Abstract
This study focuses on investigating the effects of AI on the accounting profession and providing possible solutions to its negative effects from the prisms of education and training. Four research questions guided the review. They aimed to find out how AI affects the performance of accounting professionals, investigate whether there have there been changes in employee attitudes toward AI, explore factors that could influence changes in the attitudes of accounting professionals towards AI and understand the attitudes of accounting professionals towards AI. Data was collected using interviews. The respondents were teachers and accounting professionals.
Their views suggested that there was a low level of awareness about AI in the accounting profession. They also provided a mixed review about the effects of Ai on the accounting sector. However, it was established that low-levels accounting jobs are most vulnerable to job losses.
Introduction
Background
Many companies around the world are redesigning their business processes to maximise their operational efficiencies (Kirschner & Stoyanov 2018). The accounting industry is one of the key tenets of corporate operations that are experiencing such changes (Cortese & Walton 2018). Their effects are heightened by the introduction of artificial intelligence (AI), which has been linked to high levels of productivity, accuracy and low operating costs (Fox 2018). Fox (2018) defines AI as the ability to help machines think, almost as a human being does. This type of technology equips machines or computers to make predictions about operational issues and adjust them to boost performance (Kirschner & Stoyanov 2018). Similarly, they can adapt to their environments the same way as human biology equips people to do the same (Carriço, 2018).
Professions that require the meticulous implementation of tasks are deemed the biggest beneficiaries of AI because it adds another layer of efficiency to their tasks (Birtchnell 2018). This outcome often occurs by extending a computer’s normal input and output processes to increase productivity (Felzmann, Villaronga, Lutz & Tamò-Larrieux 2019). Based on a general review of industry reports, AI is increasingly being used to conduct administrative duties and present accounting results in business through various structural changes (Fox 2018). Some common accounting tasks linked with AI include quarterly close procedures, procurement planning, developing accounts payables, answering audit queries and expense management (Ferri, Lusiani & Pareschi 2018).
Kirschner and Stoyanov (2018) support the above assertion by saying that most bookkeeping tasks, which are traditionally associated with accounting, are already being partially done through AI. For example, the technology is already being used to compute accounts payables and receivables (Geiger 2017). Relative to this discussion, observers have reported that some accounting firms have already integrated AI tasks to initiate payments and match purchase orders (Kirschner & Stoyanov 2018).
Some data entry procedures are also being undertaken using AI (Geiger 2017). The same outcome is true for data categorisation processes. For example, some global accounting firms, such as Deloitte, have adopted an advanced usage of AI to analyse the language used in contracts when reviewing client agreements (Birtchnell 2018). They have also relied on the same processes to analyse financial trends (Geiger 2017).
The healthcare field has also started using AI to improve industry outcomes and enhance efficiency (Pakdemirli 2019a; Liu, Keane & Denniston 2018). For example, some facilities use it to analyse healthcare claims for thousands of patients (Pakdemirli 2019b). In this regard, potential complexities have been observed during the initial stages of claim processing (Pakdemirli 2019b). The accounting sector has also used AI in the healthcare field by subjecting transaction approval processes, which are ordinarily done by human beings, to linked processes (Cortese & Walton 2018). Consequently, payroll, auditing and tax remittance procedures are increasingly being undertaken by AI-aided systems (Birtchnell 2018).
A broad review of these processes and their importance to accounting tasks shows that AI has the potential to oversee the implementation of complicated tasks, which have been traditionally a preserve of human intelligence (Bechmann & Bowker 2019; Mann 2017).
The trend towards the adoption of AI in accounting partly stems from increased work pressures that have forced most professionals to look for alternative resources for completing their project tasks (Rana 2018). Consequently, artificial intelligence (AI) has emerged as a technique that works much as human resources do in completing organisational tasks (Ferri et al. 2018). The only difference it has with other methods is that it is much more efficient and effective in completing accounting processes (Carriço 2018). It has also gained popularity among most organisations because it could help managers to complete tasks quicker than when they use conventional ways and without asking for a salary at the end of the process (Carriço 2018). This is why some managers prefer it to human labour (Rana 2018).
Stano, Kuruma and Damiano (2018) say that artificial intelligence significantly escalates the level of computing in the accounting field. Notably, it helps professionals to make appropriate changes in their decision-making processes that would be beneficial to the realisation of their professional or industry goals. In this regard, computers can adjust systemic processes through machine-based learning, as would be the case if human beings did the same job (Rana 2018).
The accounting profession could significantly benefit from this capability because it is characterised by the fulfilment of role tasks and the improvement of human capabilities (Rana 2018; Carriço 2018). Firms or companies that have developed a strong competitive advantage in the last decade based on this competence could attribute their success to the AI development platform.
The MIT-Boston Consulting Group is one organisation that supports the above-mentioned assertion because it recently reported that about 80% of professionals believe that AI contributes to the development of competitive advantages in the accounting field (Rana 2018). The same report suggested that about 70% of people associate improved productivity in organisational processes to technological development (Rana 2018). This idea has encouraged many accountants to increasingly relying on AI to assess large volumes of data – a process that was previously time-consuming when done by human beings (Stano et al. 2018).
Small and medium-sized organisations do not have the same resources that large organisations do to employ AI or develop associated products for their internal processes (Carriço 2018). However, Dahbi, Ezzine and Moussami (2017) say that AI will become more readily available and affordable to different firms in the future. Already, industry observers have demonstrated the positive impact of this technology on the marketing sector and predict that the accounting field would not be spared either (Carriço 2018). Indeed, AI technology has created significant cost reductions and improved productivity in multiple business sectors (Kirschner & Stoyanov 2018).
The same benefits are likely to be realised in the accounting field as well. This advantage is in addition to the gains made through improved accuracy and precision in the development of new products and the provision of quality services (Kirschner & Stoyanov 2018).
Although AI is set to revolutionise how industry processes are undertaken, generating all this information without the involvement of an accountant would be unwise because there is a place for human intervention in accounting processes, especially when making meaningful conclusions from the data generated (Dahlin 2019). In other words, even though AI could lead to job losses, there is a place for accountants in the analysis of data. However, their professional roles need to change from the traditional model, which is akin to the use of a calculator to make sense of data to a consultancy and advisory role that gives direction on what to do about the pieces of information obtained (Dahbi et al. 2017). This way, they would be advancing the profession and at the same time helping their clients to grow.
Artificial Intelligence poses several advantages and disadvantages to the accounting field. However, most professionals in this sector are ill-equipped to manage them (Cortese & Walton 2018). The need for training is consequently emphasised to equip them to not only respond well to this trend but also understand its potential impact on their careers (Rana 2018). For example, Levine (2019) posits that artificial intelligence could change a company’s structure, while Oleinik (2019) adds that it may have a significant impact on organisational culture as well.
Therefore, managers have to make changes to embrace some of these developments, as they need not oppose the trend but exploit it to realise their organisations’ objectives. This dissertation focuses on investigating the effects of AI on the accounting profession and providing possible solutions to its negative effects from the prisms of education and training. The purpose of the study is explained below.
Problem Statement
Although artificial intelligence is largely regarded as the frontier technology tool for the next generation of business progress, there is little understanding of its impact on different fields of business and accounting (Clarke, Chambers & Barry 2017). In this regard, many uncertainties are associated with artificial intelligence because, through a poor understanding of the concept, myths and misconceptions about its effects are widespread (Cortese & Walton 2018). For example, questions linger regarding the probability of artificial intelligence to solve all problems humans can address (Clarke et al. 2017). In these debates, issues relating to the limits of artificial intelligence are often discussed because the discussions revolve around understanding whether human and artificial intelligence are equal, or not (Cortese & Walton 2018).
Doubts also exist concerning the potential of artificial intelligence to replace human skills (Frude & Jandrić 2015). These issues stem from fears that some jobs or positions in the workplace would become obsolete because of the increased adoption of technology in the workplace (Clarke et al. 2017). The potential implications of such an outcome on employees and their families is even more commonly discussed because it is unclear how people, who could potentially lose their jobs through AI, will cater to the financial needs of their families when they lose their main sources of income (Clarke et al. 2017). This issue has affected professionals from different fields, including accounting. Indeed, from this concern, some companies have experienced opposition from some stakeholders or worker unions about the adoption of AI (Cortese & Walton 2018).
A deeper focus on the effects of AI on employee perception further highlights performance challenges that may occur when implementing AI. In other words, there are concerns that AI could influence employee performance by affecting their motivations to carry out simple tasks at work (Frude & Jandrić 2015). For example, they could sabotage work processes when they believe that AI is replacing them. Similarly, they would not create synergy between machines and human capital when they do not understand the roles of the machines in the workplace in the first place.
The adoption of AI in the accounting field has also been clouded by concerns from some quarters, about the potential for criminals to commandeer the technology and engage in unethical or immoral practices (Frude & Jandrić 2015). This issue stems from concerns about the potential to centrally controlled AI machines without human detection. The bigger question is whether it could be deduced that machines can behave ethically (as human beings do) or whether ethics should be disregarded in totality when using artificial intelligence. Frude and Jandrić (2015) delve further into this argument by questioning whether a machine can have a “mind” that is similar to a human being.
They also ask whether it could be conscious of the challenges or opportunities that exist in the real-world professional environment (Frude & Jandrić 2015). Questions regarding whether a machine can be treated with the same rights accorded to a human being also arise in such debates and it is still unclear how they can be solved in a world where people are still demanding for their rights in different social and political fields.
Purpose of the Study
There has been a lot of scepticism about AI in different fields of business management (Frude & Jandrić 2015). The same reservations have been noted in the accounting field because many professionals are still unclear about its effects on the practice and its relevance to the field (Dahlin 2019). Consequently, some researchers have developed negative attitudes towards it (Dahlin 2019). Nonetheless, the rate of development in artificial intelligence is increasing and it is worrying many professionals who believe that it would replace their jobs or make them irrelevant to the field (Dahlin 2019).
Although the development and integration of AI in the accounting field are still in their infancy stages, there is a need to properly understand the challenge and benefits of artificial intelligence in the accounting field, especially from an education and training point of view. The focus on training and education is pursued in this study because the integration of human and artificial intelligence depends on the effective understanding of AI through similar processes (Frude & Jandrić 2015). This need is supported by the fact that many accounting professionals are unfamiliar with AI and unaware of how it would impact their practice (Dahlin 2019).
Regardless of their lack of misinformation, the human element of accounting cannot be ignored even in the wake of increased developments in the AI field. Therefore, while developments in AI continue to improve through rapid technological changes, human intelligence needs also be improved through education and training. This is why this paper adopts a similar focus in understanding the challenges and benefits of artificial intelligence in the accounting field.
Aim
To investigate the effects of AI on the accounting profession and providing possible solutions to its negative effects from the prisms of education and training
Research Questions
- How does AI affect the performance of accounting professionals?
- Have there been any changes in employee attitudes toward AI in the past five years?
- What factors could influence changes in the attitudes of accounting professionals towards AI?
- How can the attitudes of accounting professionals towards AI be improved?
Overview of the Research
This study will be a qualitative investigation aimed at understanding the challenges and benefits of AI in the accounting field. The implications of the technology on the discipline will be based on its implications on education and training. The phenomenology research design will form the research design for the review because AI is considered a phenomenon in the business world (Fox 2018).
The research informants will be professionals who worked in accounting firms that have used AI. They were based in China and contacted by the researcher using the WeChat platform – a popular social media tool in China. Data will be analysed using the thematic and coding method, which is generally associated with the review of qualitative data. However, before delving into these discussions, it is important to conduct a review of past findings. The literature review section outlines the findings.
Literature Review
Introduction
This chapter provides an overview of what scholars have written about the impact of AI on accounting and businesses. Key sections of the chapter explain attitudes towards AI, its advantages, applications across different industries and the connection it shares with the accounting field. At the end of the chapter, the research gap, which necessitates this study, will be demonstrated. However, before delving into these details, it is essential to understand the theoretical underpinning of AI.
Theoretical Review
It is important to conduct a theoretical review of AI to understand the concepts and models that have underpinned its application. A broad review of current literature suggests that there is no specific of group of theories that directly explain AI integration (Ferri et al. 2018; Rana 2018; Carriço 2018). This gap in literature could be explained by the dynamism of the concept and its involvement with different aspects of business intelligence, including adaptive systems, intelligent distribution systems, data mining and knowledge discovery among other factors (Rana 2018; Carriço 2018).
The absence of theories explaining Ai integration has created a vacuum in analysis, which has been filled by the emergence of various mathematical models of application (Ferri et al. 2018). These models include numerical methods of learning, data assimilation techniques and language theories for analytical development, such as linear and logistic regression (Rana 2018; Carriço 2018). Collectively, they create value for organisations and industries. Although there is scanty evidence explaining AI integration, select studies show that two types of models are applicable in understanding the phenomenon: descriptive and normative (Frude & Jandrić 2015; Carriço 2018).
Descriptive models are often developed by observing the natural environment. The associated data is later used to make predictions about the future or guide people’s behaviours. Comparatively, normative models rely on the formulation of specific assumptions and theories to explain technological integration (Frude & Jandrić 2015; Carriço 2018). Therefore, accepting a model’s assumptions implies that their associated conclusions should also be tolerated. AI integration often assumes a dichotomous nature because it is both descriptive and normative. The models used to implement it are often designed to understand commonalities between how computers and the human brain work (Ferri et al. 2018). The basic premise of model development is that computers can effectively undertake the functions of employees but certain aspects of human function remain difficult to replicate.
The purpose of an AI model is to bridge the distance between human and machine capabilities. Based on this understanding, Kirschner and Stoyanov (2018) say that many AI models seek to identify similarities and differences between human and machine functions. One of the differences and similarities observed so far is that human intelligence occurs naturally but AI has to be built over a long period. This observation means that AI theories should be descriptive in their characterisation of human intelligence and, at the same time, be normative in their review of artificial intelligence (Frude & Jandrić 2015; Carriço 2018).
Stated differently, such theories should explain how the human mind works and outline how systems may be developed to replicate the same type of intelligence. Intelligence is the common concept underlying most of these models. Its application has been linked to several advantages associated with AI.
Advantages of AI
Several researchers have examined the potential benefits of AI to business processes (Fuatx 2019). Broadly, their findings explain how the technology influences specific aspects of business operations. According to figure 1 below, the main benefit of AI to companies is the enhancement of features and performance of products and services.
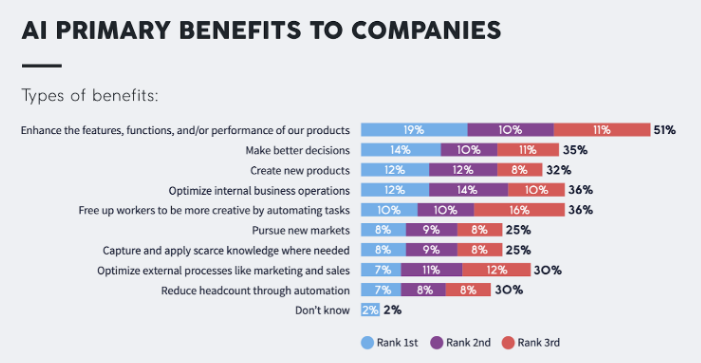
Multiple research studies have also shown that AI has helped many companies to make better decisions about their operations (Felzmann et al. 2019). Consequently, participating entities have improved the quality of their decision-making processes. A reduction in the number of workers (as a cost-reduction measure) and the optimisation of marketing processes through sales are the least documented benefits of AI (Fuatx 2019). However, some researchers have drawn comparisons between the effects of AI on business processes with the impact of the internet on corporate operations (Cortese & Walton 2018). They suggest that AI will have the same effects on business operations, or at least follow the same pattern of change, as the internet did in the 1990s.
There is little doubt among scholars that AI is already changing how people conduct businesses and who is running them (Fuatx 2019). From this assertion, there is a growing school of thought, which suggests that AI will first destroy conventional business principles before it is rebuilt again to accommodate new developments (Felzmann et al. 2019).
Here, the integration of AI into business processes should be conceived in the same manner as the growth of a plant because they both require nurturing because different business styles are assimilated into the broader technological platform (Felzmann et al. 2019). Therefore, it is difficult to conceive a situation where change occurs abruptly and an organisation absorbs the shocks without affecting core organisational processes. The transition has to occur slowly because seamless integration is likely to be realised only when all stakeholders consent to it and new pathways of integration are identified.
The realisation of improved connectivity will happen through increased data collection, enhanced training and improved engineering processes (Cortese & Walton 2018). Consistent monitoring also needs to occur at this stage to make sure that AI improves accuracy and refrains from becoming a destabilising force for an organisation (Cortese & Walton 2018). Some studies have shown that changes involving AI should be carried out through an explicit recognition of the challenges affecting a business and the prevailing metrics of success used to measure performance (Frude & Jandrić 2015).
However, running multiple AI models at the same time is the main problem associated with making sure this alignment is realised. Therefore, there is a need to make sure data, business and technical teams are working seamlessly together to guarantee success. According to figure 2 below, the transformation of AI processes has to occur by cementing four key pillars of excellence: business, data, technology and learning.
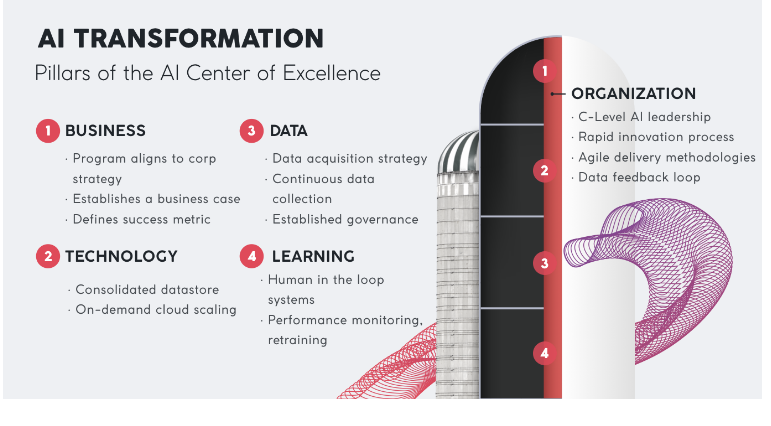
The business pillar of AI transformation requires the proper alignment of existing programs with the overall corporate strategy. Therefore, the AI technology to be adopted needs to help establish a business case. Stated differently, the use of AI should make business sense (Fuatx 2019). This process may involve a cost-benefit analysis but a success metric needs to be identified first.
Comparatively, the technological pillar of AI integration requires the creation of a consolidated database of analysis and on-demand cloud scaling. This process also demands the recognition of the concept, which should define the data acquisition strategy. This process demands that companies should continuously collect data that would be used to develop AI-based algorithms (Fuatx 2019). There should also be an established governance model that would define the data treatment process (Fuatx 2019).
According to figure 2 above, the learning component is the last pillar of AI transformation. This tenet of the transformation plan defines the focus of this study, which is training and education because it suggests that these two processes should keep professionals in the loop of AI transformation processes. It also encourages high levels of performance monitoring and retraining to equip personnel with the requisite skills for completing the transformation process (Fuatx 2019).
A different group of researchers suggest that the integration of AI into organisational processes requires the creation of a centre of excellence in corporate entities (Rana 2018; Carriço 2018). Furthermore, they suggest that there needs to be a new department formed to oversee the process and follow business-led decisions aimed at fulfilling the corporate strategy (Rana 2018; Carriço 2018). The centre of excellence is expected to consolidate and govern all organisational processes that require AI integration. Indeed, from a business standpoint, all AI functions need to be closely integrated with existing corporate objectives (Fuatx 2019). Based on this relationship, machine learning objectives are linked with data analytics and the development of measurable outcomes.
It is projected that new roles and positions, such as a Chief AI officer, will be formulated or developed to manage the above-mentioned functions (Fuatx 2019). There is also a high possibility that new positions (such as AI strategists and product managers) will be developed to ensure data collection supports the overall corporate mission. The creation of these new roles in organisational structures would make sure there is a proper definition of team success (Fuatx 2019). They would also make sure there is preferential treatment for projects that have the most impact but require the least amount of resources (Fuatx 2019).
Most AI-driven organisations recognise the above developments and depend on technology and data to drive their organisational processes (Kirschner & Stoyanov 2018). Concisely, new technologies are often needed to acquire and process data. This is because AI models demand a significant incorporation of new data to produce reliable solutions for business problems. Therefore, data creation is the most important part of AI development and many organisations are exploiting the opportunities created by the generation of free data from content users to enhance their systems (Fuatx 2019).
Creating this type of data also requires significant computing power to train and run models. However, many organisations will not invest heavily in the development of sophisticated analytical processes because they come with significant infrastructural requirements on organisations (Kirschner & Stoyanov 2018). Instead, they prefer to outsource the process through could-based computing services (Fuatx 2019).
Based on the above insights, data generation should not be conceived as a one-step process but rather a continuous activity that needs regular monitoring. Therefore, when organisations create new roles and positions in an organisation, it will be easier for them to indentify existing data and find a way of capturing it to improve AI systems. However, privacy and security policies should govern the process.
Broadly, several researchers take a positive view of AI by saying that its benefits cut across different industries and functions (Cortese & Walton 2018; Ferri et al. 2018). However, most of them suggest that its early stages of adoption and implementation were confined in consumer application systems (Felzmann et al. 2019). Over time, the trend has changed and businesses are increasingly adopting it in different aspects of their operations with striking results (McKinsey & Company 2019). Their findings have shown that AI can improve business applications in different areas of operation, including predictive maintenance and the detection of anomalies in factor line assemblies (McKinsey & Company 2019). These advantages are ordinarily observed when high volumes of high-dimensional data are used in AI (McKinsey & Company 2019).
The aviation sector provides an example of an industry that has effectively integrated AI applications in its operations (Kaartemo & Helkkula 2018). For example, aircraft manufacturers have used the system to improve the detection of engine problems (McKinsey & Company 2019).
In some cases, technology has been used to autocorrect operational functions (Fenwick & Edwards 2016). The transportation sector has also had a similarly successful application of AI technologies in delivery route maintenance, optimisation of service orders and improvements in fuel efficiency (McKinsey & Company 2019). The service industry is also another group of businesses that have effectively integrated AI in the internal business processes and are reaping its benefits through improved customer service and sales (through improved integration of customers’ demographic details with service-centred processes to provide individualised services) (McKinsey & Company 2019).
The increased productivity and efficiency generated from AI mostly come from improvements in traditional analytical techniques (Kaartemo & Helkkula 2018). Statistics also support this finding because they show that AI improved productivity and efficiency in about 60% of the times used (McKinsey & Company 2019). Figure 1 below shows that AI can improve the incremental value of products and services offered in different industries by varying percentages.
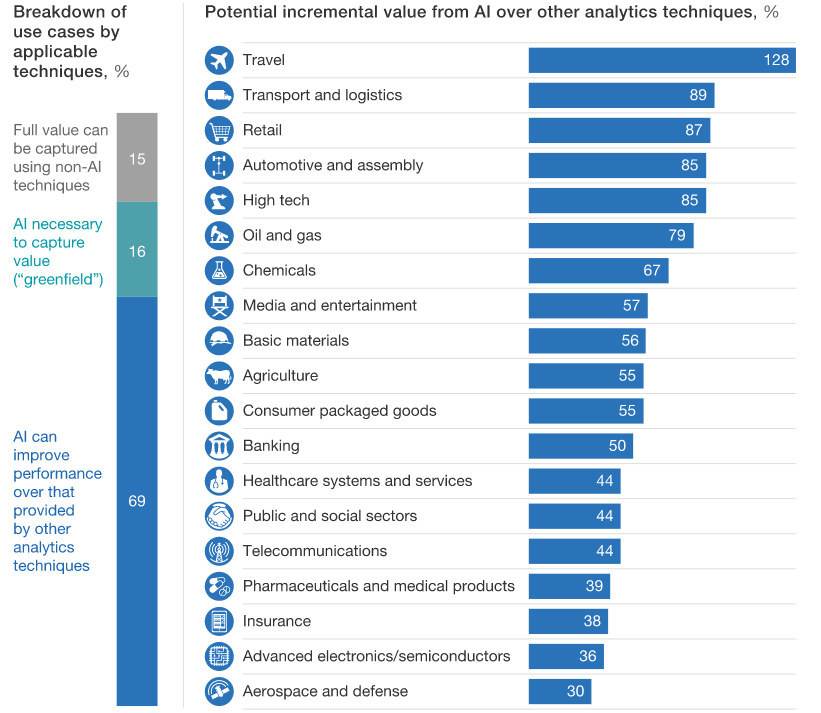
According to figure 1 above, the travel industry stands to benefit the most from AI services. The transport and logistics industry has also shown potential in improving process outcomes using AI. However, the fields of advanced electronics and aerospace engineering have benefited the least from AI. Nonetheless, the technology remains one of the best catalysts for improving business process outcomes.
Researchers have pointed out varying scopes and extents of AI adoption in different countries (Cortese & Walton 2018). The pace of adoption and the extent of AI integration have particularly defined this variation across multiple sectors (Kaartemo & Helkkula 2018). Several studies suggest that most companies have adopted AI in at least one of their business processes (Cortese & Walton 2018; Kaartemo & Helkkula 2018; McKinsey & Company 2019).
About a third of companies are also considering using AI in their internal systems but their efforts are still confined to pilot phases of review. The adoption of AI across multiple business processes is estimated at 20% but the highest percentage of integration has been observed in giant multinational firms, which are projected to adopt AI in at least 97% of their business processes (McKinsey & Company 2019).
Current research suggests that companies or firms that adopt AI tend to think of the technology as having vast effects on their mid-term and long-term goals (Liu et al. 2018). The potential to expand market share is the main motivation for using AI among most of these businesses sampled but slow adopters are more focused on cost reduction (McKinsey & Company 2019). Researchers have also noted that corporations or enterprises that have devolved their functions on the digital platform are the best adopters of AI (Lazzini et al. 2018). They are also considered the most probable companies to get the most value from the technology (Dudhwala & Larsen 2019).
An analysis of AI adoption across different sectors shows a widening gap between companies that base their operations on virtual production platforms and those that do not (McKinsey & Company 2019). For example, technology companies and financial entities are the leading adopters of AI. The gap between early and late adopters of AI in this field is deemed impactful on the competitive advantage of a business because early adopters gain a lot of experience with AI and use the same capability to leverage their operations, thereby making it difficult for the competitors to “catch up” (McKinsey & Company 2019). Figure 2 below shows that different industries and sectors of the global economy have varying adoption levels of AI.
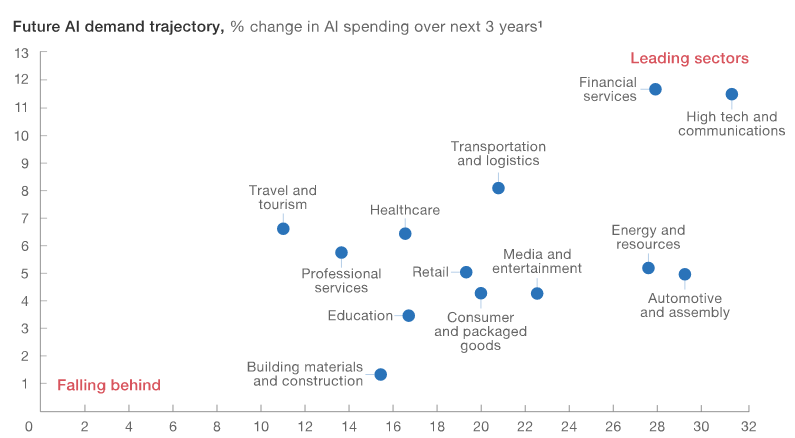
As mentioned above, the financial and technology sectors are the leading adopters of AI. The rate of technological adoption in the tourism industry, the transport sector and healthcare are also high compared to other fields, such as construction, education and consumer packaging businesses (McKinsey & Company 2019). These varying levels of adoption are not only linked to the nature of businesses in these sectors but also the attitudes of professionals in the industries (Frude & Jandrić 2015).
For example, the information technology (IT) sector is often comprised of professionals who are versant with current developments in AI, thereby allowing them to understand the technology better than employees from another sector, like the healthcare industry, will. Therefore, IT professionals are naturally going to have a stronger inclination towards the adoption of AI compared to their counterparts in the healthcare sector. Alternatively, it could be assumed that professionals in the technology industry have a more positive attitude towards AI compared to their healthcare counterparts. Nonetheless, several challenges to the adoption of AI persists and the burden of implementation has been left mostly to business leaders and managers who are expected to be committed to its adoption.
Attitudes towards Artificial Intelligence
The role of accountants in presenting the true financial performance of a company cannot be overemphasised in a fast-paced world where multiple variables affect corporate performance. This group of professionals not only maintain various systems of recording but also verify data contained in financial books of accounts. In this regard, the purpose of an accountant, in business management, is to present a monetary snapshot of a business or company’s financial health. By relying on professional insight, they could also predict what financial outcomes could occur in the short-term or near-term (Frude & Jandrić 2015).
Indeed, by understanding a company’s financial performance, it is easy to comprehend its strengths and weaknesses and their influence on corporate success. The introduction of AI threatens to change this traditional view of accounting by isolating key processes from human control.
Many researchers have pointed out that developments in AI could influence different aspects of professionals and personal lives (Cortese & Walton 2018). Evidence has been given of its potential effects on the labour market, transport industry, healthcare, education and other fields (McKinsey & Company 2019). Although pundits and policymakers have started to discuss the ramifications of AI, the opinions of professional groups have yet to be understood.
Particularly, the views of accounting professionals towards AI need to be framed within the context of public opinions towards the same phenomenon. For example, Zhang and Dafoe (2019) say that most people (44%) in America express support for AI, while only a small percentage (about 22%) have expressed strong reservations about it (McKinsey & Company 2019).
Evidence suggests that demographic differences could influence people’s support for AI (McKinsey & Company 2019). For example, people with high levels of education express more support for the practice compared to those who have lower qualifications (McKinsey & Company 2019). To explain this position, Zhang and Dafoe (2019) noted that college graduates expressed higher support (51%) for AI compared to those who had a high-school education (29%). Income disparities within households have also been linked with the support for AI because there was higher support for the concept (59%) among people who had an income of more than $100,000 annually compared to those who earned less than $30,000 annually (33%) (Zhang & Dafoe 2019).
People’s professions have also been associated with support or disapproval for AI because employees who hail from a technology background, such as computer programmers, have expressed higher support for AI (58%) compared to those who do not come from such a background (McKinsey & Company 2019). If this assertion were to be applied to the context of this study, it could be assumed that accountants would have lower support for AI compared to computer programmers or software developers.
Gender differences have also been associated with the support for AI because researchers have noted that men express a higher approval rating for AI (44%) compared to their female counterparts, who only have a 35% support for AI (McKinsey & Company 2019). Broadly, although demographic differences have been used to explain support or disapproval of AI, many researchers suggest that a majority of people believe that AI needs to be managed (Zhang & Dafoe 2019). The same view is also held by researchers who have explored people’s views of the use of robots to carry out human functions (Kaartemo & Helkkula 2018).
How Accountants are Using AI
To understand the future of AI in the accounting field, it is important to review how professionals in the same discipline are using AI today. Stated differently, it is vital to understand how AI is being used to solve accounting and business problems relating to today’s globalised environment. However, to gain a proper understanding of this role, it is pivotal to note that many accountants are commonly motivated to use their skills and expertise to help business managers and stakeholders make better decisions (Persson, Radcliffe & Stein 2018).
To do so, they rely on high-quality financial and non-financial instruments of accounting to make their technical analyses (Napitupulu 2018). This role is reflected in their work through several tasks, responsibilities and areas of specialisation that strive to enhance the quality of data available for review (Kaartemo & Helkkula 2018).
Technology has been used to improve the above-mentioned decision-making systems and has been deployed to solve three main types of problems: providing high quality and cheaper data for better decision-making, generating new insights from data analysis processes and freeing up the time to solve other pressing business challenges (Fenwick & Edwards 2016). Indeed, the nature of machine learning itself is predicated on the ability to improve accounting roles and equipping professionals with new skills and competencies to undertake their tasks (Sterne & Razlogova 2019).
Therefore, it is essential to identify accounting problems that could easily be solved using machine learning and those that may not benefit from the same process (or require human intelligence). This approach to problem-solving will make sure that changes are primarily driven by the fulfilment of business objectives and not technological requirements.
Studies suggest that there is limited use of AI in the accounting field but some common areas of operation where implementation has been relatively well-received are in the use of machine language to code accounting entries and improve the accuracy of rule-based approaches to accounting (Nijam & Jahfer 2018). AI has also been partially adopted in fraud detection and the prediction of possible areas of fraud activities (Napitupulu 2018). Machine-based predictive models of learning have also been developed using AI. Moreover, deep learning models for analysing unstructured data have also been developed from machine-based learning (Nijam & Jahfer 2018).
Practical Challenges Associated with the Adoption of AI
Although studies have shown the potential of AI in improving professional practice (Bechmann & Bowker 2019), many challenges still stand in the way of its full implementation. One of them that relates to training is the need for immense human effort in labelling data. This problem is challenging because supervised learning requires the proper labelling of data (Clarke et al. 2017). Furthermore, many organisations today have a problem of developing large amounts of data that are sufficient for training (Bechmann & Bowker 2019).
The complexity of machine learning is also problematic in data analysis and implementation because it makes it difficult to understand decision-making processes that lead to the formulation of potential solutions (Napitupulu 2018). This challenge is notably increased by the use of multiple variables in decision-making but it is impactful to the accounting field because the relationship between clients and professionals is often underpinned by trust. Since trust is a human attribute, it becomes difficult to account for it in the AI-aided decision-making process. Nonetheless, current research on AI is designed to address this problem by increasing transparency in model development (Sterne & Razlogova 2019).
Another challenge associated with the implementation of AI is the difficulty in generalising applications (Birtchnell 2018). The problem arises from the challenge of transferring experience from an AI model to another one. Stated differently, machine learning is often programmed to suit specific business contexts and it becomes increasingly difficult to exchange applications across different learning groups spread across various corporate sectors (Birtchnell 2018). This problem creates a governance issue.
Different researchers have highlighted the use of AI in the accounting field because human resource management has been the norm for many organisations in the sector (Lazzini, Iacoviello & Ferraris 2018). However, when there is a threat to the traditional role of human resources in the field, issues of governance emerge because it is difficult to ascertain how robots or machines can be managed, devoid of human control. Consequently, issues of governance have emerged as a key area of research in the integration of AI in the accounting space. More importantly, professionals and observers alike have raised concerns regarding how to prevent AI-aided technology from contravening existing liberties and privacy concerns in the business sector (Lazzini et al. 2018).
Similarly, the use of AI in propagating false information or misinformation through virtual platforms have also been explored in the same way as the potential threat of using AI to carry out cyber attacks on governments, companies and institutions have been investigated (Bechmann & Bowker 2019). Therefore, the main issue emerging in the investigation of governance issues relating to AI are commonly reviewed through a need to protect the privacy of data held by people and organisations.
Importance of Education and Training in Artificial Intelligence
Different scholars have highlighted the importance of education in accounting (Nijam & Jahfer 2018). Their goal is usually to equip professionals with skills and competencies relating to major developments in the sector (Stevenson, Power, Ferguson & Collison 2018). This goal is necessitated by the fact that the accounting field is linked with multiple changes in the operating environment, including technology, law and ethics (Nijam & Jahfer 2018).
AI falls within the context of technological development and education has been emphasised as a way of sensitising professionals about its implications on the sector. The current emphasis on research has been on how to use some of the skills and competencies offered by AI in the accounting field and how professionals or managers could harness them to solve existing problems, including employee retention and other human resource issues.
The importance of training is not only confined to improving practice but also reinvigorating traditional accounting practices by making them better and more efficient. Rapid changes in globalisation and the need to standardise accounting standards and procedures have further emphasised the importance of effective training in the accounting field (Gammie, Allison & Matson 2018). AI-related education processes are even more central to the growth of the discipline, relative to the impact that they have on the field.
However, some researchers have noted that some organisations are hesitant to plan for education seminars because of the perceived lack of adequate teachers or personnel to oversee the process (Gammie et al. 2018). Moreover, some managers and CEOs still deem some of the developments made in AI to be insufficient in necessitating radical changes in an organisation (McKinsey & Company 2019). This development is informed by the fact that there are still many major developments going on in AI that may deem some of the information currently available obsolete.
Summary
Anecdotal evidence gathered in this paper show that most of the accounting journals and works of literature that have investigated the link between AI and accounting do not sufficiently emphasise the role of education and training. In other words, the existing evidence is summarily explained without a keen understanding of the role of education and training in explaining what the concept is about and its influence on accounting. This study aims to fill this research gap. The techniques used by the researcher in fulfilling this goal are explained in chapter three below.
Methodology
Introduction (Project Summary)
This chapter will highlight the strategies adopted to answer the research questions. To recap, this study is designed to investigate the effects of AI on the accounting profession from the prisms of education and training. The study was guided by five questions, which focused on evaluating whether there have been changes in employee attitudes toward AI in the past five years, examining factors that could influence the attitudes of accounting professionals toward AI and investigating how the attitudes of accounting professionals towards AI could be improved. The approach taken by the researcher in answering the above-mentioned questions is discussed below.
Research Approach
The qualitative and quantitative research approaches are the main techniques used in academic research (Steen, DeFillippi, Sydow, Pryke & Michelfelder 2018). The latter approach is often used in investigations that measure quantifiable variables, while the qualitative technique is applicable when measuring subjective variables (Archibald, Radil, Zhang & Hanson 2015). The researcher used the qualitative research method in this study because it focused on the attitudes of accounting professionals regarding AI.
Attitude is a subjective variable because it is individualistic. In other words, two people may have completely different experiences when subjected to the same stimuli (AI). Similarly, they may have different perspectives on the technological phenomenon. These qualities of the research variable made it difficult to use the quantitative technique to undertake this analysis because it was not possible to measure the variables numerically.
The justification for using the qualitative research approach is rooted in the fact that it provided the researcher with an opportunity to get in-depth details regarding the questions asked (Allana & Clark 2018). In other words, it allowed the researcher to go beyond the superficial elements of the investigation and probe the respondents’ reasons for making specific statements. This advantage is highlighted by several research studies, which have investigated the merits of the qualitative research approach (Walker and Baxter 2019; de Block and Vis 2018; Wagner, Kawulich & Garner 2019).
Research Design
According to UMSL (2019), there are five main research designs associated with the qualitative research approach. They include phenomenology, grounded theory, ethnography, historical methods, and case studies (UMSL 2019). The characteristics of each of these designs are provided below.
Grounded Theory
The purpose of the grounded theory design is to develop a theory (Chun Tie, Birks & Francis 2019). Typically, many researchers use it to assess social problems and examine how people address them (Ralph, Birks & Chapman 2014). Before findings are developed, scholars often use prepositions to explain specific research phenomena after formulating, testing and redeveloping them (Collins & Stockton 2018). These characteristics of the grounded approach made it inappropriate for use in the study because its focus was not on theory development but rather on understanding the impact that AI would have in the accounting field. The second research design considered for review was the case study approach and it is discussed below.
Case Studies
Researchers often use the case study research design to gain an in-depth understanding of the workings of a specific social group or institution (Ebneyamini & Sadeghi 2018; Rule & John 2015). The main method of data collection is observation because scholars often immerse themselves in a group’s social structure and observe patterns of behaviour (Rashid, Rashid, Warraich, Sabir & Waseem 2019; Herdlein & Zurner 2015). Although these characteristics of analysis make this research design useful in understanding the inner workings of a professional group, it did not apply to this study because its focus was not on a specific social group or institution but a wide profession – accounting.
Ethnography
Researchers who want to describe the characteristics of a cultural group have typically used the ethnography approach to undertake their studies (Lubet 2019; Jerolmack & Khan 2017). They do so by gaining access to culture and gathering data by immersing themselves in it to observe behaviour (Rashid, Caine & Goez 2015; Newmahr & Hannem 2018). The focus on the cultural aspects of scientific dogma made it difficult to use this research design in the investigation. Instead, the study was focused on examining the effects of AI on accounting professionals. The next approach considered for review was the historical technique. Its characteristics are described below.
Historical Methods
The use of historical methods in qualitative research approaches is informed by the need to examine past events to make sense of current findings or anticipate future outcomes (Stutz & Sachs 2018; Chong 2014). This methodological approach is systematic in the manner research variables are assessed and by reconciling conflicting evidence (Crossen-White 2015; Kelly 2019). This dissertation did not follow a systemic approach to data analysis because the questions posed to the respondents were semi-structured. Furthermore, the focus of the study was not on the use of historical records to make sense of the research phenomenon. Consequently, this research design was not selected for use in the study. However, the phenomenology research approach was reviewed and its findings highlighted below.
Phenomenology
The main goal of the phenomenology research design is to describe people’s lived experiences (van Manen 2017). As its name insinuates, this research design is often associated with the examination of different phenomena that affect societies or professions (Ignatow 2018). From this analysis, the uniqueness of people’s lived experiences is observed because the main premise of analysis is understanding that people have different perceptions of reality.
According to Strandmark (2015) and Bovin (2019), scholars who use this research design are often preoccupied with the need to understand what people’s lived experiences mean for a research phenomenon under investigation. These characteristics of the research design made it appropriate for use in the study because AI was the phenomenon under investigation and the researcher intended to understand its impact on accounting professionals – a process, which is akin to understanding their lived experiences.
Data Collection
Data was collected using semi-structured interviews. The questions posed to the respondents were professionally developed because the informants were accounting professionals in different fields of business. Interviews were selected because they allowed the researcher to explore the research questions in-depth compared to alternative data collection methods, such as surveys, which only require a “yes” or “no” response. The interviews were conducted online on the WeChat platform. The researcher selectively used this technique because of the logistical difficulties of conducting the interviews face-to-face because the respondents were in China.
Research Participants
The target population was comprised of five professionals who have advanced experience with the use of artificial intelligence in the accounting profession. They were based in China and worked as teachers or accountants. The respondents were made up of two accounting teachers from a Chinese education institution and three professionals who worked as accountants in a Beijing-based financial institution. The accountants held junior, semi-senior and senior-level positions in the workplace.
The exact positions held in the organisation were that of a chief financial officer (CFO), technology manager, chief executive officer (CEO) and a human resource (HR) manager. Since all the informants were Chinese, the researcher spoke to them in the same language. However, for purposes of data analysis, findings were transcribed into the English language and the final report presented in the same manner. The interview questions posed to them are provided in appendix 1.
Sampling Procedure
The researcher used the purposive sampling method to contact the respondents. This non-probability sampling method is selective in the manner respondents are chosen because there is a bias to only recruit informants who are knowledgeable about the study area (Ignatow 2018). In other words, the selection of the informants depends on the interviewer’s judgement. The researcher employed the purposive sampling method because some of the respondents were known through family members. Therefore, it was easy to contact them and schedule an interview. This technique was appropriately used for this study because it is commonly applied when a small sample is desired (Brayda & Boyce 2014).
The small number of participants was chosen for the study based on the recommendations of Brayda and Boyce (2014), which suggest that a sample of fewer than 12 participants is appropriate for a comprehensive qualitative study. This figure is targeted because a high number of participants could make it difficult for the researcher to establish a good relationship with all of them. This concern is raised because qualitative investigations require the researcher to develop a good rapport with the respondents.
Data Analysis
The researcher used the thematic and coding method to analyse data. Armborst (2017), Aveling, Gillespie and Cornish (2015) say that many researchers have successfully used the technique to analyse qualitative data. Today, it is among the leading models of information analysis for the qualitative research method (Armborst 2017). Its proven reliability made it attractive for this study (Elo, Kääriäinen, Kanste, Pölkki, Utriainen & Kyngäs 2014; Ando, Cousins & Young 2014; Nowell, Norris, White & Moules 2017).
Consequently, important themes were identified from the interview responses provided by the informants. The process was done by recognising patterns of assertions that would help to answer the research questions. The identified patterns were later correlated with different research nodes, as suggested by Hilton and Azzam (2019). Developing the nodes later paved the way for the coding process and the development of the research findings. Overall, the researcher undertook the thematic and coding method by following the steps highlighted in figure 3 below.
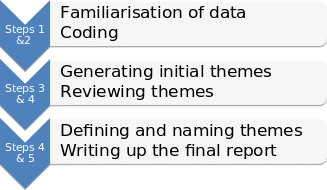
Ethical Considerations
It is important to review the ethical considerations of this paper because human subjects were used. According to Colnerud (2014), Saunders, Kitzinger and Kitzinger (2015), the use of human subjects emphasises the need to conduct a study ethically to protect the rights of the respondents and the researcher. The main ethical issues the characterised the study are as follows:
Anonymity and Confidentiality
Sugiura, Wiles and Pope (2017) say that anonymity and confidentiality in qualitative research are closely associated with the respect for human dignity and fidelity to the research objectives. Relative to this assertion, the views of the respondents were presented in the study anonymously. In other words, their identities will not be revealed unless expressly stated by the informant in writing. The aim of doing so is to protect them from any consequence that may arise through a publication of the research findings (Lowman & Palys 2014). As suggested by Hannes and Parylo (2014), doing so will make sure that the views presented in the investigation are solely aimed at answering the research questions and not to probe an informant’s personal life. This ethical provision also allowed the participants to give or withhold as much information as they wanted (Scarth 2016).
Voluntary Participation
Brewer (2016), Dixon and Quirke (2018) say that informants should voluntarily be allowed to participate in research studies. Relative to this provision, all the respondents who took part in the study did so voluntarily. Stated differently, they were not coerced or paid to give their views. Furthermore, they were informed of their rights, details of the study and its purpose before participating in the investigation.
The purpose was to equip them with all relevant information that would help them to make an informed choice about their involvement in the research. Before volunteering to participate in it, they had to sign an informed consent form. Lastly, the informants were also informed of their right to withdraw from the study without any repercussions. Therefore, they did not feel like they were indirectly forced to participate in it.
Reliability and Validity of Data
It was important to understand the reliability and validity of the interview data because researchers note the difficulty in replicating these types of investigations (Doll 2018; Jackson, Kay & Frank 2015). Stated differently, the flexible nature of qualitative inquiry makes it difficult to replicate the findings, thereby making it easy to question the reliability of information generated. To address these issues, the researcher used the member-check technique to improve the reliability and validity of information obtained from the respondents. This method works by relaying the study’s findings back to the respondents so that they assess whether they represent their actual views, or not (Oranye 2016).
Therefore, the researcher furnished the informants with a statement of the final findings before they were included in the edited report. The respondents had an opportunity to state whether the information presented represented their views, or not and adjustments were made appropriately. Broadly, the member-check technique is an appropriate tool for improving the reliability of qualitative findings. Its merits have been highlighted by several researchers, including Jackson, Kay and Frank (2015).
Findings
Introduction
This chapter outlines the results obtained from implementing the strategies outlined in chapter three above. Key sections of the analysis explain the themes and codes developed from a review of the data pertaining to the respondents’ views regarding different areas of AI implementation. However, before delving into these areas of analysis, it is important to review the demographic characteristics of the informants.
Demographic Findings
As highlighted in chapter three of this dissertation, the researcher interviewed five respondents who practiced as teachers (2) or accountants (3). The accountants held junior, semi-senior and senior-level positions in the workplace. The exact positions held in the organisation were CFO, technology manager and a HR manager. The CFO gave valuable insights regarding the effects of AI on computing the financial performance of a company because this position is associated with long-term financial planning, management of company finances, risk management and record-keeping (among other tasks). These responsibilities are closely aligned with accounting tasks because the profession is related to the development of financial statements to present the true performance of a company.
The above-mentioned tasks and responsibilities mostly relate to a CFO’s duties. Therefore, the inputs provided by this respondent were insightful in understanding how AI affects current accounting and financial processes of an organisation. The views provided by the technology manager were similarly insightful because they provided explanations regarding the effects of AI on IT-related tasks. Therefore, discussions relating to technological integration and the hardware components of AI were comprehensively discussed in the interview with the technology manager.
The views of the HR manager were similarly vital to the analysis because the effects of AI on human resource were a key part of the discussions. Therefore, integrating the views of the HR and technology managers in this report provided a balanced understanding of the study questions because AI is a technological tool, while human intelligence is a HR topic.
Broadly, the respondents held the above-mentioned positions for more than five years and had not vacated them from the time of company inception. This lack of mobility could largely be attributed to the fact that they were all working for small businesses, which did not have a high staff turnover. The long-term nature of their employment statuses meant that they observed the effects of AI on business performance much more keenly than an employee who had worked for shorter periods. Lastly, the integration of the view of teachers and practicing professionals in the interviews was to gain a dual understanding of the effects of AI from professional and educational perspectives. Therefore, the discussions presented in this chapter are holistic.
As highlighted in the methodology chapter of this dissertation, the thematic and coding method was used as the main data analysis tool. The researcher used this technique to review hours of interview data. Seven themes were generated as outlined in table 1 below.
Table 1. Themes and Codes Generated (Source: Developed by Author).
The first theme identified in the discussion was the impact of AI on job losses. The findings appear below.
Impact of AI on Job Losses
One of the biggest fears associated with the implementation of AI in the accounting field is the possible loss of jobs because conventional literature suggests that increased integration of AI machines in organisational tasks would render some tasks obsolete (Miller 2019). The respondents were asked to comment on this matter and they presented mixed views. The accounting professionals suggested that job losses are inevitable because, unlike a human being, a machine can do multiple tasks at the same.
The teachers disagreed with this view and said that new areas of competence requiring human intervention will emerge through the increased adoption of AI functions. However, they admitted that some job losses are inevitable because of the increased efficiency of machines, subject to human potential. Accounting areas that require an interpretation of large amounts of data were identified to be the most vulnerable areas of job losses because they were most likely to be influenced by machines.
However, many organisations have already automated most of these tasks (Miller 2019). Therefore, it is unlikely that significant job losses would emerge from this area of competence. However, human intelligence will be relied on in corporate decision-making areas and in the development of operational strategies in an unpredictable business environment.
Therefore, all information that were to be captured by AI, but are unusable, had to be disregarded. Thus, there was a strong need to check the information relayed in these machines to improve relevance. Human expertise is required in this task because it makes sure the “big picture” is observed and respected. The path to the realisation of the “big picture” can be redefined or changed but the respondents insinuated that the goal had to remain constant.
The integration of human and artificial intelligence was also proposed as a viable strategy to prevent job losses because it is easy to create solutions through this development. The place of human intelligence in the decision-making structure is still safeguarded because new developments have to be made to improve organisational processes. It is easy for such a situation to happen because AI works by following a set of algorithms.
These algorithms are often repeated until an update is required. The respondents referred to this review as a “key human function.” Stated differently, people will be needed to constantly re-evaluate AI functions and make sure they are still working towards achieving their intended goals. More importantly, employees are required to examine the system from multiple angles and develop solutions that do not necessarily have to align to specific or existing patterns of development. To support this view, one of the respondents said,
You have to look at it like a blend of interpersonal and intrapersonal influences. A combination of both forces would help the system to adapt accordingly. Therefore, when AI is introduced into an existing system, it does not only create new processes but also develops new areas of development that require adaptation. In other words, human beings have to adapt and not the machines. By orienting themselves to the new order of things, the professionals would later find that novel areas of job development could emerge. For example, look at market research… the demand for new knowledge is extremely high. It is almost like a niche market. Human intelligence is required on this front.
The above assertion shows that the respondents favoured a segmented labour structure where job losses would be reviewed based on their importance to organisational functions. Low-paying jobs were deemed to be most affected because machine learning could replace simple tasks. However, higher-order jobs emerged as having the least probability of replacement because their complicated nature required human sophistication. Therefore, the worry about job losses was confined to lower ranking jobs.
One of the respondents affirmed the above assertion from a data sourcing perspective. He said that there is insufficient data to use in AI-aided processes to make effective and rational decisions regarding complex tasks. This assertion stems from current data collection problems linked with AI because there is an insufficient supply of quality and reliable data to use in these machines. Furthermore, some areas of application are insufficiently explored. Therefore, there is a similarly inadequate source of data coming from these quarters. In such cases, expert opinions are highly valued, thereby securing the place for human intelligence in AI processes.
How AI is Changing Accounting Processes
The review about the effects of AI on accounting processes comes from the conflicting views regarding whether it has positive or negative effects on the accounting profession. The literature review findings suggested that the effects are largely positive but the experts sampled presented a mixed review of its impact on the accounting sector. One of the respondents said that understanding the effects of AI on accounting processes requires an examination of how some giant multinational firms are using the technology. He said,
Look at how EY and Deloitte are using AI in their daily accounting processes. Their document review strategies are being updated using AI everyday. Initially, these organisations had to rely on extensive and protracted labour-intensive processes to undertake the same tasks and to transfer ownership of client assets. This has fundamentally changed. Just based on these examples alone, I would say that AI is doing the job that most accountants would do when examining convoluted contracts. I should add that this is just “scratching the surface” (laughs).
One of the respondents also said that stakeholders should consider AI a tool for improving the efficiency of undertaking accounting procedures and refrain from looking at it as a negative tool of development, which is designed to take away their jobs. Relative to this assertion, one of the respondents said the best way to encourage accounting firms to appreciate AI is to make them look for areas of redundancy or bureaucracy that could be solved using the technology. By doing so, they would be looking at AI as a solutions provider, as opposed to a problematic “trend” in accounting.
Another respondent said that professionals should stop opposing AI because it is a “lost war.” Instead, he proposed that they should adapt to the new times and re-evaluate how it would enhance their roles. When asked to clarify how such an evaluation should occur, the informant said that accounting professionals should consider looking at their positions as that of an “adviser” and not a “doer.” In other words, since machines can carry out conventional accounting processes with higher accuracy and efficiency than a human being, their accounting inputs should be confined to advisory.
Furthermore, few employers would choose an employee over a machine because people require a salary at the end of the month and a machine does not have such demands. Consequently, the respondents considered comparing AI to human beings as a fruitless exercise because human beings cannot match the efficiency of a machine. Furthermore, employees come with their challenges, such as the need to keep them motivated or happy in the organisation, while machines do not have this problem. Therefore, the respondent said, from a suitability perspective, accounting professionals need to rethink their role in the field.
How Artificial Intelligence will affect the Industry
One of the respondents suggested a different view of the effects of AI on the accounting field by saying that it has the potential to create new jobs, as opposed to “killing” them. He believed that AI would create new positions for accountants, especially in the areas of training and testing because a lot of work is needed in developing workable AI tools and integrating them into accounting processes. This exercise will not only be dependent on machine learning but also human intelligence. He also suggested that there would be new job opportunities created in auditing algorithms because of developments in AI intelligent systems.
However, he cautioned that this progress will only happen if accountants take part in projects that involve AI integration because it is the only way they would be able to come up with solutions to familiar challenges. He also said that integrating results into business processes requires the involvement of accountants in linked projects. Other professionals are expected to be more involved in the direct handling of inputs and outputs. For example, exception handling and data preparation may be associated with these tasks.
The respondents also said that the evolution of the accounting practice, in the wake of changes that are stemming from AI, would have to be reflected in their skills and competencies. For example, they needed to have sufficient knowledge in machine learning to understand AI processes. The respondent also said that critical thinking should emerge as one of the most coveted skills accountants should have. In addition, they recommended that communication skills will also become increasingly important in the future.
AI and the Professional Context
When asked whether AI could replace human jobs in the accounting sector, one of the respondents said there is a need to review this issue within an institutional context because accounting is a profession exercised within this limit. Notably, he drew attention to the role played by regulators and standard setters in the accounting profession because they have veto power to define acceptable and unacceptable accounting practices, regardless of whether they involve AI or not. Therefore, if they are not comfortable with the risks of AI, they could easily stifle its advancement in the profession. To support this assertion one of the informants said,
For you to understand the importance of institutional change in the accounting profession, it is pertinent to look at auditing… for example. This is a highly regulated sector of the accounting field and it would be difficult to make any significant change without effective reforms in the legal environment. For example, I believe it is standard procedure for regulators to ask auditors where they get their evidence. If it comes from AI, they would ask different types of questions. More importantly, if they do not understand how AI helps to generate data, they would completely “shoot it down.”
The same respondent noted that even when regulators understand how AI should be integrated in the profession, issues of reliability might emerge. Therefore, they need to overcome this hurdle as well.
Regulation emerged as a significant issue of concern involving the integration of AI in the accounting profession. However, when the researcher asked the respondents about the same issue, they did not only have a bleak outlook of AI because regulators in the sector are already discussing the regulatory limitations of AI adoption. Therefore, they argued that it is possible for the limiting framework of AI to change in the coming years as stakeholders in the sector continue to understand its implications on the industry.
One of the informants also said that he has been a part of similar discussions in China because considerations about machine learning already form part of their discussions. He also said that many institutions in China are having similar discussions and some have even gone ahead to implement AI, as a competitive advantage over their rivals, without much consultations from stakeholders.
One of the respondents said that the skills and training agenda for accountants in the context of AI use has been a subject of debate for many accountancy-linked professional bodies as well as educators and peers in the field. Current discussions propose the need for more training, especially in areas of technology and data management. One of the respondents also mentioned the need for improving soft skills in the areas of analysis, thinking and adaptability. The need for life-long learning was also mentioned as a tool for making sure professionals always remain abreast with developments in the field. To support this statement, one of the respondents said,
What we need to realise is that there are competing visions for the future of AI, especially for highly qualified professional bodies and lower-cadre employees. Particularly, the use of technology to access expertise knowledge influences most of the decisions and perceptions of the future.
Another respondent said that change and adaptability to AI in the accounting profession will only be realised when there is a consensus among most stakeholders on how to overcome this challenge. Particularly, it will be easier to embrace change when they agree on one direction to follow concerning the adoption of AI.
When asked to explain their views regarding the ability of machine learning to replace human intelligence, one of the respondents said that such an outcome is unlikely to occur because of the difficulty in replicating human intelligence. In this regard, he said that accountants should not be fearful of losing their work because human intelligence also evolves. To support this assertion, he said..
I do not believe people should be worried because we cannot be threatened by a system that we created in the first place. I do not conceive a situation where human beings would be helpless to machines, especially when they are believed to cause harm. All you have to do is read a few books about human resilience or just look around to know that we have survived many adversities and AI is not going to be the one that stops us on our track.
Based on the above assertion, the respondent said the best way to secure the future of accounting is to make sure that human beings and machines work together.
AI and Education
One of the main issues discussed with the respondents was the correlation between effective AI use and higher education. This topic of discussion was necessitated by the sophistication of AI technologies and the possible need to develop new skills to be able to operate or integrate them into everyday practice. There was a consensus among the informants that some type of higher education is needed to operate AI-aided machines. In fact, they alluded to the creation of advanced specialised companies that would be servicing these machines because their sophistication would not allow untrained personnel to understand what to do. To support this assertion, one of the interviewees said,
I believe it will be more like how we purchase and use software. The software creators will essentially be the developers of the technology and they will sell it to accounting firms who will then rely on their expertise to integrate the technology onto their existing platforms. I do not think much of the maintenance and operational activities of AI will be left to the accountants… perhaps only the learning and orientation parts. That may need some level of education.
The above assertion suggested that the respondents did not think higher education was of critical importance to the operationalisation of AI technologies in accounting. However, when the respondents were asked to explain the kind of changes that should occur in the accounting sector within the nest two, five or ten years, they suggested that the mindset of stakeholders need to change before any meaningful reform can be realised. They believed that the greatest hurdle to the implementation of AI in the sector was not because of hardware of resource limitations but people’s resistance and ill-conceived attitudes about the technology.
However, one of the informants differed with his colleagues when he argued that the curriculum needs to pave the way for the realisation of significant changes in the practice. He believed that the software changes mentioned should happen in the classroom setting, as professionals need to be equipped with the requisite knowledge needed to comprehend the benefits of AI. Therefore, he believed that curriculums need to be updated to include more educational materials about the impact of technology on the practice. However, this respondent was cognisant of the limitations of such a development by recognising that technology keeps changing and it will not be sufficient to equip the students about one aspect of IT, such as AI.
Therefore, he believed that technology should be reviewed in its totality and incrementally be added to existing curriculum at a basic level of learning. The respondent also said that current accounting certifications need to be reviewed and updated to include courses on AI. The idea stemmed from the belief that it was impossible to be an effective accountant in today’s business world without having a proper understanding of AI. Stated differently, it was inconceivable to think of a certified accountant who had little understanding of AI.
Besides the analysis on education, the informants also said that in the short-term, educational institutions, professional bodies and accountants should reallocate their resources to support AI integration. This area of management is relatively underfunded because few managers understand the importance of AI in the field. However, with a change in attitude, emphasis can be redirected to researching the best ways to integrate AI into accounting processes.
In the long-term, the researchers proposed that organisations should develop separate departments for managing AI-related matters. They also said that the creation of AI posts should be done because this is a specific area of application that requires critical attention. However, they recognised that this proposal might affect the governance structure of the existing financial system because it is designed to rely on brick-and-mortar processes or outdated accounting practices.
However, digitising financial systems could pave the way for the integration of AI-aided accounting processes, thereby eliminating the need for physical visits or audits of financial accounts. Furthermore, integrating AI processes into the financial system would enhance transparency and increase investor confidence because people often trust machines better than they do human beings. One of the respondents added that if these changes are adopted, they would affect the recruitment and professional training systems of accountants.
Effects of AI on Organisational Structure
The researcher sought to find out the respondents’ views about the effects of AI on organisational structures. This issue emerged from a review of studies, which suggested that AI could influence how businesses are organised because of its ability to render certain positions obsolete (Cortese & Walton 2018; Kaartemo & Helkkula 2018; McKinsey & Company 2019). The ability of AI to create new positions in an organisation also informed this discussion because there was a need to find out whether this change would affect the structure of the organisation.
Most of the respondents said that AI would have a significant impact on organisational structure by creating new models of data analysis based on its potential to create different ways of doing business.
This predicted change was compared with the reforms that the internet brought to the business environment in the 1990s. For example, the position of information manager was non-existent in the pre-internet era. Therefore, the adoption of IT tools in the contemporary society necessitated the creation of this position. This type of change is expected to influence the accounting sector when AI becomes widely adopted. Two of the respondents also said that organisations have consistently reorganised their departments to cope with such changes. From the process, new internal organisations and roles have been created, as different tasks require a new set of skills and competencies. The sum total of the projected changes is expected to significantly affect organisational structures.
Skills Needed in AI
One of the issues discussed in this study was the nature of skills required to operate AI-aided machines. This topic of discussion emerged from concerns about job losses that could occur because of the widespread use of AI. The respondents suggested that professionals should acquire computer literacy skills as a key part of their training plans. This view was informed by the belief that most AI technologies that will be developed in the future will be computer-based. Three of the respondents also said it was vital for professionals to acquire administrative and leadership skills because these are key tenets of future accountancy practices when machines replace most low-level jobs. To support this view, one of them said,
Leadership is a human attribute that will not be easily replaced by machines. Therefore, the acquisition of such skills would improve an employee’s job security by equipping them to take new positions.
At a technical level, the researchers said professionals who acquire mathematical and algorithm skills will have an added advantage over those who do not get the same skills. These competencies could be complemented with the acquisition of problem-solving and analytical skills because most AI processes are geared towards such tasks. Therefore, the respondents believed that gaining a deeper insight into this area of analysis would enhance the skills that a professional would need to navigate current changes in the field.
One of the respondents disagreed with the above view by arguing that most of the skills required in AI development are not related to accounting but other supporting sciences, such as computer science. Therefore, he believed that a distinction should be recognised between the skills an accountant would need in AI development and the competencies underlying AI change in the first place. The competencies required to develop AI is a prerogative of software developers, computer sciences and other professionals in the IT field. However, future accounting tasks should be focused on human tasks.
The informants also recognised that professionals who have acquired specialised knowledge in AI would be considered for high-level positions in the industry. This is because AI is an “added advantage” in accounting because it offers a new tool for completing human tasks.
Summary
The findings generated from this study show that the successful implementation of AI in the accounting practice requires both dedication and commitment to the process. There is also a need to acquire commensurate skills of operation to effectively manage any change that would occur from its implementation. The biggest challenge that many organisations face is abandoning long-held traditions and procedures in accounting (Miranti 2014).
Therefore, it is difficult to effect the change process. Particularly, this is true for many organisations because many accountants were not trained to have AI skills. Therefore, they have to participate in organisational processes and seminars that would update them on developments in the field. The findings highlighted above presented a holistic picture of the study issue because professionals and educators alike provided the insights documented.
Although the views outlined were insightful, they portrayed a mixed review of the effects of AI on the accounting practice. In other words, it was difficult to develop an absolute understanding of the effects AI on accounting. Based on this outcome, it was necessary to compare the findings with what other researchers have said about the study topic. The discussions of the investigation appear below.
Discussions
Introduction
This chapter is a critical review of the findings highlighted in chapter four above. Key sections show how AI can be integrated in the accounting field, challenges associated with the process and the future of the technology on the practice. However, a broader review of its impact on accounting is provided below.
Impact of AI on Accounting
Some of the first AI tools developed were reliant on expert advice, which was later built into existing systems, thereby enabling them to make decisions or develop recommendations in the manner a human being would (Gammie et al. 2018). Although this technique produced viable results, the quality of output was not the same as human intelligence (Gammie et al. 2018). Therefore, despite the technicalities involved in making such models, they could not withstand the realities of the business environment. Particularly, the system was ineffective in making decisions, which had to rely on ambiguous knowledge.
Based on the findings highlighted in this paper, the place of AI in the accounting sector is secured because evidence shows that it is increasingly influencing decision-making processes (Kirschner & Stoyanov 2018). Although accountants have always used technology to undertake their business processes, AI offers an opportunity to improve the quality of decisions made. In this regard, it should not be seen as an invasive element in the profession because its purpose is to improve the quality of output.
Therefore, to achieve this goal, AI should be conceived as a tool for making prudent accounting decisions. Consequently, it would be unwise to obstruct its use because of fear and cynicism, which are mostly unsubstantiated. Furthermore, few researchers have explored its impact in the accounting sector. Possibly, existing fears are motivated by wrongful applications of the tool in other industries. However, stakeholders may not understand that other industries have a different set of variables affecting AI implementation.
The findings of this study suggest the existence of mixed views regarding the adoption of AI in accounting. However, the examination of contracts in the accounting field using AI is one area of interest that emerged in the study to show the extent that the technology has been integrated into the discipline. This issue was mentioned by the respondents and highlighted in excerpts of studies done by Amnuai (2019), Naujokaitiene, Tereseviciene and Zydziunaite (2015). Both sets of informants notably mentioned how giant multinational, accounting firms, such as Deloitte, have used AI to examine contracts and client agreements.
It is important to understand that this task was mainly a preserve of the professionals because it is a qualitative process, subject to the ability of human beings to understand varied implications of contractual agreements. However, as demonstrated by the informants sampled, AI tools have already been equipped with the capability to analyse such contracts, thereby carrying out tasks that would otherwise be deemed impossible for a machine to do.
Accounting firms that are unfamiliar with AI need to rethink how they undertake their organisational processes because they will lose the competitive advantage they should have over other industry players who are yet to acquire any experience by using it. However, integrating AI into existing organisational processes is difficult because of the need to make several adjustments concerning organisational processes (Dudhwala & Larsen 2019). As Murphy and Quinn (2018) point out, these adjustments may involve fulfilling their roles as trusted professionals and even devising new ways of meeting client expectations.
The accuracy of predicting the impact of AI on accounting largely depends on people’s ability to understand differences between human and machine intelligence and how the two work together to improve business outcomes. So far, several researchers have explored this issue and pointed out that human intelligence could broadly be classified into two categories: intuition and reasoning (Lazzini et al. 2018; Kaartemo & Helkkula 2018). People’s subconscious thinking often influences the intuition part. Decisions made within this context may be quickly processed or involve little effort from concerned parties because of the use of well-established thought patterns. This type of thinking may require support from professionals to recognise patterns of analysis and make intuitive decisions based on history.
Logic and reasoning also affects human intelligence because people often rely on existing knowledge to make informed decisions. Accountants are typically trained to use intuition and logic because of their reliance on knowledge and training to make informed decisions about various issues. For example, most of them make intuitive decisions based on their experiences in the field.
Although people use intuitive thinking on different aspects of their daily lives, it is susceptible to bias. AI has the potential to address this concern because it is detached from human emotion. For example, Kirschner and Stoyanov (2018) point out that it can address availability and confirmation biases because of their high accuracy. Current approaches to AI will continue to improve on human competencies but they come from a long line of logic-based rules and decision-trees that may be difficult to change (Lazzini et al. 2018). However, AI will continue to try to replicate human intelligence and thinking patterns.
Integration of AI with Other Technologies
This study has demonstrated the advantages of AI and its potential in improving the efficiency of operations in the accounting field. However, what many respondents sampled in this dissertation failed to account for was the multifaceted nature of AI and how different technologies could be plugged into the platform to improve accounting process. For example, developments in the technology sector could be easily interfaced with accounting processes (using AI) because the latter outlines a broader group of machine learning technologies and not necessarily a single application. Therefore, the kind of applications that could be developed and interfaced on the AI platform is unlimited.
Block chain and quantum computing are only a few technological platforms that easily meet the criteria of AI integration in today’s accounting practice (Kirschner & Stoyanov 2018). As accountants ponder on how to integrate AI into their existing tasks, it is equally important to recognise that there is a high pace of technological development taking place around and the world and it could significantly impact the capability or capacity of accounting firms to embrace AI. Consequently, learning-based and data-driven systems of technological development should be developed further to make sure that the pace of development and integration continues to be at par with current trends in industry development.
It is essential to be clear about the unique characteristics of AI and its potential to solve real-time problems when integrating it in accounting processes. Kirschner and Stoyanov (2018) say that the process may be lengthy because of the long time taken to complete technological development and implementation processes. Here, AI seeks to address specific problems in the accounting profession by improving how professionals use existing tools in data analysis. However, more discussions and engagement among technology and accounting experts need to be fostered to come up with the right applications of AI. These discussions should help to redefine how the technology helps to solve fundamental business problems, particularly using it as the baseline for making changes.
Change can only be realised if professionals choose to be open-minded, as opposed to merely opposing AI for the sake of maintaining status quo. Therefore, as AI makes greater improvements in data analysis, the ability of human intelligence to make accounting decisions should also be significantly enhanced. Therefore, professional bodies regulating the discipline need to be adaptable to change because their standards of operation have to match market demands as environmental factors evolve. The same is also true for accounting firms that have lasted for many years because they have had to change to current market conditions to remain relevant in business. AI integration requires the same level of commitment.
Lastly, the uniqueness of AI, relative to other technologies, is its tendency to adopt a bottom-up approach where past mistakes are used to improve existing models. Traditional data analytical approaches typically relied on the top-down management structure where superiors were the main source of organisational intelligence. Although there are different types of fields associated with AI, machine learning (through pattern recognition) is the main driver for the change and hype caused by AI (Kaartemo & Helkkula 2018). By combining the need for pairing human and machine learning, computers could be effectively used to improve human thinking and enhance intelligence.
Training and Education
The views presented in this study suggest that AI would have a mixed impact on the accounting field. This ambiguity may be stemming from low levels of awareness among accounting professionals regarding the effects of AI. Consequently, the need for education and training should be emphasised because they are the best strategies to use in creating more awareness about the effects of AI. However, both techniques should not be conceived as a homogenous concept that applies to all groups of accountants because there is a distinction in the kind of assistance that existing and new professionals should acquire. Notably, education should be directed to new and younger professionals because their training curriculum should equip them with necessary skills associated with AI.
Comparatively, training exercises should target existing professionals because their training did not include AI. Therefore, they should attend workshops and seminars on AI to update their knowledge on the practice. Doing so would help to address the weaknesses of employees that are linked to poor AI adoption. Training would enable them to strengthen the skills required to implement AI, thereby minimising some of the weak links associated with its implementation.
In addition, it would improve collaboration and team effort in the workplace because attending seminars means that more employees would become knowledgeable about AI and they will be able to transfer the same knowledge to others. In this regard, there would be an overall improvement of skills and knowledge on AI, which could later be harnessed to improve organisational performance.
Lastly, training and education would improve employee performance by enabling them to acquire knowledge associated with AI. Doing so would enhance their confidence in working with the technology because they would no longer see it as a threat to their jobs but rather a tool of analysis. This strategy would also improve the importance of the professionals in the accounting field by giving them access to new and cutting-edge technology in the industry.
Future of AI
The effects of AI on the accounting profession were a significant topic of analysis in this study because the literature review findings pointed it out as a key concern among observers. Although the literature review findings suggested that the effects of AI are largely positive, the respondents sampled in this review presented mixed reviews of the issues. In other words, an almost equal number of people thought that job losses would occur compared to those who thought such an outcome would not suffice. However, of importance to this study was the emergence of a biased view of the findings because the respondents alluded to massive low-level job losses.
Therefore, people who worked simple tasks are the most vulnerable to retrenchment compared to their peers who hold high-ranking positions. Consequently, it could be assumed that job losses are unlikely to occur from supervisory to senior-level positions because this group of employees carry out complicated tasks, which machines cannot do. However, jobs that require a direct analysis of data or simple commands can be easily replaced.
The above findings suggest that AI may complicate or simplify organisational structures depending on how managers reconfigure their work processes. For example, a simplified organisational structure may emerge when lower ranking jobs are minimised or eliminated and higher-level positions maintained. Since fewer positions exist at the top, it is possible for managers to coordinate organisational functioning using a leaner organisational structure. However, firms that may resist change and want to maintain their original organisational structures may experience conflict because people tasks may conflict with machine functions.
Several researchers have explored the impact of AI in other industries and found that it will not be significantly as disruptive to organisational processes as much as automation did from the 1950s to the 1980s (Frude & Jandrić 2015). The fear of job losses mostly come from reports which predict a “doomsday scenario” when AI is implemented. For example, an article by Fuatx (2019) suggested that there would be massive unemployment in the US because up to 46% of jobs in the country are at risk of being eliminated by AI. These reports have fanned fears but they fail to take into account the contextual factors that influence job outcomes.
For example, the nature and characteristics of the industry involved is a significant determinant of the effects of AI because some sectors are more vulnerable to automation than others are. For example, the manufacturing sector is susceptible to job losses because of AI. Comparatively, service-oriented businesses, such as accounting, may not have such type of exposure.
Broadly, the views of the respondents highlighted in this study suggest that the use of algorithms to undertake accounting functions cuts into a space that has never been explored before. This approach means that there is going to be an unprecedented application of logic in accounting process. However, human intelligence is not often dependent on logic because its main function is to safeguard a company’s vision. Logic and vision are two key parts of organisational processes that need to be nurtured to realise the overall long-term vision of the company. Notably, the two need to be implemented concurrently to complete organisational tasks.
This is because the use of one technique could be problematic for organisations that intend to use both qualitative and quantitative aspects of process reviews. Therefore, it is unlikely that one of the functions would be substituted for the other. Consequently, people should not be concerned about job losses in accounting, which may arise from the implementation of AI.
The uniqueness of the accounting sector as a wing of investment decision-making should guide future discussions on AI because its potential advantages could significantly improve current performance. The increased efficiency and accuracy of AI output reported in multiple studies supports this assertion because decisions made using artificial intelligence networks are better in terms of timeliness and accuracy (Bechmann & Bowker 2019). Future applications of AI should focus on educating and training stakeholders about its advantages to eliminate the stigma associated with it. Doing so should prepare professionals to work alongside machines.
It is difficult to predict a future where the accounting profession will be immune from the influences of AI technology because it is an inherently analytical discipline and machines are good at that. Indeed AI could be a powerful data analytics tool. Therefore, it can be naturally integrated with the accounting profession. The potential increase in the quality of output that could be reported from its use could only be imagined for now because few accounting firms admit to using AI and even fewer can comprehensively understand how it can influence their operational plans.
The evidence gathered from the literature review and expert opinions suggest that multinational accounting firms, such as Deloitte, were early adopters of the technology and are using it to undertake complicated tasks, such as analysing client agreements (Rana 2018; Carriço 2018). This analogy means that medium and smaller accounting firms are yet to realise the benefits of AI because they have not acquired the experience of working with it.
Capital and infrastructural requirements of AI could be impeding efforts to use the technology extensively across different subsectors of the accounting practice but this problem could be solved in the future through increased investments and awareness of the benefits of AI. Technological advancements in the AI sector could also fan this development by making expertise cheaper to obtain in the same way as computers have become accessible to the average person. Overall, concerns about the future of AI need to be disregarded because they are based on the lack of a proper understanding of its potential implications on practice.
Summary
This chapter shows that the findings of this paper align with the views of other researchers who have investigated the impact of AI on different aspects of business performance in the sense that they both present a mixed review of its impact on accounting. This problem is exacerbated by the minimal levels of awareness about the technology. However, education and training could help to change this situation.
Conclusion and Recommendations
Conclusion
From the onset of this study, the possible effects of AI on corporate performance was established as a significant area of business analysis, relative to its potential to impact human resource performance in the short, medium and long-term. The focus on the accounting field was necessitated by rapid developments in the industry, particularly in the areas of data analytics, which favour the use of machine learning as opposed to human intelligence. The review was skewed towards investigating the research phenomenon from a training and education perspective because most of the challenges associated with AI integration stemmed from a poor awareness of the technology in the industry.
Furthermore, observations were made to suggest that current professionals lack the requisite skills to thrive in a post-AI world. Key to these discussions was the long-term viability of human labour in the accounting profession. This topic of discussion underscored the development of the first research question, which sought to find out how developments in AI would influence performance among accounting professionals.
The respondents sampled in this study presented mixed reviews about this study topic but their general sentiments suggested the possibility of massive job losses at the lower end of the work continuum. They did not conceive a situation where such job losses could occur in higher-level positions because they were more attuned to human intelligence through different relationship-based competencies, such as leadership and communication. Therefore, there is a potential mixed impact of AI on the performance of accounting professionals.
A key part of the findings suggests that there have been insignificant changes in employee attitude towards AI, which have occurred in the past five years. In other words, most accountants are deemed to have negative or neutral attitudes towards AI because of their lack of experience with the technology. This problem could be addressed through increased seminars to educate professionals about the technology. By doing so, there would be a greater awareness of the technology, which would allow meaningful discussions to take place thereafter.
However, since few steps have been taken to realise this outcome, the integration of AI in the accounting sector may take a long time. The process should ideally involve meaningful discussions among stakeholders about its potential ramifications on the practice but awareness needs to be created first. This is why the focus of this paper was on training and education.
This area of analysis was analysed because of its potential to influence accounting professionals to change their attitudes about the technology. There seems to be a larger and growing trend among stakeholders to achieve this goal because many employers are organising workshops that would equip their employees to learn about current changes in the industry. Furthermore, professional accounting bodies, such as the Association of Chartered Certified Accountants (ACCA), are already organising workshops where such issues are being discussed. Consequently, accountants should have a strong focus on purpose if they are to effectively exploit the advantages of AI.
Stated differently, people’s understanding of AI needs to shift from a methodological review to a goal-oriented perspective. Doing so would change people’s understanding of AI as a threat to a tool for achieving organisational outcomes or meeting accounting objectives. This transition will be in alignment with the nature of accounting because the profession is not an end unto itself; instead, it is a tool for helping stakeholders to make informed financial decisions about a company.
The integration of AI into accounting processes should help stakeholders to underpin their investment, growth and business decisions. The development of more intelligent systems will enable stakeholders to make fundamentally different decisions regarding their corporate plans and in turn expand the effectiveness of accounting. AI has the potential to significantly improve the quality of decisions made by investors because it increases their confidence and trust in the financial decisions made by companies, which are properly audited.
For example, tax compliance, resource allocation and expenditure control are some areas of corporate governance that could benefit from this progress. It is important to make these changes because they support the realisation of organisational objectives. Accountancy is an important tool for making sure the process happens smoothly.
The use of AI can help to transform new problems using new data by creating intelligent systems of analysis. However, it is important to recognise that a lot of work needs to be done to make this vision a reality. At the very least, there should be an effective criteria for allocating resources, which are vital in making informed choices. AI-aided technologies would help to promote accountability when making these decisions. Furthermore, it is important to understand that the focus should always be on identifying important decisions that could be influenced by the use of AI and position them to effectively exploit the advantages of the technique.
The above insights highlight the importance of exploiting the advantages of new technology. This study highlights the strengths of machine learning in the accounting profession not only because they are expanding new areas of research but also because they are sufficiently addressing some of the perennial problems noted in accounting. However, technology is complex and its integration in the accounting profession may be problematic, subject to privacy concerns and the general mistrust that some professionals have towards it.
The findings of this study will be instrumental in building a positive image of the future, especially regarding the integration of AI and human intelligence. The goal is to minimise the scepticism associated with AI and focus more on the probability or potential of the technology to solve perennial accounting problems and enhance existing professional skills to address current challenges in the field.
Overall, based on the findings generated from this study, it is important to acknowledge the difficulty in correctly predicting the effects of AI on the accounting field because many factors have to be considered in evaluation. Furthermore, the field of analysis is too broad to understand all the variables that have to be evaluated to come up with correct predictions. Therefore, the long-term trajectory of the accounting field will ultimately shape how professionals choose to interact with machines. Furthermore, a myriad of social, economic and political factors will have to be evaluated to come up with a correct prediction of how this relationship will be formed. The kind of AI technology that will be available in future and people’s understanding of it will also change in coming years, thereby highlighting the need to be adaptable to change.
Recommendations
Overall, to comprehend the challenges and potential ramifications of AI in the accounting field, it is essential to understand that AI equips machines with the ability to think. In other words, AI centres on the development of intelligent machines. While there are reports and criticisms regarding the role of this concept in improving or ending human existence, this paper focused on a scientific perspective of its integration with human intelligence in the accounting field. The findings suggested that there are low levels of awareness of AI and a low capacity to adopt new changes. These issues could be addressed by implementing the following recommendations.
Compulsory Training
Based on the insights provided in this paper; there is a strong need for compulsory training of accounting professionals about AI. The training and education requirements need to be made compulsory through a decree or legislative amendment because professionals have to be compelled to be versant with the technology. Indeed, it would be difficult to exploit its benefits if accountants are ignorant about its implications and yet it will not only change the sector but also others related to it. For example, the need for compulsory training is supported by the double-faceted nature of the accounting field because it is often pegged with auditing. Training and education would help to master both conceptual and practical aspects of AI and its relationship with the discipline.
Compulsory training of accounting professionals means that they need to gain access to the right skills that would help them to exploit existing opportunities for development. The starting point should be in the acquisition of technical skills linked to machine learning. However, similar to other skills and competencies implemented in business contexts, accountants also need to understand the context of these skills and their potential to align with today’s contemporary globalised business environment. The need to acquire these skills and competencies rests in the understanding that accounting roles are already changing and adapting to the capabilities that exist in the data analysis sector.
Accountants should exploit some of the advantages associated with using advanced data analytical techniques because their conventional training processes already include an understanding of numerical data, which could be easily combined with their awareness of business dynamics to create a perfect blend of strategy that would improve the business’s goals, vis-a-vis existing accounting strategies.
Relative to the above assertion, Birtchnell (2018) says that some training procedures will need to emphasise technical accounting expertise, but others need to lay emphasis on the need for human intelligence to solve complex issues. Nonetheless, organisations should make it a point to increase the scope of collaboration between accountants and other professionals as well as business departments. This effort will improve synchrony in task assimilation across multiple levels of organisational control. The AI trend is supposed to accelerate this process because it has the potential to integrate different aspects of a company’s operational tasks to create a bigger and more coherent operational plan. In this regard, different professionals in an organisation (not only accountants) will derive the right meanings from the existing data and models.
Generally, accountants need to develop new skills and competencies that are aligned to AI development if they are to maximise the potential that this technology holds. For example, dedicating most of their time to predictive and proactive learning would help them to stay ahead of the curve in terms of leveraging AI potential in their careers. Here, they could put predictions in context or develop capabilities that would enable them to change their course quickly because AI technologies support such a future in business. Broadly, new ways of thinking are needed to make this happen.
Improve Capacity for AI Absorption
Some of the respondents interviewed in this study claimed that AI needs to be reviewed within the institutional setting because professionals and policy makers regulate rules and procedures of accounting. In this regard, there is a need for institutions involved in regulating accounting practices to adapt to these new technologies, otherwise it would be difficult to reap their benefits, as most professionals would be bound by traditional accounting regulations and policies that do not support integration.
However, regulation is often slow to recognise innovation and correctly adapt to its potential. Therefore, there could be many missed opportunities for AI integration in the accounting profession because of fixed regulations and rules. Consequently, there needs to be an expanded capacity for regulators to understand the potential positive ramifications of AI on the accounting profession for its advantages to be truly understood or conceptualised.
The need for better integration between human and machine intelligence is necessitated by the fact that the sum of both is better than individual applications. Research studies conducted in the areas of chess and medicine affirm this view (Cortese & Walton 2018; Ferri et al. 2018). Nonetheless, it is equally vital to understand that AI developments are still ongoing and they may move to complicated areas of decision-making, which may lead to job losses.
However, it is unlikely that specific human capabilities, such as leadership and creativity will be substituted using technology. Furthermore, it is unwise to present a “dooms day” scenario concerning the adoption of AI in the accounting field because human beings are extremely resilient and adaptable to new situations. Therefore, it is unlikely that a permanent crisis will be created because of the adoption of AI in the accounting field. However, decisions involving human judgement may be significantly changed through the adoption of AI because the latter has access to more superior data, thereby making it difficult for accountants to compete with it. Therefore attempts at suppressing the use of Ai in accounting, simply to protect the viability of traditional accounting processes, may fail because AI may supersede the need for human judgement in a majority of the cases.
Reference List
Allana, S. and Clark, A (2018) Applying Meta-Theory to Qualitative and Mixed-Methods Research: A Discussion of Critical Realism and Heart Failure Disease Management Interventions Research. International Journal of Qualitative Methods 17.1 pp 1-10.
Amnuai, W (2019) Analyses of Rhetorical Moves and Linguistic Realizations in Accounting Research Article Abstracts Published in International and Thai-based Journals. SAGE Open 9.1 pp 1-10.
Ando, H., Cousins, R. and Young, C (2014) Achieving Saturation in Thematic Analysis: Development and Refinement of a Codebook. Comprehensive Psychology 3.1 pp 1-10.
Archibald, M. M., Radil, A. I., Zhang, X. and Hanson, W. E (2015) Current Mixed Methods Practices in Qualitative Research: A Content Analysis of Leading Journals. International Journal of Qualitative Methods 14.2 pp 5-33.
Armborst, A (2017) Thematic Proximity in Content Analysis. SAGE Open 7.2 pp. 1-10.
Aveling, E. L., Gillespie, A. and Cornish, F (2015) A Qualitative Method For Analysing Multivoicedness. Qualitative Research 15.6 pp 670-687.
Bechmann, A. and Bowker, G. C (2019) Unsupervised by any Other Name: Hidden Layers Of Knowledge Production In Artificial Intelligence On Social Media. Big Data & Society 6.1 pp 1-10.
Birtchnell, T (2018) Listening Without Ears: Artificial Intelligence in Audio Mastering. Big Data & Society 5.2 pp. 1-10.
Bovin, A. J (2019) Breaking the Silence: The Phenomenology of the Female High School Band Director. Update: Applications of Research in Music Education 4.1 pp 1-10.
Brayda, W. C. and Boyce, T. D (2014) So you Really Want to Interview Me?: Navigating “Sensitive” Qualitative Research Interviewing. International Journal of Qualitative Methods 13.1 pp 318-334.
Brewer, J. D (2016) The Ethics of Ethical Debates in Peace and Conflict Research: Notes towards the Development of a Research Covenant. Methodological Innovations 9.1 pp 1-10.
Carriço, G (2018) The EU and Artificial Intelligence: A Human-Centered Perspective. European View 17.1 pp 29-36.
Chong, J. I (2014) Popular Narratives versus Chinese History: Implications for Understanding an Emergent China. European Journal of International Relations 20.4 pp. 939-964.
Chun Tie, Y., Birks, M. and Francis, K (2019) Grounded Theory Research: A Design Framework for Novice Researchers. SAGE Open Medicine 7.1 pp. 1-10.
Clarke, A. M., Chambers, D. and Barry, M. M (2017) Bridging the Digital Disconnect: Exploring the Views of Professionals on Using Technology to Promote Young People’s Mental Health. School Psychology International 38.4 pp 380-397.
Collins, C. S. and Stockton, C. M (2018) The Central Role of Theory in Qualitative Research. International Journal of Qualitative Methods 17.1 pp 1-10.
Colnerud, G (2014) Ethical Dilemmas in Research In Relation To Ethical Review: An Empirical Study. Research Ethics 10.4 pp. 238-253.
Cortese, C and Walton, P (2018) Histories of Accounting Standard-Setting – An Introduction. Accounting History 23.3 pp. 261-264.
Crossen-White, H. L (2015) Using Digital Archives in Historical Research: What Are the Ethical Concerns for A ‘Forgotten’ Individual? Research Ethics 11.2 pp 108-119.
Dahbi, S., Ezzine, L. and Moussami, H (2017) Modeling of Cutting Performances In Turning Process Using Artificial Neural Networks. International Journal of Engineering Business Management 9.1 pp 1-10.
Dahlin, E (2019) Are Robots Stealing Our Jobs? Socius 5.1 pp 1-10.
de Block, D. and Vis, B (2018) Addressing the Challenges Related to Transforming Qualitative Into Quantitative Data in Qualitative Comparative Analysis. Journal of Mixed Methods Research 4.1 pp 1-10.
Dixon, S. and Quirke, L (2018) What’s the Harm? The Coverage of Ethics and Harm Avoidance in Research Methods Textbooks. Teaching Sociology 46.1 pp. 12-24.
Doll, J. L (2018) Structured Interviews: Developing Interviewing Skills in Human Resource Management Courses. Management Teaching Review 3.1 pp. 46-61.
Dudhwala, F. and Larsen, L. B (2019) Recalibration in Counting and Accounting Practices: Dealing with Algorithmic Output in Public and Private. Big Data & Society 6.2 pp. 1-10.
Ebneyamini, S. and Sadeghi, M. R (2018) Toward Developing a Framework for Conducting Case Study Research. International Journal of Qualitative Methods 17.1 pp. 1-10.
Elo, S., Kääriäinen, M., Kanste, O., Pölkki, T., Utriainen, K. and Kyngäs, H (2014) Qualitative Content Analysis: A Focus on Trustworthiness. SAGE Open 4.1 pp. 1-10.
Felzmann, H., Villaronga, E. F., Lutz, C. and Tamò-Larrieux, A (2019) Transparency You Can Trust: Transparency Requirements For Artificial Intelligence Between Legal Norms and Contextual Concerns. Big Data & Society 6.1 pp. 1-10.
Fenwick, T. and Edwards, R (2016) Exploring the Impact of Digital Technologies on Professional Responsibilities and Education. European Educational Research Journal 15.1 pp. 117-131.
Ferri, P., Lusiani, M. and Pareschi, L (2018) Accounting for Accounting History: A topic modeling approach (1996–2015). Accounting History 23.2 pp. 173-205.
Fox, S (2018) Domesticating Artificial Intelligence: Expanding Human Self-Expression through Applications of Artificial Intelligence in Prosumption. Journal of Consumer Culture 18.1 pp. 169-183.
Frude, N. and Jandrić, P (2015) The Intimate Machine – 30 years on. E-Learning and Digital Media, 12.3 pp. 410-424.
Gammie, E., Allison, M. and Matson, M (2018) Entry routes into the Institute of Chartered Accountants of Scotland training: Status versus sustainability. Accounting History 23.1 pp. 14-43.
Geiger, R. S (2017) Beyond Opening up The Black Box: Investigating the Role of Algorithmic Systems in Wikipedian Organizational Culture. Big Data & Society 4.2 pp. 1-10.
Hannes, K. and Parylo, O (2014) Let’s Play it Safe: Ethical Considerations from Participants in a Photovoice Research Project. International Journal of Qualitative Methods 13.1 pp. 255-274.
Herdlein, R. and Zurner, E (2015) Student Satisfaction, Needs, and Learning Outcomes: A Case Study Approach at a European University. SAGE Open 5.2 pp. 1-10.
Hilton, L. G. and Azzam, T (2019) Crowdsourcing Qualitative Thematic Analysis. American Journal of Evaluation 4.9 pp.1-10.
Ignatow, G (2018) Beyond Bourdieu: From Genetic Structuralism to Relational Phenomenology. Contemporary Sociology 47.1 pp. 42-43.
Jackson, J. L., Kay, C. and Frank, M (2015) The Validity And Reliability Of Attending Evaluations Of Medicine Residents. SAGE Open Medicine 3.1 pp. 1-10.
Jerolmack, C. and Khan, S (2017) The Analytic Lenses of Ethnography. Socius 3.1 pp. 1-10.
Kaartemo, V. and Helkkula, A (2018) A Systematic Review of Artificial Intelligence and Robots in Value Co-creation: Current Status and Future Research Avenues. Journal of Creating Value 4.2 pp. 211-228.
Kelly, M. G (2019) A Map Is More Than Just a Graph: Geospatial Educational Research and the Importance of Historical Context. AERA Open 5.1 pp. 1-10.
Kirschner, P. A. and Stoyanov, S (2018) Educating Youth for Nonexistent/Not Yet Existing Professions. Educational Policy 2.6 pp. 1-10.
Lazzini, A., Iacoviello, G. and Ferraris F. R (2018) Evolution of Accounting Education in Italy, 1890–1935. Accounting History 23.1 pp. 44-70.
Levine, D (2019) Automation as Part of the Solution. Journal of Management Inquiry 28.3 pp. 316-318.
Liu, X., Keane, P. A. and Denniston, A. K (2018) Time to Regenerate: The Doctor in the Age of Artificial Intelligence. Journal of the Royal Society of Medicine 111.4 pp. 113-116.
Lowman, J. and Palys, T (2014) The Betrayal Of Research Confidentiality In British Sociology. Research Ethics 10.2 pp. 97-118.
Lubet, S (2019) Accuracy in Ethnography: Narratives, Documents, and Circumstances. Contexts 18.1 pp. 42-46.
Mann, S (2017) Big Data is a Big Lie without Little Data: Humanistic Intelligence as a Human Right. Big Data & Society 5.2 pp 1-10.
McKinsey & Company (2019) The Promise and Challenge of the Age of Artificial Intelligence. Online. Web.
Miranti, P (2014) The Emergence Of Accounting As A Global Profession – An Introduction. Accounting History 19.1 pp. 3-11.
Murphy, B. and Quinn, M (2018) The Emergence Of Mandatory Continuing Professional Education At The Institute of Certified Public Accountants in Ireland. Accounting History 23.1 pp. 93-116.
Napitupulu, I. H (2018) Organizational Culture in Management Accounting Information System: Survey on State-owned Enterprises (SOEs) Indonesia. Global Business Review 19.3 pp. 556-571.
Naujokaitiene, J., Tereseviciene, M. and Zydziunaite, V (2015) Organizational Support for Employee Engagement in Technology-Enhanced Learning. SAGE Open 5.4 pp. 1-10.
Newmahr, S. and Hannem, S (2018) Surrogate Ethnography: Fieldwork, the Academy, and Resisting the IRB. Journal of Contemporary Ethnography 47.1 pp. 3-27.
Nijam, H. M. and Jahfer, A (2018) IFRS Adoption and Value Relevance of Accounting Information: Evidence from a Developing Country. Global Business Review 19.6 pp. 1416-1435.
Nowell, L. S., Norris, J. M., White, D. E. and Moules, N. J (2017) Thematic Analysis: Striving to Meet the Trustworthiness Criteria. International Journal of Qualitative Methods 16.1 pp. 1-10.
Oleinik, A (2019) What are Neural Networks Not Good At? On Artificial Creativity. Big Data & Society 6.1 pp. 1-10.
Oranye, N. O (2016) The Validity of Standardized Interviews Used for University Admission into Health Professional Programs: A Rasch Analysis. SAGE Open 6.3 pp. 1-10.
Pakdemirli, E (2019a) Artificial Intelligence In Radiology: Friend Or Foe? Where Are We Now And Where Are We Heading? Acta Radiologica Open 8.2 pp. 1-10.
Pakdemirli, E (2019b) New Subspecialty or Close Ally Emerging On the Radiology Horizon: Artificial Intelligence. Acta Radiologica Open 8.3 pp. 1-10.
Persson, M. E., Radcliffe, V. S. and Stein, M (2018) The Pursuit of A Cognitive Standard for the Accounting Profession. Accounting History 23.1 pp. 71-92.
Ralph, N., Birks, M. and Chapman, Y (2014) Contextual Positioning: Using Documents as Extant Data in Grounded Theory Research. SAGE Open 4.3 pp. 1-10.
Rashid, Y., Rashid, A., Warraich, M. A., Sabir, S. S. and Waseem, A (2019) Case Study Method: A Step-by-Step Guide for Business Researchers. International Journal of Qualitative Methods 18.1 pp. 1-10.
Rana, R (2018) How Artificial Intelligence Will Impact the Accounting Industry? Online. Web.
Rashid, M., Caine, V. and Goez, H (2015) The Encounters and Challenges of Ethnography as a Methodology in Health Research. International Journal of Qualitative Methods 14.5 pp. 1-10.
Rule, P. and John, V. M (2015) A Necessary Dialogue: Theory in Case Study Research. International Journal of Qualitative Methods 14.4 pp. 1-10.
Saunders, B., Kitzinger, J. and Kitzinger, C (2015) Anonymising Interview Data: Challenges and Compromise in Practice. Qualitative Research 15.5 pp. 616-632.
Scarth, B. J (2016) Bereaved Participants’ Reasons For Wanting Their Real Names Used In Thanatology Research. Research Ethics 12.2 pp. 80-96.
Stano, P., Kuruma, Y. and Damiano, L (2018) Synthetic Biology and (Embodied) Artificial Intelligence: Opportunities and Challenges. Adaptive Behavior 26.1 pp. 41-44.
Strandmark, M (2015) Method Development at Nordic School of Public Health NHV: Phenomenology and Grounded Theory. Scandinavian Journal of Public Health 43.16 pp 61-65.
Steen, J., DeFillippi, R., Sydow, J., Pryke, S. and Michelfelder, I (2018) Projects and Networks: Understanding Resource Flows and Governance of Temporary Organizations with Quantitative and Qualitative Research Methods. Project Management Journal 49.2 pp. 3-17.
Sterne, J. and Razlogova, E (2019) Machine Learning in Context, or Learning from LANDR: Artificial Intelligence and the Platformization of Music Mastering. Social Media and Society 5.2 pp. 1-10.
Stevenson, L., Power, D., Ferguson, J. and Collison, D (2018) The Development Of Accounting In UK Universities: An Oral History. Accounting History 23.1 pp. 117-137.
Stutz, C. and Sachs, S (2018) Facing the Normative Challenges: The Potential of Reflexive Historical Research. Business & Society 57.1 pp. 98-130.
Sugiura, L., Wiles, R. and Pope, C (2017) Ethical Challenges In Online Research: Public/Private Perceptions. Research Ethics 13.3 pp. 184-199.
UMSL (2019) Qualitative Research Designs. Online. Web.
van Manen, M (2017) But Is It Phenomenology? Qualitative Health Research 27.6 pp. 775-779.
Wagner, C., Kawulich, B. and Garner, M (2019) A Mixed Research Synthesis of Literature on Teaching Qualitative Research Methods. SAGE Open 9.3 pp. 1-10.
Walker, C. and Baxter, J (2019) Method Sequence and Dominance in Mixed Methods Research: A Case Study of the Social Acceptance of Wind Energy Literature. International Journal of Qualitative Methods 18.1 pp. 1-10.
Zhang, B. and Dafoe, A (2019) Artificial Intelligence: American Attitudes and Trends. Online. Web.
Fuatx, K (2019) How AI Will Impact Organisational Structures. Online. Web.
Miller, R (2019) Ai in Business – Why Human Expertise Continues To Be Needed. Online. Web.
Appendix 1
Interview questions
- What role do you hold in your organisation? What does it involve?
- Have you been doing this job since you graduated? What was your previous work experience like? (the reason for changing; effects of AI on different periods, how to adapt to the changing business landscape)
- With the development of artificial intelligence, how do you think it will affect the industry based on your professional field or working environment? Prompt for challenge and benefits.
- Do you think this will affect recruitment processes in your organization?
- As we know, many jobs have been replaced by artificial intelligence robots. What skills do you think employees need to have to keep their jobs even as companies embrace AI? How do you think these skills should be acquired or nurtured?
- Do you think artificial intelligence could replace professional personnel to some extent? What kind of work can be finished by AI and what cannot be accomplished?
- Will development in AI require higher education?
- Will developments in AI change the organization structure?
- What kind of changes or revolutions should be made in the next 2, 5 and 10 years?
- Accounting course curriculums; accounting certification (teachers)
- Financial system; accounting certification; recruitment; professional training (accountants)
- Professional training; management system; recruitment; (CEO, CFO, AI technology manager and HR manager
- Is there anything else about AI, professional education and training that you think is important?