Problem Statement
With the speedy growth of urban settings globally, the municipal waste remains a tenacious matter facing cities worldwide. Currently, the amount of generated refuse in many urban settings is nearly stretching out of control, and the numbers are expected to swell following consistent development in urban centers (Johnson et al. 3).
Efficient waste management remains a vital service to partly attain viable, livable cities since the collection and dumping of refuse influence carbon emanations, traffic jamming, quality of air, and considerable investment in operating expenditures (Kontokosta et al. 151). Local authorities need to develop innovative strategies that seek to establish efficiency in desired systems of waste management to lower the volumes of waste taken to landfills. Such new methods can also grow the diversion rates through composting and recycling programs. Historical and real-time data collection and translation coupled with inventive strategies such as applying machine learning arithmetical methods facilitate data-oriented interventions in tackling persistent challenges in city waste management.
Goals and Objectives
This research aims to forecast daily and weekly tonnage of municipal garbage generation across New York City using sophisticated data mining capabilities and other technological approaches such as machine learning. Statistical analysis remains an immense strategy in the planning and management of various waste collection systems. The desire for exemplary determination and a directed urban refuse administrative plan remains indispensable in lowering the prospect of adverse ecological effects resulting from municipal garbage (Kontokosta et al. 152). New York City has all of its refuse collected through an integrated network of contractors who use an amalgamation of direct haul, long trucks, and trains to transport and dispose of them in designated landfills.
The operational budget allocated by the city to ensure the provision of waste collection and disposal run in billions of dollars. Despite the high financial implication involved in waste collection, inefficiency remains a concern. Therefore, like other metropolises, New York City requires robust urban waste management structures for effective waste forecasting to enable excellent service rendition to residents. Specific objectives of the investigation include:
- To embed technology such as machine learning in monitoring and predicting waste generation volumes for efficient, dependable urban refuse management systems.
- To employ historical data on municipal waste provided by the city’s sanitation department to predict refuse generation for enhanced facilitation of services in a financially sustainable and environmentally sound way.
Scope
The study has a three-pronged scope in attempts to realize its core objective. First, it cultivates close partnership with the responsible agency to provide detailed data on waste operations and challenges. In this scenario, New York City’s department of sanitation provided historical data on refuse collection spanning from 2003 to 2005. The submitted data sets comprise collection information for a solitary truck, including a unique vehicle number, tonnage, and time (Johnson et al. 4). It also encompassed the nature of collected material (paper, plastic, glass, metal, or refuse) and the geospatial data of the assortment point.
Second, reviewing and translating the breadth and depth of the provided historical information on the urban waste, spanning to a nearly ten-year period for vigorous statistical analysis and robust model cross-validation (Johnson et al. 4). Data modeling and analysis were performed by the gradient boosting technique, allowing the historical data to be assessed both spatially and temporally to accurately predict weekly tonnage generations for all the selected locations across the city. Lastly, the research shall entail the utilization of Gradient Boosting Regression theory to estimate both space and time for the generated waste in New York City.
State-of-the-Art Review
Numerous approaches have been applied to quantify waste products, including multiple regression techniques, group comparison, time-series analysis, correlation assessment, dynamic system modeling, and input-output analysis.
The above models emphasize the identification of underlying connections between elements, driving garbage production. For example, in the municipal stage, research by Oribe-Garcia recognized tourism activity, educational status, income, and urban morphology as key influencing aspects, resulting in a high waste generation (Johnson et al. 3). Daskalopoulos also established through one regression analysis the linkage of total consumer expenditure and gross domestic product as the leading factor in increased waste production at the country-based level (Johnson et al. 4). The model gives accurate quantification and forecasting of municipal waste.
Previous pieces of literature have also documented ways of improving urban waste management structures by applying diverse dynamics and data-driven modeling approaches to forecast refuse generation. Such approaches have also proved useful in identifying factors that expound waste and recycling attributes. Explicitly, research employing temporal models using lagged refuse data has shined for forecasting and prediction in big portions as a result of time series auto connection noticeable in waste produced at local or regional level (Kontokosta et al. 152). The absence of literature is trying to prefigure waste production for individual structures in larger municipalities.
Challenging aspects are data constraints since few sanitation authorities gather and avail granular refuse collection figures (Kontokosta et al. 152). Moreover, small region approximation challenges can confound efforts to accurately downscale prediction efforts to exactly downscale predictions from the town or locality to individual houses.
Methodology
For efficient and accurate data analysis for enhanced predictability, the employed the simulated gradient boosted regression formula in estimating temporary production. The model functions based on the decision tree regression concept because of its interpretability and competence in managing complex and non-linear relations between data, guaranteeing higher forecasting accuracy (Johnson et al. 7). Spatiotemporal tonnage data was also pooled with other external data sets to construct a Gradient Boosting Model, which further anchored in the past data extending from 2004 to 2015 to permit both temporal and even spatial validation.
Data Collection
- The research incorporated data on the waste collection that spans over a decade, with every record containing information for each truck.
- Data on total weekly refuse tonnage were recorded in all sections of the metropolis.
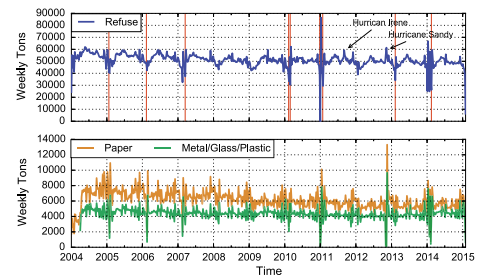
Data Presentation
The research is aware of the significance of municipal waste projections due to their indispensable role in managing left-overs. Reliable and effective prediction gives city authorities the capacity to boost waste collection, dumping works and establish long-term plans for disposal strategies (Kontokosta et al. 152). The research presents various mediums, including charts, tables, and graphs for adequate visualization and understanding by stakeholders. Data were also resampled to a weekly average to match the temporal frequency of the refuse generated figures.
Data Analysis
Overall, the data collected through the designed model shows the capability to forecast waste production with temporal accuracy and is highly granular across many streams of refuse. The collected and analyzed data is critical for improved planning, better decision-making, and enhanced operations (Johnson et al. 5). Furthermore, separate geographies manifest their gathering timings of either three or two days a week; the researchers aggregate the daily gatherings data to compare with the issued historical ones based on the mutual temporal gauge.
Inference/Conclusion
The study has contributed to creating a transitory analytic framework for refuse production in the city. Using data sets drawn from various sources coupled with earlier garbage gathering statistics from the town’s sanitation unit, the researcher established a predictive model for waste management. Moreover, simulation through the gradient boosting method was built to calculate weekly refuse data across multiple streams with an 88 percent accuracy rate.
Next Step
First, the research has provided much-needed insights for the metropolis establishments to initiate testing as well as comprehend the effectiveness of trash management interventions. Second, the findings in the study can be implemented in developing incentive arrangements to shove individuals to change their waste dumping behavior. Single valuing approaches, for example, “charge as you dump,” can trigger equity apprehensions (Johnson et al. 9).
However, by perfectly forecasting individual construction waste production volumes and reconciling them with demographic variations and building populations, an enhanced equitable pricing model can be crafted. Other inducements comprising demand valuing during high-garbage periods can also be embraced. Moreover, innovative ways such as machine learning techniques can be used to inform urban planning and operations.