phase of clinical research could cost pharmaceutical companies up to $15,700 to maintain one participant in the first phase of drug development. In the second stage, this cost could rise further to $19,300, after which it later increases to $26,000 in the third and final stages of the clinical trial. For most drug developers these costs are often prohibitive and inhibit their ability to develop reliable drugs and present them to the market affordably (11). This is why the cost of some drugs is often high and unreachable to patients who need them the most (10). High rates of incomplete clinical trials, reported in some cases, further compound the problem. Figure 2 below shows that researchers fail to complete about 80% of clinical trials fail on time, while another 20% are delayed for more than six months due to reasons attributed to recruitment and retention of patients in these investigations (11).
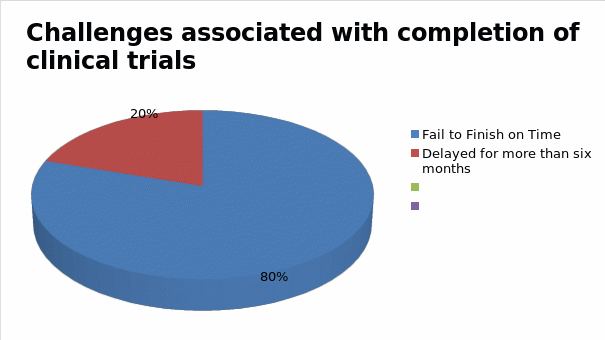
The findings highlighted above show that many pharmaceutical companies are experiencing challenges maintaining a healthy balance between the overall cost of conducting clinical research and the time it takes to complete them. This problem has affected clinicians across different areas of research (10). Consequently, there is a need to find better ways of managing these variables. AI and ML provide opportunities for doing so as highlighted below.
Bolstering Patient Recruitment Efforts
As mentioned above, recruitment is one of the most significant barriers for researchers to undertake successful clinical trials. In one study authored by Woo (15), this challenge manifested in identifying patients who suffered early-stage breast cancer. It was reported that out of the possible 40,000 women in the US who suffered this type of cancer, researchers only managed to recruit 636 patients in five years (15). It was proposed that AI and ML were useful in increasing the number of participants and the duration of identifying and accessing them. Particularly, AI was highlighted as having the power to help researchers to achieve this objective using different types of technologies associated with it (1). For example, Natural Language Processing (NLP) – a technique that identifies written and spoken words to find patients who have been diagnosed with specific conditions – was mentioned as having the ability to identify researchers who could take part in such an investigation within a short time. For example, it could be used to search doctors’ notes to find cases involving patients with a specific type of disorder. By doing so, clinical trials could be better focused to investigate the efficacy of drugs that are intended for a specific subpopulation.
In line with the above recommendations, A California-based company, known as Cedars-Sinai Smidt Heart Institute, used AI analytics to identify 16 participants suitable for a clinical trial in one hour (15). The use of traditional methods of recruitment could have taken months to achieve the same outcome. This example shows that AI is effective in recruiting relevant participants for a clinical trial within a short time. It does so without compromising the integrity of the research or its participants (5). Mayo Clinic, which is located in Rochester, Minnesota, has also reported similar impressive results in recruiting volunteers for clinical research because they reported an 80% increase in recruitment for participants wishing to take part in breast cancer clinical trials (15). By most standards of measurement, an 80% increase is significant enough to pay attention to the strength of AI in improving the recruitment and retention of research participants.
Cohort Enrichment
Another way that AI and ML may help to improve patient recruitment levels is via cohort enrichment. This could happen when the two technologies help to identify a subset of the population that a clinical trial outcome is best applicable. Broadly, the action means that the technologies are not designed to highlight the effectiveness of treatment options across a population of randomized control trials (1). Instead, they can help to improve the efficacy of trial outcomes by identifying patients that are not suitable for a specific investigation because their involvement would undermine the effectiveness of the trial outcomes (6). Relative to this observation, Woo (15) cautions that, even though AI may help to increase the number of participants that would be involved in a clinical trial, the surge does not guarantee an increased likelihood of success. However, the inclusion of unsuitable candidates in a clinical trial is almost definitely likely to minimize the success of such outcomes (3). AI and ML technologies help to minimize this risk by identifying persons who would undermine the efficacy of the clinical trials and by doing so, help to enrich the outcomes.
In an ideal clinical trial setting, the recruitment of patients should be done using genome patient-specific diagnosis tools where biomarkers that are targeted by a drug are present in a patient that should be the ideal recipient of a drug under development (15). Trials that follow this methodology exist but they are few in percentage compared to the overall number of investigations that are reported in research (2). At the same time, they are more expensive compared to conventional trials, particularly when medical imaging techniques are deployed to identify the right group of participants for an investigation (10). AI and ML help to bridge this gap in the research by identifying patients with unique biomarkers that would be relevant to a specific trial (4). For example, sophisticated AI and ML techniques that are under development have the potential of merging specific Omic data with electronic medical records (EMR) to identify patients or clinical trial participants with specific types of data that would be relevant to a clinical trial (17). This process helps to identify endpoints in clinical research trials that can be sufficiently measured for improved efficacy and outcomes. They improve a researcher’s ability to identify and characterize specific subpopulations of patients that are suitable for specific trials through AI and ML-based techniques, such as NLP and Optical Character Recognition (OCR) methods (15). The application of these techniques in clinical research trials means that the process of reading and compiling pieces of evidence relating to clinical trials will be fully automated using the technologies.
AI and ML techniques also have the potential of harmonizing EML data because they are commonly scattered and available in different formats owing to their large volumes and velocity (5). The data source-agnostic nature of AI data helps to overcome these barriers, thereby leading to the development of harmonized EMR datasets for comprehensive analysis (8). This process of analysis is important in designing tools for clinical trial enrichment and the discovery of patients with unique biomarkers for specific clinical research. Other benefits associated with AI and ML use that are realizable using this technique include pre-clinical compound discovery and improved techniques for highlighting compounds associated with clinical trial testing (4). Prediction-based AI and ML tools could also help to achieve these outcomes by identifying correlations among patients, biomarkers, and clinical outcome indications. This process has the potential of identifying candidates that have a higher likelihood of success in the implementation of clinical trials as well as those who are unsuitable for a trial before they take part in it (3). In this regard, AI and ML help to improve the selection of patient cohorts for clinical research, thereby enriching cohorts of patients willing to volunteer in an investigation.
Improving Clinical Trial Design
The design of clinical trials is important in understanding the flow of information in a given investigation that would ultimately lead to the collection of reliable data and the development of quality findings. Indeed, as highlighted by Harrer, Shah, and Antony (3), clinical trials often contain protocols that stipulate processes and procedures that researchers should follow when designing clinical trials. Given that most of them rely on a variety of sources to develop their findings, AI and ML could help to analyze such data faster and more efficiently than a human being does, thereby providing a stronger foundation for developing more reliable clinical trial designs (7). For example, the technologies could scan information from relevant clinical journals, drug labels, and information emerging from private pharmaceutical companies, faster and more effectively, thereby making it easier to calibrate information that would be better suited for a treatment design.
By using such information, it is easier to understand how different aspects of a proposed trial could influence the cost and eligibility requirements for a specific case or analysis (16). Broadly, the above findings are important in understanding specific aspects of a clinical research design that affect key success factors of a clinical trial. In this regard, AI and ML emerge as data-driven guides for developing better clinical trial designs compared to conventional means. Therefore, they are useful in improving the clinical trial design, thereby enabling researchers to make improvements in specific areas of research. At the same time, the use of AI and ML in improving protocol design makes it is possible to develop drugs faster and at a cheaper cost than conventional methods do.
Optimizing Dosing Regimens
Clinical trials aimed at improving the efficacy of drugs could also use AI to optimize dosing regimens. This may happen through improved efficacy in assessing issues relating to treatment and drug administration (9). For example, AI and ML have been used to understand the efficacy of combining different types of drugs by tinkering with existing schedules and developing a larger body of literature that estimates their efficacy and safety (13). By optimizing dosing requirements, AI and ML also help to minimize the risk of patients suffering from adverse events relating to clinical trials (10). At the same time, they could help to minimize the incidence of trial delays, which are commonly associated with insufficient dosage requirements (17). Indeed, by analyzing data relating to how different groups of patients respond to specific treatment plans, specific dosages could be better tailored to suit unambiguous demographics to minimize their side effects and improve their overall efficacy.
The role of AI and ML in optimizing dosing regimens has been demonstrated by companies that have used the technique to find the right treatment plans for patients with cancer (15). For example, Zenith Epigenetics used these technologies to find the correct treatment plan for a patient suffering from Prostate cancer. Notably, the company used AI algorithms to find the right dosage for the drug ZEN-3694, which when combined with Enzalutamide, could treat the same condition (15). The AI algorithm used was known as CURATE and the efficacy of the treatment plan was assessed by reviewing the patient’s clinical data and comparing it with tumor size before and after treatment (15). The findings were more vivid using the two technological tools. Cancer biomarkers in the blood were also assessed on the same platform to understand the efficacy of the treatment plan and the results were used to find the best treatment regimen for the patient (15).
Overall, this example shows how AI and ML could be used to find individualized treatment options for patients suffering from different types of conditions. Their importance is magnified when understanding the best combination of drugs that patients could take to treat a specific condition. Therefore, their use is important in improving the efficacy of existing and new treatment plans, thereby improving the administration of drugs. Doing so will be a departure from traditional eyeballing techniques that are commonly used by physicians worldwide to improve treatment regimens (15). Despite their commendable track record in enhancing this area of clinical research, some challenges have been associated with the adoption of AI and ML.
Limitations of AI and ML
Implementing AI in clinical settings is one of the foremost challenges associated with its adoption. However, given the unstructured nature of doctor’s notes, it may be prudent to have background information about specific cases associated with patients who manifest specific symptoms or have been diagnosed with specific conditions (12). For example, specialists may describe one condition differently or in multiple ways. For example, some doctors may describe a heart attack as a myocardial infarct, or a myocardial infarction, depending on their training or institutional environment (7). In some cases, the same condition may be described as MI (15). Such discrepancies may limit the ability of ML techniques to generate accurate data to support clinical trials. However, it is possible to address such concerns through improved feedback loops where machine learning is deployed to train AI to detect and correct the effects of such variations on clinical outcomes (9). This possibility leaves room for further application of AI in clinical trials.
Additionally, although machine learning has been proposed as one of the most significant ways of improving AI efficiency, it still requires significant investments in data generation (13). This is a challenge for most data analysts and researchers because they need many hours to manually annotate data that would be used in tests (14). Variations in the management of data across different medical fields and data management processes across medical institutions are still too significant to ignore because they could cause discrepancies in data usage, which may affect clinical trial outcomes (2). In this regard, there is no universal understanding of clinical trials data.
Another challenge associated with the use of AI involves the use of third-party tools to access patient data. Most developers engage third parties to extract and manage data to improve research outcomes (12). This strategy could cause ethical violations stemming from a breach of confidentiality agreements between patients and their medical service providers (12). Particularly, the involvement of third-party actors in data mining poses the biggest challenge in this regard because they are foreign players in the management of doctor-patient relationships. Therefore, there is a need to be cognizant of the effect of including other players in the use of AI and ML in clinical trials especially because different countries and institutions have varied interpretations of this concern.
Conclusion
The findings of this investigation show that AI and ML are useful tools for recruiting the right participants for clinical trials and maintaining their participation throughout their lifecycles. They also help to reduce the cost and time taken to complete such trials because they are more effective and inexpensive to implement compared to traditional methods. However, it is important to be mindful of their limitations because they could cause ethical violations and misinterpretations in data analysis, depending on the institutional policies and environments of various healthcare facilities.
References
Bhatt A. Artificial intelligence in managing clinical trial design and conduct: man and machine still on the learning curve? Perspectives in Clinical Research. 2021; 12(1): 1–3.
Blease C, Locher C, Leon-Carlyle M, Doraiswamy M. Artificial intelligence and the future of psychiatry: qualitative findings from a global physician survey. Digital Health. 2020; (6)1: 234-244.
Harrer S, Shah P, Antony B, Hu J. Artificial intelligence for clinical trial design. Trends in Pharmacological Sciences. 2019 ; 40(8): 577-591.
Fonseka TM, Bhat V, Kennedy SH. The utility of artificial intelligence in suicide risk prediction and the management of suicidal behaviors. Australian and New Zealand. Journal of Psychiatry. 2019; 53(10): 954-964.
Kerr D, Klonoff DC. Digital diabetes data and artificial intelligence: a time for humility, not hubris. Journal of Diabetes Science and Technology. 2019; 13(1):123-127.
Lai KHA, Ma SK. Sensitivity and specificity of artificial intelligence with Microsoft Azure in detecting pneumothorax in the emergency department: a pilot study. Hong Kong Journal of Emergency Medicine. 2020; 17(2): 151-162.
Mathur P, Srivastava S, Xu X, Mehta JL. Artificial intelligence, machine learning, and cardiovascular disease. Clinical Medicine Insights. 2020; 14(1): 112-119.
Rahman MM, Khatun F, Uzzaman A, Sami SI, Bhuiyan MA, Kiong TS. A comprehensive study of artificial intelligence and machine learning approaches in confronting the Coronavirus (COVID-19) pandemic. International Journal of Health Services. 2021; 51(4): 446–461.
Randhawa GK, Jackson M. The role of artificial intelligence in learning and professional development for healthcare professionals. Healthcare Management Forum. 2020; 33(1): 19-24.
Ranschaert ER, Morozov S, Algra PR. Artificial intelligence in medical imaging: opportunities, applications, and risks. Springer; 2019.
Roth C. The real cost of clinical trials [Internet]. Buffalo, NY: Praxis. 2017.
Samuel G, Chubb J, Derrick G. Boundaries between research ethics and ethical research use in artificial intelligence health research. Journal of Empirical Research on Human Research Ethics. 2021; 16(3): 325-337.
Shinners L, Aggar C, Grace S, Smith S. Exploring healthcare professionals’ understanding and experiences of artificial intelligence technology used in the delivery of healthcare: an integrative review. Health Informatics Journal. 2020; 26(2): 1225-1236.
Wang C, Zhu X, Hong JC, Zheng D. Artificial intelligence in radiotherapy treatment planning: present and future. Technology in Cancer Research and Treatment. 2019; 18(1): 651-667.
Woo M. Trial by artificial intelligence: a combination of big data and machine-learning algorithms could help to accelerate clinical testing. Nature. 2019; 573(26): 100-102.
Wood EA, Ange BL, Miller DD. Are we ready to integrate artificial intelligence literacy into the medical school curriculum: students and faculty survey. Journal of Medical Education and Curricular Development. 2021; 8(1): 424-447.
Zahren C, Harvey S, Weekes L, Bradshaw C, Butala R, Andrews, J. Clinical trials site recruitment optimization: guidance from clinical trials: impact and quality. Clinical Trials. 2021; 18(5): 594-605.