Statistical Test
The data analysis intends to employ SPSS in performing principal component analysis (PCA). Particularly, PCA aims to reduce the number of variables into principal components that explain most of the variation in SPSS anxiety among students. According to Field (2013), PCA eases exploration of data by establishing patterns, linear relationships, and the influence of each variable. In essence, PCA eliminates redundant variables and promotes dimensionality of variables (Jackson, 2015). For robust PCA, data ought to meet the assumptions of the multiplicity of continuous variables, the linearity of data, sample adequacy, and the absence of significant outliers. Elliott and Woodward (2015) elucidate that PCA applies orthogonal transformation in converting raw variables that highly correlate to principal components with low correlation coefficients. Thus, PCA enables the determination of principal components with major influence on the variation of data.
The Basis of Data
The data emanated from the questionnaire developed and utilized in collecting data regarding SPSS anxiety among 2571 students. The questionnaire comprises 23 closed-ended questions with a five-point Likert scale answers indicating the strength of agreement from 1 (strongly disagree) through strongly agree (5) (Field, 2013). Since students experience anxiety in the course of learning SPSS, the questionnaire aims to measure and ascertain the extent of SPSS anxiety. Through the interview of students with and without anxiety, the study formulated 23 questions with viability to measure SPSS anxiety. The examination of data indicates that they meet the assumptions of PCA because the questions are 23, variables have linear relationships, data do not have significant outliers, and the sample size is more than 300 (N = 2571). The use of the questionnaire would indicate the extent of anxiety in each student learning SPSS. Moreover, the questionnaire would reveal forms of SPSS anxiety existing among students. In essence, the study aims to use PCA in revealing principal variables that explain most of SPSS anxiety among students.
Research Questions
- What are the principal components that account for the variation in SPSS anxiety among students?
- What are the dominant themes in the extracted components?
Hypotheses
- H0: The 23 questions in the questionnaire are not statistically significant variables in explaining the variation in SPSS anxiety among students.
- H1: The 23 questions in the questionnaire are statistically significant variables in explaining the variation in SPSS anxiety among students.
Statistical Outcomes
Descriptive Statistics
The exploration of the data using descriptive statistics indicates that there are no missing data because 2571 students answered all the Likert items in the questionnaire. Denis (2016) asserts that descriptive statistics form the basis of data analysis for they reveal patterns and trends of data. Descriptive statistics also show that students neither agree nor disagree with most Likert statements (18) because their mean scores ranged from 2.23 to 2.89 as demonstrated in Table 1. Moreover, students agree with the remaining four Likert statements for their mean scores ranged from 3.16 to 3.62.
Table 1.
Assumption Tests
The study employed the correlation in testing the assumption of multicollinearity. The scrutiny of the correlation matrix shows that the 23 Likert items have both negative and positive relationships (Appendix A). The correlation analysis indicates that the relationships of the Likert items range from moderate to very weak positive and negative relationships. Further scrutiny of significance coefficients shows that all Likert items have statistically significant relationships with one or more Likert items (p < 0.001). According to Field (2013), Likert items with correlation coefficients greater than 0.9 exhibit multicollinearity. Therefore, as correlation coefficients are less than 0.6, it implies that no variables exhibit multicollinearity, and thus, no elimination of Likert items.
Regarding the assumption of the adequacy of sample size, KMO statistic (0.93) indicates that the sample size is adequate for principal component analysis (Table 2). Since KMO statistic is considerably greater than the threshold of 0.5, Field (2013) describes the adequacy of sample size as marvelous. Anti-image correlation matrices (Appendix B) indicates that individual OKM values of anti-image covariance and anti-image correlation are greater than 0.5, which means all Likert items are viable for PCA. As illustrated in Table 2, Bartlett’s test of sphericity is statistically significant (p = 0.000), and thus, it rejects the null hypothesis that the correlation matrix is similar to the identity matrix.
Table 2.
Factor Extraction
From Table 3, it is apparent that four factors out of 23 factors have eigenvalues greater than one while the remaining 19 factors have eigenvalues less than one
Table 3.
Before extraction, factors 1, 2, 3, and 4 had eigenvalues of 7.290, 1.739, 1.317, and 1.227, which accounted for 31.696%, 7.560%, 5.725%, and 5.336% of variance in that order. After extraction, factors 1, 2, 3, and 4 had the same eigenvalues that explained the same proportion of variance respectively. However, after extraction, factors 1, 2, 3, and 4 had eigenvalues of 3.730, 3.340, 2.553, and 1.950, which accounted for 16.219%, 14.523%, 11.099%, and 8.476% of variance correspondingly. Overall, the four factors cumulatively accounted for 50.317% of the variation in SPSS anxiety among students.
The table in Appendix C shows that shared variance of factors ranged from 0.343 to 0.739. The mean of common variance is 0.5032 (11.573/23), which is less than Kaiser’s threshold of 0.7. Component matrix (Appendix D) shows the distribution of factors’ loadings into the four components selected. According to Field (2013), factors with loadings greater than 0.4 are significant while factors with loadings less than 0.4 are not significant.
The scree plot demonstrates that the four components explain most of the variation in eigenvalues. According to McCormick, Salcedo, Peck, Wheeler, and Verlen (2017), the elbow of the scree plot provides a threshold of components in which further additions have no significant impact on the variation of data. Thus, the scree plot confirms the extraction of the four components in PCA.
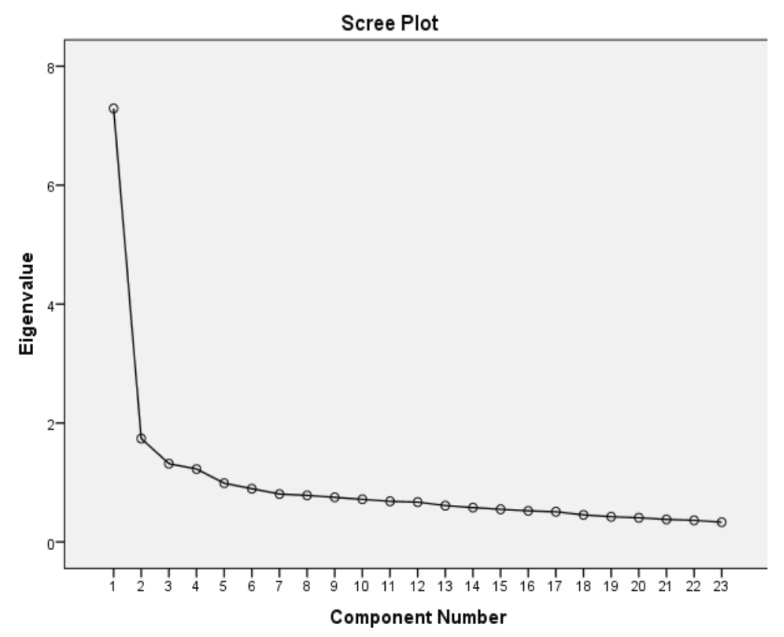
The analysis of loadings in the rotated component matrix (Appendix E) shows themes of anxiety that are in each component. Pallant (2016) holds that the threshold of suppressing loading coefficients determines the emergence of these themes. The type of anxiety that loaded onto the first component relates to the fear of computers while the form of anxiety that loaded onto the second component relates to the fear of statistics. The same number of questions (8) loaded onto the second and third components. The questions that loaded onto the third component relate to the fear of mathematics whereas the questions that loaded onto the fourth component relate to the fear of social perception.
Interpretation
PCA was performed to determine which among the 23 Likert items considerably explain SPSS anxiety among students. The analysis showed the data met the assumptions of sample adequacy (OKM = 0.93), the absence of multicollinearity (r < 0.6), and significance of Bartlett’s test of sphericity. Factor extraction established four components with eigenvalues greater than one. Moreover, the four eigenvalues collectively accounted for 50.317% of the variation in SPSS anxiety among students. The scree plot confirms the extraction of the four components because the major inflection point occurred at the fourth factor. The analysis of how each question loaded onto different components revealed varied themes in the questions. Eight questions that loaded onto the first component relate to the fear of computers while another eight questions that loaded onto the second component relate to the fear of statistics. Whereas three questions that loaded onto the third component relate to the fear of mathematics, the remaining five questions that loaded onto the fourth component relate to the fear of social perception. Thus, in answering the research question, PCA shows that fears of computers, statistics, mathematics, and social perception are principal factors in the questionnaire that influence the occurrence of anxiety among students. Moreover, PCA rejects the null hypothesis for it demonstrates that the 23 questions in the questionnaire are statistically significant variables in explaining the variation in SPSS anxiety among students.
References
Denis, D. (2016). Applied univariate, bivariate, and multivariate statistics. Hoboken, NJ:Wiley.
Elliott, A. C., & Woodward, W. A. (2015). IBM SPSS by example: A practical guide to statistical data. Thousand Oaks, CA: SAGE Publications.
Field, A. (2013). Discovering statistics using IBM SPSS statistics (4th ed.). Los Angeles, CA: SAGE Publications.
Jackson, S. J. (2015). Research methods and statistics: A critical thinking approach (5th ed.). Belmont, CA: Cengage Learning.
McCormick, K., Salcedo, J., Peck, J., Wheeler, A., & Verlen, J. (2017). SPSS statistics for data analysis and visualization. Indianapolis, IN: Wiley.
Pallant, J. (2016). SPSS survival manual: A step by step guide to data analysis using IBM SPSS. Sydney, Australia: Allen & Unwin.